Asking for Feedback as a Data Scientist Individual Contributor
Asking for feedback can be difficult, or even intimidating. There are so many factors that could influence how you ask for feedback: company culture, personal fears, an uninvolved manager, past feedback being useless, etc…
While these might be true, they shouldn't deter you from asking feedback. As a person who leads teams, I have to provide tons of feedback, both on a regular basis but also in every performance review. In a previous post, I describe how I provide feedback using the SBIN framework. I want to lean on this framework to also provide you with a set of template/guidance questions which will help you target your feedback, and in return, get a higher quality response.
PS 1: Whilst the title of the blog post is targeting individual contributors, Data Science managers may find the content valuable to pass on to their teams.
PS 2: The inspiration for this post came from working together with Stefano Franco. Very thoughtful team lead in helping others through developing standard team tooling.
PS 3: All images are authored by me, unless otherwise specified.
The 4 old school questions for annual performance reviews
Imagine you are in the usual cycle of a performance review. This could be every 6 months or every year. You want to understand where your performance stands for both future opportunities, but also – and don't tell me this is not true – to see what your bonus and salary review will be. Your manager has asked you to get some Feedback from peers and stakeholders. This is to ensure she has a view of how have you performed and behaved outside of what she sees.
With the ‘asking for feedback' task in hand, you prepare the 4 questions everyone has used in the past (in fact, it's in the ‘asking for feedback' template your company provides). Here are the 4 old school questions:
- One thing you would like to see me continue trying is…
- One thing you would like to see me develop is…
- Something I have done really well recently is…
- It has had a positive impact because…
Honestly, when I receive this template, it makes me want to cry. It is sooooo generalist.

The person who has to provide feedback has to scratch their brains to figure out what happened over the past 6 months and tie it back to this feedback.
There is an added problem. As human beings, we want to be part of a tribe, so conflict and confrontation is something not desirable. Unless someone has a clear constructive feedback example in mind, the vast majority of the times, feedback comes back with lots of positive comments. "Keep doing what you are doing", "Great presentation", "Thanks for helping me on short notice"…
These are definitely nice to receive, but I personally want to grow through feedback. If you feel the same way, it's time to change what questions you ask and when you ask them.
Why do I provide feedback and how do I provide it?
There are 3 things I want to achieve when I am providing feedback to someone else:
- Recognize something that an individual is doing well.
- Develop an individual to perform more efficiently / effectively or learn something new for their growth.
- Improve or correct behaviours which negatively impact the individual's performance.
At work, to make sure that I am fair and consistent, I lean on the SBIN framework which stands for Situation, Behaviour, Impact and Next steps.
The key aspect of SBIN is about being specific. For example, instead of me telling my colleague "you did a great presentation", I would say: "Your presentation was great because you were constantly reminding the main takeaway in each slide and your charts had very little clutter. This made it very clear what should we would be doing next".
If the key of providing good feedback is to be specific, then the same applies to asking for feedback.
Breaking down feedback dimensions into categories
As I just mentioned; recognition, growth and corrections are my main dimensions of consideration when providing feedback. We can use the same categories but asking for feedback. I have also highlighted that specificity is important. Therefore, my suggestion for you is to think on sub-categories that would fit each of the core dimensions. In the screenshot below I have provided an example of how I break down each main category.
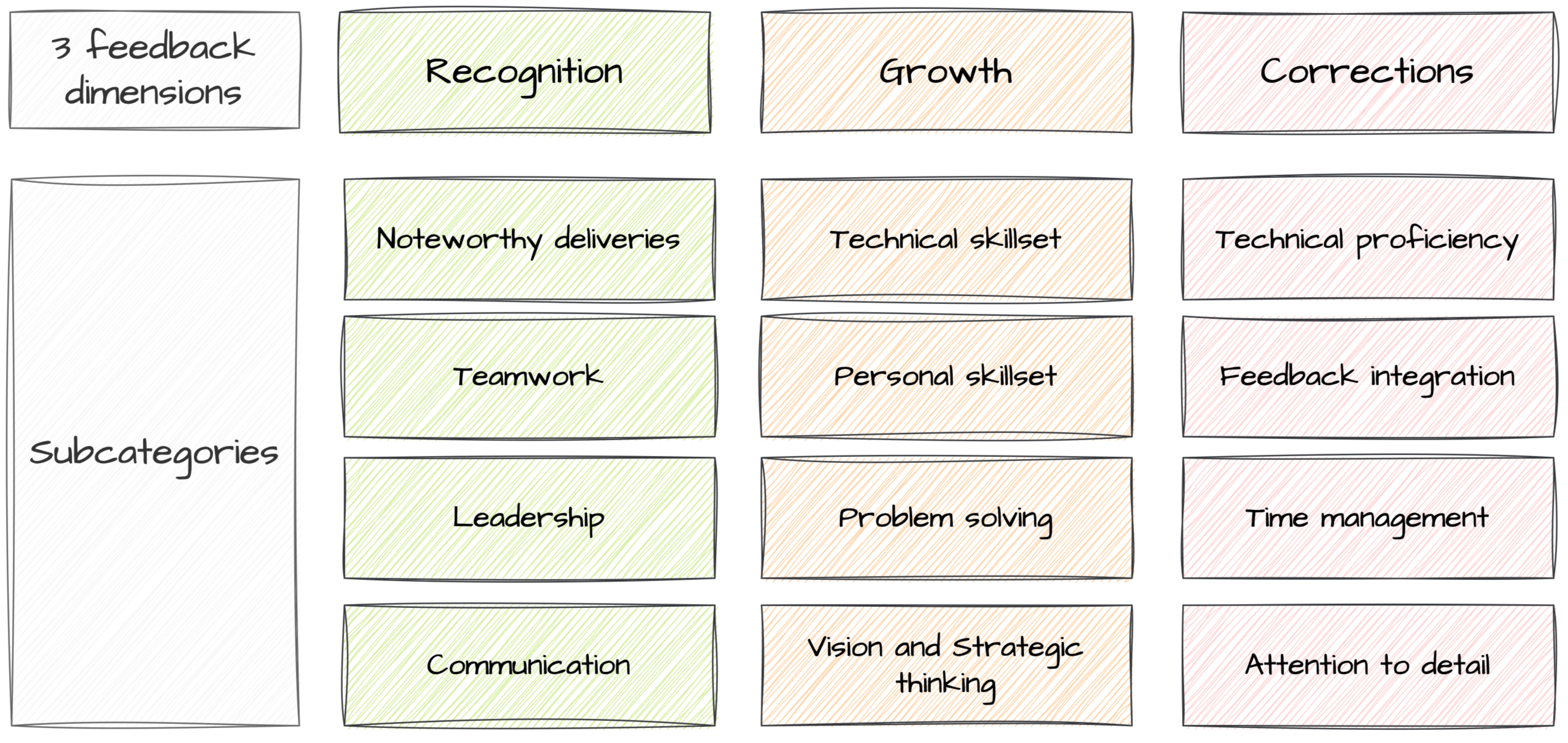
In the following sections, I will take each of these subcategories and provide you with a set of example questions you could use in your next feedback request.
Questions related to recognition
In all feedback requests, there is an element of recognition you want to capture. These pieces of feedback provide personal pride. But it is not only about pride; the reality is that this recognition is really needed for performance reviews. If you want to achieve a higher bonus or salary increase, you will have to demonstrate why. Adding an external view to the KPIs you have delivered is super important.
I propose 4 dimensions to describe recognition: specific noteworthy deliveries, teamwork, Leadership and communication.
Noteworthy deliveries:
This category acknowledges the specific contributions you've made to a project or task, highlighting where you have added value.
- Was there a specific solution that you think positively impacted the project?
- Have any of my technical solutions significantly enhanced performance, reliability, or scalability?
- Was there a specific work stream that achieved or surpassed expected performance?
- Was there a specific contribution(s) that had a positive impact on the team?
- Was there any innovative ideas or approaches I've brought forward that led to favourable outcomes.
- Were there any challenges I navigated particularly well that improved the project's outcome?
- Was there a situation where my data-driven insights and analysis contributed to a key decision?
- Did I effectively communicate complex data findings in a way that influenced stakeholder decisions?
Teamwork:
Teamwork captures how effectively you collaborate and contribute to the collective success of the team.
- How well do I contribute to our team collaboration sessions?
- Was there a specific situation were my support helped you?
- Was there a moment where I helped foster collaboration within the team to overcome a major challenge?
- How well do I facilitate communication between team members, particularly when dealing with cross-functional teams?
- Have I contributed to the team's morale or culture in a noticeable way?
- Have I shown adaptability when team dynamics shifted (e.g., new tools, processes, or members)?
Leadership qualities:
Leadership isn't only about formal roles but also about taking initiative, guiding the team, or leading discussions.
- Was there an occasion where I exhibited leadership qualities, even if not in a leadership role?
- Have I stepped up in a crisis or deadline situation to provide clear direction or reduce friction?
- Is there an instance where I helped coach or mentor a junior team member or peer?
- Is there a specific project where you believe I could/should be the person to move it forward?
- Have I demonstrated accountability by owning outcomes, even when facing difficult situations?
Communication:
Since data scientists often have to present complex findings to non-technical stakeholders, communication is a critical skill. If you get recognition on this area, you will stand out amongst your peers.
- How effectively do I communicate complex data insights to non-technical audiences?
- Was there a moment where my communication helped bridge a gap between technical and non-technical team members?
- Have I improved my storytelling abilities in presenting data to make the outcomes more compelling?
- Was my feedback during meetings clear and constructive? Did it guide discussions toward actionable steps?
Delivery feedback wrap-up
You can tailor the questions above by being even more specific. For example, ask about the presentation you gave, the experiment that was ran or when you lead the weekly performance meetings where 3 teams where involved.
Questions related to development
Recognition covered what you have done right. But what about things that could have been better? The best pieces of feedback are the ones that allow you to grow. In order to get the most out of someone's opinion to help in your development, I propose 4 dimensions to cover: technical skills, personal skills, problem solving and vision & strategic thinking.
Technical skillset improvement:
This category targets improvements in technical knowledge and practical skills like coding, algorithms, and handling data infrastructure.
- Are there any best practices when putting ML in production that I should be doing?
- Is there any algorithm framework that could help me with this type of problem?
- Is my code structure easy to maintain and understand for other team members?
- Are there specific data engineering practices I could implement to improve the efficiency of my pipelines?
- Have I demonstrated strong model evaluation techniques, or is there a better approach I could use (e.g., cross-validation, hyperparameter tuning)?
- Is there a specific technology (e.g., Docker, Kubernetes, CI/CD) I should learn to better integrate with production systems?
- Can you suggest ways I could improve the interpretability of my models?
Personal skillset developments:
Personal skills often involve leadership, communication, and relationship-building, which can greatly influence your career trajectory.
- Is there any feedback you have for how I handled a difficult conversation or situation?
- Are there any tips or examples where I could improve my leadership skills?
- What would you suggest for me to change in order to gain more influence with other teams?
- Was there a situation where I could have been more assertive in defending my technical choices? How can I improve?
- What could I do to improve my presentation skills when communicating insights to non-technical stakeholders?
- Are there any habits or practices I could adopt to improve my time Management or organisation skills?
Problem-solving
In data science, problem-solving and analytical skills are crucial. This category focuses on how you approach complex problems, evaluate data, and draw conclusions.
- Can you provide feedback on my approach to problem-solving, particularly how I frame problems and consider various solutions?
- Are there any alternative methods or models I should explore to improve the accuracy or efficiency of my solutions?
- How could I improve my ability to identify the root cause of a problem and develop a clear action plan?
- Do you have any suggestions for improving my hypothesis-driven approach when testing and iterating on models?
Vision and strategic thinking
Data scientists who can think beyond the technical aspects and consider broader business implications add immense value. This subdimension focuses on aligning data science with organisational goals.
- How can I better align my technical solutions with the broader strategic goals of the company?
- Are there any areas where I could improve my ability to think more long-term or strategically about the projects I'm involved in?
- Do you see opportunities for me to influence higher-level business decisions with my insights?
- What advice do you have for developing my ability to anticipate future challenges or opportunities in our industry?
Development feedback wrap-up
Of course, these questions should be bespoke to your situation. For example, if you are already working in MLOps, you might want to improve on something very specific, such as building efficient data unit tests or best practices for monitoring KPIs.
Questions related to corrections
Finally, we need to cover where we have got it wrong. It is important to acknowledge errors. The worst thing you can do is to hide them. Therefore, the best thing you can do is get feedback on them. Most errors come from technical problems, not great time management, feedback integration, and attention to detail.
Technical proficiency:
Mistakes in technical work are common, especially in the fast-paced, complex world of data science. This category focuses on uncovering and addressing technical shortcomings.
- Do you have any insights on the incident that happened and how I could have approached it better?
- Could you recommend me ways to try to improve the ways that I design and run A/B tests? I hadn't realised that my previous experiment was underpowered.
- I was told that I exploded the cluster costs the past month. Is there something you could help with to make sure this doesn't happen again?
- What could I have done differently to prevent the issue I faced with the data pipeline/ETL process?
- Are there specific debugging or testing practices I can adopt to avoid similar bugs or system failures in the future?
- Do you have suggestions for improving my documentation or version control processes to prevent future errors?
- I encountered difficulties tuning my model's hyperparameters. Could you suggest best practices or tools that could have made this process smoother?
Time management:
Time management issues often result in missed deadlines or rushed work, leading to poor-quality outcomes. Corrective feedback here helps you plan and execute your work more efficiently.
- I didn't meet the deadline last time. I could learn from someone who leads more projects than I do, and understand if I could do something differently in terms of planning and organising my workload.
- Was there something I could have prioritized differently to meet the deadline last time?
- Could you provide feedback on how I manage competing priorities across multiple projects?
- Are there tools or methodologies (e.g., agile frameworks, time-blocking) you would recommend for better time management?
- How can I improve my ability to estimate the time required for more complex data science tasks, like feature engineering or model training?
Feedback integration:
This subdimension focuses on how well you take previous feedback into account and whether there's room for improvement in how you implement suggestions.
- Please let me know how effectively I incorporate feedback into my work and my willingness to learn from past experiences.
- Have there been instances where I didn't fully incorporate previous feedback? How could I have done so more effectively?
- Are there any specific areas where I've struggled to adopt feedback, and how can I improve my approach?
- How can I show that I'm taking proactive steps to integrate feedback into my daily work?
- Do you feel that I've responded well to constructive criticism, or are there instances where I became defensive? How can I approach feedback more openly?
Attention to detail
Lack of attention to detail can result in small mistakes that have large impacts. This category addresses how carefully you approach your work to minimise errors.
- Can you identify areas where my attention to detail was lacking, and how I could avoid similar mistakes in the future?
- How could I improve the way I validate data or results to ensure they are error-free before delivering them to stakeholders?
- Were there moments where I should have performed more thorough checks (e.g., data validation, peer reviews) before finalizing my work?
- Are there habits or practices you could recommend to help me catch errors earlier in the workflow?
Correction feedback wrap-up
Effective correction feedback is about more than just identifying mistakes – it's about gaining actionable insights that lead to growth. This structured approach not only helps you understand what went wrong but also offers clear guidance on how to avoid similar issues in the future. Asking targeted questions in these areas enables your peers and stakeholders to provide more constructive, focused feedback, driving meaningful progress in your development as a data scientist.
Category interchange
The presented feedback questions are not set in stone. For example, if you read again the "communication" subcategory under "recognition", you can see questions such as "Have I improved my storytelling abilities in presenting data to make the outcomes more compelling?" or "Was my feedback during meetings clear and constructive? Did it guide discussions toward actionable steps?". You can take these questions and re-purpose them to fall under the "growth" or "corrections" category. For example:
- Repurposed for growth: How can I further improve my storytelling abilities when presenting data to ensure the outcomes are more compelling and easier to understand for non-technical audiences?
- Repurposed for corrections: There were instances where my interventions during meetings didn't seem to guide discussions effectively. How can I make my input clearer and more constructive to drive discussions toward actionable steps?
The bonus question
We have covered loads of specific example questions on 3 feedback areas: recognition, development and corrections. Do you know there is one magic question that you can always use to trigger great responses?

Here is the question:
In situation [X], what would you have done differently?
Whenever you ask this question, the following happens:
- The other person is putting themselves on your shoes
- They need to really think about what was done vs what they expected
- Solving a ‘challenge' is mentally attractive, and many people would love to provide solutions to the problem/situation.
The replies you get back tend to be detailed. You will probably learn solutions you hadn't thought about. You will understand how the other person is thinking about your work. Even more, if you do actually apply these suggestions and praise the other person for the ideas, your influence with them will improve (this will pay off in the future).
How can you increase the frequency of feedback?
Ideally feedback should be continuous, but nearly everyone falls in the trap of asking for it twice a year (i.e. when performance reviews happen). I have been rambling about being specific with your feedback.
One thing that really helps with specificity is frequency.
Try the following moving forward:
- Have you delivered a presentation to your squad, DS team or a wider group? Immediately after, ask a few individuals for how it went.
- Have you written a confluence page with detailed analysis? Ask those who read it if it was clear or if something could be improved.
- Did you lead a meeting with stakeholders where another DS colleague was also present? Ask them how did they think the meeting went and how to prepare better for the next.
- Did you run an interview and were shadowed by another interviewer? Ask what was their feeling on how you conducted the interview.
What I mean to highlight with these 4 examples is to try to integrate informal chats about specific pieces of work. The more of these you do, the more feedback you get. And the more regular feedback you get, the sooner and the more the elements you can improve on.

Summary
Hopefully, this post has made you think about how you currently ask for feedback. By shifting away from generic feedback questions and focusing on targeted areas such as recognition, development, and correction, you can foster more meaningful conversations that drive real improvements. Frequent, well-framed feedback helps you continuously refine your skills, gain valuable insights, and strengthen your relationships with peers and stakeholders. Ultimately, it's about creating a feedback loop that supports ongoing growth and success.
If there is a main takeaway it would be the following:
If the key of providing good feedback is to be specific, then the same applies to asking for feedback.
Further reading
Thanks for reading the article! If you are interested in more of my written content, here is an article capturing all of my other blogs posts organised by themes: Data Science team and project management, Data storytelling, Marketing & bidding science and Machine Learning & modelling.
Stay tuned!
If you want to get notified when I release new written content, feel free to follow me on Medium or subscribe to my Substack newsletter. In addition, I would be very happy to chat on Linkedin!
Get notified with my latest written content about Data Science!