A Proposed Perfect Package Prototype for Python Projects

Introduction
There are many options to consider and many decisions to make when laying out the structure of a Python package but once those design choices are committed to it is very difficult to make fundamental structural changes.
Also, if your users have started embedding the namespaces in their projects any changes you do make mean that those users will need to change their source code.
Therefore it is vital to make a good set of design and layout decisions for your packages at the outset.
The Problem
There are a lot of options for organising a Python package, hence it can be challenging to find the right design or at least one that will not cause issues later on.
The Opportunity
If a standard package layout can be established that addresses all the challenges and then prototyped in a skeleton project, creating future packages will be a quick and simple copy-and-paste.
The Way Forward
The requirements for the proposed perfect package prototype can be established and then a standard project structure created that addresses all of those needs.
Background
I have written many Python packages over the years and experimented and modified the project structures each time in an attempt to find my perfect structure, but every one has been imperfect in one way or another.
This led me to write down a set of requirements for my perfect package structure and to set about doing the research to find a way to achieve everything I wanted.
Requirements
- To mimic the namespace conventions of popular, professional packages (for example
from statsmodels.regression.linear_model import RegressionResultsWrapper
). - To organise the code and classes in a well structured set of folders and files.
- For the namespaces exposed to package users to reflect a well named scheme like
statsmodels.regression.linear_model
and not to slavishly follow the folder and file names. - For classes in sub-folders to be able to reference and access classes in other sub-folders and in folders higher up the folder hierarchy.
- To include a top-level folder containing Jupyter Notebooks that can be used to develop and test the package code.
- To include a top-level folder containing unit tests that are organised into sub-folders where those unit tests can discover all code and classes in the package.
- To include a top-level folder containing documentation that is automatically generated from the docstrings of the classes in all package folders and sub-folders.
- To avoid all hard-coding of the package location in the Jupyter Notebooks, Unit Tests and in any other project that accesses the package.
Proving the Solution Works
To prove the solution I created an empty project template or skeleton that contained 2 test classes –
class BaseLearner()
is a fictitious class that is designed to be the base class for a set of algorithm classes.class Fisher(BaseLearner)
is a fictitious class that will contain the code for an algorithm called "Fisher" that inherits fromBaseLearner
(which resides in a completely different part of the folder structure )
Starting at the End
Here is a preview of the completed project / package structure that addresses all of the requirements and provides outlines for the 2 test classes (noting that the Fisher
class is contained in fisher_file.py
and the BaseLearner
class is contained in base_file.py
)

So What is The Big Problem?
This structure would appear to have solved every one of the requirements, but there are some fundamental problems with this structure that require attention …
Naming Conventions
Given the package layout the code to import BaseLearner
would be
from common.base_file import BaseLearner
whereas the desired import is
from ghpackage.common import BaseLearner
Hence the naming conventions used by consumers of the package will be unintuitive and will not match standards like from statsmodels.regression.linear_model import RegressionResultsWrapper
.
Relative and Absolute Referencing
In fisher_file.py the code from ghtestpackage.common import BaseLearner
will not work because the referencing is relative at that point. i.e from ghtestpackage.common import BaseLearner
will be attempting to resolve in algorithms.ghtestpackage.common
which will not locate BaseLearner
.
There is no way to tell the import to go back up to the top level and then back down into
ghtestpackage.common
This is The Big Problem
It gets even worse than that. In an attempt to unify diverse requirements the package is attempting to be 3 different things at once:
- A package that can be externally referenced.
- An execution environment for Jupyter Notebooks.
- An execution environment for
pytest
unit tests.
The problem, or near impossibility, is that those 3 different use cases execute in different ways and start executing at different relative places.
When a package is imported into a Python program, the starting point for that package is its parent folder. That can be seen by considering the following system paths …
['c:UsersGHarrOneDrivePython ProjectsPublic-GithubPackage Structure',
'c:UsersGHarranaconda3envsproject-envpython310.zip',
'c:UsersGHarranaconda3envsproject-envDLLs',
'c:UsersGHarranaconda3envsproject-envlib',
'c:UsersGHarranaconda3envsproject-env',
'',
'C:UsersGHarrAppDataRoamingPythonPython310site-packages',
'c:UsersGHarranaconda3envsproject-envlibsite-packages',
'C:UsersGHarrOneDrivePython ProjectsPackages',
'c:UsersGHarranaconda3envsproject-envlibsite-packageswin32',
'c:UsersGHarranaconda3envsproject-envlibsite-packageswin32lib',
'c:UsersGHarranaconda3envsproject-envlibsite-packagesPythonwin']
I am currently running Anaconda and having selected my project-env
channel it can be seen from the paths that the site packages reside in c:UsersGHarranaconda3envsproject-envlibsite-packages
.
Note that this can easily be checked by running CMD.exe Prompt
from Anaconda and listing the directories in the site-packages
folder …

So when a program executes an import like from pandas import DataFrame
the referencing is starting the search in C:UsersGHarranaconda3envsproject-envLibsite-packages
and expecting to find a directory called pandas
that will contain DataFrame
.
Jupyter Notebooks behave differently. Their "home" location (or starting execution path) is the folder that contains the .ipynb
source file and all referencing in a Jupyter Notebook is relative (and downwards) from that folder.
Lastly pytest unit tests in Visual Studio Code behave differently again. The home / execution path for pytest unit tests inside VS Code is the root folder of the project.
In summary …
- A referenced package references relative to the parent of the project folder.
- A Jupyter Notebook references relative to the folder containing the notebook.
- A pytest unit test references relative to the root folder of the project.
This means that however the imports are structured in the proposed ideal package structure it will fail because it needs to work in 3 different ways in 3 different circumstances.
Solving the Big Problem
For a long time the only solution I could find to this conundrum was to directly insert a path into the import code of projects that needed to import from my packages …
This does work but it has some serious draw-backs.
Firstly this is code is very slow to execute. The first time the project is loaded and run in VS Code it takes 52 seconds on a decent i7 processor to resolve the external references and process the imports.
The next drawback is that the project called ghlibrary
is poorly structured.
For example dag_tools.py
and causal_tools.py
reside directly in the root of ghlibrary
with limited options to change that. Hence over time those source files have grown large and VS Code is slow at processing linting, tool-tips and other functions.
Also the code that adds the path has to be included in every project that accesses the package and effectively hard codes the package location. This could be moved into a config file but even then if several projects access the package and the package is moved they all need updating.
Lastly the biggest drawback is that taking this approach prevents the option of distributing the package to the wider Python community.
The home / execution folder is the package folder rather than the parent so it works differently to pandas
and pgmpy
etc. But worse than that it would not be reasonable to expect the Python community to include the manual referencing code and to incur the slow processing times.
A better alternative is required.
It is a little known fact that Anaconda can be easily modified to add additional paths which will then be automatically used to search for packages and to resolve references.
The path for site-packages
can easily be identified by executing sys.path
and we have already seen that on my computer it is C:UsersGHarranaconda3envsproject-envlibsite-packages
.
If a file is created with any name and a .pth
extension and then saved in the site-packages
folder it can have package paths added that Anaconda will automatically read and insert on start-up.
Here is what my .pth
file looks like …

This means that if ghtestpackage
is created as a sub-folder of C:UsersGHarrOneDrivePython ProjectsPackages
it will automatically be included in the search path of all Python projects and all files and classes contained in it can be referenced and imported …
- At a stroke this removes the need to hard code the addition of specific paths in all projects that use it.
- It also means there is just a single reference to the
Packages
path on the entire computer, it works in exactly the same way aspandas
and other popular packages. - If this package is distributed using
GitHub
andpip
users will download it into theirsite-packages
folder and it will all continue to work.
And all of that is resolved by adding one line of code to one file!
With the big problem solved the rest of the requirements will be much easier to achieve.
Solving the Remaining Problems
Organising the Package into Sub-Folders and Files
You may have noticed that I have named the source files base_file.py
and fisher_file.py
and likewise the sub-folders have been named base_folder
and fisher_folder
.
This has been done deliberately to illustrate the point that source files should be organised into sub-folders and files but conversely we would not want to use those names in the imports.
For example the import …
from ghlibrary.algorithms.fisher_folder.fisher_file import Fisher
… is long-winded and unintuitive. A better import would be
from ghlibrary.algorithms import Fisher
However, it is possible to have both i.e. to have full flexibility to split code hierarchically into folders and files and still control how the imports appear to a consumer of the package and this is done via the __init__.py
files that exist at each level of the package.
Starting with ghpackagealgorithmsfisher_folder
the __init__.py
file looks like this …
from .fisher_file import Fisher
is instructing the pre-processor to look in fisher_file.py
in the current folder (.
is the notation for current folder) and import it directly so the Fisher
class now exists directly in fisher_folder
rather than in fisher_file
and can be referenced from there.
At this point the import could be shortened to from ghlibrary.algorithms.fisher_folder import Fisher
which is better but still not perfect.
The next step is to provide an __init__.py
in the algorithms folder as follows …
This takes the reference added in the previous step which made Fisher
available in fisher_folder
and now makes it available in the algorithms folder.
from .fisher_folder import Fisher
is instructing the pre-processor to start in the current folder (.
says start here – in algoritms
) the go down one level into fisher_folder
and import the Fisher
class.
A consumer of the package can now use –
from ghlibrary.algorithms import Fisher
.
This solution enables classes and source code to be organised with complete flexibility and split into any combination of folders and files whilst maintaining control on how the users and consumers of the package see the namespaces and add the referencing.
To finish off the base class needs the same treatment.
In the ghpackagecommon
sub-folder the __init__.py
file looks like this …
And as the common folder does not have any further sub-folders that is it.
Consumers can reference as follows …
from ghpackage.common import BaseLearner
… even though the BaseLearner
class is stored in base_file.py
.
The project classes that have been organised hierarchically into sub-folders and files can now be referenced by a client as follows …
There is just one mystery left to unpick. Consider the source code for the Fisher
class …
The question is – how can Fisher()
which is located in ghtestpackage/algorithms/fisher_folder/fisher_file.py
import BaseLearner
using from ghtestpackage.common import BaseLearner
.
BaseLearner
is located in ghtestpackage/common/base_file.py
and hence from ghtestpackage.common import BaseLearner
should not work.
The reason it does work comes back to using the .pth
file to tell Anaconda to include C:UsersGHarranaconda3envsproject-envlibsite-packages
which is the parent folder of ghtestpackage so the reference from ghtestpackage.common import BaseLearner
is resolved from the parent folder of the the package.
At this stage the "perfect package prototype" has been created, all that remains is to test it thoroughly to prove that it works as expected.
Testing the Perfect Package Prototype
To start the proof and testing here is the source code for BaseLearner()
from ghtestpackage/common/base_file.py
and Fisher()
from ghtestpackage/algorithms/fisher_folder/fisher_file.py
…
The idea is that BaseLearner()
will end up being the base class for multiple algorithms in different folder locations, hence it is separated out into common
.
For the purposes of this demonstration the base class has a single method that should be callable from the sub-class and each class uses icecream
to output a debug message to prove that it has been called.
Testing a Jupyter Notebook Client
ghtestpackagenotebooks
contains a Jupyter Notebook called ghtest_notebook.ipynb
to satisfy my requirement that the "perfect package" structure should be able to contain notebooks to help develop and test the package code.
Here is the code in the notebook …
And here is the output …
ic| 'BaseLearner.init'
ic| 'BaseLearner.init'
ic| 'fisher.init'
ic| 'BaseLearner.test'
… thus proving that the base class and the algorithm class can be referenced, instantiated and called.
Also it should be noted that if a notebook is created in a totally separate project and the code above entered in a cell it still all works correctly.
Testing a pytest Client
Here is the code for unit testing the base class stored in ghtestbackage/unit_tests/test_base/test_base.py
…
… and here is the code from ghtestbackage/unit_tests/test_fisher/test_fisher.py
Again the referencing works because of the instruction in the .pth
file to add the parent folder of the package into the system path
The final proof is to go into the unit testing panel in VS Code, to check that the unit tests have been identified and discovered and to execute them all to check that they complete with no errors …

This is one of the key benefits of the proposed perfect package prototype –
The unit tests can be organised in sub-folders and still reference the classes and code that can be in their own sub-folder structure keeping source code and unit tests separate and distinct whilst retaining full flexibility in folder and file layout.
Creating Documentation
The last requirement relates to the generation of documentation and one approach is to ensure that modules, classes and functions have a comprehensive docstring (the stub of which can be automatically generated by VS Code).
Here is an example of a well-structured and comprehensive docstring …
A docstring like this will take time to write but it provides a complete record of what the method does, what the parameters are, what is returned, an example of how to call it and a set of contextual notes.
If this approach is taken for all the source code it is easy to automatically generate help files in HTML format using pydoc.
In the proposed project prototype there is a folder called docs
that contains makedocs.bat
…
python -m pydoc -w "..algorithmsfisher_folderfisher_file.py"
python -m pydoc -w "..commonbase.py"
All that is required is to execute makedocs
from the docs
folder and the documentation will be automatically generated from the docstrings …
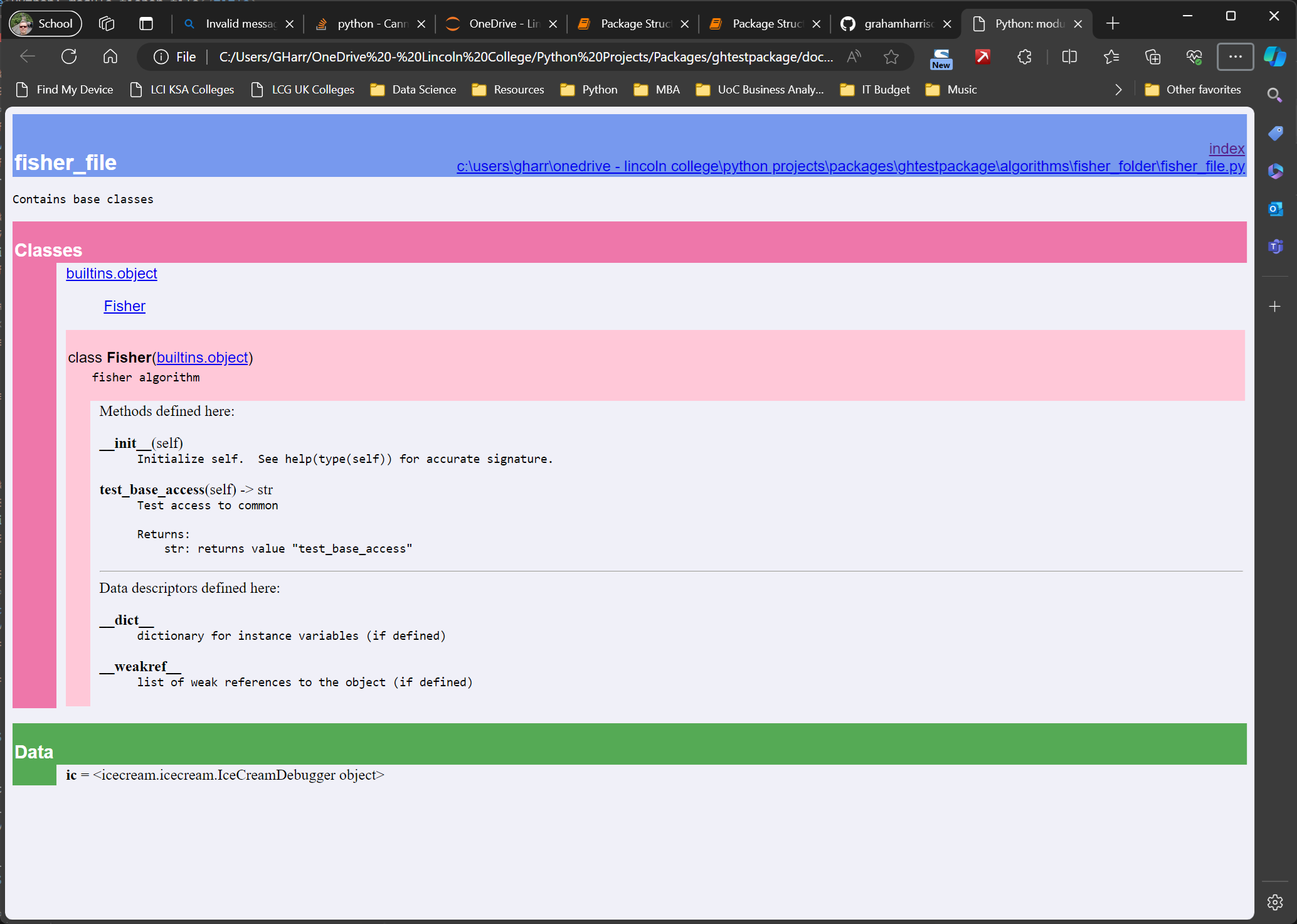
There are many different ways of writing and generating help files but pydoc has been chosen here as it provides a quick and easy way to create documentation that can explain the purpose and use of code and classes.
One Last Thing – Debugging Tests
The proposal as it stands almost works but there is one last thing …
If a unit test fails or produces unexpected results it may require debugging and in this configuration debugging will not start inside a unit test in VS Code.
If debugging is attempted the process crashes with "Invalid message: Found duplicate in "env": PATH".
The error dialog gives the option to open launch.json
which creates a launch.json
file in the .vscode
folder that looks like this …
This still does not quite work, an additional line needs adding to set the purpose
attribute as follows …
And here is the final proof – the pytest unit test called test_fisher_3()
is being debugged and is about to print "test_fisher"
to the command line …

Conclusion
The long term success of any Programming project relies on the code being well structured and well written so that the code can be build efficiently and debugged and fixed effectively when it produces unexpected results.
Also it is inevitable that at some point the code will need to be changed either by the original programmer or by someone else and the effectiveness of future maintainability requires well written code.
That in turn can only happen if the project that contains the code is well structured and well organised to provide the foundations for well written code.
As projects grow to 1000 lines of code or more, which is still a relatively modest size, if they are not distributed intelligently across multiple .py files VS Code will start to slow down and things like linting, code outlines and type-ahead will perform poorly and unreliably.
The key to all of this is to get the project structure right before the first line of code is written.
This article has laid out one proposal for a "perfect" package structure by describing a set of demanding and often conflicting requirements and then presenting a project layout and structure that has been proven through testing to meet those requirements.
The main challenges were unifying the best folder and file layout with professional looking namespaces and ensuring the classes could be imported using a common notation into standard projects, Jupyter Notebooks and pytest unit tests by configuring the .pth
file and the entries in the __init__.py
files.
There may be many other proposals for the perfect package prototype for Python projects but this one works very well.
Bonus Section: Using the Prototype Quickly and Easily …
The package prototype project can be downloaded from GitHub using this link …
… and following these steps …
- Download the project.
- Place it immediately below a parent folder that you will use to store projects for your development packages.
- Copy the
mypackages.pth
file from the docs sub-folder to thesite-packages
folder for your Anaconda installation. - Edit the
mypackages.pth
and replace the path with the path created on the local computer in step 2. - Test the Jupyter Notebook and the pytest units tests to verify the referencing and namespaces.
- Copy the skeleton project, replace
Fisher()
andBaseLearner()
with real classes and start populating the layout with production code.
If it is not clear where the site-packages
folder is on the local computer simply import sys
and run sys.path
command and look for a path that resembles the following …
C:UsersGHarranaconda3envsproject-envlibsite-packages
Connect and Get in Touch …
If you enjoyed this article please follow me to keep up to date with future articles.
If you have any thoughts or views, especially on causal inference (see my other recent articles) and where this exciting new branch of data science is going I would love to hear from you – please leave a message and I will get in touch.
My previous articles can be reviewed by Taking a quick look at my previous articles and this website brings together everything I am doing with my research and causal inference – The Data Blog.