5 Data Science Portfolio Mistakes
When trying to get hired as a data scientist, it's not just about what you know but also what you show. In other words, effectively conveying your expertise and past work is a critical part of the process.
One of the best ways to do this is via a portfolio website. A portfolio gives you credibility and makes your work readily available to clients and hiring managers.
However, not all portfolios are equal. There are key mistakes that separate bad portfolios from good ones. In this article, I break down 5 mistakes that will guarantee your portfolio never gets you hired.
Mistake 1: Don't make a portfolio website
The worst portfolio is no portfolio.

Since most people don't have a portfolio, just having something will yield most of the benefits and give you an edge over other candidates.
However, if you've never made a website, you might be hesitant. You might worry that it'll cost money or you'll need to spend hours learning how to code in HTML and CSS.
I was in this boat too. Funny enough, it took me 8 months to make my first portfolio, not because of some tremendous cost or time commitment but because I held myself back. In fact, my first portfolio didn't cost me anything and took me about a weekend to create.
With all the free tools out there, it's never been easier to make a website. For example, in a past article, I describe a free and easy way to create a portfolio (without code) using GitHub Pages.
How to Make a (Free) Data Science Portfolio Website With GitHub Pages
Mistake 2: Include irrelevant projects
When you try to be everything to everyone, you're not right for anyone.
If you avoided mistake 1, the next step is picking the right projects to showcase. But what makes a project "right"?
An essential part of curating your portfolio is knowing your audience. Who is this for? What are they looking for? How can I directly speak to their needs?
For example, if looking for entry-level Data Science roles, pick projects that convey technical data skills (e.g. Python and SQL), and don't pick projects more suitable for software engineering or web development (e.g., javascript, HTML, etc). While the latter may be helpful in a data science role, they are not critical and are ultimately a distraction to your audience.
Additionally, choosing a niche can be beneficial here. Niching helps make you a big fish in a small pond and helps avoid the "I specialize in everything" pitch.
Even if this is true, this can make it difficult for your audience to see how you fit into their world. If the audience can't quickly connect what's on your Portfolio to their problems, they will move on to the next candidate.
Mistake 3: Cram in as many Kaggle projects as possible
1 real-world project > 10 toy projects.
A mistake made by many when choosing Projects is taking quantity over quality. This often manifests itself as portfolios full of projects using toy datasets from websites like Kaggle.
While these projects can be great educational tools, they lack essential elements of real-world data science projects, e.g. understanding the business problem, working within existing processes, and synthesizing data from multiple sources.
When trying to assess the quality of a project, focus on impact. Using impact as a filter for picking projects not only makes your work more impressive but also conveys that your work is relevant to someone in the world. Examples of impact could be: you won a hackathon, the work was published, it generated $X in savings, X number of people downloaded the project, you presented it in front of X people, etc.
For those just getting started and lacking good projects to choose from, the most obvious path is doing an independent project. While this is easier said than done, here are a few quick tips to help guide your effort.
- Tailor projects to your interests or job description or (ideally) both
- Acquire (real-world) data from an existing business or organization
- Build a web scraper or explore public APIs to aggregate data
- Contribute to open-source projects
- Find a mentor
Mistake 4: Don't include any visuals
Show don't tell.
If the worst portfolio is no portfolio. The 2nd worst is a portfolio with no pictures.
The key advantage of a website is you can embed images, gifs, and videos, to not only describe your work but to show it. Rather than trying to make this point through words, consider the gif below.
On the left, I have a text description of the project, while on the right, I simply show a demo. Which do you find more compelling?

If you don't have any pretty pictures readily available, take the time to create them. No need for anything fancy here. For example, in my portfolio, I made most of the images using Keynote (i.e. Apple's version of PowerPoint).
Mistake 5: Ignore all non-technical aspects
The data science version of "all chest, no legs."
It's easy to get caught up in all the cool and exciting technical aspects of data science while virtually ignoring all other non-technical aspects.
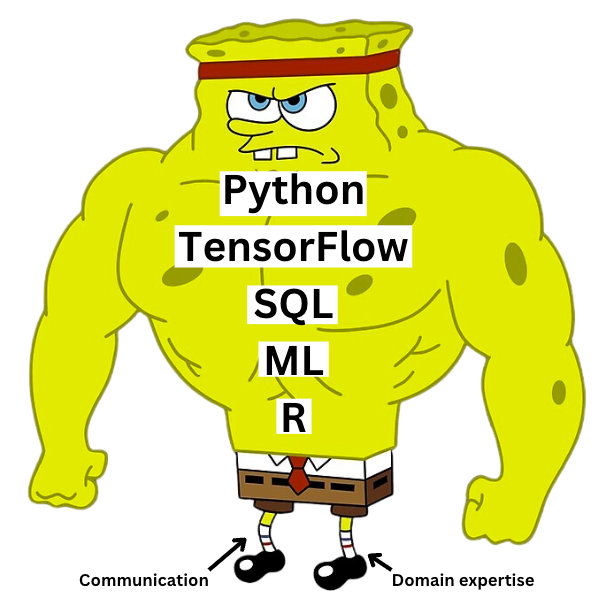
Although technical skills are absolutely critical, non-technical aspects such as domain knowledge and communication skills are also important. This is especially true if hiring is done by a non-technical audience (which is often the case at smaller organizations without a dedicated data science team).
One simple thing that can help is listing out all the domains in which you've worked. Additionally, having video or written content in which you are presenting a project or technical topic can help convey those critical communication skills.
Resources
Connect: My website | Book a call
Socials: YouTube