AI Mapping: Using Neural Networks to Identify House Numbers

Introduction
One of the most interesting tasks in Deep Learning is to recognise objects in natural scenes. The ability to interpret visual data through machine learning algorithms holds significant practical value, as can be seen across a large range of applications (from autonomous vehicles to facial recognition). One such example of this is in locating houses on a map based on their house numbers.
The Google Street View House Numbers dataset contains over 600,000 labeled digits extracted from street-level photographs – it's one of the most popular image recognition datasets. Google has applied the images in this dataset to enhance map accuracy, by employing neural networks to automatically extract address numbers from these images. The output of these models, coupled with known street addresses, helps to precisely locate addresses in Google Maps.

The aim of this article is to illustrate how Artificial Neural Networks (also known as Fully Connected Feed-Forward) and Convolutional Neural Networks are used in predicting digits in these datasets. I show examples below of two models, one using ANN and the other using CNN, before fitting them to the SVHN dataset and comparing the results.
Data Preparation
Before jumping in, it's best practice to take a look at the structure of the unprocessed data. I visualised the first 10 images in the dataset below.

The output shows snippets of house number images taken from Google Street View, with one of the figures identified and labeled. The images sometimes contain partials of digits to either side of the identified digit, which may be a challenge for the neural network models.
Some necessary preparation steps are taken – the images are flattened from a 2D array into a 1D array, then divided by 255 to normalise (as these are greyscale images). The target variable is also converted to categorical, to ensure the deep learning algorithms can understand the given values.
#flattening dataset, dividing by 255 to normalise
X_train = X_train.reshape(X_train.shape[0], 1024)/255
X_test = X_test.reshape(X_test.shape[0], 1024)/255
#encoding target variable
y_train = to_categorical(y_train)
y_test = to_categorical(y_test)
With these steps complete, it's now time to define the deep learning models, beginning with an artificial neural network.
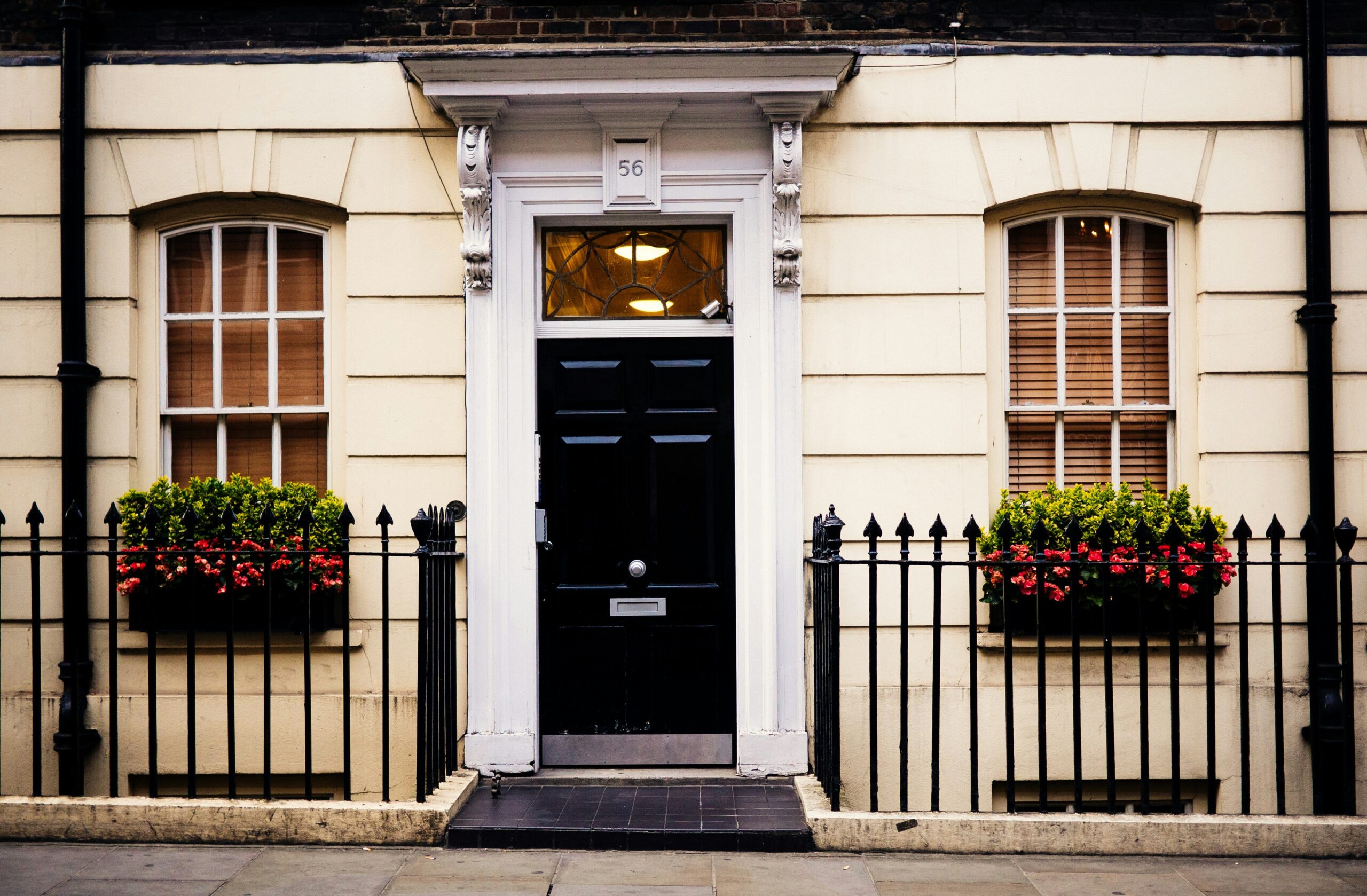
First Approach: Artificial Neural Networks
The first algorithm I applied to this problem is an artificial neural network (or ANN). ANNs are made up of interconnected neurons organised into layers: input, hidden, and output. Neurons in the hidden layers consist of weights and biases, with activation functions applied to introduce non-linear characteristics into the classification process. During training, the network adjusts its weights in order to minimise differences between predicted and true outputs. This algorithm is commonly used in the field of image recognition, making it a reasonable algorithm to apply to this problem.
Beginning with model building, I defined a function for an ANN model below – the model consists of five dense layers with ReLU activation functions, including dropout regularisation and batch normalisation (to reduce the risk of overfitting). I applied the Adam optimiser which is commonly used for its adaptive learning rate properties, while categorical crossentropy (common for multi-class classification tasks) is applied as the loss function. The model summary is then printed, which can be seen below.
#defining model function
def ann_model():
model = Sequential([
Dense(256, activation='relu', input_shape=(1024, )),
Dense(128, activation='relu'),
Dropout(rate = 0.2),
Dense(64, activation='relu'),
Dense(64, activation='relu'),
Dense(32, activation='relu'),
BatchNormalization(),
Dense(10, activation = 'softmax')
])
#instantiating adam optimiser
adam = Adam(learning_rate=0.0005)
#compiling model
model.compile(optimizer=adam,
loss='categorical_crossentropy', metrics='accuracy')
return model
#setting model function as variable
ann_model = nn_model()
#printing model summary
print(ann_model.summary())
#fitting model
hist_ann_model = ann_model.fit(X_train,y_train,
epochs=30, validation_split=0.2,
batch_size=128, verbose = 1)

Following this, I fit the model to the training data and set it to run 30 epochs. I've plotted the training and validation accuracies based on the model's history, which can be seen below.

Some points to note from the training-validation curves above:
- The training accuracy and validation accuracy are closely matched throughout the model training process – there does appear to be dips and spikes in the validation accuracy from epoch 6 onwards, however the overall trend is positive. Therefore the model is well fitted to the data, and can be expected to generalise well to unseen data.
- The training accuracy rapidly improves through the initial epochs, before decreasing in gradient and improving more gradually from epoch 7 onwards. The model achieves a final accuracy of 77.7% by epoch 30 – this is promising but still leaves a sizable number of values which were misclassified.
Additionally the model's confusion matrix below shows that the model often confuses similar numbers, for example 0 with 9, 2 with 7, and 3 with 5. This indicates some drawbacks to using this model in predicting numerical values.

While this is promising, 77.7% accuracy is too low to reasonably use this at scale – a more accurate solution will need to be built for this purpose. Now to compare these results against the performance of a convolutional neural network model.
