Advanced Prompt Engineering: Chain of Thought (CoT)
Working with Large Language Models

_If you're not a member but want to read this article, see this friend link here._
Chain Of Thought (CoT) has been around for quite some time and is technically a type of advanced prompt engineering, but it remains relevant even now, a few years after it was first introduced. CoT, in its various forms, is typically an effort to force large language models to reason.
After OpenAI's preview of their model o1 was released this September, we saw the hype around CoT increase.
No one completely knows how o1 works (except for OpenAI, that is), whether it's a combination system, what kind of data it has been fine-tuned with, if they are using reinforcement learning, or if there are several models working together.
Maybe one model does the planning, another the thinking, and a third rates. But we do know they are employing some type of step-by-step reasoning.
There has been quite a lot of open research around this that you might want to dig into. So for this piece, I will go through what's out there so you know what you can use. Naturally I will test the different techniques to see how and if we can achieve any real improvements.
Then, if you're keen to do something technical, I'll help you build a system that looks at a model's internal confidence levels to produce an answer.
The Research
There have been many papers released in the last two years and I've gathered quite a lot of the ones I have found here.
The reasoning techniques they talk about you'll see in the image below.
Most of the work comes directly from DeepMind or Princeton. Kudos to them for open sourcing so much work.

The term CoT came from DeepMind in 2022, in terms of only using it in prompting, and the latest papers have explored Three of Thoughts with Monte Carlo Search and CoT without prompting.
For this piece we'll go through simple Chain of Thought (CoT), CoT chains, Greedy Decoding, CoT-SC, Decoding CoT, and Three of Thoughts (ToT) with Monte Carlo Tree Search.
We will also use our own set of data to get an understanding of the improvements we can achieve as we employ some of these reasoning techniques.
Baseline Scores of LLMs
To understand how we can improve the results of LLMs, we first need to establish some kind of baseline score.
When a model is introduced, it usually comes with evaluation metrics. There are several popular ones, such as MMLU (language understanding), BigBench (reasoning), HellaSwag (commonsense reasoning) and so on.

However, you should be aware that some of these datasets are rather outdated and may be a bit contaminated.
Hugging Face introduced a new LLM Leaderboard now in December that evaluates based on newer datasets, and you can clearly see that most models have much lower scores than they did for the original datasets.
It's worth doing some research here to understand how you should think in terms of model evaluation and on what grounds you and your organization should evaluate. Having an internal private dataset to test may not be the worst idea.
But in any case, I dragged out about 350 questions from various datasets alongside a few popular questions I found online to evaluate up to 11 different models.
I needed to know what these datasets looked like as well as the answers that were generated from the LLMs.
So, I built my own scripts to loop through the questions and then evaluate the LLMs with a 0 or a 1 for each question.
Call me a perfectionist. You can see the results I found below.

What does this tell us? Well, not much.
I used questions from Big Bench, MMLU, Putnam, alongside popular questions such as ‘How many r's are in Strawberry,' but we have no way of knowing if they have been contaminated by these questions. Also, it's a rather small dataset.
However, we can clearly see that the larger models perform better.
What will be interesting to see is if we can improve these scores by applying methods that make the model reason and ‘think' before answering.
Chain of Thought (CoT)
Chain-of-Thought (CoT) prompting was introduced by the paper ‘Chain-of-Thought Prompting Elicits Reasoning in Large Language Models' in 2022 by the Brain Team at DeepMind.
So, the idea of CoT has been with us for quite some time.
This first paper, however, was research on how to force models to reason on a problem by activating the model's inherent reasoning capabilities using prompting strategies.
At this time, people were simply prompting in the correct way by asking the model to ‘think step by step,' either through zero-shot (providing no examples) or few-shot (providing a few examples) approaches.

You can do this for various models today such as Claude, ChatGPT, or others by simply adding ‘Let's think step by step' at the end of a prompt. If you want to try few-shot learning, you give it a few examples in the prompt.
DeepMind reported that they could verifiably see that there was a significant improvement using CoT techniques by prompting correctly.
Since then, many papers have built on these techniques, branching out into paths that are becoming more and more advanced.
Building Reasoning Chains
There are many people within the Prompt Engineering community that experiment with CoT-style techniques. I have gathered most of the repositories I've found here so it's easy to find.
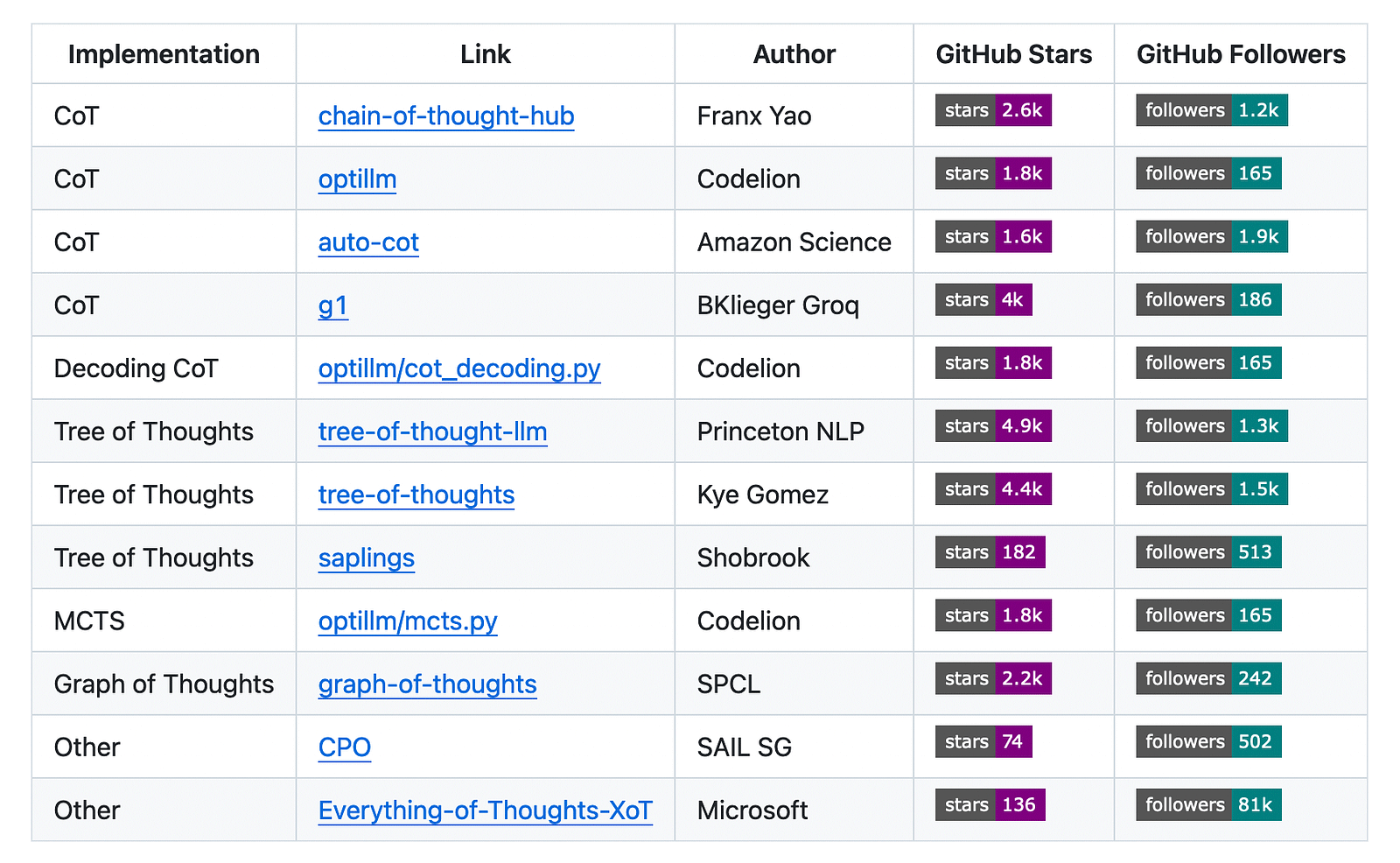
One that stood out not too long ago was Benjamin Klieger, who built a prompt-style application eliciting chain of thought thinking with the use of Groq and Llama 3.1 70b by breaking down the thinking process further.
You'll find his application here.
The idea is to ask the LLM to break down its thinking into chains where it continues to think until it feels confident about the answer.
The system would then continue to generate LLM calls for each part of the chain, rather than have the entire thinking process in one response.
See an example of applying this to Grok-Beta with the question ‘How many R's are in Strawberry?'

The model itself is setting up each part, giving it a title and decides whether it needs another ‘thought' and should continue or if it has reached the final answer.
This is still a form of CoT-style technique as it is linear, but it is slightly more advanced than simply asking a model to ‘think step by step.'
I used some of his code to build a script to loop through the base questions for some of the LLMs I tested to see how much improvement it would actually elicit with such a system. I adapted the script for Claude and Grok to evaluate this strategy on them too.
You'll see the percentage improvements below.

Llama 3.1 70B saw the best improvement in the first three categories. Grok did worse on popular questions (and so did Haiku).
The Putnam dataset is advanced mathematics, and very few LLMs can do well here, so imagine my surprise when Claude Sonnet 3.5 was able to do better than o1-preview at 68.75% with these CoT chains compared with o1-preview at 63%.
In total Sonnet had an 81% improvement for advanced maths with the use of CoT.
Remember, I used a very small dataset here, and it was only to get an idea of what they did well in and whether we could improve the scores. It tells us nothing concrete without testing it on a much larger dataset.
Nevertheless, I also observed that smaller models can produce worse results if they start to overanalyze on an easy problem. This was evident with Grok-Beta and Haiku on the popular ‘easier' questions.
Easier, non-mathematical problems may not reap the same benefits of CoT.
We also have to remember that we can push a model to perform within its abilities, but rarely beyond it. If it doesn't know the answer, it doesn't know.
Fine-Tuning for Reasoning
I want to mention fine-tuning before moving on.
One of the very interesting areas has been the work into trying to fine-tune smaller models on CoT datasets to increase their accuracy to that of models 1–2x larger.
I have found multiple resources for this, but unfortunately, I haven't found a significant improvement from the base model that I felt warranted a proper analysis.
You'll see the open source models I found below.

You'll see the CoT datasets I found that have also been open sourced below.

That is not to say that fine-tuning for CoT won't work, there just needs to be better models built that are well documented.
If you are keen to try fine-tuning on your own, go check out those resources. I'm sure there is more out there as well.
Alternative Generation Techniques
What we've talked about are Chain of Thought techniques, but there are other ways to optimize a language model's output accuracy without prompting.
This involves those sampler settings that we mostly ignore when making a call to an LLM – parameters like temperature, top_p, and do_sample – which can play a role in controlling the behavior of the outputs.
Now, we don't always have access to all these settings for a commercial API, but we do have access to temperature. In technical terms temperature means we can scale the logits when we set it as high and thus increase the chance that a low probability token will get picked.
This may seem confusing if you are new to LLMs but it's not as complicated as it sounds.
You can see my scribbles below on how the probability increases for tokens as we scale up temperature.

Let's say the token "mat" has the highest initial logit at the start, but as we increase the temperature we see that it starts to scale down decreasing the probability. The opposite happens for an inital logit that has a lower number.
What does this mean? It means that a model will more likely chose a word that feel less "safe" if the temperature is high.
Most call it randomness or creativity.
For top_p, which not all commercial APIs may have access to, you can restrict or expand the token pool depending on the number you set.
A low score will restrict the pool to tokens with a high probability score and vice versa – a low score means just the high probability tokens will be in the pool of candidates.
A high top_p combined with a high temperature would then create more innovative and creative outputs, as many more tokens will be candidates.
The do_sample parameter decides whether the model uses sampling at all to generate the next token. This setting you rarely have the ability to set via a commercial model through an API.
When the do_sample is set to True though, the model samples from the pool of candidates and has more freedom (this is the default behavior in all APIs). When set to False, it selects the highest probability token only (and completely ignores temperature or top_p).
We can use the do_sample setting to force the model to produce more deterministic outputs, i.e., the highest probability token at every stage.
This is called Greedy Decoding.
It's a strategy where the model selects the highest probability token at each step, which may result in more accurate answers (if it has the inherent knowledge needed).
I applied Greedy Decoding using do_sample to the model Llama 3 8b (instruct) to see if we could elicit an improvement in the base questions.
You'll see the results below.

I did see some improvements in MMLU and Big-Bench but very little for advanced maths.
Now, as commercial APIs won't have access to do_sample, to apply something similar without access to the model, you could possibly set the temperature=0 to try to mimic this behavior, but it's not a guarantee.
So, a question you may have by now, why not always use greedy decoding if we do see small improvements?
If we disregard the need for some creativity in outputs, you'll also find that less capable LLMs can go into a loop of repetition for difficult problems, such as saying ‘The color is blue blue blue blue,' where ‘blue' seems to be the highest probable token, so it gets repeated.
Advanced CoT
Up until this point, we've been looking at linear techniques where the model is producing outputs in one thread – or chain.
But it wasn't long after the first CoT paper was introduced that another more advanced technique was introduced called Chain of Thought with Self-Consistency (CoT-SC) by DeepMind.
This technique creates several reasoning paths and uses some method to select the most consistent answer (or path) at the end.
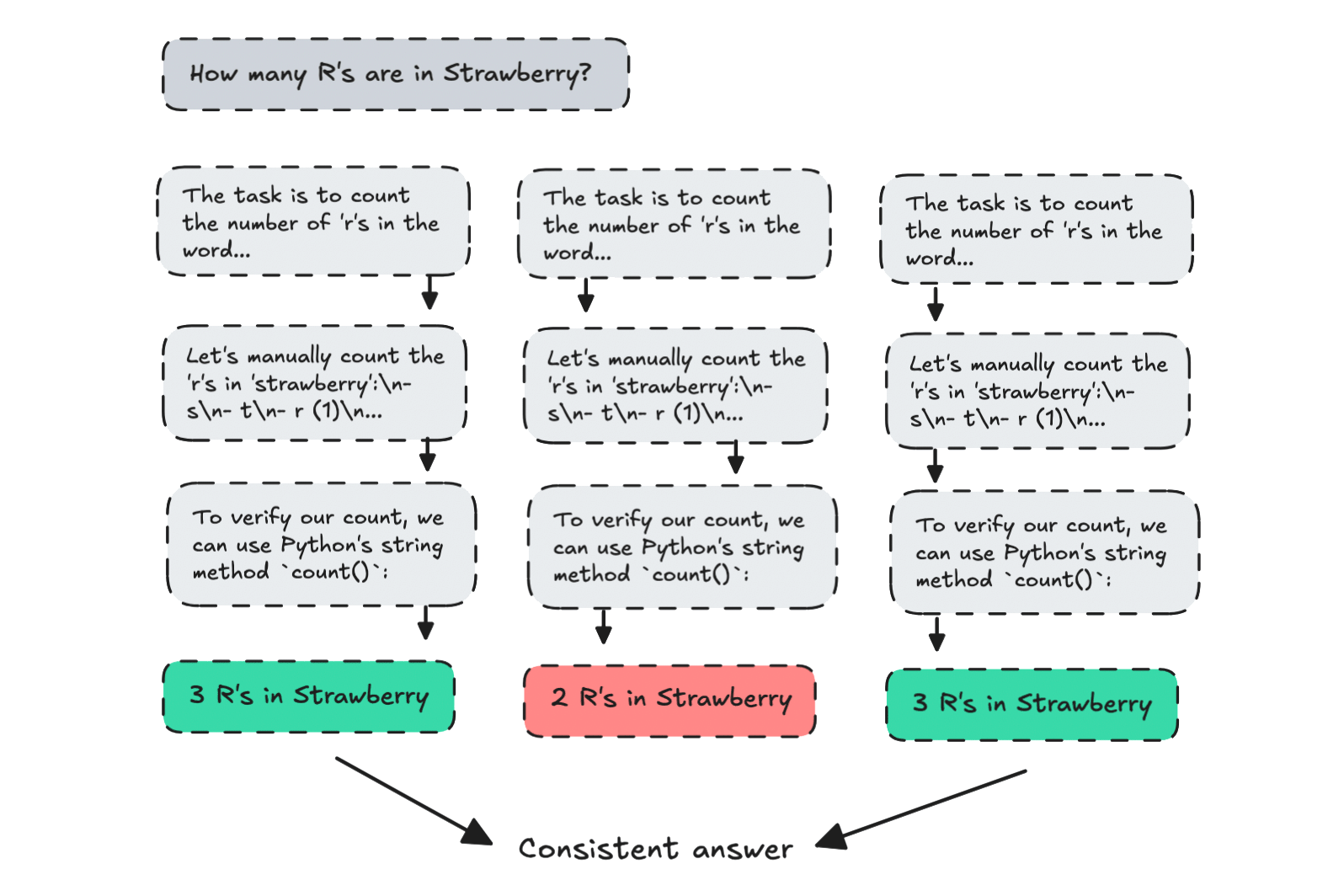
They reported finding around a 1–8% improvement in arithmetic reasoning using this method.
Another method introduced just this year follows a bit of the same idea of using multiple paths but without using any prompting.
Remember the idea of Greedy Decoding that I talked about in the previous section?
This method is similar, except it's not just about forcing the most probable token but also looking at the confidence scores of the entire responses.

To do this, the system first initiates a certain number k of initial top tokens and then generates paths from each of them. Once the answers are generated, it calculates the confidence scores by analyzing the probabilities (logits) of each token in the different paths.
The answer – or path – with the highest probability is returned.
This method is called Decoding CoT and was introduced by DeepMind. The idea of this method is to look at the internal confidence of the model in the answers returned.
But what happens if it doesn't have the inherent knowledge to answer the question? As with CoT-SC, this method would heavily depend on the model having the correct answer in the first place.
Nevertheless, that doesn't mean we shouldn't test it.
For all these techniques, there are people out there open sourcing different practical implementations, and this one is no different.
Therefore, it was easy for me to set up a system to test these methods and compare which did better with a smaller open source model, Llama 3 8b.

Kudos to Codelion for open sourcing his implementation making it easy for me to replicate.
Looking at the results above, you can see we are clearly producing the best results with Decoding CoT compared to other methods such as Entropy or simply using greedy decoding for this specific model.
We'll create an API that will use this Decoding CoT system in the technical section so you can see how it works.
Newer Techniques
It's hard to keep up, but the research has advanced much further than using simple CoT for reasoning within more high-stakes domains.
I won't go into all these strategies now, as that's a topic for another time, but I do want to mention Three of Thoughts (ToT) especially in combination with Monte Carlo search.
ToT was introduced at the end of 2023 by Princeton University and DeepMind but generally builds on the previous method of tree-based reasoning.
Three of Thoughts (ToT) is a bit different than Chain of Thought with Self-Consistency (CoT-SC). Where instead of generating multiple paths and evaluating them only after they have been generated, ToT evaluates thoughts dynamically as they progress.

Think of this as 4 different people coming together to solve a problem. At each step, they propose their ideas and collectively evaluate which ones seem most promising. If one person's reasoning appears flawed, they leave, so the others continue working through their solutions.
In the end, the person who has been reasoning correctly will be able to offer you their answer.
This allows the model to dynamically prune paths that seem lackluster, focusing on more promising threads, thus possibly saving resources.
However, one might question, how does the system decide which thread is right and wrong? This is decided by the model itself.
This is also why extensions like Monte Carlo Tree Search (MCTS) come in to provide more unbiased evaluation mechanisms. MCTS allows backpropagation which means it can revisit and refine earlier steps based on new information, whereas simple ToT only moves forward.
In the case of the 4 people solving a problem, MCTS would allow for people to have less than ideal thoughts and still stay in the game for longer. The evaluation method would be different.
MCTS can simulate multiple future paths, evaluate their potential, and backtrack to improve earlier decisions. It introduces external metrics (rewards) instead of completely relying on the model.
Statistics like UCB (Upper Confidence Bound) uses those rewards to decide which ideas to explore further or revisit.
MCTS is a bit more complicated than simple ToT and should possibly be an article by itself.
Economics of CoT
So, up until now, you might think, well, we have some improvements, why not always work with more advanced forms of Chain of Thought?
Well, first of all, cost (and also the amount of thinking time).
For the chains I applied to the different models, I calculated the average amount of reasoning steps.

Looking at this, you'd be paying up to 8 times more on average for each question. For Sonnet, which did best on advanced mathematical questions, you would be paying up to $15 per 500 questions.
This may not seem like much, but once you are using this system every day to generate answers for customer service or your team, you would be looking at hundreds if not thousands per month.
In some cases, it makes sense to use advanced reasoning methods, but not always.
Now there might be a case for fine-tuning for CoT, essentially eradicating the need to produce multiple calls, but I haven't as of yet seen any open-source model that has done this well.
There's a bit of a trade-off here. We want to increase the thinking time to allow the model enough time to reason effectively, but by doing so, we also increase user frustration and costs.
Building Intelligent Systems
In September of this year, a paper was released titled "To CoT or not to CoT?" that argued most improvements from applying CoT were mainly in mathematical and complex reasoning.
We saw this too here, where simple questions give us limited improvements.
When we apply these chains, we have to wait longer for a response. Is it worth it? It should be noted though that all these strategies can be overkill for simple tasks.
This is why you may feel frustrated using OpenAI's o1 for most questions, where a simple answer usually does well enough.
But if you are building a system where you need to ensure the answer is correct, then employing some form of CoT or decoding could be good.
It might be worth using one model to set up the first steps based on the question's difficulty, and then to analyze if it's confident it can answer it in the first place. Then have the model reason (via chains) and have another model at the end to rate the response.
Notes
Are there more frameworks than what I have introduced here? Absolutely, but I've presented the ones I felt were interesting to understand. This gives you an idea of how far we have come without the information being overwhelming.
Most AI engineers – are well versed in these frameworks, but it's a pity that this research isn't reaching the general public as quickly.
Understanding how to implement CoT should be part of the basics when building LLM applications, even if you decide against using them.
Technical Work
Let's put this into practice.
We'll implement a Decoding CoT system using an open-source model, Llama 3.1 8b.
The method of decoding CoT comes from the paper, "Chain-of-Thought Reasoning Without Prompting," released this year, and the implementation is grabbed from Codelion, found here. I've added some functionality so the system checks for the level of difficulty to decide on the amount of paths (k).
Since I went with Modal last time, this time we can use Beam, also a Serverless LLM serving platform. They offer 15 hours of free tier so this will be free. The script we'll use for this you'll find here.
If you'd rather use Colab to test, you can run this script here.
The result should be an API endpoint that lets us ask a question, and it will evaluate the difficulty and then perform Decoding CoT on the problem and return a response like below.

You'll see the amount of requests to the LLM and how the question was classified by the system. You'll also notice that the system is quite slow as it is generating multiple answers to evaluate.
However, if we try Groq with the same 8b model, we see that it can't quite answer the question correctly.

The correct answer is 27.3, with bonus points for additional fuel.
In terms of the final answer, I will note, though, that a smaller model like this will only get us so far. Unfortunately, using a larger model is a bit more work as we need to store it somewhere, which can be expensive.
To set up this system, I will grab 5 minutes of your time. You can follow the directions below.
Hugging Face
We'll start by gaining access to the model we'll be using. To use the Llama 3 8b model, you'll need to be granted access to it via Hugging Face.
This process is usually quite quick if you already have a Hugging Face account. If you don't have one, you can create one for free and the navigate to the model card.

Once we are in the model card we might as well test the model for a question that we can use to test this new system as well.

This is a rather standard question to ask and I have used it in the evaluation earlier but the standard Llama 3 8b model has a hard time with this one.
After you've been granted access, navigate to ‘Settings‘ to get an access token.

Save this token somewhere as we will need to set it in Beam.
Beam.Cloud
If you don't have a Beam account, you will need to create one (unless you chose to use Colab directly). You can, of course, build your own system on a different platform.
If you decide to go with Beam, grab an API key from their dashboard.

Set up the Environment
Now, we can get started. Open up a new terminal and create a new directory, and then cd into it.
mkdir my-testing-dir
cd my-testing-dir
Clone the repository I have set up.
git clone https://github.com/ilsilfverskiold/decoding-cot-beam.git
Create a virtual environment (you need to have python installed for this).
python3 -m venv .venv && source .venv/bin/activate
Install beam and authenticate.
pip install beam-client
beam configure default --token "your_token_here"
Make sure you set the HF_TOKEN we got earlier from Hugging Face.
beam secret create HF_TOKEN
You can serve it directly from here but let's walk through the code for a bit.
If you're uninterested you can skip this next part.
The Code
We have three python files in the root folder.
│
├── app.py
├── question_classifier.py
└── cot_decoder.py
In app.py
, we have code from Beam that lets us download the weights of the model from Hugging Face (on start) and cache it via Volumes. This means that the first time we run this, it may be clunky and slow.
Beam also lets us load the packages when the script is running remotely on Beam.
Here's the start of app.py
with my comments:
[...]
# This ensures that these packages are only loaded when the script is running remotely on Beam
if env.is_remote():
import torch
from transformers import AutoModelForCausalLM, AutoTokenizer
from cot_decoder import cot_decode
from question_classifier import get_k_value
# Model parameters & where to cache it in Volumes
MODEL_NAME = "meta-llama/Meta-Llama-3-8B-Instruct"
CACHE_PATH = "./cached_models2"
# Load the model and tokenizer
def load_models():
tokenizer = AutoTokenizer.from_pretrained(MODEL_NAME, cache_dir=CACHE_PATH)
tokenizer.pad_token = tokenizer.eos_token
model = AutoModelForCausalLM.from_pretrained(
MODEL_NAME, device_map="auto", torch_dtype=torch.float16, cache_dir=CACHE_PATH
)
return model, tokenizer
# Define the endpoint
# You can specify CPU/Memory/GPU + the image
@endpoint(
secrets=["HF_TOKEN"],
on_start=load_models, # load the model on start to be cached
name="meta-llama-3-8b-instruct",
cpu=2,
memory="32Gi",
gpu="A100-40",
image=Image(
python_version="python3.9",
python_packages=["torch", "transformers", "accelerate"],
),
volumes=[Volume(name="cached_models2", mount_path=CACHE_PATH)],
)
[...]
We have defined an @endpoint
with the resources we want for it (A100 GPU & 2 CPU cores). You'll also see that we are loading the model on start.
Once the API call comes in, we run the generate_text()
function.
[...]
def generate_text(context: Dict[str, Any], **inputs: Dict[str, Any]) -> Dict[str, Any]:
# Retrieve model and tokenizer from on_start
model, tokenizer = context.on_start_value
# Get adaptive k value based on question complexity
classification_type = None
if k is None:
k, classification_type = get_k_value(messages, context)
try:
output_text, confidence, llm_calls = cot_decode(
model=model,
tokenizer=tokenizer,
messages=messages,
k=k, # Use adaptive k value
**inputs # Pass any additional parameters directly to cot_decode
)
# Return the output
return {
"output": output_text,
"confidence": confidence,
"complexity_info": {
"k": k,
"total_calls": llm_calls + 1, # + classification call
"classification": classification_type
}
}
except Exception as e:
return {"error": f"Error during generation: {str(e)}"}
We have a function that first calculates k based on complexity using get_k_value()
. But the key function here is cot_decode()
, which will perform the decoding chain of thought on our question.
This function will take in the message, the model, and the tokenizer and make a first initial call to predict the k amount of next possible tokens with the highest logits.
The logits are the raw scores that the model assigns to each possible next token, letting us know the model's confidence score for each option.
These will serve as potential starting points for generating multiple answers. For each of these starting points, or starting tokens, we generate a full answer, which is then scored as a whole.
Remember we talked about greedy decoding, where we only generated the next token if it had a high probability? This will instead look at the sentences as a whole rather than just token by token by calculating a confidence score that reflects how certain the model is about the full answer.
After we have the path with the highest confidence score, it will be returned alongside the k value.
There are some additional options, such as adding in the aggregate_answers
bool when the model return several high confidence answers but we are not using that here.
Let's Run It
Now that I have explained the code briefly, we'll run it to see how it does.
You should be able to simply call serve.
beam serve app.py:generate_text
This is if everything is set up correctly.
Your first call will take quite a bit as it will be caching the model. Run serve again if it times out, it is caching the model for you.
To see where the model is stored, you can go to Volumes in the Beam.Cloud platform.
Once it is running you'll see something like below.

This means it is ready to be tested.
You can boot up Postman or use use cURL (which means you run the call to the endpoint in a terminal window)
curl -X POST 'https://app.beam.cloud/endpoint/id/[ENDPOINT-ID]'
-H 'Connection: keep-alive'
-H 'Content-Type: application/json'
-H 'Authorization: Bearer [AUTH-TOKEN]'
-d '{
"messages": [
{"role": "user", "content": "Give me three sentences that end in 'is'"}
]
}'
The response should look like something below.

As you see it can perform a bit better.
If you want to deploy the model you can simply run deploy.
beam deploy app.py:generate_text
I was just using this to test so I can close it down for now.
Hopefully this was educational and fun and you learned something.
If you want to look the results from the LLMs and the CoT techniques, you can look into this sheet and all other resources you can find in this repository.
Leave a comment and give me a few claps if it was helpful.
❤