A fAIry tale of the Inductive Bias
|INDUCTIVE BIAS| TRANSFORMERS| COMPUTER VISION|

As we have seen in recent years deep learning has had exponential growth both in use and in the number of models. What paved the way for this success is perhaps the transfer learning itself-the idea that a model could be trained with a large amount of data and then used for a myriad of specific tasks.
In recent years, a paradigm has emerged: transformer (or otherwise based on this model) is used for NLP applications. While for images, vision transformers or convolutional networks are used instead.
On the other hand, while we have plenty of work showing in practice that these models work well, the theoretical understanding of why has lagged behind. This is because these models are very broad and it comes difficult to experiment. The fact that Vision Transformers outperform convolutional neural networks by having a theoretically less inductive bias for vision shows that there is a theoretical gap to be filled.
This article focuses on:
- What exactly is inductive bias? Why this is important and what inductive bias do our favorite models have?
- The inductive bias of transformers and CNNs. What are the differences between these two models and why these discussions are important?
- How can we study inductive bias? How to be able to leverage the similarity between different models in order to capture their differences.
- Can a model with weak inductive bias succeed in the same in computer vision? a field where inductive bias is traditionally believed to be important instead.
What is inductive bias?

Learning is the process of apprehending useful knowledge by observing and interacting with the world. It involves searching a space of solutions for one expected to provide a better explanation of the data or to achieve higher rewards. But in many cases, there are multiple solutions which are equally good. (source)
Imagine encountering a swan in a lake. From this simple swan, we might assume that all swans are white (until we see a black swan), that they are waterfowl, that they feed on fish, and so on.
This process is called inductive reasoning. From a simple observation, we may be able to derive thousands (if not billions) of hypotheses, and clearly, not all of them are true. In fact, we might think that the swan is incapable of flight since we only observe it swimming at that time.
Obviously, it is difficult to be able to decide which hypothesis is correct without direct observations. So according to Occam's principle, we could state "Swans can swim in lakes."
Why is this important for machine learning?
A dataset is a collection of observations, and we want to create a model that can generalize from these observations. The idea is that starting from our dataset we can infer some rules that are also valid for the general population. In other words, we can consider our model as a set of hypotheses.
In theory, the hypothesis space is infinite. In fact, if we consider two points in Cartesian space it can pass a straight line but infinite curves. Without more points, we cannot know which hypothesis is the most correct.
Generally, the simplest hypothesis is the most correct one. A curve that fits the points perfectly is generally overfitting.

Inductive bias can be defined as the prioritization of certain hypotheses (thus reducing the hypothesis space). For example, when there is a regression task we decide to consider linear models, at this time we are reducing our hypothesis space by allowing our hypotheses to be only linear.

An inductive bias allows a learning algorithm to prioritize one solution (or interpretation) over another, independent of the observed data (source)
On the one hand, we have different types of data, and we have different types of models with different assumptions and different inductive biases (i.e., different reductions in the hypothesis space). One might therefore be tempted to choose one model for all types of data.
In 1997, though, the no-free lunch theorem ended this temptation. No one model can work for all situations. In fact, there is no optimal bias that allows a model to generalize for all tasks. In other words, assumptions in a model that may be optimal for one task may not be optimal for another.
This is one reason why we use convolutional neural networks for images, RNNs (or LSTMs) for text sequences, and so on.

To better understand here are some examples of inductive bias:
- Decision trees are based on the assumption that a task can be solved by a series of binary decisions (binary splits).
- Regularization, the assumption is directed to solutions where the parameters have small values.
- Fully connected layer. There is an all-to-all bias where all the units of layer i are connected with the following layer j (all the neurons in one layer are connected to the next layer). Which it s meaning there is a very weak relational bias since any unit can interact with other units.
- Convolutional neural networks are based on the idea of locality where the features are drawn using local pixels and are combined in hierarchical patterns. In another world, we are assuming that pixels that are near are actually related and this relationship should be considered by the model (during the convolutional step).
- Recurrent neural networks have a bias related to sequentiality since each word is processed in sequence. There is also temporal equivariance (or recursion) because the weights are reused for all the elements of the sequence (we update the hidden state).
- Transformer. It has not a strong inductive bias, which should provide more flexibility (at the cost of high data for training). In fact, in a low data regimen, the model is performing worst than others.

The inductive bias of CNN and transformer

Convolutional neural networks for a long time dominated computer vision, until Vision Transformers came along. As we mentioned above, CNNs are based on the principle that neighboring pixels have a relationship. Therefore during convolution, several pixels share the same weight.
Moreover, the use of the pooling layer is used to achieve translational invariance. Which is meaning that a pattern is recognized wherever it is in the image (for example, a face that is at the left or right corner of an image)
These biases are very effective for processing natural image data because there is high covariance within local neighborhoods, which diminishes with distance, and because the statistics are mostly stationary across an image. (source)
These biases actually were inspired by the inferior temporal cortex, which seems to provide the corresponding biological for scale, translation, and rotation invariance. These biases were considered important for the CNN to be resistant to changes in image translation, scaling, or other deformations and therefore incorporated through convolution and pooling.

On the other hand, images are complex and information-rich objects. Given their use, an attempt was made to understand in more detail what CNNs see and what other biases are present.
In a 2017 study, the authors showed that Inception models (a type of CNNs) have a strong "shape bias." In other words, CNNs rely more on the shape of an object to recognize it than on other types of patterns. The authors used an image triplet to classify an object and used an image that had the same color but a different shape (color match) or the same shape but different color (shape match) to study whether the pattern gave more importance to shape or color.

In a later study, some authors showed instead that more than color is a texture that is important for the model. The authors used ResNet50 to test this hypothesis.

They showed that in case of texture-shape conflict the model tends to use texture. so for the authors, CNNs have a strong "texture bias"
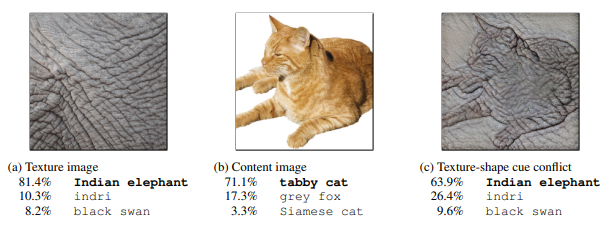
The authors conclude, however, that models that have a shape bias are more robust:
Remarkably, networks with a higher shape bias are inherently more robust to many different image distortions (for some even reaching or surpassing human performance, despite never being trained on any of them) and reach higher performance on classification and object recognition tasks. (here)
So actually, for images, it would be desirable to have shape bias. This can be achieved by using either the appropriate dataset or by using data augmentation techniques that include color distortion, noise, and blur (which precisely decrease texture bias). While conversely, random cropping increases texture bias.

So concretely we can say that the bias depends not only on the architecture for a CNN but also on the dataset with which it is trained. Depending on the dataset a CNN acquires a bias toward either shape or texture.
The authors of a study state that these biases are complementary. The model can then focus on either texture or shape for prediction. Sometimes, however, only one of these two elements is not enough for correct prediction (reduces performance). The authors state that as the model can learn either bias it can also "automatically figure out how to avoid being biased toward either shape or texture from their training samples." In other words, using examples that are conflicting (for texture and shape) can instruct the model not to have bias.

The Vision transformer is derived from the transformer, and as mentioned above it is a model that does not have a strong bias.
Some studies have shown that there are still several similarities between CNNs and ViTs. In fact, ViTs also learn a layer-by-layer hierarchical view that can also be visualized.

A later study though suggests that ViTs actually have a higher shape bias than CNNs. Which is actually surprising. Moreover, the authors point out how this shape bias plays a positive role in robustness to image corruption:
[…] highlight a general inverse relationship between shape bias and mean corruption error. As a model is more robust to common corruptions (smaller mCE), its shape bias increases. (source)

Several groups hypothesized that adding adequate inductive bias might have allowed ViTs to outperform CNNs even without having to train them with millions of images. On the one hand, this hypothesis led to the creation of so many models but made training extremely inefficient.
So remains the question:
how much of the lack of inductive bias can be compensated by scaling of parameters and the number of training examples?
How to study inductive bias?
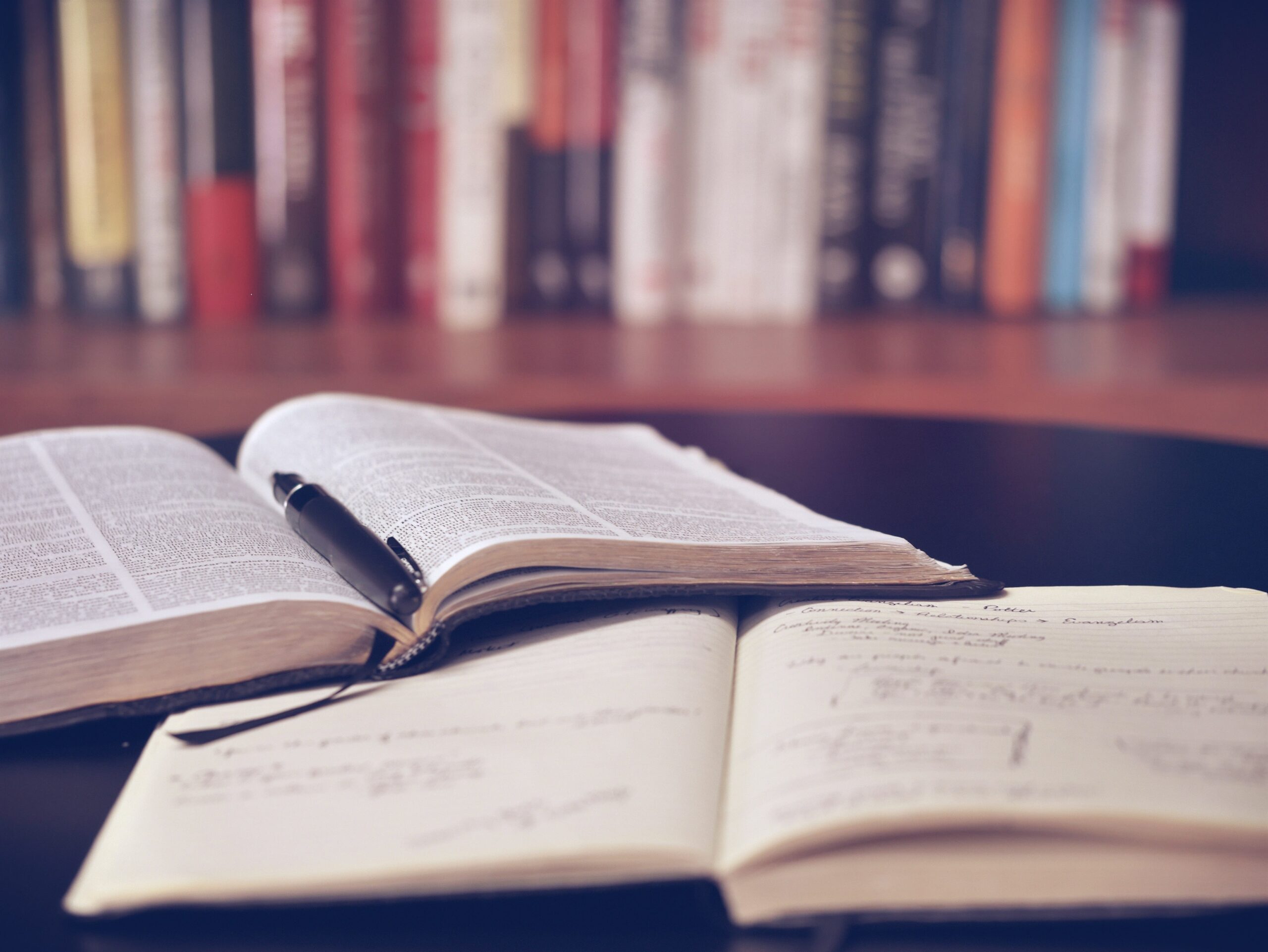
As we have seen, several open questions remain. Although there are a great many studies on CNNs and ViTs, many points of theoretical background behind these advances in performance remain obscure.
"MLPs are the simplest of these neural network architectures that hinge on this stacking idea, and thus provide a minimal model for an effective theory of deep learning." (source)
In general, many such studies on more theoretical aspects are conducted using multi-layer perceptrons (MLPs). This is because it is a layer composed of simple matrix multiplications encapsulated in a nonlinear function. Its simplicity allows many experiments to be conducted at a low computational cost. Studies then conducted on simpler models are then translated to more complex and sophisticated models. MLP has inferior performance in many settings, though, leaving open how much of what is seen can then be carried over to models that have far superior performance.
On the other hand, MLP has another advantage, it has a weak inductive bias. Which makes it a good candidate for ViT studies. There is also a derivative model that has even less inductive bias: MLP-Mixer

Intriguingly, MLP-Mixer uses neither convolution nor self-attention. Instead, it relies on multi-layer perception layers that are either applied to spatial locations or feature channels. All this is thanks to the clever usage of matrix multiplication and non-linearity.
Briefly, patches are linearly projected into an embedding space (then transformed into tabular data that can be harnessed by MLP). After that, we have a series of mixer layers. The input enters and is transposed, after which we have a simple fully connected layer. This layer identifies features that are common in the patches (aggregating channels). Then the result is transposed and a second fully connected layer to identify features in the patches themselves (associating it with the channel).
In addition, there are also skip connections, GELU as a non-linear function, and layer normalization. In addition, the authors comment:
Our architecture can be seen as a unique CNN, which uses (1×1) convolutions for channel mixing, and single-channel depth-wise convolutions for token mixing. However, the converse is not true as CNNs are not special cases of Mixer. (source)

Another interesting relationship is that convolution can be seen as a special case of an MLP, where the matrix of W weights is sparse and has shared entries. This sharing of the weights does in fact cause the learning to be spatially localized (as we mentioned above about the spatial bias of convolution).
Considering a matrix W, an image 2x3x1 pixels, and a filter f of size 2×2 this relationship becomes clear:

This has the advantage of making the model translation invariant, sacrificing the robustness of MLPs if there are permutations in the image.
But what about the Vision Transformers?
There is also a close relationship between ViTs and convolution (despite having the same biases). In fact, as shown above, self-attention layers process images in a similar way to convolution layers. In a 2020 paper, the authors show how self-attention layers can express any convolutional layer.
So as we said there are strong relationships between MLP, MLP-mixer, convolutional networks, and Vision Transformer. While these models have strong differences in inductive bias and how they process images.

In summary, since there are strong relationships and correspondences between the various models, but also differences between induction bias, we can use MLP as a simple model to understand whether the lack of inductive bias can be compensated by scaling and examples in the training set.
David against Goliath

In a recent paper, they did exactly that. They took MLP, a model that is simple in structure anyway and tried to understand what was happening with scaling. Can scaling improve the performance of the simple fully connected layer?
The authors took an MLP and built a model where they stacked equal layers of MLPs of the same size. Taking advantage of recent literature, they added layer normalization and skip connections to see if they made the training more stable. They also created a simple architecture called inverted bottleneck, where with two weight matrices they expand and collapse in the same block the input:

On the one hand, it is true that these additions increase the inductive bias but compared to modern complex architectures this is negligible. After that, they decided to explore what happens when comparing [MLP](https://en.wikipedia.org/wiki/Multilayer_perceptron) with other models in computer vision tasks (where generally MLP performance is far inferior).

The authors tested these architectures with interesting results on some popular computer vision datasets:
- the MLP standard goes directly into overfitting.
- Adding data augmentation marginally improves performance.
- Using bottleneck increases performance. Using data augmentation with an inverted bottleneck has a significantly higher impact (about 20 % in performance gain).
- Despite this, ResNet18 has far superior performance.
These data are in line with the literature, where it is stated that with a small sample size (after all, these datasets are small) inductive bias is important. In fact, the same has been observed with ViTs and MLP mixers.
In recent years, the advantage of large models has been that they can be trained on large amounts of images and then transfer knowledge to smaller datasets (transfer learning). For this, the authors used ImageNet21k (12 million images and 11k classes). After that, they conducted fine-tuning on a new task.

The results are surprising, the model is able to transfer what it has learned about a dataset to another task. Moreover, the results are far superior to what has been seen before.
While of course pre-trained on a large quantity of data, we nevertheless want to highlight that such an MLP becomes competitive with a ResNet18 trained from scratch for all the datasets, except for ImageNet1k where performance falls surprisingly short. (source)
This confirms that MLP is a good proxy for being able to analyze transfer learning, data augmentation, and other theoretical elements. This is surprising because it is an elementary model in comparison with modern models.
Another surprising result is that using large batch sizes in training increases performance.

Generally, the opposite effect is observed. Especially in the case of CNN where one tries to preserve the performance of small batch size when training with many more examples. After all, using a small batch means doing many more gradient updates during an epoch (at the cost of longer training, though). On the other hand, large batch sizes are faster and can be split across multiple devices with decisive time gain.
In addition, some observations conducted on transformers would seem that even these wide models benefit from a larger batch size.

In general, there has been much discussion in recent years about the scaling law: according to which as parameters increase there is a highly predictable increase in performance (and a quantifiable power law follows). This scaling law has been observed for LLMs although in recent times several groups have questioned it.
Although the discussion on scaling law is still open, it is still interesting to analyze whether this is possible with simple models like MLP (after all, MLP by increasing the number of parameters should tend to overfit).
In this study, the authors also defined a family of models with an increasing number of parameters.

Indeed, MLP also seems to exhibit power-law-like behavior.

This is definitely an interesting result because it shows even a simple model like MLP can show a presumed power law.
[MLP](https://en.wikipedia.org/wiki/Multilayer_perceptron) is a model that was not designed to work with images. In fact, the authors note that MLP given its bad inductive bias is more dependent on the number of examples. So yes one can compensate for a weak inductive bias, but this requires a large number of examples.
A decidedly interesting point is that all these models were run on a single GPU. A single epoch for ImageNet21k with the largest architecture took 450 seconds on a single 24 GB GPU. In other words, these experiments can be run quickly on any commercial GPU.
The authors point out that MLPs are clearly more efficient and much larger batches can be used:
As it quickly becomes eminent, MLPs require significantly less FLOPs to make predictions on individual images, in essence utilizing their parameters a lot more methodically. As a result, latency and throughput are significantly better compared to other candidate architectures. (source)

Conclusions

Inductive bias is one of the fundamental concepts of machine learning. In general, it is one of the main reasons why depending on the type of data we choose one model and not another. Although there have been a lot of studies still there are gaps in theory.
It is intriguing to think how narrow the field of hypotheses a priori can lead to better results. That comes at a cost, though, both level of theory and model complexity. As we have seen previously trying to add inductive bias to models of ViTs has led to creating increasingly complex and computationally inefficient models.
Although MLP is an extremely simple model, it has the advantage of being computationally efficient, which is why it has been used for many studies to try to fill theoretical holes. One of the main problems is that the performance of MLP in computer vision is far inferior to other models.
Recent results show that with the right accommodations, this gap can be overcome. Also, the lack of inductive bias can be compensated for with scaling. So MLPs can be a good proxy for studying modern architectures and how they behave in different situations.
Why is all this important?
In general, the last few years of research in AI have focused on a single paradigm: more parameters, more data. There has been a new race to the percentage point of accuracy. After all, though, the architecture of the Transformer remained the same since 2017.
These huge models have a not inconsiderable training cost. In recent months a research interest in alternatives has begun to grow: both in terms of obtaining the same results with fewer parameters and in looking for an alternative to the transformer ( and its quadratic computational cost).
Welcome Back 80s: Transformers Could Be Blown Away by Convolution
In each case, academic research is being forced to chase industry-led research. Very few institutions can afford to train an LLM from scratch. Yet studies like this show that results can be obtained at scale even with simple models like MLP. This opens up very interesting perspectives to better understand model behavior and start thinking about an alternative to the transformer.
What do you think? Let me know in the comments.
If you have found this interesting:
You can look for my other articles, you can also subscribe to get notified when I publish articles, you can become a Medium member to access all its stories (affiliate links of the platform for which I get small revenues without cost to you) and you can also connect or reach me on LinkedIn.
Here is the link to my GitHub repository, where I am planning to collect code and many resources related to machine learning, Artificial Intelligence, and more.
GitHub – SalvatoreRa/tutorial: Tutorials on machine learning, artificial intelligence, data science…
or you may be interested in one of my recent articles:
The AI college student goes back to the bench
Reference
Here is the list of the principal references I consulted to write this article, only the first name for an article is cited.
- Goodman, Nelson. Fact, Fiction, and Forecast (Fourth Edition). Harvard University Press, 1983
- Battaglia et al, 2018, Relational inductive biases, deep learning, and graph networks, link
- Kauderer-Abrams, 2017, Quantifying Translation-Invariance in Convolutional Neural Networks, link
- Ritter et al, 2017, Cognitive Psychology for Deep Neural Networks: A Shape Bias Case Study, link
- Conway et al, 2018, The Organization and Operation of Inferior Temporal Cortex, link
- Geirhos et al, 2022, ImageNet-trained CNNs are biased towards texture; increasing shape bias improves accuracy and robustness, link
- Hermann et al, 2020, The Origins and Prevalence of Texture Bias in Convolutional Neural Networks, link
- Li et al, 2021, Shape-Texture Debiased Neural Network Training, link
- Ghiasi et al, 2022, What do Vision Transformers Learn? A Visual Exploration, link
- Morrison et al, 2021, Exploring Corruption Robustness: Inductive Biases in Vision Transformers and MLP-Mixers, link
- Mormille et al, 2023, Introducing inductive bias on vision transformers through Gram matrix similarity based regularization, link
- Tolstikhin et al, 2021, MLP-Mixer: An all-MLP Architecture for Vision, link
- Cordonnier et al, 2020, On the Relationship between Self-Attention and Convolutional Layers, link
- Bachmann et al 2023, Scaling MLPs: A Tale of Inductive Bias, link
- Kaplan et al, 2020, Scaling Laws for Neural Language Models, link
- Lei Ba et al, 2016, Layer Normalization, link
- He et al, 2015, Deep Residual Learning for Image Recognition, link
- Ridnik et al, 2021, ImageNet-21K Pretraining for the Masses, link
- Sharad Joshi, 2022, Everything you need to know about : Inductive bias, MLearning.ai