Addressing Missing Data
In an ideal world, we would like to work with datasets that are clean, complete and accurate. However, real-world data rarely meets our expectation. We often encounter datasets with noise, inconsistencies, outliers and missingness, which requires careful handling to get effective results. Especially, missing data is an unavoidable challenge, and how we address it has a significant impact on the output of our predictive models or analysis.
Why?
The reason is hidden in the definition. Missing Data are the unobserved values that would be meaningful for analysis if observed.
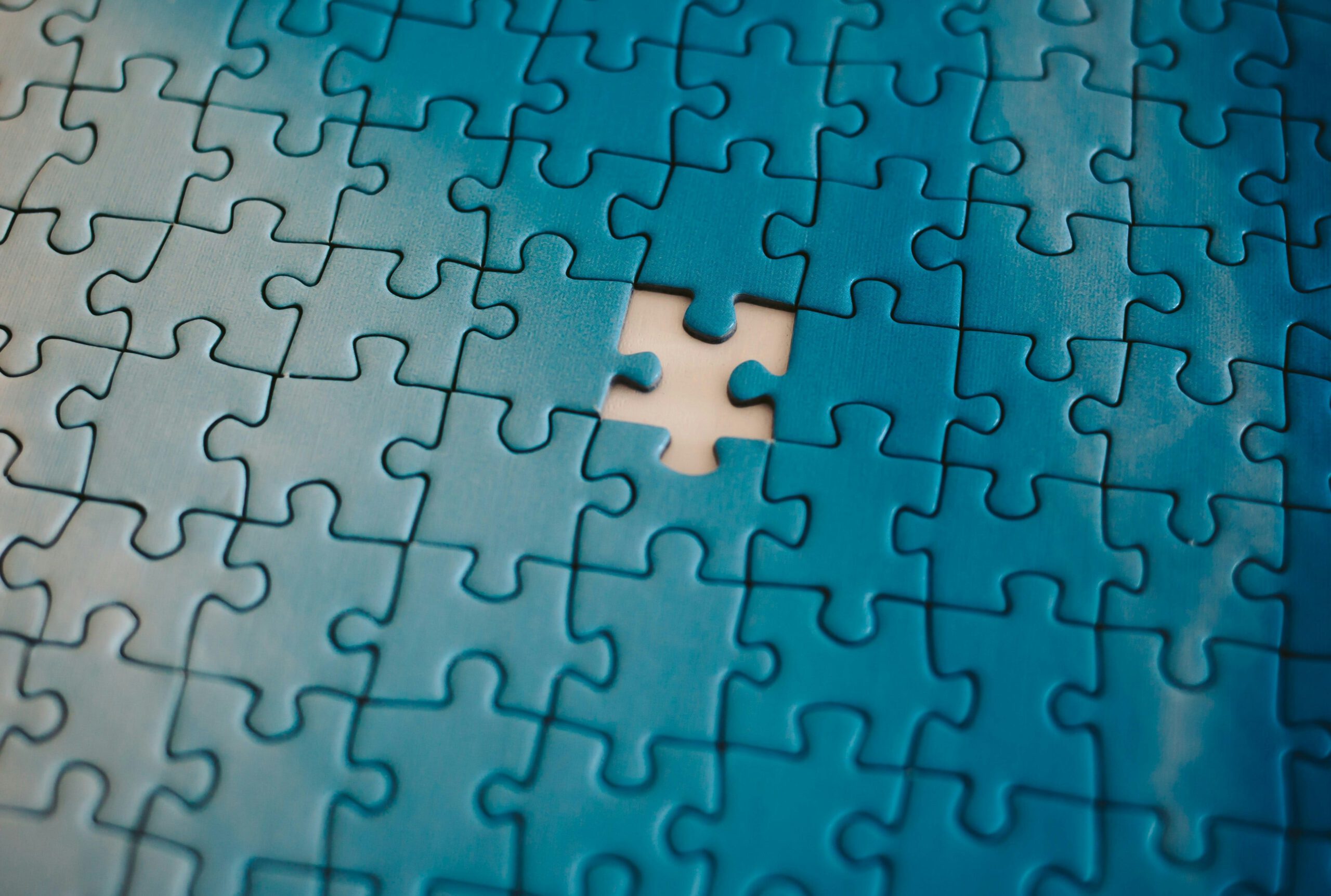
In the literature, we can find several methods to address missing data, but according to the nature of the missingness, choosing the right technique is highly critical. Simple methods such as dropping rows with missing values can cause biases or the loss of important insights. Imputing wrong values can also result in distortions that influence the final results. Thus, it is essential to understand the nature of missingness in the data before deciding on the correction action.
The nature of missingness can simply be classified into three:
- Missing Completely at Random (MCAR) where the missingness do not has a relationship to the missing data itself.
- Missing at Random (MAR) where the missingness is related to observed data but not to the missing data itself.
- Missing Not at Random (MNAR) where the missingness is related to the unobserved data. That is why it is more complex to address.
These terms and definitions initially seem confusing, but hopefully they will become clearer after reading this article. In the upcoming sections, there are explanations about different types of missingness with some examples, also analysis and visualization of the data using the missingno library.
To show the different missingness types, National Health and Nutrition Examination Survey (NHANES) data between August 2021 – August 2023 for Diabetes is used in this article [1]. It is an open source data which is available and can be downloaded through this link.
The survey data can be downloaded as .xpt file. We can convert it into a pandas DataFrame to work on:
import pandas as pd
# Path to the XPT file
file_path = 'DIQ_L.xpt'
# Read the XPT file into a DataFrame
df = pd.read_sas(file_path, format='xport', encoding='utf-8')
In this dataset, SEQN shows the respondents' sequence number. All the other columns corresponds to a question in the survey. Short descriptions of each question are as follows:
- DIQ010: Doctor told you have diabetes?
- DID040: Age when first told you had diabetes?
- DIQ160: Ever told you have prediabetes?
- DIQ180: Had blood tested past three years?
- DIQ050: Are you now taking insulin?
- DID060: How long taking insulin?
- DIQ060U: Unit of measure
- DIQ070: Take diabetic pills to lower blood sugar?
If you would like to read more about the questions and answer options in the survey, you can read from this link.
Exploring Missing Values
In order to understand the nature of the missing values, we should understand the patterns and distribution of the missing data.
Let's firstly discuss how we can basically see whether our data has missing and/or null values.
# Display the first few rows of df
df.head()

For this case, we can see even from the first rows, there are many null values in the data. The code below could be used to see there are how many missing values in each variable.
#shows how many null values in each column
df.isna().sum()
# Missingno bar chart
msno.bar(df, figsize=(4,4))

Now, more significant question is:
_