Building a Batch Data Pipeline with Athena and MySQL

In this story I will speak about one of the most popular ways to run data transformation tasks – batch data processing. This data pipeline design pattern becomes incredibly useful when we need to process data in chunks making it very efficient for ETL jobs that require scheduling. I will demonstrate how it can be achieved by building a data transformation pipeline using MySQL and Athena. We will use infrastructure as code to deploy it in the cloud.
Imagine that you have just joined a company as a Data Engineer. Their data stack is modern, event-driven, cost-effective, flexible, and can scale easily to meet the growing data resources you have. External data sources and data pipelines in your data platform are managed by the data engineering team using a flexible environment setup with CI/CD GitHub integration.
As a data engineer you need to create a business intelligence dashboard that displays the geography of company revenue streams as shown below. Raw payment data is stored in the server database (MySQL). You want to build a batch pipeline that extracts data from that database daily, then use AWS S3 to store data files and Athena to process it.

Batch data pipeline
A data pipeline can be considered as a sequence of data processing steps. Due to logical data flow connections between these stages, each stage generates an output that serves as an input for the following stage.
There is a data pipeline whenever there is data processing between points A and B.
Data pipelines might be different due it their conceptual and logical nature. I previously wrote about it here [1]:
We would want to create a data pipeline where data is being transformed in the following steps:
- Use a Lambda function that extracts data from MySQL database tables
myschema.users
andmyschema.transactions
into S3 datalake bucket. - Add a State Machine node with Athena resource to start execution (
arn:aws:states:::athena:startQueryExecution.sync
) and create a database calledmydatabase
-
Create another data pipeline node to show existing tables in Athena database. Use the output of this node to perform required data transformations. If tables don't exist then we would want our pieline to create them in Athena based on the data from the datalake S3 bucket. We would want to create two external tables with data from MySQL:
- mydatabase.users (LOCATION ‘s3:///data/myschema/users/')
- mydatabase.transactions (LOCATION ‘s3:///data/myschema/transactions/')
Then we would want to create an optimized ICEBERG table:
- mydatabase.user_transactions (‘table_type'='ICEBERG', ‘format'='parquet') using the SQL below:
SELECT
date(dt) dt
, user_id
, sum(total_cost) total_cost_usd
, registration_date
FROM mydatabase.transactions
LEFT JOIN mydatabase.users
ON users.id = transactions.user_id
GROUP BY
dt
, user_id
, registration_date
;
- We will also use MERGE to update this table.
MERGE is an extremely useful SQL techniques for incremental updates in tables. Check my previous story [3] for more advanced examples:
Athena can analyse structured, unstructured and semi-structured data stored in Amazon S3 by running attractive ad-hoc SQL queries with no need to manage the infrastructure.
We don't need to load data and it makes it a perfect choice for our task.
It can be easily integrated with Busines Intelligence (BI) solutions such as Quichksight to generate reports. ICEBERG is an extremely useful and efficient table format where several separate programs can handle the same dataset concurrently and consistently [2]. I previously wrote about it here:
MySQL data connector
Let's create an AWS Lambda Function that will be able to execute SQL queries in MySQL database.
The code is pretty simple and generic. It can be used in any serverless application with any cloud service provider.
We will use it to extract revenue data into the datalake. Suggested Lambda folder structure can look as follows:
.
└── stack
├── mysql_connector
│ ├── config # config folder with environment related settings
│ ├── populate_database.sql # sql script to create source tables
│ ├── export.sql # sql script to export data to s3 datalake
│ └── app.py # main application file
├── package # required libraries
│ ├── PyMySQL-1.0.2.dist-info
│ └── pymysql
├── requirements.txt # required Python modules
└── stack.zip # Lambda package
We will integrate this tiny service into the pipeline using AWS Step functions for easy orchestration and visualisation.
To create a Lambda function that can extract data from MySQL database we need to create a folder for our Lambda first. Create a new folder called stackand then folder called
mysql_connector` in it:
mkdir stack
cd stack
mkdir mysql_connector
Then we can use this code below (replace database connection settings with yours) to create app.py
:
import os
import sys
import yaml
import logging
import pymysql
from datetime import datetime
import pytz
ENV = os.environ['ENV']
TESTING = os.environ['TESTING']
LAMBDA_PATH = os.environ['LAMBDA_PATH']
print('ENV: {}, Running locally: {}'.format(ENV, TESTING))
def get_work_dir(testing):
if (testing == 'true'):
return LAMBDA_PATH
else:
return '/var/task/' + LAMBDA_PATH
def get_settings(env, path):
if (env == 'staging'):
with open(path + "config/staging.yaml", "r") as f:
config = yaml.load(f, Loader=yaml.FullLoader)
elif (env == 'live'):
with open(path + "config/production.yaml", "r") as f:
config = yaml.load(f, Loader=yaml.FullLoader)
elif (env == 'test'):
with open(path + "config/test.yaml", "r") as f:
config = yaml.load(f, Loader=yaml.FullLoader)
else:
print('No config found')
return config
work_dir = get_work_dir(TESTING)
print('LAMBDA_PATH: {}'.format(work_dir))
config=get_settings(ENV, work_dir)
print(config)
DATA_S3 = config.get('S3dataLocation') # i.e. datalake.staging.something. Replace it with your unique bucket name.
logger = logging.getLogger()
logger.setLevel(logging.INFO)
# rds settings
rds_host = config.get('Mysql')['rds_host'] # i.e. "mymysqldb.12345.eu-west-1.rds.amazonaws.com"
user_name = "root"
password = "AmazingPassword"
db_name = "mysql"
# create the database connection outside of the handler to allow connections to be
# re-used by subsequent function invocations.
try:
conn = pymysql.connect(host=rds_host, user=user_name, passwd=password, db=db_name, connect_timeout=5)
except pymysql.MySQLError as e:
logger.error("ERROR: Unexpected error: Could not connect to MySQL instance.")
logger.error(e)
sys.exit()
logger.info("SUCCESS: Connection to RDS MySQL instance succeeded")
def lambda_handler(event, context):
processed = 0
print("")
try:
_populate_db()
_export_to_s3()
except Exception as e:
print(e)
message = 'Successfully populated the database and created an export job.'
return {
'statusCode': 200,
'body': { 'lambdaResult': message }
}
# Helpers:
def _now():
return datetime.utcnow().replace(tzinfo=pytz.utc).strftime('%Y-%m-%dT%H:%M:%S.%f')
def _populate_db():
try:
# Generate data and populate database:
fd = open(work_dir + '/populate_database.sql', 'r')
sqlFile = fd.read()
fd.close()
sqlCommands = sqlFile.split(';')
# Execute every command from the input file
for command in sqlCommands:
try:
with conn.cursor() as cur:
cur.execute(command)
print('---')
print(command)
except Exception as e:
print(e)
except Exception as e:
print(e)
def _export_to_s3():
try:
# Generate data and populate database:
fd = open(work_dir + '/export.sql', 'r')
sqlFile = fd.read()
fd.close()
sqlCommands = sqlFile.split(';')
# Execute every command from the input file
for command in sqlCommands:
try:
with conn.cursor() as cur:
cur.execute(command.replace("{{DATA_S3}}", DATA_S3))
print('---')
print(command)
except Exception as e:
print(e)
except Exception as e:
print(e)
To deploy our microservice using AWS CLI run this in your command line (assuming you are in the ./stack folder):
# Package Lambda code:
base=${PWD##*/}
zp=$base".zip" # This will return stack.zip if you are in stack folder.
echo $zp
rm -f $zp # remove old package if exists
pip install --target ./package pymysql
cd package
zip -r ../${base}.zip .
cd $OLDPWD
zip -r $zp ./mysql_connector
Make sure that AWS Lambda role exists before running the next part - role arn:aws:iam::
.
# Deploy packaged Lambda using AWS CLI:
aws
lambda create-function
--function-name mysql-lambda
--zip-file fileb://stack.zip
--handler /app.lambda_handler
--runtime python3.12
--role arn:aws:iam:::role/my-lambda-role
# # If already deployed then use this to update:
# aws --profile mds lambda update-function-code
# --function-name mysql-lambda
# --zip-file fileb://stack.zip;
Our MySQL instance must have S3 integration which enables data export to S3 bucket. It can be achieved by running this SQL query:
-- Example query
-- Replace table names and S3 bucket location
SELECT * FROM myschema.transactions INTO OUTFILE S3 's3:///data/myschema/transactions/transactions.scv' FIELDS TERMINATED BY ',' LINES TERMINATED BY 'n' OVERWRITE ON;
How to create MySQL instance
We can use CloudFormation template and infrastructure as code to create MySQL database. Consider this AWS command:
aws
cloudformation deploy
--template-file cfn_mysql.yaml
--stack-name MySQLDB
--capabilities CAPABILITY_IAM
It will use cfn_mysql.yaml
tempalte file to create CloudFormation stack called MySQLDB. I previously wrote about it here [4]:
Create MySQL and Postgres instances using AWS Cloudformation
Our cfn_mysql.yaml
should look like this:
AWSTemplateFormatVersion: 2010-09-09
Description: >-
This
template creates an Amazon Relational Database Service database instance. You
will be billed for the AWS resources used if you create a stack from this
template.
Parameters:
DBUser:
Default: root
NoEcho: 'true'
Description: The database admin account username
Type: String
MinLength: '1'
MaxLength: '16'
AllowedPattern: '[a-zA-Z][a-zA-Z0-9]*'
ConstraintDescription: must begin with a letter and contain only alphanumeric characters.
DBPassword:
Default: AmazingPassword
NoEcho: 'true'
Description: The database admin account password
Type: String
MinLength: '8'
MaxLength: '41'
AllowedPattern: '[a-zA-Z0-9]*'
ConstraintDescription: must contain only alphanumeric characters.
Resources:
### Role to output into s3
MySQLRDSExecutionRole:
Type: "AWS::IAM::Role"
Properties:
AssumeRolePolicyDocument:
Version: "2012-10-17"
Statement:
- Effect: "Allow"
Principal:
Service:
- !Sub rds.amazonaws.com
Action: "sts:AssumeRole"
Path: "/"
Policies:
- PolicyName: MySQLRDSExecutionPolicy
PolicyDocument:
Version: "2012-10-17"
Statement:
- Effect: Allow
Action:
- "s3:*"
Resource: "*"
###
RDSCluster:
Properties:
DBClusterParameterGroupName:
Ref: RDSDBClusterParameterGroup
Engine: aurora-mysql
MasterUserPassword:
Ref: DBPassword
MasterUsername:
Ref: DBUser
### Add a role to export to s3
AssociatedRoles:
- RoleArn: !GetAtt [ MySQLRDSExecutionRole, Arn ]
###
Type: "AWS::RDS::DBCluster"
RDSDBClusterParameterGroup:
Properties:
Description: "CloudFormation Sample Aurora Cluster Parameter Group"
Family: aurora-mysql5.7
Parameters:
time_zone: US/Eastern
### Add a role to export to s3
aws_default_s3_role: !GetAtt [ MySQLRDSExecutionRole, Arn ]
###
Type: "AWS::RDS::DBClusterParameterGroup"
RDSDBInstance1:
Type: 'AWS::RDS::DBInstance'
Properties:
DBClusterIdentifier:
Ref: RDSCluster
# AllocatedStorage: '20'
DBInstanceClass: db.t2.small
# Engine: aurora
Engine: aurora-mysql
PubliclyAccessible: "true"
DBInstanceIdentifier: MyMySQLDB
RDSDBParameterGroup:
Type: 'AWS::RDS::DBParameterGroup'
Properties:
Description: CloudFormation Sample Aurora Parameter Group
# Family: aurora5.6
Family: aurora-mysql5.7
Parameters:
sql_mode: IGNORE_SPACE
max_allowed_packet: 1024
innodb_buffer_pool_size: '{DBInstanceClassMemory*3/4}'
# Aurora instances need to be associated with a AWS::RDS::DBCluster via DBClusterIdentifier without the cluster you get these generic errors
If everything goes well we will see a new stack in our Amazon account:

Now we can use this MySQL instance in our pipeline. We can try our SQL queries in any SQL tool such as SQL Workbench to populate table data. These tables will be used later to create external tables using Athena and can be created using SQL:
CREATE TABLE IF NOT EXISTS
myschema.users AS
SELECT
1 AS id,
CURRENT_DATE() AS registration_date
UNION ALL
SELECT
2 AS id,
DATE_SUB(CURRENT_DATE(), INTERVAL 1 day) AS registration_date;
CREATE TABLE IF NOT EXISTS
myschema.transactions AS
SELECT
1 AS transaction_id,
1 AS user_id,
10.99 AS total_cost,
CURRENT_DATE() AS dt
UNION ALL
SELECT
2 AS transaction_id,
2 AS user_id,
4.99 AS total_cost,
CURRENT_DATE() AS dt
UNION ALL
SELECT
3 AS transaction_id,
2 AS user_id,
4.99 AS total_cost,
DATE_SUB(CURRENT_DATE(), INTERVAL 3 day) AS dt
UNION ALL
SELECT
4 AS transaction_id,
1 AS user_id,
4.99 AS total_cost,
DATE_SUB(CURRENT_DATE(), INTERVAL 3 day) AS dt
UNION ALL
SELECT
5 AS transaction_id,
1 AS user_id,
5.99 AS total_cost,
DATE_SUB(CURRENT_DATE(), INTERVAL 2 day) AS dt
UNION ALL
SELECT
6 AS transaction_id,
1 AS user_id,
15.99 AS total_cost,
DATE_SUB(CURRENT_DATE(), INTERVAL 1 day) AS dt
UNION ALL
SELECT
7 AS transaction_id,
1 AS user_id,
55.99 AS total_cost,
DATE_SUB(CURRENT_DATE(), INTERVAL 4 day) AS dt
;
Process data using Athena
Now we would want to add a data pipeline workflow that triggers our Lambda function to extract data from MySQL, save it in the datalake and then start data transformation in Athena.
We would want to create two external Athena tables with data from MySQL:
- myschema.users
- myschema.transactions
Then we would want to create an optimized ICEBERG table myschema.user_transactions to connect it to our BI solution.
We would want to INSERT new data into that table using MERGE statement.
CREATE EXTERNAL TABLE mydatabase.users (
id bigint
, registration_date string
)
ROW FORMAT DELIMITED
FIELDS TERMINATED BY ','
STORED AS INPUTFORMAT 'org.apache.hadoop.mapred.TextInputFormat'
OUTPUTFORMAT 'org.apache.hadoop.hive.ql.io.HiveIgnoreKeyTextOutputFormat'
LOCATION 's3:///data/myschema/users/' TBLPROPERTIES ( 'skip.header.line.count'='0')
;
select * from mydatabase.users;
CREATE EXTERNAL TABLE mydatabase.transactions (
transaction_id bigint
, user_id bigint
, total_cost double
, dt string
)
ROW FORMAT DELIMITED
FIELDS TERMINATED BY ','
STORED AS INPUTFORMAT 'org.apache.hadoop.mapred.TextInputFormat'
OUTPUTFORMAT 'org.apache.hadoop.hive.ql.io.HiveIgnoreKeyTextOutputFormat'
LOCATION 's3:///data/myschema/transactions/' TBLPROPERTIES ( 'skip.header.line.count'='0')
;
select * from mydatabase.transactions;
CREATE TABLE IF NOT EXISTS mydatabase.user_transactions (
dt date,
user_id int,
total_cost_usd float,
registration_date string
)
PARTITIONED BY (dt)
LOCATION 's3:///data/myschema/optimized-data-iceberg-parquet/'
TBLPROPERTIES (
'table_type'='ICEBERG',
'format'='parquet',
'write_target_data_file_size_bytes'='536870912',
'optimize_rewrite_delete_file_threshold'='10'
)
;
MERGE INTO mydatabase.user_transactions as ut
USING (
SELECT
date(dt) dt
, user_id
, sum(total_cost) total_cost_usd
, registration_date
FROM mydatabase.transactions
LEFT JOIN mydatabase.users
ON users.id = transactions.user_id
GROUP BY
dt
, user_id
, registration_date
) as ut2
ON (ut.dt = ut2.dt and ut.user_id = ut2.user_id)
WHEN MATCHED
THEN UPDATE
SET total_cost_usd = ut2.total_cost_usd, registration_date = ut2.registration_date
WHEN NOT MATCHED
THEN INSERT (
dt
,user_id
,total_cost_usd
,registration_date
)
VALUES (
ut2.dt
,ut2.user_id
,ut2.total_cost_usd
,ut2.registration_date
)
;
When new table is ready we can check it by running SELECT *
:

Orchestrate data pipeline using Step Functions (State Machine)
In the previous steps, we learned how to deploy each step of the data pipeline separately and then test it. In this paragraph, we will see how to create a complete data pipeline with required resources using infrastructure such as code and pipeline orchestration tool such as AWS Step Functions (State Machine). When we finish the pipeline graph will look like this:

Data pipeline orchestration is a great data engineering technique that adds interactivity to our data pipelines. The idea was previously explained in one of my stories [5]:
To deploy the complete orchestrator solution including all required resources we can use CloudFormation (infrastructure as code). Consider this shell script below that can be run from the command line when we are in the /stack
folder. Make sure exists and replace it with your actual S3 bucket::
#!/usr/bin/env bash
# chmod +x ./deploy-staging.sh
# Run ./deploy-staging.sh
PROFILE=
STACK_NAME=BatchETLpipeline
LAMBDA_BUCKET= # Replace with unique bucket name in your account
APP_FOLDER=mysql_connector
date
TIME=`date +"%Y%m%d%H%M%S"`
base=${PWD##*/}
zp=$base".zip"
echo $zp
rm -f $zp
pip install --target ./package -r requirements.txt
# boto3 is not required unless we want a specific version for Lambda
# requirements.txt:
# pymysql==1.0.3
# requests==2.28.1
# pytz==2023.3
# pyyaml==6.0
cd package
zip -r ../${base}.zip .
cd $OLDPWD
zip -r $zp "./${APP_FOLDER}" -x __pycache__
# Check if Lambda bucket exists:
LAMBDA_BUCKET_EXISTS=$(aws --profile ${PROFILE} s3 ls ${LAMBDA_BUCKET} --output text)
# If NOT:
if [[ $? -eq 254 ]]; then
# create a bucket to keep Lambdas packaged files:
echo "Creating Lambda code bucket ${LAMBDA_BUCKET} "
CREATE_BUCKET=$(aws --profile ${PROFILE} s3 mb s3://${LAMBDA_BUCKET} --output text)
echo ${CREATE_BUCKET}
fi
# Upload the package to S3:
aws --profile $PROFILE s3 cp ./${base}.zip s3://${LAMBDA_BUCKET}/${APP_FOLDER}/${base}${TIME}.zip
aws --profile $PROFILE
cloudformation deploy
--template-file stack.yaml
--stack-name $STACK_NAME
--capabilities CAPABILITY_IAM
--parameter-overrides
"StackPackageS3Key"="${APP_FOLDER}/${base}${TIME}.zip"
"AppFolder"=$APP_FOLDER
"S3LambdaBucket"=$LAMBDA_BUCKET
"Environment"="staging"
"Testing"="false"
It will use stack.yaml to create a CloudFormation stack called BatchETLpipeline. It will package our Lambda function, create a package and upload it into S3 bucket. If this bucket doesn't exist it will create it. It will then deploy the pipeline.
AWSTemplateFormatVersion: '2010-09-09'
Description: An example template for a Step Functions state machine.
Parameters:
DataLocation:
Description: Data lake bucket with source data files.
Type: String
Default: s3://your.datalake.aws/data/
AthenaResultsLocation:
Description: S3 location for Athena query results.
Type: String
Default: s3://your.datalake.aws/athena/
AthenaDatabaseName:
Description: Athena schema names for ETL pipeline.
Type: String
Default: mydatabase
S3LambdaBucket:
Description: Use this bucket to keep your Lambda package.
Type: String
Default: your.datalake.aws
StackPackageS3Key:
Type: String
Default: mysql_connector/stack.zip
ServiceName:
Type: String
Default: mysql-connector
Testing:
Type: String
Default: 'false'
AllowedValues: ['true','false']
Environment:
Type: String
Default: 'staging'
AllowedValues: ['staging','live','test']
AppFolder:
Description: app.py file location inside the package, i.e. mysql_connector when ./stack/mysql_connector/app.py.
Type: String
Default: mysql_connector
Resources:
LambdaExecutionRole:
Type: "AWS::IAM::Role"
Properties:
AssumeRolePolicyDocument:
Version: "2012-10-17"
Statement:
- Effect: Allow
Principal:
Service: lambda.amazonaws.com
Action: "sts:AssumeRole"
MyLambdaFunction:
Type: "AWS::Lambda::Function"
Properties:
Handler: "index.handler"
Role: !GetAtt [ LambdaExecutionRole, Arn ]
Code:
ZipFile: |
exports.handler = (event, context, callback) => {
callback(null, "Hello World!");
};
Runtime: "nodejs18.x"
Timeout: "25"
### MySQL Connector Lmabda ###
MySqlConnectorLambda:
Type: AWS::Lambda::Function
DeletionPolicy: Delete
DependsOn: LambdaPolicy
Properties:
FunctionName: !Join ['-', [!Ref ServiceName, !Ref Environment] ]
Handler: !Sub '${AppFolder}/app.lambda_handler'
Description: Microservice that extracts data from RDS.
Environment:
Variables:
DEBUG: true
LAMBDA_PATH: !Sub '${AppFolder}/'
TESTING: !Ref Testing
ENV: !Ref Environment
Role: !GetAtt LambdaRole.Arn
Code:
S3Bucket: !Sub '${S3LambdaBucket}'
S3Key:
Ref: StackPackageS3Key
Runtime: python3.8
Timeout: 360
MemorySize: 128
Tags:
-
Key: Service
Value: Datalake
StatesExecutionRole:
Type: "AWS::IAM::Role"
Properties:
AssumeRolePolicyDocument:
Version: "2012-10-17"
Statement:
- Effect: "Allow"
Principal:
Service:
- !Sub states.${AWS::Region}.amazonaws.com
Action: "sts:AssumeRole"
Path: "/"
Policies:
- PolicyName: StatesExecutionPolicy
PolicyDocument:
Version: "2012-10-17"
Statement:
- Effect: Allow
Action:
- "lambda:InvokeFunction"
Resource: "*"
- Effect: Allow
Action:
- "athena:*"
Resource: "*"
- Effect: Allow
Action:
- "s3:*"
Resource: "*"
- Effect: Allow
Action:
- "glue:*"
Resource: "*"
MyStateMachine:
Type: AWS::StepFunctions::StateMachine
Properties:
# StateMachineName: ETL-StateMachine
StateMachineName: !Join ['-', ['ETL-StateMachine', !Ref ServiceName, !Ref Environment] ]
DefinitionString:
!Sub
- |-
{
"Comment": "A Hello World example using an AWS Lambda function",
"StartAt": "HelloWorld",
"States": {
"HelloWorld": {
"Type": "Task",
"Resource": "${lambdaArn}",
"Next": "Extract from MySQL"
},
"Extract from MySQL": {
"Resource": "${MySQLLambdaArn}",
"Type": "Task",
"Next": "Create Athena DB"
},
"Create Athena DB": {
"Resource": "arn:aws:states:::athena:startQueryExecution.sync",
"Parameters": {
"QueryString": "CREATE DATABASE if not exists ${AthenaDatabaseName}",
"WorkGroup": "primary",
"ResultConfiguration": {
"OutputLocation": "${AthenaResultsLocation}"
}
},
"Type": "Task",
"Next": "Show tables"
},
"Show tables": {
"Resource": "arn:aws:states:::athena:startQueryExecution.sync",
"Parameters": {
"QueryString": "show tables in ${AthenaDatabaseName}",
"WorkGroup": "primary",
"ResultConfiguration": {
"OutputLocation": "${AthenaResultsLocation}"
}
},
"Type": "Task",
"Next": "Get Show tables query results"
},
"Get Show tables query results": {
"Resource": "arn:aws:states:::athena:getQueryResults",
"Parameters": {
"QueryExecutionId.$": "$.QueryExecution.QueryExecutionId"
},
"Type": "Task",
"Next": "Decide what next"
},
"Decide what next": {
"Comment": "Based on the input table name, a choice is made for moving to the next step.",
"Type": "Choice",
"Choices": [
{
"Not": {
"Variable": "$.ResultSet.Rows[0].Data[0].VarCharValue",
"IsPresent": true
},
"Next": "Create users table (external)"
},
{
"Variable": "$.ResultSet.Rows[0].Data[0].VarCharValue",
"IsPresent": true,
"Next": "Check All Tables"
}
],
"Default": "Check All Tables"
},
"Create users table (external)": {
"Resource": "arn:aws:states:::athena:startQueryExecution.sync",
"Parameters": {
"QueryString": "CREATE EXTERNAL TABLE ${AthenaDatabaseName}.users ( id bigint , registration_date string ) ROW FORMAT DELIMITED FIELDS TERMINATED BY ',' STORED AS INPUTFORMAT 'org.apache.hadoop.mapred.TextInputFormat' OUTPUTFORMAT 'org.apache.hadoop.hive.ql.io.HiveIgnoreKeyTextOutputFormat' LOCATION 's3://datalake.staging.liveproject/data/myschema/users/' TBLPROPERTIES ( 'skip.header.line.count'='0') ;",
"WorkGroup": "primary",
"ResultConfiguration": {
"OutputLocation": "${AthenaResultsLocation}"
}
},
"Type": "Task",
"Next": "Create transactions table (external)"
},
"Create transactions table (external)": {
"Resource": "arn:aws:states:::athena:startQueryExecution.sync",
"Parameters": {
"QueryString": "CREATE EXTERNAL TABLE ${AthenaDatabaseName}.transactions ( transaction_id bigint , user_id bigint , total_cost double , dt string ) ROW FORMAT DELIMITED FIELDS TERMINATED BY ',' STORED AS INPUTFORMAT 'org.apache.hadoop.mapred.TextInputFormat' OUTPUTFORMAT 'org.apache.hadoop.hive.ql.io.HiveIgnoreKeyTextOutputFormat' LOCATION 's3://datalake.staging.liveproject/data/myschema/transactions/' TBLPROPERTIES ( 'skip.header.line.count'='0') ;",
"WorkGroup": "primary",
"ResultConfiguration": {
"OutputLocation": "${AthenaResultsLocation}"
}
},
"Type": "Task",
"Next": "Create report table (parquet)"
},
"Create report table (parquet)": {
"Resource": "arn:aws:states:::athena:startQueryExecution.sync",
"Parameters": {
"QueryString": "CREATE TABLE IF NOT EXISTS ${AthenaDatabaseName}.user_transactions ( dt date, user_id int, total_cost_usd float, registration_date string ) PARTITIONED BY (dt) LOCATION 's3://datalake.staging.liveproject/data/myschema/optimized-data-iceberg-parquet/' TBLPROPERTIES ( 'table_type'='ICEBERG', 'format'='parquet', 'write_target_data_file_size_bytes'='536870912', 'optimize_rewrite_delete_file_threshold'='10' ) ;",
"WorkGroup": "primary",
"ResultConfiguration": {
"OutputLocation": "${AthenaResultsLocation}"
}
},
"Type": "Task",
"End": true
},
"Check All Tables": {
"Type": "Map",
"InputPath": "$.ResultSet",
"ItemsPath": "$.Rows",
"MaxConcurrency": 0,
"Iterator": {
"StartAt": "CheckTable",
"States": {
"CheckTable": {
"Type": "Choice",
"Choices": [
{
"Variable": "$.Data[0].VarCharValue",
"StringMatches": "*users",
"Next": "passstep"
},
{
"Variable": "$.Data[0].VarCharValue",
"StringMatches": "*user_transactions",
"Next": "Insert New parquet Data"
}
],
"Default": "passstep"
},
"Insert New parquet Data": {
"Resource": "arn:aws:states:::athena:startQueryExecution.sync",
"Parameters": {
"QueryString": "MERGE INTO ${AthenaDatabaseName}.user_transactions as ut USING ( SELECT date(dt) dt , user_id , sum(total_cost) total_cost_usd , registration_date FROM ${AthenaDatabaseName}.transactions LEFT JOIN ${AthenaDatabaseName}.users ON users.id = transactions.user_id GROUP BY dt , user_id , registration_date ) as ut2 ON (ut.dt = ut2.dt and ut.user_id = ut2.user_id) WHEN MATCHED THEN UPDATE SET total_cost_usd = ut2.total_cost_usd, registration_date = ut2.registration_date WHEN NOT MATCHED THEN INSERT ( dt ,user_id ,total_cost_usd ,registration_date ) VALUES ( ut2.dt ,ut2.user_id ,ut2.total_cost_usd ,ut2.registration_date ) ;",
"WorkGroup": "primary",
"ResultConfiguration": {
"OutputLocation": "${AthenaResultsLocation}"
}
},
"Type": "Task",
"End": true
},
"passstep": {
"Type": "Pass",
"Result": "NA",
"End": true
}
}
},
"End": true
}
}
}
- {
lambdaArn: !GetAtt [ MyLambdaFunction, Arn ],
MySQLLambdaArn: !GetAtt [ MySqlConnectorLambda, Arn ],
AthenaResultsLocation: !Ref AthenaResultsLocation,
AthenaDatabaseName: !Ref AthenaDatabaseName
}
RoleArn: !GetAtt [ StatesExecutionRole, Arn ]
Tags:
-
Key: "keyname1"
Value: "value1"
-
Key: "keyname2"
Value: "value2"
# IAM role for mysql-data-connector Lambda:
LambdaRole:
Type: AWS::IAM::Role
Properties:
AssumeRolePolicyDocument:
Version: "2012-10-17"
Statement:
-
Effect: Allow
Principal:
Service:
- "lambda.amazonaws.com"
Action:
- "sts:AssumeRole"
LambdaPolicy:
Type: AWS::IAM::Policy
DependsOn: LambdaRole
Properties:
Roles:
- !Ref LambdaRole
PolicyName: !Join ['-', [!Ref ServiceName, !Ref Environment, 'lambda-policy']]
PolicyDocument:
{
"Version": "2012-10-17",
"Statement": [
{
"Effect": "Allow",
"Action": [
"logs:CreateLogGroup",
"logs:CreateLogStream",
"logs:PutLogEvents"
],
"Resource": "*"
}
]
}
If everything goes well the stack for our new data pipeline will be deployed:

If we click the State Machine resource, then click ‘Edit' we will see our ETL pipeline as a graph:

Now we can execute the pipeline to run all required data transformation steps. Click ‘Start execution'.

Now we can connect our Athena tables to our BI solution. Connect our final Athena dataset mydataset.user_transactions
to create a dashboard.

We just need to adjust a couple of settings to make our dashboard look like this:
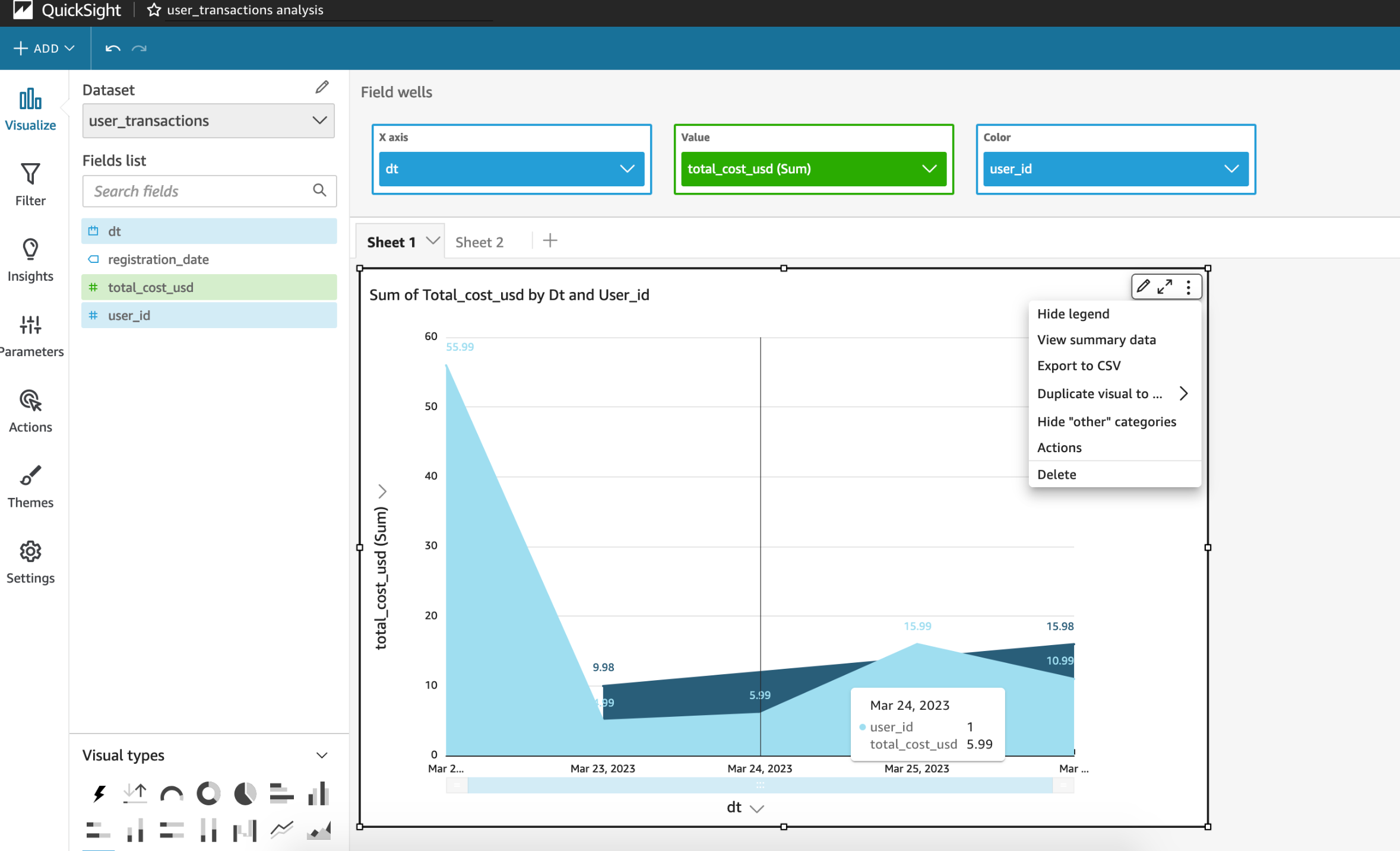
We would want to use dt
as dimension and total_cost_usd
as metric. We also can set a breakdown dimension for each user_id
.
Conclusion
Batch data pipelines are popular because historically workloads were primarily batch-oriented in data environments. We have just built an ETL data pipeline to extract data from MySQL and transform it in datalake. This pattern works best for datasets that aren't very large and require continuous processing because Athena charges according to the volume of data scanned. The method works well when converting data into columnar formats like Parquet or ORC, combining several tiny files into bigger ones, or bucketing and adding partitions. I previously wrote about these Big Data file formats in one of my stories [6]:
We learned how to use Step Functions to orchestrate the Data Pipeline and visualise the data flow from source to final consumer and deploy it using infrastructure as code. This setup makes it possible to use CI/CD techniques for our data pipelines [7].
I hope this tutorial was useful for you. Let me know if you have any questions.
Recommended read
[1] https://towardsdatascience.com/data-pipeline-design-patterns-100afa4b93e3
[2] https://medium.com/towards-data-science/introduction-to-apache-iceberg-tables-a791f1758009
[3] https://medium.com/towards-data-science/advanced-sql-techniques-for-beginners-211851a28488
[5] https://medium.com/towards-data-science/data-pipeline-orchestration-9887e1b5eb7a
[6] https://medium.com/towards-data-science/big-data-file-formats-explained-275876dc1fc9