A Machine Learning Mobius: Can Models Learn from Each Other?
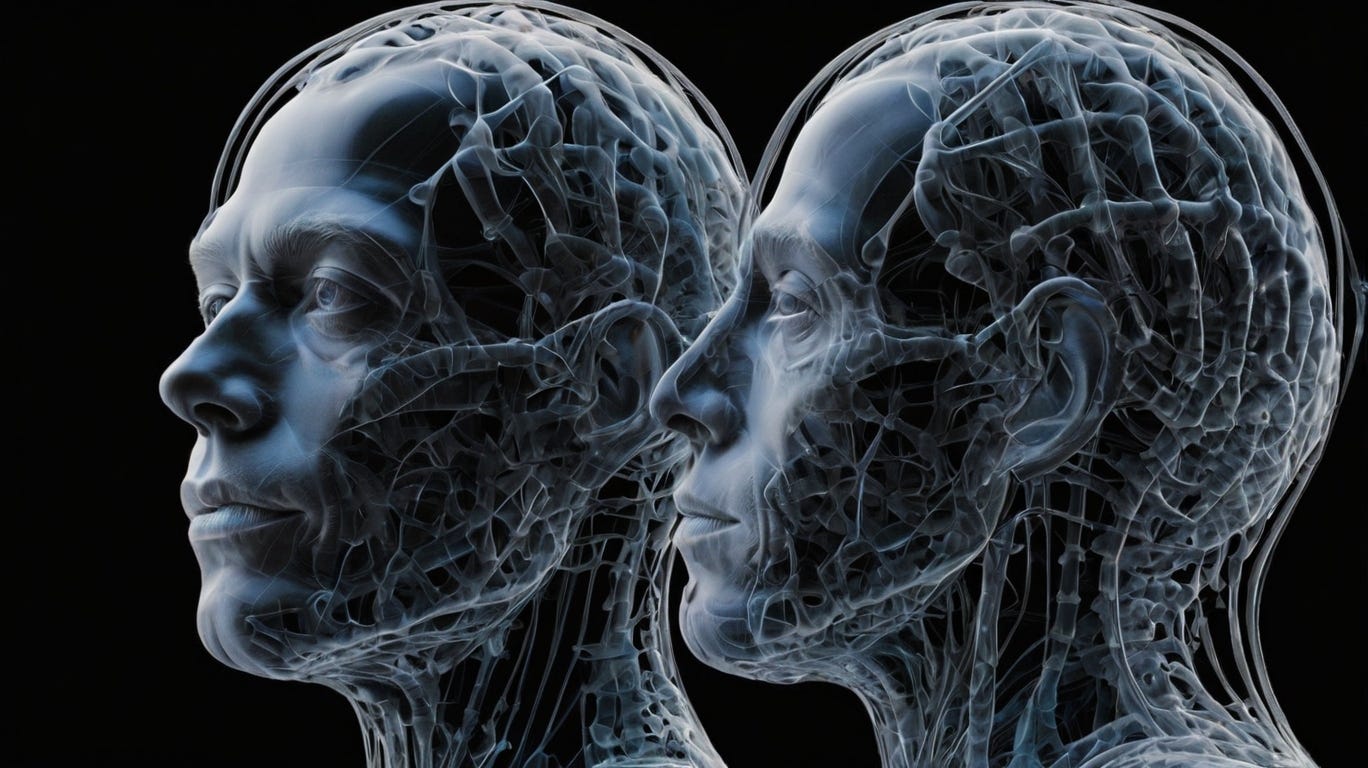
The Artificial Intelligence hype has tangible benefits for those interested in the underlying principles. The field advances at a rapid pace, and reading preliminary studies is surprisingly enjoyable. However, the downside of this tempo is the vast array of (sometimes) chaotic and incoherent terminology that accompanies it. As a result, I often revisit my decade old-lecture notes or books to refresh my knowledge gaps. This, combined with my desire to experiment with newer findings, has led to my renewed interest in the intersection of human-machine learning.
Neural networks, the foundation of modern artificial intelligence, draw inspiration from the human brain's architecture. Revisiting this fact led me to a seemingly basic question: Can machines learn from each other in ways analogous to human learning?
While this topic isn't novel – indeed, it's the very basis of neural networks – the broader implications, from dystopian scenarios to the excitement fueled by cutting-edge AI demonstrations, are mesmerizing. Beyond this latent feeling of AI autocatalysis, my question carries some immediate relevance. Two intertwined issues emerge. First, many data scientists acknowledge the growing challenge of data scarcity as current methods approach their limits, raising the question of how to progress when human-generated data is exhausted. Second, there's the issue of accessibility, particularly relevant to tinkering enthusiasts, academia and generally smaller entities. The challenge lies in making AI technology more accessible in terms of resources and applications. Currently, training a foundational LLM is beyond the reach of individuals or small businesses due to hardware and data constraints. Researchers face similar challenges in competing with well-funded companies. To rephrase the question with this in mind: How can AI be democratized and made accessible to all?
In the course of this article, I want to examine a new twist on existing Machine Learning paradigms, shedding some light on potential paths for artificial self-evolution without relying on human-generated data. With that, I'll address how small companies and individuals can make use of this field, exploring avenues for applications that don't require vast resources or datasets.
This perspective focuses on collaborative learning through model interaction, rather than on implementation details of specific techniques or summarizing the broader landscape of fine-tuning approaches. As a software engineer currently working on data-related projects, I frequently research and evaluate short-lived proof of concepts. My initial interest in integrating Retrieval-Augmented Generation (RAG) into one of my projects led me to explore beyond fine-tuning and RAGs. While this piece offers a limited view, I hope it provides value and that readers might discover something new in the field of AI and machine learning.
For those interested in details, related studies and their associated GitHub repositories (where available) are listed in the appendix.
Quick note on the title: Believe it or not, it came from a random chat with a friend about Möbius loops. My brain made this weird jump to Machine Learning Models being stuck in their data bubbles and… voilà! This title was born. Now I can't shake this silly connection. I know, it's ridiculous, but here we are.
Transfer Learning with Synthetic Data
When approaching the question of human-inspired learning naively, there is the obvious question if more capable models can teach smaller ones. The next immediate thing that pops into mind is, if yes, what's the benefit?
These naive questions are actually a pretty hot topic at the time of writing. As big LLMs are trained in incomprehensible massive data centers on shady amounts of data and consuming corresponding amounts of energy, the industry behind this acknowledges the inherent problems. Training of foundational models is limited in multiple dimensions, we might not have the energy to scale to the use cases, or we might run out of available data.
I'm aware of at least one study predicting that if the current way of training continues, models will be trained on the complete stock of public human data by 2026–2032. Of course, these predictions should be taken as that.¹
From a naive viewpoint, using large models to train smaller ones mirrors real-life teaching scenarios. The big model acts as a teacher, guiding one or more smaller models, much like a classroom dynamic in school. This approach naturally raises questions: Is it effective? What are the advantages?
The initial response is encouraging: Yes, it works, with benefits that vary depending on the specific application and goals.
Prologue: Learning Paradigms
Machine learning employs diverse learning paradigms that shape how models learn. Foundational approaches like supervised learning form the basis of a hierarchical system. Besides scaling models, architectures and data mining, also novel learning paradigms continue to emerge.
In recent years, practical approaches have shifted from using highly specialized models to larger generalists, to compound systems like RAGs, and now pivot to agentic architectures. I'd argue the field is searching for use cases, leading to a more system-design oriented approach. Such trends are reflected in the changing landscape of learning methods. The rapid progress in machine learning creates a landscape where uniform, coherent definitions often struggle to keep pace. However, a popular umbrella term for concepts around more human-inspired learning, may be resembled in the paradigm of transfer learning. It involves leveraging knowledge gained from one task to improve performance on a different but related task. Fine-tuning, a closely related term, is often the underlying process that involves making small adjustments to a pre-trained model for a specific purpose.
The relevance of these concepts has grown with the advent of foundational models – large, versatile models trained on vast amounts of data. These models serve as a starting point for various knowledge transfer techniques, allowing for efficient use of computational resources and the creation of smaller, more efficient models for specific applications. This article could span pages summarizing the myriad of concrete techniques and their underlying paradigms, but as I'm interested in human-inspired concepts, I'll focus on the use of Synthetic Data in the context of transfer learning.
Two key methods in this space are iterative refinement, where models generate and learn from their own generated data, and distillation, where knowledge is transferred from a larger (stronger) teacher model to a smaller (weaker) student ** model. Both are applied with concrete goals in mind, for example in instruction tunin**g, where the capability of a model to follow instructions is fine-tuned. To not overload this article, I'll focus on instruction tuning to showcase one approach and walk through one self-teaching and one student-teacher example to finally come to a more recent and novel twist on these methods.
When LLMs Become Their Own Tutor
The following is a cool example of fine-tuning and artificial autodidactism in action. It's a bit different from self-reinforcement learning – no trial and error here, but it's still all about improving itself.
A particularly informative read on this subject is the 2023 paper "Self-Instruct: Aligning Language Models with Self-Generated Instructions". In the authors' own words, the study describes its approach as:
a framework for improving the instruction-following capabilities of pretrained language models by bootstrapping off their own generations
In essence, models improve their own output with a straightforward learning goal: enhancing instruction-following capability. Technically speaking, the model undergoes fine-tuning with synthetic instructions to boost its zero-shot prompting performance. The fascinating nuance lies in the instruction generation method – namely, they're created by the very model being trained, resulting in a self-improving loop. I'm not so much surprised by the idea of self-improvement, after all, auto-encoders are not far from such a concept, but the aspect of model interaction gives it a new dimension.

To be more precise without getting into excessive detail, the fundamental unit of the training data is called a task. Each task consists of three components: an instruction, optional contextual input, and the desired output. The authors differentiate between classification and non-classification tasks.
Two methods for output generation are considered, depending on the task type:
- Input-first: Similar to few-shot prompting, this method includes examples from other similar tasks.
- Output-first: This method first generates a class label for the instruction, then generates the input.
The authors argue that the output-first approach prevents biased input generation towards a single class label.
The process is bootstrapped with a seed pool of human-generated tasks. From there, iteratively more synthetic tasks are added. To maintain quality, especially in the early stages, the pipeline incorporates an additional filtering step. This step evaluates task quality using multiple metrics and heuristics.
When I first encountered this approach, I was admittedly skeptical. My skepticism grew upon learning that researchers manually assessed roughly 54% of the generated tasks as correct. Initially, it seemed counterintuitive that the model could improve when exposed to a lot of flawed examples.
However, after reading the paper's positive conclusion and reflecting on the process, my intuition shifted. I now lean towards the understanding that the quality filter's heuristics and metrics likely play a crucial role. By consistently selecting the "better" tasks and with that skewing the models' distribution, the model gradually adapts to these higher-quality examples. It remains baffling that only about half of the examples need to be of high quality.
From Llama to Alpaca: Teaching Weaker Students
Following Self-Instruct, Alpaca represents a step forward in a similar fashion. Alpaca's learning strategy uses a hierarchical process where a more capable model creates tasks for a less advanced one. It moves from self-teaching to a teacher-student approach. As of this writing, there is no published paper with more details yet.
The main goal of Alpaca, as I understand from reading the related report published on Stanford's website¹¹, is to tackle the challenge of training good instruction-following models on an academic budget. The team uses Meta's LLaMA 7B as their starting point and then employs OpenAI's text-davinci-003 to create 52K instruction-following examples.
What I find particularly clever is this hierarchical setup. They're essentially using a smarter model (text-davinci-003), potentially more costly, to teach a smaller yet more efficient one (LLaMA 7B). It seems to work well – Alpaca can match text-davinci-003 on some tasks, despite being smaller and much cheaper to train. This shows that you don't need deep pockets to develop great instruction-following models, which would be a fantastic step towards more accessible training.
But it's not all roses. From what I've gathered, Alpaca still struggles with common language model issues like making stuff up, being toxic, and reinforcing stereotypes. The model is available for non-commercial testing, and it's worth pointing out that the hosted demo was taken offline after safety and cost concerns shortly after launch¹⁸. After playing with it, I noticed that Alpaca seems particularly prone to hallucination. However, I should note that this observation might be influenced by my prior reading about the model's known issues.
Real-World Implementations
Before proceeding, I want to provide at least some practical applications of these concepts. While precise categorization is challenging, these methods typically fall on a spectrum between self-supervised learning and transfer learning. Thus, numerous variations of these approaches can be found in real-world scenarios.
I'll be upfront: I haven't personally tested this system, and I'm not here to advertise it. But it does provide a glimpse into how businesses might approach training specialized models more efficiently. It's nice to have an exemplary business approach to a business-related goal.
IBM's Large-scale Alignment for chatBots (LAB) method caught my eye as a potential solution for companies looking to create task-specific language models in a building block fashion². The approach revolves around using synthetic data and targeted alignment to enhance smaller models, potentially rivaling their larger counterparts in specific tasks.
Here's the gist of it:
- It uses a taxonomy-driven system to generate synthetic instruction data.
- This data is then fed to the model through a two-stage training protocol.
- The focus is on alignment rather than extensive pre-training.
What's particularly interesting is how this method might allow businesses to sidestep the need for massive, general-purpose LLMs when they only need models for specific tasks. It's a good example of how the concept of models teaching each other could translate into practical, cost-effective solutions in the business world.
Beyond cost reduction, these approaches excel in scenarios where human-generated data is unavailable, unsuitable, or potentially problematic. Consider the following cases:
- The "Five Finger" Problem: In image generation, models sometimes struggle with accurately depicting human anatomy. A potential solution involves artificially creating correct anatomical features, such as hands, to train away these inaccuracies.
- The Privacy Problem: Training face generation models without compromising individual privacy.
- The Copyright Problem: Developing language models without relying on copyrighted text.
While numerous examples exist for the aforementioned list, I've chosen to limit their discussion here to maintain a concise article. For those interested, additional examples can be found in the appendix. One particularly interesting case involves generating synthetic data for face recognition to address privacy concerns associated with using real human faces. I recommend reviewing the related paper in the appendix for more details.
Feynman's Twist: Learning by Teaching
Richard Feynman is widely celebrated for his approach to learning and physics. Less well-known is that Feynman was an early proponent of machine learning concepts, recognizing their potential at a time when symbolic AI was far more popular⁴. Feynman's influence becomes evident again in modern machine learning, through a twist on existing transfer learning techniques: Learning by Teaching. As someone who often finds himself pacing in circles and animatedly explaining concepts aloud (an admittedly quirky habit), I've experienced firsthand how phenomenally effective this technique can be for deepening understanding. My interest in this topic is further fueled by my personal connections with educators and professional experience dealing with algorithmic challenges in digital classrooms. While the idea of applying this concept to machine learning models might seem intuitive in hindsight, it's a relatively recent idea that I accidentally stumbled upon.
A Preliminary Study
The initial discovery of this approach was with a very recent paper from 2024 entitled "Can LLMs Learn by Teaching? A Preliminary Study", which exercises a rather novel approach to distillation. The initial problem, as the title suggests, is the question: compared to human learning through teaching, is there a comparable effect in machine learning?
The core of this approach builds on earlier ideas but adds one important element: A feedback path from student to teacher. This feedback takes the form of scores that show how well students solved exam problems similar to the example they were taught. By incorporating this feedback loop, teachers can learn from their students' performance and adjust their teaching methods. This two-way interaction allows for continuous improvement.

According to the authors, humans learn from teaching on three levels:
- Observing feedback from students
- Learning from the observed feedback
- Iteration on the previous steps
Without getting bogged down by a lot of acronyms the idea reads as follows:
- Teaching: The teacher model demonstrates a problem category by using a specific example and solution.
- Student Learning: The student solves similar problems in a context-learning fashion. Each answer given by the student receives a numerical score.
- Feedback Learning: The teacher evaluates its effectiveness by analyzing student performance. Scores of the student answers are projected on the teaching materials and serve as an evaluation metric for the teacher.
- Evaluation: The authors use either the maximum score or aggregated sum, depending on the problem type.
- Iterative Improvement: The teaching process evolves based on student performance. When students struggle, the teacher model generates new materials to address identified issues.
Thereby different approaches are proposed to make use of the student's feedback, all in the realm of using synthetic data for knowledge transfer, but this time based on the feedback data. Each approach addresses the corresponding level of learning in the human analogy.
- Search-based output generation pipeline (M1): The feedback is used directly to construct a search history of scored teaching problems that were proposed to students, based on the student's feedback. The model uses this history actively to produce more optimal output when prompted for similar problems. Notably, this approach employs no additional training of the teacher.
- Fine Tuning (M2): The teacher is fine-tuned with direct preference tuning, using the sampled feedback.
- Iterative Refinement (M3): Direct iterative improvement of the teaching materials generated by the teacher, as opposed to previous approaches where only the problem space was scaled.
The datasets for the different approaches are generally prepared for research and vary from math problems to more textual problems. They are designed to work with automatic assessment of proposed solutions, a necessary condition in this case. Again human-generated data is used to bootstrap the process, while synthetic data in the form of teaching materials and feedback scores are used for the subsequent learning.
The analysis of results involves assessing and comparing each method. However, identifying comparable studies for benchmarking remains challenging at this stage. The paper examines different teacher-student model configurations and constructs various evaluation cases based on learning approaches M1-M3. Overall, the net outcome is positive, with the teacher's answer quality showing improvement. The paper highlights several factors influencing these outcomes, with one finding which particularly stood out to me, is that teachers can improve through the process of teaching weaker students. This is counterintuitive and unexpected when compared to knowledge distillation, as discussed in previous sections, where typically a weaker model learns from a more capable model.
New Learning Tricks: Meh?
After all, it seems to me all approaches are promising considering their goal, but I'm not entirely convinced of their wider applications beyond fine-tuning. Including Feynman's way of learning is clever, but it will show if the extra hassle and cost provide more than what we already have.
When considering fine-tuning, I'm reminded of catastrophic forgetting. It's a known issue in machine learning that I think applies here too. From what I've seen, fine-tuning a model makes it great at new tasks, but it might lose some of its broader abilities in the process. The model gets good at specific skills, probably at the cost of some general knowledge. It's useful for targeted tasks, sure, but I think it's worth noting how this affects the model's overall capabilities. It could be a worthwhile trade-off but I'm wondering if this is a step towards more effective training or merely different tiny steps towards improving specific capabilities.
Final Thoughts
I feel gravitated to the concept of machines teaching machines, with its philosophical nuance fueling my curiosity. Even for those averse to statistics or math, this field resembles a high-tech playground. It's an era for tinkering, clever tricks, and unconventional approaches. For me, the method described exemplifies how ingenuity can make this field accessible and exciting to explore without innovating on the underlying math.
Further Readings & Resources
[1] Pablo Villalobos, Anson Ho, Jaime Sevilla, Tamay Besiroglu, Lennart Heim, & Marius Hobbhahn. (2024). Will we run out of data? Limits of LLM scaling based on human-generated data. https://arxiv.org/abs/2211.04325
[2] IBM Article about their LAB Project
[3] Xuefei Ning, Zifu Wang, Shiyao Li, Zinan Lin, Peiran Yao, Tianyu Fu, Matthew B. Blaschko, Guohao Dai, Huazhong Yang, & Yu Wang. (2024). Can LLMs Learn by Teaching? A Preliminary Study. https://arxiv.org/abs/2406.14629
[4] Eric Mjolsness. (2022). Feynman on Artificial Intelligence and Machine Learning, with Updates. https://arxiv.org/abs/2209.00083
[5] Florian Hartmann, Duc-Hieu Tran, Peter Kairouz, Victor Cărbune, & Blaise Aguera y Arcas. (2024). Can LLMs get help from other LLMs without revealing private information? https://arxiv.org/html/2404.01041v1
[6] Xinyun Chen, Maxwell Lin, Nathanael Schärli, & Denny Zhou. (2023). Teaching Large Language Models to Self-Debug. https://arxiv.org/abs/2304.05128
[7] Jiaxin Huang, Shixiang Shane Gu, Le Hou, Yuexin Wu, Xuezhi Wang, Hongkun Yu, & Jiawei Han. (2022). Large Language Models Can Self-Improve. https://arxiv.org/abs/2210.11610
[8] Emma Strubell, Ananya Ganesh, & Andrew McCallum. (2019). Energy and Policy Considerations for Deep Learning in NLP. https://arxiv.org/abs/1906.02243
[9] Yizhong Wang, Yeganeh Kordi, Swaroop Mishra, Alisa Liu, Noah A. Smith, Daniel Khashabi, & Hannaneh Hajishirzi. (2023). Self-Instruct: Aligning Language Models with Self-Generated Instructions. https://arxiv.org/abs/2212.10560
[10] https://github.com/yizhongw/self-instruct
[11] https://crfm.stanford.edu/2023/03/13/alpaca.html
[12] https://github.com/imagination-research/lbt
[13] https://github.com/fdbtrs/idiff-face
[14] https://github.com/tatsu-lab/stanford_alpaca
[15] Nvidia Blog about Pruning, and Distillation
[16] Yun Luo, Zhen Yang, Fandong Meng, Yafu Li, Jie Zhou, & Yue Zhang. (2024). An Empirical Study of Catastrophic Forgetting in Large Language Models During Continual Fine-tuning. https://arxiv.org/abs/2308.08747
[17] Nicholas Carlini, Jamie Hayes, Milad Nasr, Matthew Jagielski, Vikash Sehwag, Florian Tramèr, Borja Balle, Daphne Ippolito, & Eric Wallace. (2023). Extracting Training Data from Diffusion Models. https://arxiv.org/abs/2301.13188