Association Rule Mining in Unsupervised Learning
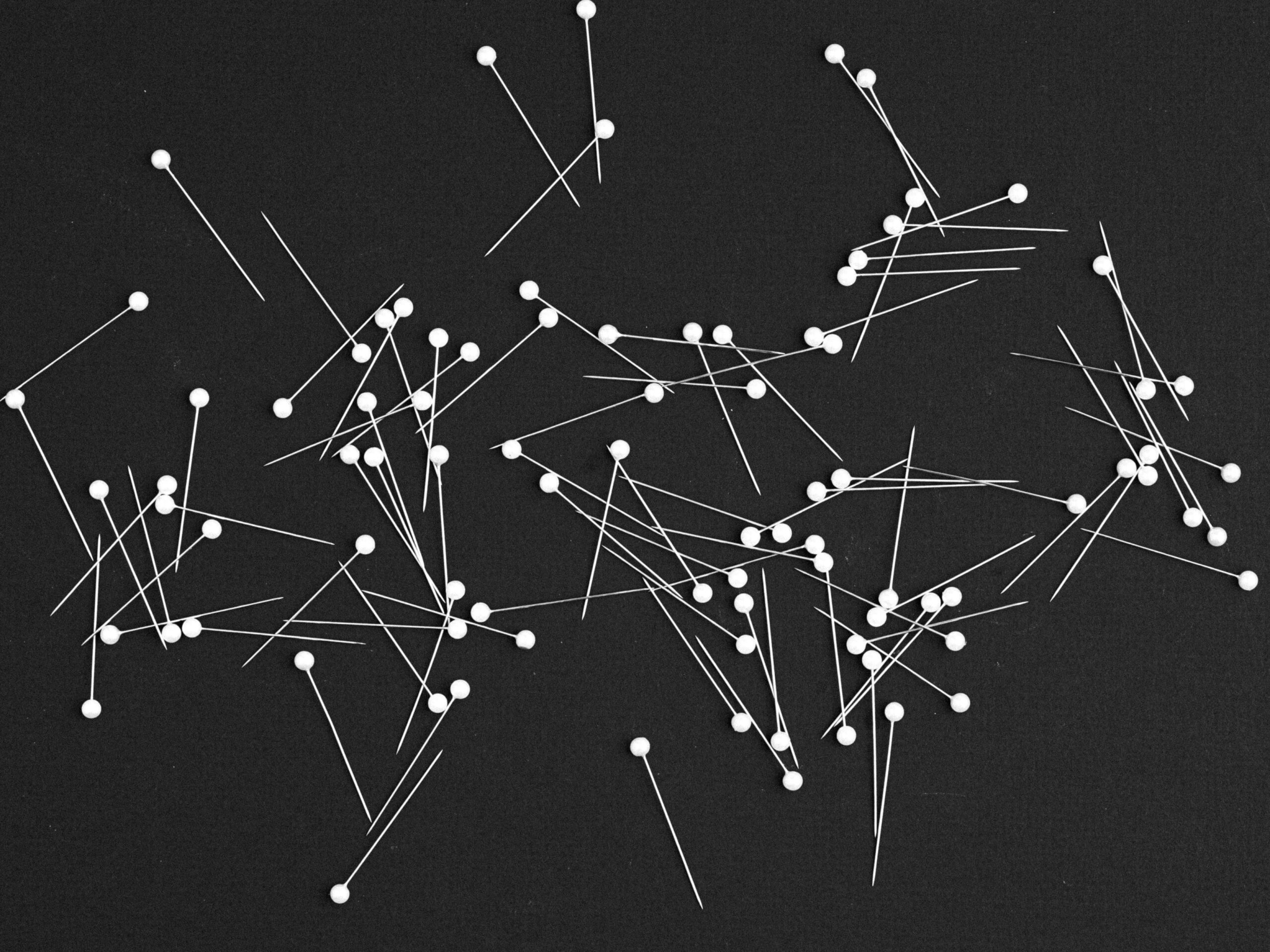
Pattern discovery tries to uncover patterns in a massive dataset, which forms the foundation for many data mining tasks such as Association, correlation, and causality analysis, cluster analysis, to name a few.
This article introduces common terminology in association rule mining, followed by association rule mining techniques for frequent patterns and sequential patterns.
Table of Contents
Frequent Patterns
Sequential Patterns
Terminologies
- Support: The probability of item
X
appearing, denotedP(X)
, measures the popularity of the item - Confidence: Conditional probability of getting item
X
after getting itemY
, denotedP(X|Y)
- Lift: Confidence divided by support, denoted
P(X|Y)/P(X)
, measures the independence of items and how much more likely itemX
is going to be bought given that itemY
is added to the cart - Leverage: Difference between support of both items and expected support if both items are independent, denoted
P(XUY)-P(X)P(Y)
, measures independence of items - Conviction: Support divided by confidence, denoted
(1-P(X))/(1-P(X|Y))
, measures independence of items and high conviction is a combination of strong confidence ofY->X
and a low support ofX
Apriori Algorithm
A horizontal breadth-first search algorithm
The Apriori algorithm identifies association by specifying a minimum confidence threshold. The intuition for the association is how confident one is that a consequent item will be selected after an antecedent item is selected, denoted P(consequent|antecedent)
.

For example in Fig 1, Confidence(A->C) = P(C|A) = 0.75
since item C
is bought following item A
3 out of 4 times. If this confidence is above the minimum confidence threshold (say 0.5), then an association of A->C
can be drawn.
Instead of computing confidence between every item set, a downward closure principle is applied to speed up the search for frequent item sets. The downward closure principle states that any subset of a frequent item set must be frequent, for example, if item set A, B, C
is frequent, it must follow that the subset item set A, B
is frequent as well.
The drawback of the Apriori algorithm is that the data has to be repeatedly scanned to compute the support and confidence of every item or item set.
Equivalence CLAss Transformation (ECLAT)
A vertical depth-first search algorithm using set intersections
In the Apriori algorithm, data is viewed as horizontal transaction-level data, where each transaction has one or more items. In ECLAT, data is transformed to vertical item-level data where each item has a set of transaction IDs where they appear (called the TIDset
).

Following the transaction data example in Fig 1, it can be rearranged to vertical item-level data as shown in Fig 2. For example, item B
appeared in transactions 1, 2, and 3, and this will result in the TIDset {1, 2, 3}
.
Support and confidence calculation for items can then be done with just set intersections between TIDset
. ECLAT algorithm is more memory efficient and computationally efficient than the Apriori algorithm as it uses depth-first search and does not scan the data multiple times to get support for every item.
Frequent Pattern Growth (FP-Growth)
A vertical depth-first search algorithm using the Trie data structure
The intuition behind FP-Growth is to find frequent single items and partition the database based on each such item and recursively grow frequent patterns for each partitioned database. These are efficiently done with a Trie data structure (FP-tree).
The data is scanned twice – once to find single item frequent patterns that are above the minimum support and a second time to construct the FP-tree.

Following the transaction data example in Fig 1, an FP-tree can be created as shown in Fig 3. Transactions 1 and 2 follow the path A-B-C-D
, transaction 3 follows path A-B-D
, and transaction 4 follows path A-C
.

From this FP-tree, we can easily compute the conditional pattern base (Fig 4), such as item C
appearing with {A, B}
twice and {A}
once – without scanning the data again.
Generalized Sequential Patterns (GSP)
Apriori-based sequential pattern mining, breadth-first search
GSP is similar to the Apriori algorithm, where data is first scanned for singleton sequences and filtered for frequent sequences. The data is then continuously scanned and filtered to retrieve longer sub-sequences. It is important to note that items do not have to be consecutive in sub-sequences, and a pattern can contain duplicated items.
Other Apriori-based sequential pattern mining includes Sequential Pattern Discovery using Equivalent Class (SPADE) algorithm which is similar to the ECLAT algorithm.
Prefix-Projected Sequential Pattern Mining (PrefixSpan)
Pattern-growth-based sequential pattern mining, depth-first search
PrefixSpan separates entries into prefixes and suffixes. Frequent prefixes are captured and the prefix's projection becomes a suffix.
PrefixSpan scans the data once to find length-1 sequential patterns and extends frequent length-1 sequential patterns recursively. Extending is done by setting length-1 as the prefix and the remaining items that start with length-1 as the suffix (referred to as the projected database), and recursively increase the length of the prefix. The projected database will shrink after every iteration as the suffix decreases in length.
This algorithm is slow but optimization can be done with pseudo-projection to use pointers instead of physically copying the suffix.
Other pattern-growth-based sequential pattern mining includes Frequent Pattern-Projected Sequential Pattern Mining (FreeSpan) which is not as efficient as PrefixSpan.
Compared to clustering, which is another topic under unsupervised learning, I feel that Association Rule Mining is more statistically grounded, making it more challenging to understand. Nevertheless, hope this article provided a general introduction to a few popular association rule mining techniques!
Related Links
- Terminology Definition: http://rasbt.github.io/mlxtend/user_guide/frequent_patterns/association_rules/
Papers
- Frequent Pattern: ECLAT, FP-Growth
- Sequential Pattern Apriori-based: SPADE
- Sequential Pattern pattern-growth-based: PrefixSpan, FreeSpan