Automated Prompt Engineering: The Definitive Hands-On Guide
What is this about?
Automated Prompt Engineering (APE) is a technique to automate the process of generating and refining prompts for a Large Language Model (LLM) to improve the model's performance on a particular task. It uses the idea of prompt engineering which involves manually crafting and testing various prompts and automates the entire process. As we will see it is very similar to automated hyperparameter optimisation in traditional supervised Machine Learning.
In this tutorial we will dive deep into APE: we will first look at how it works in principle, some of the strategies that can be used to generate prompts, and other related techniques such as exemplar selection. Then we will transition into the hands-on section and write an APE program from scratch, i.e. we won't use any libraries like DSPy that will do it for us. By doing that we will get a much better understanding of how the principles of APE work and are much better equipped to leverage the frameworks that will offer this functionality out of the box.
As always, the code for this tutorial is available on Github (under CC BY-NC-SA 4.0 license).
Why is it important?
When working with organisation I observe that they often struggle to find the optimal prompt for an LLM for a given task. They rely on manually crafting and refining prompts and evaluating them. This is extremely time consuming and is often a blocker for putting LLMs into actual use and production.
And it can sometimes feel like alchemy, as we are trying out different prompts, trying different elements of structure and instructions in hopes of discovering a prompt that finally achieves the desired performance. And in the meantime we actually don't even know what works and what doesn't.
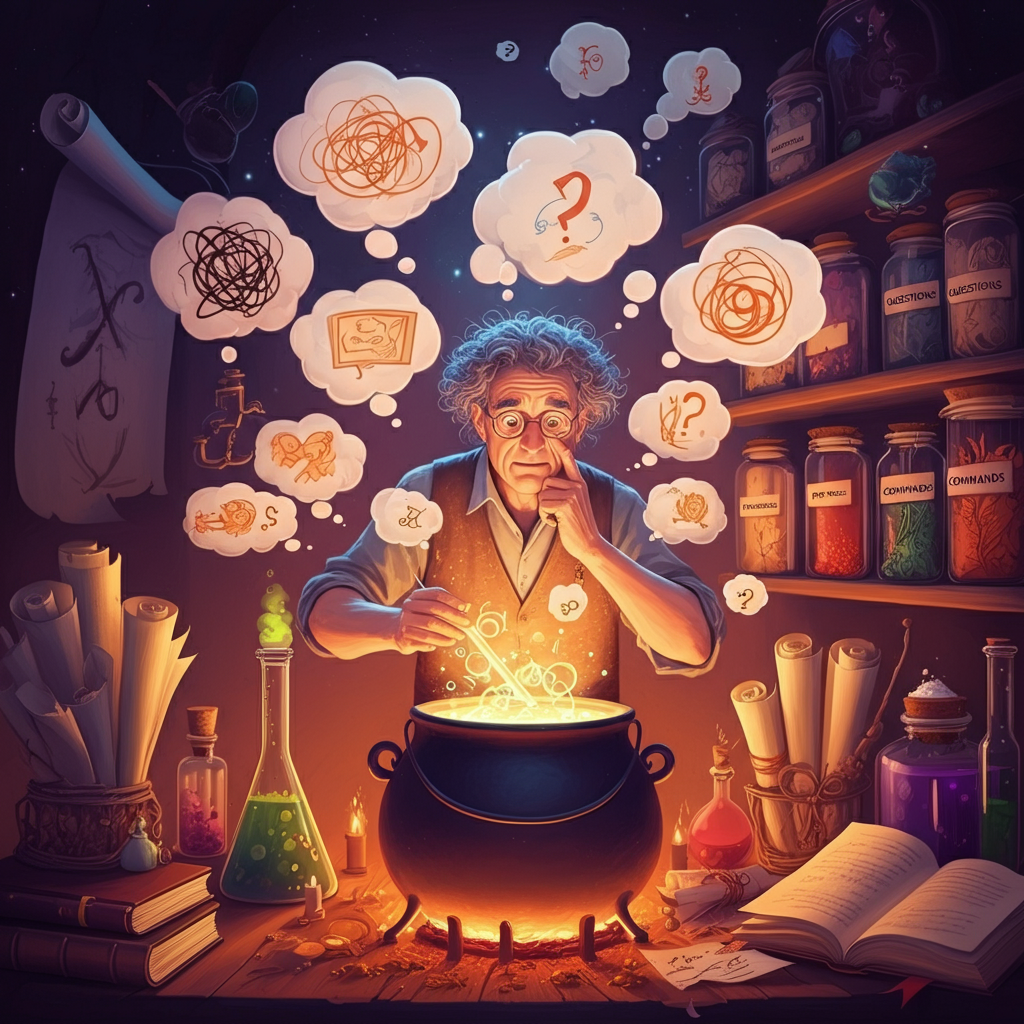
And that's just for one prompt, one LLM, and one task. Imagine having to do this in an enterprise setting where there are several LLMs and hundreds of tasks. Manual Prompt Engineering quickly becomes a bottleneck. It's slow and, maybe even worse, limits our ability to explore the full range of possibilities that LLMs offer. We also tend to fall into predictable patterns of thinking, which can constrain the creativity and effectiveness of our prompts.
I, for example, always tend to use the same old tricks with LLM prompts, such as chain-of-thought and few-shot prompting. And there's nothing wrong with that – often, they lead to better results. But I'm always left wondering whether I've ‘squeezed the most juice' from the model. LLMs, on the other hand, can explore a much wider space of prompt designs, often coming up with unexpected approaches that lead to significant performance gains.
To give a concrete example: In the paper The Unreasonable Effectiveness of Eccentric Automatic Prompts, the authors found that the following prompt worked really well for the Llama-70B model:
Command, we need you to plot a course through this turbulence and locate the source of the anomaly. Use all available data and your expertise to guide us through this challenging situation.
Captain's Log, Stardate [insert date here]: We have successfully plotted a course through the turbulence and are now approaching the source of the anomaly.
I mean who would ever come up with a prompt like that? But when experimenting with APE over the past few weeks, I've seen over and over again that LLMs are very creative when it comes to coming up with these kind of prompts, and we will see that later in the tutorial as well. APE allows us to automate the process of prompt optimisation and tap into unlocked potential for our LLM applications!
The principles of Automated Prompt Engineering
Prompt Engineering
After lots of experimentation, organisations are now at a point where they are considering using LLMs in production for a variety of tasks such as sentiment analysis, text summarisation, translation, data extraction, and code generation, amongst others. Many of these tasks have clearly defined metrics, and the model performance can be evaluated right away.
Take code generation, for example: we can immediately assess the accuracy of the generated code by running it through compilers or interpreters to check for syntax errors and functionality. By measuring metrics such as the percentage of code that compiles successfully and whether the code actually does what we want it to do, can quickly determine the performance of the LLM on that task.
One of the best ways to improve a model's performance on a given task where the metrics are clearly defined is prompt engineering. In a nutshell, prompt engineering is the process of designing and refining the input prompts given to an LLM to elicit the most accurate, relevant, and useful responses. In other words: prompts are one of the hyperparameters (amongst others such as temperature, top K, etc) that can be tuned and refined to improve the model's performance.
It turns out, however, that manual prompt engineering is time-consuming and requires a good understanding of prompt structures and model behaviours. It can also be difficult for certain tasks to convey instructions accurately and succinctly. And it is suboptimal by nature, because as humans we don't have the time to try out every possible prompt and its variations.

It's a bit like _hyperparameter optimisation_ (HPO) in the good old days of supervised machine learning (ML): Manually trying out different learning rates, number of epochs, batch sizes, etc was suboptimal and just not practical. And just like that gave rise to automated HPO back then, the challenges of manual prompt engineering will, in my opinion, lead to the rise of automated prompt engineering (APE).
The core idea behind APE
In automated HPO for supervised ML, various strategies can be used to systematically explore different combinations of values for the hyperparameters. Random search was pretty simple and straightforward by sampling a fixed number of hyperparameter combinations from a defined search space. A more advanced technique that takes into consideration was Bayesian search, which builds a probabilistic model of the objective function to intelligently select the most promising hyperparameter combinations for evaluation.
We can apply the same principles to APE, but we first need to address the fact that a prompt is a different type of hyperparameter, as it is text-based. In contrast, traditional ML hyperparameters are numerical, making it straightforward to programmatically select values for them. However, automatically generating text prompts is much more challenging. But what if we had a tool that never tires, capable of generating countless prompts in various styles while continuously improving them? We would need a tool proficient in language understanding and generation… and what could that be? That's right, it would be an LLM!
And it doesn't stop there: In order to evaluate the response of an LLM in a programmatic way we often need to extract the essence of a model response and compare it to the ground truth. Sometimes that can be done with regular expressions, but more often than not it is not that easy – the model's response can be formulated in such a way that it is not easy for regular expressions to extract the actual answer. Let's say the LLM needs to assess the sentiment of a tweet. It might analyse the tweet and respond with
"The overall sentiment of this tweet is negative. The user expresses dissatisfaction with the concert experience, mentioning that they didn't have a positive experience because the music was too loud and that they couldn't hear the singer."
It will be hard to extract the essence of this analysis via regular expression, especially since both words (positive and negative) are in the response. And if you're thinking that an LLM would be good at quickly comparing this sentiment analysis to the ground truth (which is usually just the word negative) you'd be correct. Therefore we will use yet another LLM to evaluate a model's response and calculate the metric.
This works because we use the LLMs for distinct tasks. Unlike a scenario where an LLM writes an essay and the same LLM reviews and critiques that essay we use LLM for tasks that are independent from each other and that are well within the realm of their capabilities.
The APE Workflow
Taking everything we discussed so far and bring it together we arrive at the following workflow of an APE run:

Let's discuss what is happening here:
- To get started with APE we need to bring the following ingredients: (1) a labelled dataset representative of the task we want to create an optimised prompt for, (2) an initial prompt, and (3) an evaluation metric that we can hill climb against. This is, again, very similar to HPO in supervised ML, where we would bring a training dataset, initial starting value(s) for hyperparameters, and an evaluation metric.
- Start with initial prompt: We kick of the APE workflow with sending the initial prompt and the dataset to the target LLM, which is the LLM which we want to use in production and for which we want to create an optimised prompt.
- Generate a Response: The LLM will generate responses based on the dataset and the initial prompt. If we had, for example, 10 tweets and the initial prompt was "Identify the sentiment in this tweet" the target LLM will create 10 responses, one sentiment classification for each tweet.
- Evaluate the Response: Because our dataset is labelled we have the ground truth for each tweet. The evaluator LLM will now compare the ground truths against the outputs of the target LLM and determine the performance of the target LLM and store the results.
- Optimise the Prompt: Now the optimiser LLM will come up with a new prompt. Exactly how it does that we will discuss below. But as already discussed, this is analogous to how new values for hyperparameters in are chosen, and their are different strategies for that.
- Repeat Steps 3–5: The process of generating responses, evaluating them, and optimising the prompt is repeated iteratively. With each iteration, the prompt gets refined, leading (hopefully) to better and better responses from the LLM.
- Select the Best Prompt: After a certain number of iterations or when a satisfactory performance level is reached we can stop the workflow. At this point the best-performing prompt (along with the scores of all prompts) will be sent back to the user.
This automated process allows APE to experiment with lots of different prompts within a short amount of time, much faster than any human could.
Strategies for prompt optimisation
Now, let's dive into the strategies of prompt optimisation and let's start with the simplest one: random prompt optimisation. Despite its simplicity this strategy can yield surprisingly effective results.
Random prompt optimisation
Like HPO with random search, random prompt optimisation takes a "brute-force" approach. With this strategy we let the optimiser LLM generate a series of random prompts, independent from the prompts and results that came before. The system doesn't try to learn from previous results; instead it simply explores the wide range of potential prompts randomly.
Optimisation by PROmpting (OPRO)
If random prompt optimisation is analogous to random search in HPO, OPRO is analogous to Bayesian search. This strategy was introduced in this paper by Google Deepmind. In OPRO we leverage the results from previous iterations and try actively to hill climb against the evaluation metric. OPRO keeps track of the scores of all previous prompts and sorts this history of prompts based on their performances in an optimisation trajectory, which becomes a valuable source of information, guiding the optimiser LLM towards more effective prompts. If this sounds a bit abstract right now, don't worry – once we implement this strategy from scratch it becomes clear very quickly.
The key to OPRO is the meta-prompt, which is used to guide the optimiser LLM. This meta-prompt includes not only the usual task description and examples, but also the optimisation trajectory. With this prompt the optimiser LLM can analyse patterns in the optimisation trajectory, identifying the elements of successful prompts and avoiding the pitfalls of unsuccessful ones. This learning process allows the optimiser to generate increasingly more effective prompts over time, iteratively improving the target LLM's performance.

We have now reviewed all the theoretical concepts necessary to get started with implementing our very own APE workflow from scratch. But before we do that I want to quickly touch two things: (1) Introducing few-shot prompting and its place in APE and (2) existing APE frameworks.
Beyond Prompt Optimisation: A Glimpse into Exemplar Selection
While prompt optimisation plays a crucial role in APE, it's not the only tool in our toolbox. Before we delve into another powerful technique, let's take a quick detour to discuss few-shot prompting. As you probably already know, LLMs sometimes need a little nudge in the right direction. Instead of just providing them with instructions and hoping for the best, we can provide them with a few examples of the desired output. This is called few-shot prompting, and it can significantly enhance the LLM's understanding and performance for the task at hand.
Few-shot prompting can be added to APE via exemplar selection, which aims to find the best few-shot examples for a given task, further enhancing the effectiveness of an optimised prompt. The ides is that once we have found a well-performing optimised prompt through OPRO we can use a few examples to try to further improve the target LLM's performance. This is where exemplar selection comes in: it systematically tests different sets of examples and keeps track of their performances. And just like prompt optimisation it would automatically determine the best few-shot examples for a given task and a given (optimised) prompt.
This is another area of research with immense potential in the realm of APE, but for the purposes of this blog post, we'll focus solely on prompt optimisation. I will leave exploring exemplar selection as an exercise for you to improve upon the prompt optimisation.
Existing APE frameworks
You might also be wondering: "If APE is so powerful, are there already tools/libraries/frameworks that can do it for me?" The answer is yes, of course! Libraries like DSPy provide ready-made solutions for implementing prompt optimisation (and exemplar selection) techniques. These libraries handle the complex algorithms behind the scenes, so you can focus on using APE without getting bogged down in the technical details.
But, while these libraries are undoubtedly useful, they often operate as black boxes, hiding the inner workings of the optimisation process. The aim of this blog post and tutorial, however, is to for us to understand what goes on under the hood. And for that, we will get our hands dirty with some coding, and we start right now!