Building a Knowledge Graph From Scratch Using LLMs
Turn your Pandas data frame into a knowledge graph using LLMs. Build your own LLM graph-builder from scratch, implement LLMGraphTransformer by LangChain, and QA your KG.

In today's AI world, knowledge graphs are becoming increasingly important, as they enable many of the knowledge retrieval systems behind LLMs. Many data science teams across companies are investing heavily in retrieval augmented generation (RAG), as it's an efficient way to improve the output accuracy of LLMs and prevent hallucinations.
But there is more to it; on a personal note, graph-RAG is democratizing the AI space. This is because before if we wanted to customize a model to a use-case – either for fun or business – we would have three options: pre-training the model providing a bigger exposure to a dataset within the industry of your use-case, fine-tuning the model on a specific dataset, and context prompting.
As for pre-training, this option is incredibly expensive and technical, and it's not an option for most developers.
Fine-tuning is easier than pre-training, and although the cost of fine-tuning depends on the model and the training corpus, it's generally a more affordable option. This one was the go-to strategy for most of the AI developers. However, new models are released every week and you would need to fine-tune a new model constantly.
The third option involves providing the knowledge directly in the prompt. However, this works only when the knowledge required for the model to learn is relatively small. Even though the context for the models is getting bigger and bigger, the accuracy of recalling an element is inversely correlated to the size of the context provided.
Neither of the three options sounds like the right one. Is there another option for the model to learn all the knowledge required to be specialized in a certain task or topic? No.
But, the model doesn't need to learn all the knowledge at once, as when we interrogate the LLM we are likely trying to get one or a few pieces of information. Here comes graph-RAG to help, by providing an informational retrieval that based on the query retrieves the information needed, without requiring any further training.
Let's take a look at what a Graph-RAG looks like:
- Graph Building: here, we create nodes (entities) and edges (relationships) from a data source and load them into our KG. This is usually a more manual step where we use a query language, often OpenCypher to upload both entities and connect them to each other via edges.
- Node indexing: this step involves creating a data structure that will allow us to retrieve the data efficiently. In knowledge graphs, this typically involves creating a vector search index, where every index is associated with a vector embedding.
- Graph retriever: here, we build a retrieval function that will allow us to compute a similarity score and retrieve the most relevant nodes that will be used as context for the LLM to provide an answer. In the simplest case, we compute the cosine similarity over the query, which is converted to a vector embedding, and all the vector embeddings in the vector index.
- RAG Evaluation: the last step is useful to actually measure the accuracy and performance of the LLM. It's useful during experimentation to perform how different LLMs, and RAG frameworks perform on your specific use case.
Now that we have an overall view of what a RAG pipeline consists of, we may be attracted to just jump and experiment with complex math functions for graph retrieval to guarantee the best information retrieval accuracy possible. But … hold on. We don't have a knowledge graph yet. This step may seem like the classic data cleaning and preprocessing step in data science (boring…). But what if I tell you there is a better alternative? An option that introduces more science and automation. Indeed, recent studies are focusing on how to automate the construction of a Knowledge Graph, since this step is key for good information retrieval. Just think about it, if the data in the KG is not good, there is no way your graph-RAG will have a state-of-the-art performance.
In this article, we will delve into the first step: How to build a knowledge graph without actually building it.
From CSV to Knowledge Graph
Now, let's go over a practical example to make things more concrete. Let's tackle one of the most important existential issues: what movie to watch? … How many times have you been bored, and tired from work and the only thing you could do was watch a movie? You start scrolling among movies until you realize that two hours have passed by.
To solve this issue, let's create a knowledge graph using a Wikipedia Movie Dataset, and chat with the KG. Firstly, let's implement a "from scratch" solution using LLMs. Then, let's look at one of the latest implementations via LangChain (still in the experimental phase as of November 2024), one of the most popular and powerful LLM frameworks available, and another popular solution with LlamaIndex.
Let's download this public dataset from Kaggle (License: CC BY-SA 4.0):
Or if you are lazy, just go ahead and clone my GitHub repo:
The folder knowledge-builder
contains both the Jupyter Notebook and the data we will be covering in this article.
Prerequisites
Before we can start, we need to have access to Neo4j Desktop and an LLM API Key or a local LLM. If you already have them, feel free to skip this section and jump to the action. If not, let's set them up, and don't worry it will be completely free.
There are several ways to leverage Neo4j, but for the sake of simplicity we will use Neo4j desktop, hence we will host the database locally. But it's a small dataset, so you won't be destroying your laptop by running this application.
To install Neo4j simply visit the Neo4j Desktop Download Page and click Download. Open Neo4j Desktop after installation. Sign in or create a Neo4j account (required to activate the software).
Once logged in, create a New Project:
- Click on the
+
button in the top-left corner. - Name your project (e.g., "Wiki Movie KG").
Inside your project, click on Add Database
. Select Local DBMS and click Create a Local Graph.
Configure your database:
- Name: Enter a name (e.g.,
neo4j
). - Password: Set a password (e.g.,
ilovemovies
). Remember this password for later.
Click Create to initialize the database.
Next, let's move to our LLM. The preferred way of running this notebook is using Ollama. Ollama is a locally hosted LLM solution that lets you download and set up LLMs on your laptop really really easily. It supports many open-source LLMs including Llama by Meta and Gemma by Google. I prefer this step as running your local LLM is free (excluding the degradation/energy cost of your laptop), and private, and it's just more exciting.
To download Ollama visit Ollama's official website, and download the installer for your operating system. Open the Ollama application after installation.
Open a terminal and use the following command to list available models:
ollama list
Install and run a model. we will be using qwen2.5-coder:latest
, which is a 7B Language Model fine-tuned on code tasks.
ollama run qwen2.5-coder:latest
Verify the installation:
ollama list
You should now see:
qwen2.5-coder:latest
Another free alternative is Gemini by Google, which lets us run 1500 requests per day. This solution actually outperforms the previous one since we are using a bigger and more powerful model. However, you may hit the limit depending on how many times you execute the script in a day.
To get a free API Key with Gemini, visit the website and click "Get an API Key". Then follow the instructions and copy the API key generated. We will use it in a moment.
Graph Builder from Scratch
Let's start by importing a few libraries required for the project:
# Type hints
from typing import Any, Dict, List, Tuple
# Standard library
import ast
import logging
import re
import warnings
# Third-party packages - Data manipulation
import pandas as pd
from tqdm import tqdm
# Third-party packages - Environment & Database
from dotenv import load_dotenv
from neo4j import GraphDatabase
# Third-party packages - Error handling & Retry logic
from tenacity import retry, stop_after_attempt, wait_exponential
# Langchain - Core
from langchain.chains import GraphCypherQAChain
from langchain.prompts import PromptTemplate
from langchain_core.documents import Document
# Langchain - Models & Connectors
from langchain_google_genai import ChatGoogleGenerativeAI, GoogleGenerativeAI
from langchain_ollama.llms import OllamaLLM
# Langchain - Graph & Experimental
from langchain_community.graphs import Neo4jGraph
from langchain_experimental.graph_transformers import LLMGraphTransformer
# Suppress warnings
warnings.filterwarnings('ignore')
# Load environment variables
load_dotenv()
As you can see, LangChain doesn't do a good job with the code organization, resulting in quite a few lines of import. Let's break down the libraries we are importing:
os
anddotenv
: Help us manage environment variables (like database credentials).pandas
: Used to handle and process the movie dataset.neo4j
: This library connects Python to the Neo4j graph database.langchain
: Provides tools to work with Language Models (LLMs) and graphs.tqdm
: Adds a nice UI to print statements. We will use it to show a progress bar in loops, so we know how much processing is left.warnings
: Suppresses unnecessary warnings for a cleaner output.
We load the movie dataset, which contains information about 34,886 movies from around the world. The dataset is publicly available on Kaggle (License: CC BY-SA 4.0). However, if you cloned my GitHub repo, the dataset will already be present in the data folder:
movies = pd.read_csv('data/wiki_movies.csv') # adjust the path if you manually downloaded the dataset
movies.head()
Here, we can see the following features:
- Release Year: The year in which the movie was released
- Title: The movie title
- _Origin/Ethnicity: The o_rigin of the movie (i.e. American, Bollywood, Tamil, etc.)
- Director: Director(s)
- Plot: Main actor and actresses
- Genre: Movie Genre(s)
- Wiki Page: URL of the Wikipedia page from which the plot description was scraped
- Plot: Long-form description of movie plot
By looking at these features, we could quickly come up with some of the labels and relationships we would like to see in our KG. Since this is a movie dataset, a movie would be one of them. Moreover, we may be interested in querying for specific actors and directors. Hence, we end up with three labels for our nodes: Movie, Actor, and Director. Of course, we could include more labels. However, let's stop here for the sake of simplicity.
For the sake of simplicity, let's clean a little bit this dataset, and extract only the first 1000 rows:
def clean_data(df: pd.DataFrame) -> pd.DataFrame:
"""Clean and preprocess DataFrame.
Args:
data: Input DataFrame
Returns:
Cleaned DataFrame
"""
df.drop(["Wiki Page"], axis=1, inplace=True)
# Drop duplicates
df = df.drop_duplicates(subset='Title', keep='first')
# Get object columns
col_obj = df.select_dtypes(include=["object"]).columns
# Clean string columns
for col in col_obj:
# Strip whitespace
df[col] = df[col].str.strip()
# Replace unknown/empty values
df[col] = df[col].apply(
lambda x: None if pd.isna(x) or x.lower() in ["", "unknown"]
else x.capitalize()
)
# Drop rows with any null values
df = df.dropna(how="any", axis=0)
return df
movies = clean_data(movies).head(1000)
movies.head()
Here, we are dropping the Wiki Page
column, which contains the link to the Wikipedia page. However, feel free to keep it, as this could be a property for the Movie
nodes. Next, we drop all the duplicates by title, and we clean all the string (object
) columns. Lastly, we keep only the first 1000 movies.
Since our knowledge graph will be hosted on Neo4j, let's set up a helper class to establish the connection and provide useful methods:
class Neo4jConnection:
def __init__(self, uri, user, password):
self.driver = GraphDatabase.driver(uri, auth=(user, password))
def close(self):
self.driver.close()
print("Connection closed")
def reset_database(self):
with self.driver.session() as session:
session.run("MATCH (n) DETACH DELETE n")
print("Database resetted successfully!")
def execute_query(self, query, parameters=None):
with self.driver.session() as session:
result = session.run(query, parameters or {})
return [record for record in result]
In the initialization (__init__
), we set up the connection to the Neo4j database using the database URL (uri
), username, and password. We will pass these variables later when we initialize the class.
The methodclose
terminates the connection to the database.
reset_database
deletes all nodes and relationships in the database using the Cypher command MATCH (n) DETACH DELETE n
.
execute_query
runs a given query (like adding a movie or fetching relationships) and returns the results.
Next, let's connect to the database using the helper class:
uri = "bolt://localhost:7687"
user = "neo4j"
password = "your_password_here"
conn = Neo4jConnection(uri, user, password)
conn.reset_database()
By default uri
, and user
will match the ones provided above. As for password
, this will be the one you defined while creating the database. Moreover, let's reset_database
to ensure we start with a clean slate by removing any existing data.
If you encounter any errors related to APOC not being installed in your database, go to Neo4j -> click on the database -> Plugins -> Install APOC:

We now need to take each movie from the dataset and turn it into a node in our graph. In this section, we will do it manually, whereas in the next sections, we will leverage an LLM to do it for us.
def parse_number(value: Any, target_type: type) -> Optional[float]:
"""Parse string to number with proper error handling."""
if pd.isna(value):
return None
try:
cleaned = str(value).strip().replace(',', '')
return target_type(cleaned)
except (ValueError, TypeError):
return None
def clean_text(text: str) -> str:
"""Clean and normalize text fields."""
if pd.isna(text):
return ""
return str(text).strip().title()
Let's create two short functions – parse_number
and clean_text
– to convert data into numbers for numerical columns, and properly format the text columns. If the conversion fails (e.g., if the value is empty), they return None
in case of a numerical column, and an empty string for object columns.
Next, let's create a to iteratively load data into our KG:
def load_movies_to_neo4j(movies_df: pd.DataFrame, connection: GraphDatabase) -> None:
"""Load movie data into Neo4j with progress tracking and error handling."""
logger = logging.getLogger(__name__)
logger.setLevel(logging.INFO)
# Query templates
MOVIE_QUERY = """
MERGE (movie:Movie {title: $title})
SET movie.year = $year,
movie.origin = $origin,
movie.genre = $genre,
movie.plot = $plot
"""
DIRECTOR_QUERY = """
MATCH (movie:Movie {title: $title})
MERGE (director:Director {name: $name})
MERGE (director)-[:DIRECTED]->(movie)
"""
ACTOR_QUERY = """
MATCH (movie:Movie {title: $title})
MERGE (actor:Actor {name: $name})
MERGE (actor)-[:ACTED_IN]->(movie)
"""
# Process each movie
for _, row in tqdm(movies_df.iterrows(), total=len(movies_df), desc="Loading movies"):
try:
# Prepare movie parameters
movie_params = {
"title": clean_text(row["Title"]),
"year": parse_number(row["Release Year"], int),
"origin": clean_text(row["Origin/Ethnicity"]),
"genre": clean_text(row["Genre"]),
"plot": str(row["Plot"]).strip()
}
# Create movie node
connection.execute_query(MOVIE_QUERY, parameters=movie_params)
# Process directors
for director in str(row["Director"]).split(" and "):
director_params = {
"name": clean_text(director),
"title": movie_params["title"]
}
connection.execute_query(DIRECTOR_QUERY, parameters=director_params)
# Process cast
if pd.notna(row["Cast"]):
for actor in row["Cast"].split(","):
actor_params = {
"name": clean_text(actor),
"title": movie_params["title"]
}
connection.execute_query(ACTOR_QUERY, parameters=actor_params)
except Exception as e:
logger.error(f"Error loading {row['Title']}: {str(e)}")
continue
logger.info("Finished loading movies to Neo4j")
Two important keywords to know to understand the Cypher query above are MERGE
and SET
.
MERGE
ensures the node or relationship exists; if not, it creates it. Therefore, it combines both the MATCH
, and CREATE
clauses, where MATCH
allows us to search within the graph for a certain structure, and CREATE
to create nodes and relationships. Therefore, MERGE
first check if the node/edge we are creating doesn't exist, if it doesn't then it creates it.
In the function above we use MERGE
to create a node for every Movie, Director, and Actor. In particular, since we have features for actors (Star1, Star2, Star3, and Star4), we create an Actor node for each column.
Next, we create two relationships: one from Director to Movie (DIRECTED
), and from Actor to Movie (ACTED_IN
).
SET
is used to update node or edge properties. In this case, we are providing the Movie node with Year, Rating, Genre, Runtime, and Overview; and the Director, and Actor with a Name property.
Also, note that we are using the special symbol $
to define a parameter.
Next, let's call the function and load all the movies:
load_movies_to_neo4j(movies, conn)
It will take approximately a minute to load all 1000 movies.
Once, the execution is completed, let's run a Cypher query to check if the movies are uploaded correctly:
query = """
MATCH (m:Movie)-[:ACTED_IN]-(a:Actor)
RETURN m.title, a.name
LIMIT 10;
"""
results = conn.execute_query(query)
for record in results:
print(record)
This query should now look familiar. We used MATCH
to find patterns in the graph.
(m:Movie)
: Matches nodes labeled "Movie".[:ACTED_IN]
: Matches relationships of type "ACTED_IN".(a:Actor)
: Matches nodes labeled "Actor".
Also, we used RETURN
to specify what to display—in this case, movie titles and actor names, andLIMIT
to restrict the result to the first 10 matches.
You should get an output similar to this:
[,
,
,
,
,
,
,
,
,
]
Ok, this list of 10 records representing movies and actors confirms that the movies have been uploaded on the KG.
Next, let's see the actual graph. Switch to Neo4j Desktop, select the Movie database you created for this exercise, and click open with Neo4j Browser. This will open a new tab where you will able to run Cypher queries. Then, run the following query:
MATCH p=(m:Movie)-[r]-(n)
RETURN p
LIMIT 100;
You should now see something like this:

Quite cool right?
However, this did require some time to explore the dataset, do some cleaning, and manually write the Cypher queries. But come on, it's the Chat GPT era, of course, we don't need to do that anymore unless you want it. Multiple approaches are showing several ways to automate this process. In the next section, we will create a basic one leveraging an LLM.
Creating a Custom LLM to Automate Data Upload
In this section, we create a custom process where an LLM automatically generates node definitions, relationships, and Cypher queries based on the dataset. This approach could be used on other Dataframes as well as automatically recognize the schema. However, consider that it won't match the performances of modern solutions, like LLMGraphTransformer from LangChain, which we will cover in the next section. Instead, use this section to understand a possible "from-scratch" workflow, to get creative, and later design your own Graph-Builder
. Indeed, if there is one main limitation of the current SOTA (State-Of-The-Art) approaches, that's being highly sensitive to the nature of data, and patterns. Therefore, being able to think outside the box is incredibly important in order to either design your Graph-RAG framework from scratch or being able to adapt an existing SOTA Graph-RAG to your needs.
Now, let's get into it, and set up the LLM we will be using for this exercise. You could use any LLM supported by LangChain for this exercise, as long as it's enough powerful to match decent performances.
Two free approaches are Gemini, which is free up to 1500 requests per day using their Gemini Flash
model, and Ollama
, which lets you easily download open-source models on your laptop and set up an API that you can easily call using LangChain. I tested the notebook with both Gemini, and custom Ollama models, and although Gemini guarantees superior performances, I would highly recommend going with Ollama for learning purposes, as playing around with "your own" LLM is just more cool.
In the Ollama example, we will be using qwen2.5-coder 7B
, which is fine-tune on Code-specific tasks, and has impressive performance on code generation, reasoning, and fixing. Based on your memory availability, and laptop performance, you could download the 14B, or 32B version, which would guarantee higher performance.
Let's initialize the model:
# llm = GoogleGenerativeAI(model="gemini-1.5-flash", google_api_key=api_key) # if you are using Google API
llm = OllamaLLM(model="qwen2.5-coder:latest")
If you chose Gemini as your solution, uncomment the first line of code, and comment on the second one. Also, if you chose Gemini, remember to provide the API Key.
Let's start by extracting the structure of the dataset and defining the nodes and their properties:
node_structure = "n".join([
f"{col}: {', '.join(map(str, movies[col].unique()[:3]))}..."
for col in movies.columns
])
print(node_structure)
For each column in the dataset (e.g., Genre
, Director
), we display a few sample values. This gives the LLM an understanding of the data format and typical values for each column.
Release Year: 1907, 1908, 1909...
Title: Daniel boone, Laughing gas, The adventures of dollie...
Origin/Ethnicity: American...
Director: Wallace mccutcheon and ediwin s. porter, Edwin stanton porter, D. w. griffith...
Cast: William craven, florence lawrence, Bertha regustus, edward boulden, Arthur v. johnson, linda arvidson...
Genre: Biographical, Comedy, Drama...
Plot: Boone's daughter befriends an indian maiden as boone and his companion start out on a hunting expedition. while he is away, boone's cabin is attacked by the indians, who set it on fire and abduct boone's daughter. boone returns, swears vengeance, then heads out on the trail to the indian camp. his daughter escapes but is chased. the indians encounter boone, which sets off a huge fight on the edge of a cliff. a burning arrow gets shot into the indian camp. boone gets tied to the stake and tortured. the burning arrow sets the indian camp on fire, causing panic. boone is rescued by his horse, and boone has a knife fight in which he kills the indian chief.[2], The plot is that of a black woman going to the dentist for a toothache and being given laughing gas. on her way walking home, and in other situations, she can't stop laughing, and everyone she meets "catches" the laughter from her, including a vendor and police officers., On a beautiful summer day a father and mother take their daughter dollie on an outing to the river. the mother refuses to buy a gypsy's wares. the gypsy tries to rob the mother, but the father drives him off. the gypsy returns to the camp and devises a plan. they return and kidnap dollie while her parents are distracted. a rescue crew is organized, but the gypsy takes dollie to his camp. they gag dollie and hide her in a barrel before the rescue party gets to the camp. once they leave the gypsies and escapes in their wagon. as the wagon crosses the river, the barrel falls into the water. still sealed in the barrel, dollie is swept downstream in dangerous currents. a boy who is fishing in the river finds the barrel, and dollie is reunited safely with her parents...
Generating NodesNext, we use an LLM prompt template to instruct the model on how to extract nodes and their properties. Let's first take a look at what the whole code looks like:
# Setup logging
logging.basicConfig(level=logging.INFO)
logger = logging.getLogger(__name__)
def validate_node_definition(node_def: Dict) -> bool:
"""Validate node definition structure"""
if not isinstance(node_def, dict):
return False
return all(
isinstance(v, dict) and all(isinstance(k, str) for k in v.keys())
for v in node_def.values()
)
@retry(stop=stop_after_attempt(3), wait=wait_exponential(multiplier=1, min=4, max=10))
def get_node_definitions(chain, structure: str, example: Dict) -> Dict[str, Dict[str, str]]:
"""Get node definitions with retry logic"""
try:
# Get response from LLM
response = chain.invoke({"structure": structure, "example": example})
# Parse response
node_defs = ast.literal_eval(response)
# Validate structure
if not validate_node_definition(node_defs):
raise ValueError("Invalid node definition structure")
return node_defs
except (ValueError, SyntaxError) as e:
logger.error(f"Error parsing node definitions: {e}")
raise
# Updated node definition template
node_example = {
"NodeLabel1": {"property1": "row['property1']", "property2": "row['property2']"},
"NodeLabel2": {"property1": "row['property1']", "property2": "row['property2']"},
"NodeLabel3": {"property1": "row['property1']", "property2": "row['property2']"},
}
define_nodes_prompt = PromptTemplate(
input_variables=["example", "structure"],
template=("""
Analyze the dataset structure below and extract the entity labels for nodes and their properties.n
The node properties should be based on the dataset columns and their values.n
Return the result as a dictionary where the keys are the node labels and the values are the node properties.nn
Example: {example}nn
Dataset Structure:n{structure}nn
Make sure to include all the possible node labels and their properties.n
If a property can be its own node, include it as a separate node label.n
Please do not report triple backticks to identify a code block, just return the list of tuples.n
Return only the dictionary containing node labels and properties, and don't include any other text or quotation.
"""
),
)
# Execute with error handling
try:
node_chain = define_nodes_prompt | llm
node_definitions = get_node_definitions(node_chain, structure=node_structure, example=node_example)
logger.info(f"Node Definitions: {node_definitions}")
except Exception as e:
logger.error(f"Failed to get node definitions: {e}")
raise
Here, we first set up logging using the logging
library, which is a Python module to track events during execution (like errors or status updates):
logging.basicConfig(level=logging.INFO)
logger = logging.getLogger(__name__)
We use basicConfig
to configure logging to display messages of level INFO
or higher, and initiate the logger
instance, which we will use throughout the code to log messages.
This step is not really required, and you could replace it with just print statements. However, it's a good engineering practice.
Next, we create a function to validate the nodes generated by the LLM:
def validate_node_definition(node_def: Dict) -> bool:
"""Validate node definition structure"""
if not isinstance(node_def, dict):
return False
return all(
isinstance(v, dict) and all(isinstance(k, str) for k in v.keys())
for v in node_def.values()
)
The input of the function is a dictionary, where keys are node labels (e.g., Movie
) and values are dictionaries of properties (e.g., title
, year
).
First, the function checks if node_def
is a dictionary, and verifies that each value in the dictionary is also a dictionary and that all keys inside these dictionaries are strings. Then it returns True
if the structure is valid.
Next, we create a function to invoke the LLM chain and actually generate the nodes:
@retry(stop=stop_after_attempt(3), wait=wait_exponential(multiplier=1, min=4, max=10))
def get_node_definitions(chain, structure: str, example: Dict) -> Dict[str, Dict[str, str]]:
"""Get node definitions with retry logic"""
try:
# Get response from LLM
response = chain.invoke({"structure": structure, "example": example})
# Parse response
node_defs = ast.literal_eval(response)
# Validate structure
if not validate_node_definition(node_defs):
raise ValueError("Invalid node definition structure")
return node_defs
If you are not familiar with decorators, you may be wondering what @retry(...)
is doing. Look at this as a wrapper function that surrounds the actual get_node_definitions
function. In this case, we call the retry
decorator, which automatically retries the function if an error occurs.
stop_after_attempt(3)
: Retries up to 3 times.wait_exponential
: Adds an increasing delay between retries (e.g., 4s, 8s, 16s).
The inputs for our functions are:
chain
: The LangChain pipeline (prompt + LLM). We will define the chain later.structure
: The dataset structure (columns and sample values).example
: A sample node definition to guide the LLM.
Next, chain.invoke
sends the structure
and example
to the LLM and receives a response as a string. ast.literal_eval
converts the string response into a Python dictionary.
We check if the parsed dictionary is in the correct format using validate_node_definition
, which raises a ValueError
if the structure is invalid.
except (ValueError, SyntaxError) as e:
logger.error(f"Error parsing node definitions: {e}")
raise
If the response cannot be parsed or validated, the error is logged, and the function raises an exception.
Next, let's provide a prompt template to the LLM to guide it on the node generation task:
define_nodes_prompt = PromptTemplate(
input_variables=["example", "structure"],
template=("""
Analyze the dataset structure below and extract the entity labels for nodes and their properties.n
The node properties should be based on the dataset columns and their values.n
Return the result as a dictionary where the keys are the node labels and the values are the node properties.nn
Example: {example}nn
Dataset Structure:n{structure}nn
Make sure to include all the possible node labels and their properties.n
If a property can be its own node, include it as a separate node label.n
Please do not report triple backticks to identify a code block, just return the list of tuples.n
Return only the dictionary containing node labels and properties, and don't include any other text or quotation.
"""),
)
Note that we are providing the node structure defined at the beginning of this section, and an example of how to generate a dictionary of nodes:
node_example = {
"NodeLabel1": {"property1": "row['property1']", "property2": "row['property2']"},
"NodeLabel2": {"property1": "row['property1']", "property2": "row['property2']"},
"NodeLabel3": {"property1": "row['property1']", "property2": "row['property2']"},
}
In the example, the keys are Node labels (e.g., Movie
, Director
), and the values are dictionaries of properties mapped to dataset columns (e.g., row['property1']
).
Next, let's execute the chain:
try:
node_chain = define_nodes_prompt | llm
node_definitions = get_node_definitions(node_chain, structure=node_structure, example=node_example)
logger.info(f"Node Definitions: {node_definitions}")
except Exception as e:
logger.error(f"Failed to get node definitions: {e}")
raise
In LangChain we create a chain using the structure prompt | llm | ...
, which combines the prompt template with the LLM, forming a pipeline. We use get_node_definitions
to fetch and validate the node definitions.
If the process fails, the error is logged, and the program raises an exception.
If the process succeeds, it will generate something similar to this:
INFO:__main__:Node Definitions: {'Movie': {'Release Year': "row['Release Year']", 'Title': "row['Title']"}, 'Director': {'Name': "row['Director']"}, 'Cast': {'Actor': "row['Cast']"}, 'Genre': {'Type': "row['Genre']"}, 'Plot': {'Description': "row['Plot']"}}
Generate RelationshipsOnce nodes are defined, we identify relationships between them. Again let's first take a look at what the whole code looks like:
class RelationshipIdentifier:
"""Identifies relationships between nodes in a graph database."""
RELATIONSHIP_EXAMPLE = [
("NodeLabel1", "RelationshipLabel", "NodeLabel2"),
("NodeLabel1", "RelationshipLabel", "NodeLabel3"),
("NodeLabel2", "RelationshipLabel", "NodeLabel3"),
]
PROMPT_TEMPLATE = PromptTemplate(
input_variables=["structure", "node_definitions", "example"],
template="""
Consider the following Dataset Structure:n{structure}nn
Consider the following Node Definitions:n{node_definitions}nn
Based on the dataset structure and node definitions, identify relationships (edges) between nodes.n
Return the relationships as a list of triples where each triple contains the start node label, relationship label, and end node label, and each triple is a tuple.n
Please return only the list of tuples. Please do not report triple backticks to identify a code block, just return the list of tuples.nn
Example:n{example}
"""
)
def __init__(self, llm: Any, logger: logging.Logger = None):
self.llm = llm
self.logger = logger or logging.getLogger(__name__)
self.chain = self.PROMPT_TEMPLATE | self.llm
def validate_relationships(self, relationships: List[Tuple]) -> bool:
"""Validate relationship structure."""
return all(
isinstance(rel, tuple) and
len(rel) == 3 and
all(isinstance(x, str) for x in rel)
for rel in relationships
)
@retry(stop=stop_after_attempt(3), wait=wait_exponential(multiplier=1, min=4, max=10))
def identify_relationships(self, structure: str, node_definitions: Dict) -> List[Tuple]:
"""Identify relationships with retry logic."""
try:
response = self.chain.invoke({
"structure": structure,
"node_definitions": str(node_definitions),
"example": str(self.RELATIONSHIP_EXAMPLE)
})
relationships = ast.literal_eval(response)
if not self.validate_relationships(relationships):
raise ValueError("Invalid relationship structure")
self.logger.info(f"Identified {len(relationships)} relationships")
return relationships
except Exception as e:
self.logger.error(f"Error identifying relationships: {e}")
raise
def get_relationship_types(self) -> List[str]:
"""Extract unique relationship types."""
return list(set(rel[1] for rel in self.identify_relationships()))
# Usage
identifier = RelationshipIdentifier(llm=llm)
relationships = identifier.identify_relationships(node_structure, node_definitions)
print("Relationships:", relationships)
Since this code requires a few more operations the node generation, we organize the code in a class – RelationshipIdentifier
– to encapsulate all the logic for relationship extraction, validation, and logging.
We use a similar logic, hence we provide a relationship example:
RELATIONSHIP_EXAMPLE = [
("NodeLabel1", "RelationshipLabel", "NodeLabel2"),
("NodeLabel1", "RelationshipLabel", "NodeLabel3"),
("NodeLabel2", "RelationshipLabel", "NodeLabel3"),
]
Here, each relationship is a tuple with:
- Start Node Label: The label of the source node (e.g.,
Movie
). - Relationship Label: The connection type (e.g.,
DIRECTED_BY
). - End Node Label: The label of the target node (e.g.,
Director
).
Next, we define the actual prompt template:
PROMPT_TEMPLATE = PromptTemplate(
input_variables=["structure", "node_definitions", "example"],
template="""
Consider the following Dataset Structure:n{structure}nn
Consider the following Node Definitions:n{node_definitions}nn
Based on the dataset structure and node definitions, identify relationships (edges) between nodes.n
Return the relationships as a list of triples where each triple contains the start node label, relationship label, and end node label, and each triple is a tuple.n
Please return only the list of tuples. Please do not report triple backticks to identify a code block, just return the list of tuples.nn
Example:n{example}
"""
)
In this case, we have three input variables:
structure
: The dataset structure, listing columns and sample values. We defined it at the beginning of the section.node_definitions
: A dictionary of node labels and their properties. These are the nodes generated by the LLM in the previous section.example
: Example relationships in tuple format.
Next, we initialize the class with three attributes:
def __init__(self, llm: Any, logger: logging.Logger = None):
self.llm = llm
self.logger = logger or logging.getLogger(__name__)
self.chain = self.PROMPT_TEMPLATE | self.llm
llm
: The Language Model to process the prompt (e.g., GPT-3.5 Turbo).logger
: Optional; logs progress and errors (defaults to a standard logger if not provided).self.chain
: Combines the prompt template with the LLM to create a reusable pipeline.
Similarly to before, we create a method to validate the generated relationships:
def validate_relationships(self, relationships: List[Tuple]) -> bool:
"""Validate relationship structure."""
return all(
isinstance(rel, tuple) and
len(rel) == 3 and
all(isinstance(x, str) for x in rel)
for rel in relationships
)
The method checks if each item is a tuple if each tuple contains exactly three elements, and if all elements are strings (e.g., node labels or relationship types). Lastly, it returns TRUE
if the conditions are satisfied, otherwise FALSE
.
Next, we create a method to invoke the chain and generate the relationships:
@retry(stop=stop_after_attempt(3), wait=wait_exponential(multiplier=1, min=4, max=10))
def identify_relationships(self, structure: str, node_definitions: Dict) -> List[Tuple]:
"""Identify relationships with retry logic."""
try:
response = self.chain.invoke({
"structure": structure,
"node_definitions": str(node_definitions),
"example": str(self.RELATIONSHIP_EXAMPLE)
})
relationships = ast.literal_eval(response)
if not self.validate_relationships(relationships):
raise ValueError("Invalid relationship structure")
self.logger.info(f"Identified {len(relationships)} relationships")
return relationships
We use again the retry decorator to reattempt the LLM chain in case of failure and invoke the chain similarly to how we invoked it in the nodes generation.
Additionally, we useast.literal_eval
to convert the LLM's string output into a Python list andvalidate_relationships
to ensure the output format is correct.
except Exception as e:
self.logger.error(f"Error identifying relationships: {e}")
raise
If the method fails, it logs the errors and retries the process up to 3 times.
The last method Returns unique relationship labels (e.g., DIRECTED_BY
, ACTED_IN
):
def get_relationship_types(self) -> List[str]:
"""Extract unique relationship types."""
return list(set(rel[1] for rel in self.identify_relationships()))
It calls theidentify_relationships
method to get the list of relationships. Then, it extracts the second element (relationship label) from each tuple, uses set
to remove duplicates, and converts the result back to a list.
Now, it's finally time to generate the relationships:
identifier = RelationshipIdentifier(llm=llm)
relationships = identifier.identify_relationships(node_structure, node_definitions)
print("Relationships:", relationships)
If the LLM is successful within the 3 attempts it returns a list of relationships in tuple format similar to the following:
INFO:__main__:Identified 4 relationships
Relationships: [('Movie', 'Directed By', 'Director'), ('Movie', 'Starring', 'Cast'), ('Movie', 'Has Genre', 'Genre'), ('Movie', 'Contains Plot', 'Plot')]
Generate Cypher QueriesWith nodes and relationships defined, we create Cypher queries to load them into Neo4j. The process follows a similar logic to both node generation and relationship generation. However, we define a couple more steps for validation, since the output generated will be used to load the data into our KG. Therefore, we need to maximize our chances of success. Let's first take a look at the whole code:
class CypherQueryBuilder:
"""Builds Cypher queries for Neo4j graph database."""
INPUT_EXAMPLE = """
NodeLabel1: value1, value2
NodeLabel2: value1, value2
"""
EXAMPLE_CYPHER = example_cypher = """
CREATE (n1:NodeLabel1 {property1: "row['property1']", property2: "row['property2']"})
CREATE (n2:NodeLabel2 {property1: "row['property1']", property2: "row['property2']"})
CREATE (n1)-[:RelationshipLabel]->(n2);
"""
PROMPT_TEMPLATE = PromptTemplate(
input_variables=["structure", "node_definitions", "relationships", "example"],
template="""
Consider the following Node Definitions:n{node_definitions}nn
Consider the following Relationships:n{relationships}nn
Generate Cypher queries to create nodes and relationships using the node definitions and relationships below. Remember to replace the placeholder values with actual data from the dataset.n
Include all the properties in the Node Definitions for each node as defined and create relationships.n
Return a single string with each query separated by a semicolon.n
Don't include any other text or quotation marks in the response.n
Please return only the string containing Cypher queries. Please do not report triple backticks to identify a code block.nn
Example Input:n{input}nn
Example Output Cypher query:n{cypher}
"""
)
def __init__(self, llm: Any, logger: logging.Logger = None):
self.llm = llm
self.logger = logger or logging.getLogger(__name__)
# self.chain = LLMChain(llm=llm, prompt=self.PROMPT_TEMPLATE)
self.chain = self.PROMPT_TEMPLATE | self.llm
def validate_cypher_query(self, query: str) -> bool:
"""Validate Cypher query syntax using LLM and regex patterns."""
VALIDATION_PROMPT = PromptTemplate(
input_variables=["query"],
template="""
Validate this Cypher query and return TRUE or FALSE:
Query: {query}
Rules to check:
1. Valid CREATE statements
2. Proper property formatting
3. Valid relationship syntax
4. No missing parentheses
5. Valid property names
6. Valid relationship types
Return only TRUE if query is valid, FALSE if invalid.
"""
)
try:
# Basic pattern validation
basic_valid = all(re.search(pattern, query) for pattern in [
r'CREATE (',
r'{.*?}',
r')-[:.*?]->'
])
if not basic_valid:
return False
# LLM validation
validation_chain = VALIDATION_PROMPT | self.llm
result = validation_chain.invoke({"query": query})
# Parse result
is_valid = "TRUE" in result.upper()
if not is_valid:
self.logger.warning(f"LLM validation failed for query: {query}")
return is_valid
except Exception as e:
self.logger.error(f"Validation error: {e}")
return False
def sanitize_query(self, query: str) -> str:
"""Sanitize and format Cypher query."""
return (query
.strip()
.replace('n', ' ')
.replace(' ', ' ')
.replace("'row[", "row['")
.replace("]'", "']"))
@retry(stop=stop_after_attempt(3), wait=wait_exponential(multiplier=1, min=4, max=10))
def build_queries(self, node_definitions: Dict, relationships: List) -> str:
"""Build Cypher queries with retry logic."""
try:
response = self.chain.invoke({
"node_definitions": str(node_definitions),
"relationships": str(relationships),
"input": self.INPUT_EXAMPLE,
"cypher": self.EXAMPLE_CYPHER
})
# Get response inside triple backticks
if '```' in response:
response = response.split('```')[1]
# Sanitize response
queries = self.sanitize_query(response)
# Validate queries
if not self.validate_cypher_query(queries):
raise ValueError("Invalid Cypher query syntax")
self.logger.info("Successfully generated Cypher queries")
return queries
except Exception as e:
self.logger.error(f"Error building Cypher queries: {e}")
raise
def split_queries(self, queries: str) -> List[str]:
"""Split combined queries into individual statements."""
return [q.strip() for q in queries.split(';') if q.strip()]
# Usage
builder = CypherQueryBuilder(llm=llm)
cypher_queries = builder.build_queries(node_definitions, relationships)
print("Cypher Queries:", cypher_queries)
We provide a prompt template to help the LLM:
PROMPT_TEMPLATE = PromptTemplate(
input_variables=["structure", "node_definitions", "relationships", "example"],
template="""
Consider the following Node Definitions:n{node_definitions}nn
Consider the following Relationships:n{relationships}nn
Generate Cypher queries to create nodes and relationships using the node definitions and relationships below. Remember to replace the placeholder values with actual data from the dataset.n
Include all the properties in the Node Definitions for each node as defined and create relationships.n
Return a single string with each query separated by a semicolon.n
Don't include any other text or quotation marks in the response.n
Please return only the string containing Cypher queries. Please do not report triple backticks to identify a code block.nn
Example Input:n{input}nn
Example Output Cypher query:n{cypher}
"""
)
Now we provide four inputs to the prompt:
structure
: Dataset structure for context.node_definitions
: Generated Nodes and their properties.relationships
: Generated Edges between nodes.example
: Example queries for formatting reference.
def __init__(self, llm: Any, logger: logging.Logger = None):
self.llm = llm
self.logger = logger or logging.getLogger(__name__)
self.chain = self.PROMPT_TEMPLATE | self.llm
We initialize the class in the same fashion as the relationship class.
Next, we define a validation method to check the generated output:
def validate_cypher_query(self, query: str) -> bool:
"""Validate Cypher query syntax using LLM and regex patterns."""
VALIDATION_PROMPT = PromptTemplate(
input_variables=["query"],
template="""
Validate this Cypher query and return TRUE or FALSE:
Query: {query}
Rules to check:
1. Valid CREATE statements
2. Proper property formatting
3. Valid relationship syntax
4. No missing parentheses
5. Valid property names
6. Valid relationship types
Return only TRUE if query is valid, FALSE if invalid.
"""56
)
try:
# Basic pattern validation
basic_valid = all(re.search(pattern, query) for pattern in [
r'CREATE (',
r'{.*?}',
r')-[:.*?]->'
])
if not basic_valid:
return False
# LLM validation
validation_chain = VALIDATION_PROMPT | self.llm
result = validation_chain.invoke({"query": query})
# Parse result
is_valid = "TRUE" in result.upper()
if not is_valid:
self.logger.warning(f"LLM validation failed for query: {query}")
return is_valid
except Exception as e:
self.logger.error(f"Validation error: {e}")
return False
This method does two validation steps. First a basic validation with regular expressions:
basic_valid = all(re.search(pattern, query) for pattern in [
r'CREATE (',
r'{.*?}',
r')-[:.*?]->'
])
if not basic_valid:
return False
This ensures the query contains essential Cypher syntax:
CREATE
: Ensures nodes and relationships are being created.- *`{.?}`**: Ensures properties are included.
- *`-[:.?]->`**: Ensures relationships are correctly formatted.
Then, it performs an advanced validation with LLM:
validation_chain = VALIDATION_PROMPT | self.llm
result = validation_chain.invoke({"query": query})
is_valid = "TRUE" in result.upper()
The validation is specified in the prompt where we ask the LLM to make sure that we have:
- Valid CREATE statements
- Proper property formatting
- Valid relationship syntax
- No missing parentheses
- Valid property names
- Valid relationship types
Even though we should be in a good state as of now, let's add a method, to further sanitize the generated output:
def sanitize_query(self, query: str) -> str:
"""Sanitize and format Cypher query."""
return (query
.strip()
.replace('n', ' ')
.replace(' ', ' ')
.replace("'row[", "row['")
.replace("]'", "']"))
In particular, we are removing unnecessary spaces, as well as line breaks (n
), and fix potential formatting issues with dataset references (e.g., row['property1']
).
Please consider updating this method based on the model you are using. Smaller models will likely require more sanitization.
Next, we define a query invocation method:
@retry(stop=stop_after_attempt(3), wait=wait_exponential(multiplier=1, min=4, max=10))
def build_queries(self, node_definitions: Dict, relationships: List) -> str:
"""Build Cypher queries with retry logic."""
try:
response = self.chain.invoke({
"node_definitions": str(node_definitions),
"relationships": str(relationships),
"input": self.INPUT_EXAMPLE,
"cypher": self.EXAMPLE_CYPHER
})
# Get response inside triple backticks
if '```' in response:
response = response.split('```')[1]
# Sanitize response
queries = self.sanitize_query(response)
# Validate queries
if not self.validate_cypher_query(queries):
raise ValueError("Invalid Cypher query syntax")
self.logger.info("Successfully generated Cypher queries")
return queries
except Exception as e:
self.logger.error(f"Error building Cypher queries: {e}")
raise
This method works similarly to the one in the relationship builder class, with the only addition of:
if '```' in response:
response = response.split('```')[1]
Here, the LLM may provide an additional markdown format to specify it's a code block. If this is present in the LLM's response, we only retrieve the code within the triple backticks.
Next, we define a method to break a single string of Cypher queries into individual statements:
def split_queries(self, queries: str) -> List[str]:
"""Split combined queries into individual statements."""
return [q.strip() for q in queries.split(';') if q.strip()]
For example, this Cypher query:
CREATE (n1:Movie {title: "Inception"}); CREATE (n2:Director {name: "Nolan"});
This will turn into this:
["CREATE (n1:Movie {title: 'Inception'})", "CREATE (n2:Director {name: 'Nolan'})"]
This will be useful so we can iterate over a list of queries.
Lastly, we initialize the class and generate the Cypher queries:
builder = CypherQueryBuilder(llm=llm)
cypher_queries = builder.build_queries(node_definitions, relationships)
print("Cypher Queries:", cypher_queries)
In case of success, the output will look like this:
INFO:__main__:Successfully generated Cypher queries
Cypher Queries: CREATE (m:Movie {Release_Year: "row['Release Year']", Title: "row['Title']"}) CREATE (d:Director {Name: "row['Director']"}) CREATE (c:Cast {Actor: "row['Cast']"}) CREATE (g:Genre {Type: "row['Genre']"}) CREATE (p:Plot {Description: "row['Plot']"}) CREATE (m)-[:Directed_By]->(d) CREATE (m)-[:Starring]->(c) CREATE (m)-[:Has_Genre]->(g) CREATE (m)-[:Contains_Plot]->(p)
Finally, we iterate over the dataset and execute the generated Cypher queries for each row.
logs = ""
total_rows = len(df)
def sanitize_value(value):
if isinstance(value, str):
return value.replace('"', '')
return str(value)
for index, row in tqdm(df.iterrows(),
total=total_rows,
desc="Loading data to Neo4j",
position=0,
leave=True):
# Replace placeholders with actual values
cypher_query = cypher_queries
for column in df.columns:
cypher_query = cypher_query.replace(
f"row['{column}']",
f'{sanitize_value(row[column])}'
)
try:
# Execute query and update progress
conn.execute_query(cypher_query)
except Exception as e:
logs += f"Error on row {index+1}: {str(e)}n"
Note that we define an empty string variable logs
which we will use to capture potential failures. Also, we add a sanitize function to pass to each value in the row input:
def sanitize_value(value):
if isinstance(value, str):
return value.replace('"', '')
return str(value)
This will prevent strings containing double quotes from breaking the query syntax.
Next, we loop over the dataset:
for index, row in tqdm(df.iterrows(),
total=total_rows,
desc="Loading data to Neo4j",
position=0,
leave=True):
# Replace placeholders with actual values
cypher_query = cypher_queries
for column in df.columns:
cypher_query = cypher_query.replace(
f"row['{column}']",
f'{sanitize_value(row[column])}'
)
try:
# Execute query and update progress
conn.execute_query(cypher_query)
except Exception as e:
logs += f"Error on row {index+1}: {str(e)}n"
As I mentioned at the beginning of the exercise, we use tqdm
to add a nice-looking progress bar to visualize how many rows have been processed. We pass df.iterrows()
to iterate through the DataFrame, providing the index and the row data.total=total_rows
is used by tqdm
to calculate progress. We adddesc="Loading data to Neo4j"
to provide a label for the progress bar. Lastly, position=0, leave=True
ensures the progress bar stays visible in the console.
Next, we replace placeholders like row['column_name']
with the actual dataset values passing each value in the sanitize_value
function, and execute the query.
Let's check if our dataset is now uploaded. Switch to the Neo4j browser, and run the following Cypher query:
MATCH p=(m:Movie)-[r]-(n)
RETURN p
LIMIT 100;
In my case, the LLM generated the following graph:

This is pretty similar to the knowledge graph we uploaded manually. Not bad for a Naïve LLM right? Even though that required quite some code, we can now reuse it for multiple datasets, and more importantly, we can use it as a base to create more complex LLM graph builders.
In our example, we haven't helped our LLM by providing entities, relationships, and properties for both. However, consider adding them as examples to increase the performance of the LLM. Moreover, modern approaches leverage the chain of thought to come up with additional nodes and relationships, this makes the model sequentially reason over the output to further improve it. Another strategy can be providing samples of rows to better adapt to the values provided in each row.
In the next example, we will see a modern implementation of Graph-RAG with LangChain.
LLMGraphTransformer with LangChain
To make our graph smarter, we use LangChain to extract entities (movies, actors, etc.) and relationships from text descriptions. LLMGraphTransformer
is designed to transform text-based documents into graph documents using a Language Model.
Let's start by initializing it:
llm_transformer = LLMGraphTransformer(
llm=llm,
)
The LLM provided is the same one we used for our custom Graph Builder. In this case, we are using the default parameters to give freedom to the model to experiment with nodes, edges, and properties. However, there are a few parameters that are worth knowing to potentially further boost the performances of this algorithm:
allowed_nodes
andallowed_relationships
: We haven't specified, so by default, all node and relationship types are allowed.strict_mode=True
: Ensures that only allowed nodes and relationships are included in the output if constraints are specified.node_properties=False
: Disables property extraction for nodes.relationship_properties=False
: Disables property extraction for relationships.prompt
: Pass aChatPromptTemplate
to customize the context of the LLM. This is similar to what we have done in our custom LLM.
One caveat of this algorithm is that it's quite slow, especially considering we are not providing a list of nodes and relationships. Therefore, we will only use 100 rows out of the 1000 available in the dataset to speed things up:
df_sample = df.head(100) # Reduce sample size for faster processing
Next, let's prepare our dataset. We said "LLMGraphTransformer
is designed to transform text-based documents into graph documents", this means that we need to turn our pandas dataframe into text:
df_sample = movies.head(100)
documents = []
for _, row in tqdm(df_sample.iterrows(),
total=len(df_sample),
desc="Creating documents",
position=0,
leave=True):
try:
# Format text with proper line breaks
text = ""
for col in df.columns:
text += f"{col}: {row[col]}n"
documents.append(Document(page_content=text))
except KeyError as e:
tqdm.write(f"Missing column: {e}")
except Exception as e:
tqdm.write(f"Error processing row: {e}")
This will convert each row into text and add it to a LangChain Document
object, which is compatible with LangChain's LLMGraphTransformer
.
Next, we run the LLM and start the generation:
graph_documents = await llm_transformer.aconvert_to_graph_documents(documents)
Note that in this case, we are using await
, and aconvert_to_graph_documents
instead of convert_to_graph_documents
to process documents asynchronously, enabling faster execution in large-scale applications.
Next, sit tight because this will take a few minutes (~30 minutes). Once, the conversion is finished let's print the generated nodes and relationships:
print(f"Nodes:{graph_documents[0].nodes}")
print(f"Relationships:{graph_documents[0].relationships}")
In my case, I got the following:
Nodes:[Node(id="Boone's cabin", type='Cabin', properties={}), Node(id='Boone', type='Boone', properties={}), Node(id='Indian maiden', type='Person', properties={}), Node(id='Indian chief', type='Chief', properties={}), Node(id='Florence Lawrence', type='Person', properties={}), Node(id='William Craven', type='Person', properties={}), Node(id='Wallace mccutcheon and ediwin s. porter', type='Director', properties={}), Node(id='Burning arrow', type='Arrow', properties={}), Node(id='Boone', type='Person', properties={}), Node(id='Indian camp', type='Camp', properties={}), Node(id='American', type='Ethnicity', properties={}), Node(id="Boone's horse", type='Horse', properties={}), Node(id='None', type='None', properties={}), Node(id='an indian maiden', type='Maiden', properties={}), Node(id='William craven', type='Cast', properties={}), Node(id='florence lawrence', type='Cast', properties={}), Node(id='Swears vengeance', type='Vengeance', properties={}), Node(id='Daniel Boone', type='Person', properties={}), Node(id='Indians', type='Group', properties={}), Node(id='Daniel boone', type='Title', properties={}), Node(id="Daniel Boone's daughter", type='Person', properties={})]
Relationships:[Relationship(source=Node(id='Daniel Boone', type='Person', properties={}), target=Node(id='Daniel boone', type='Title', properties={}), type='TITLE', properties={}), Relationship(source=Node(id='Daniel Boone', type='Person', properties={}), target=Node(id='American', type='Ethnicity', properties={}), type='ORIGIN_ETHNICITY', properties={}), Relationship(source=Node(id='Daniel Boone', type='Person', properties={}), target=Node(id='Wallace mccutcheon and ediwin s. porter', type='Director', properties={}), type='DIRECTED_BY', properties={}), Relationship(source=Node(id='William Craven', type='Person', properties={}), target=Node(id='William craven', type='Cast', properties={}), type='CAST', properties={}), Relationship(source=Node(id='Florence Lawrence', type='Person', properties={}), target=Node(id='florence lawrence', type='Cast', properties={}), type='CAST', properties={}), Relationship(source=Node(id="Daniel Boone's daughter", type='Person', properties={}), target=Node(id='an indian maiden', type='Maiden', properties={}), type='BEFRIENDS', properties={}), Relationship(source=Node(id='Boone', type='Person', properties={}), target=Node(id='Indian camp', type='Camp', properties={}), type='LEADS_OUT_ON_A_HUNTING_EXPEDITION', properties={}), Relationship(source=Node(id='Indians', type='Group', properties={}), target=Node(id="Boone's cabin", type='Cabin', properties={}), type='ATTACKS', properties={}), Relationship(source=Node(id='Indian maiden', type='Person', properties={}), target=Node(id='None', type='None', properties={}), type='ESCAPES', properties={}), Relationship(source=Node(id='Boone', type='Person', properties={}), target=Node(id='Swears vengeance', type='Vengeance', properties={}), type='RETURNS', properties={}), Relationship(source=Node(id='Boone', type='Person', properties={}), target=Node(id='None', type='None', properties={}), type='HEADS_OUT_ON_THE_TRAIL_TO_THE_INDIAN_CAMP', properties={}), Relationship(source=Node(id='Indians', type='Group', properties={}), target=Node(id='Boone', type='Boone', properties={}), type='ENCOUNTERS', properties={}), Relationship(source=Node(id='Indian camp', type='Camp', properties={}), target=Node(id='Burning arrow', type='Arrow', properties={}), type='SET_ON_FIRE', properties={}), Relationship(source=Node(id='Boone', type='Person', properties={}), target=Node(id='None', type='None', properties={}), type='GETS_TIED_TO_THE_STAKE_AND_TOURED', properties={}), Relationship(source=Node(id='Indian camp', type='Camp', properties={}), target=Node(id='Burning arrow', type='Arrow', properties={}), type='SETS_ON_FIRE', properties={}), Relationship(source=Node(id='Indians', type='Group', properties={}), target=Node(id="Boone's horse", type='Horse', properties={}), type='ENCOUNTERS', properties={}), Relationship(source=Node(id='Boone', type='Person', properties={}), target=Node(id='Indian chief', type='Chief', properties={}), type='HAS_KNIFE_FIGHT_IN_WHICH_HE_KILLS_THE_INDIAN_CHIEF', properties={})]
Next, it's time to add the generated graph documents to our knowledge graph. We can do that by leveraging the LangChain integration of Neo4j:
graph = Neo4jGraph(url=uri, username=user, password=password, enhanced_schema=True)
graph.add_graph_documents(graph_documents)
Let's store the graph connection in the graph
variable passing the same URL, username, and password you used at the beginning of this application. Then, let's call the add_graph_documents
method to add all the graph documents to our database.
Once, the process is complete, let's switch to Neo4j Browser one last time, and check our new knowledge graph.
As always, run the following query to see the knowledge graph:
MATCH p=(m:Movie)-[r]-(n)
RETURN p
LIMIT 100;
In my case, the KG looks like this:
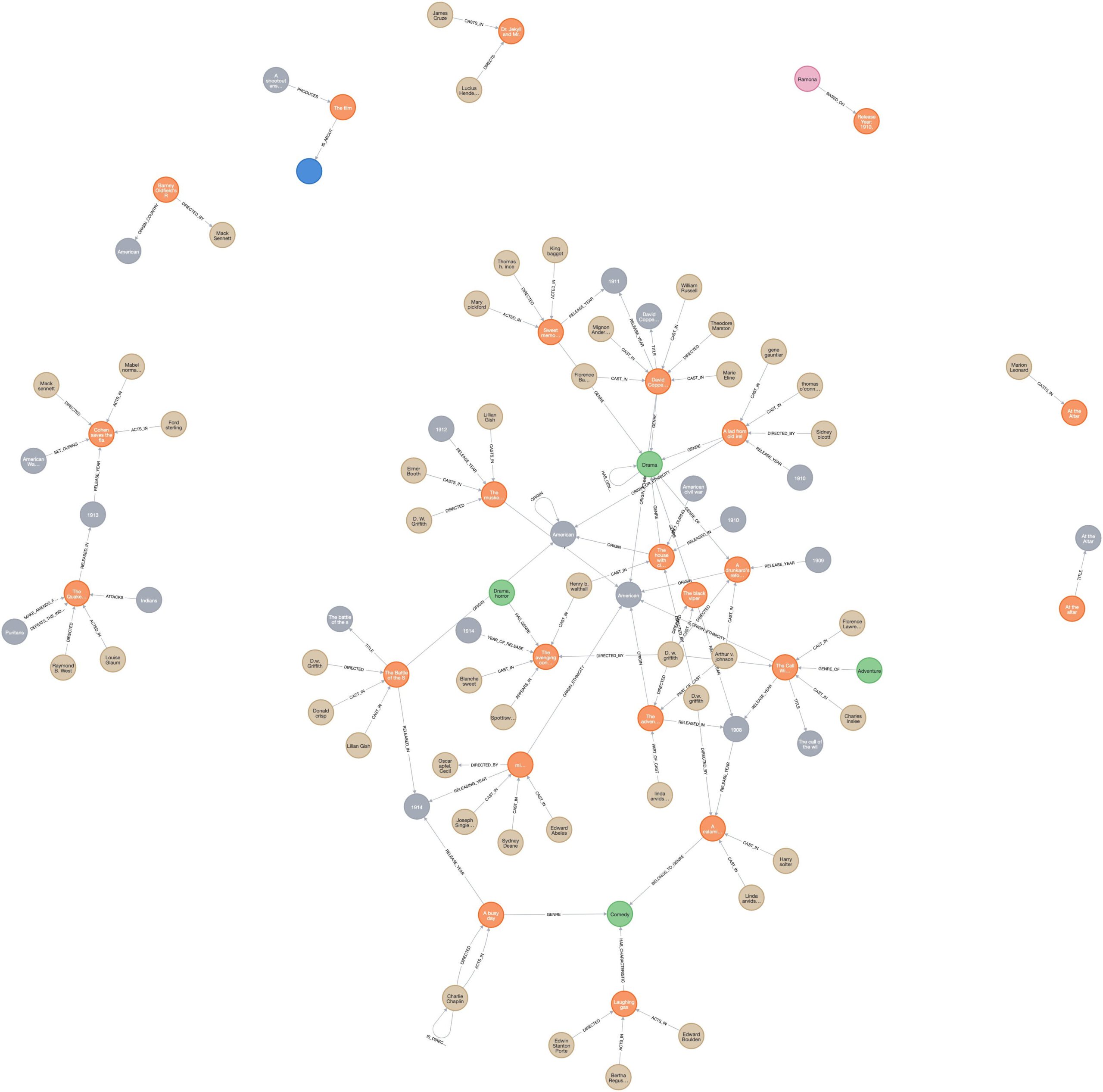
Well, that's a knowledge graph.
But we are not done yet. You may remember that our mission is to actually interrogate the knowledge graph to help us find movies. In this article, I will provide a simple Text to Cypher approach which will leverage an LLM to generate a Cypher query, run the Cypher query, and use the retrieved information as context to answer the user query. However, consider that this is just a simple approach, and we will explore advanced retrieval methods in a future article.
First of all, let's refresh the schema of the graph since we will use it to give an understanding of our KG to the LLM:
graph.refresh_schema()
Now, it's time to set up the QA chain:
# llm = ChatGoogleGenerativeAI(
# model="gemini-1.5-pro",
# temperature=0,
# max_tokens=None,
# timeout=None,
# max_retries=2,
# api_key=api_key
# )
CYPHER_GENERATION_TEMPLATE = """Task:Generate Cypher statement to query a graph database.
Instructions:
Use only the provided relationship types and properties in the schema.
Do not use any other relationship types or properties that are not provided.
Schema:
{schema}
Note: Do not include any explanations or apologies in your responses.
Do not respond to any questions that might ask anything else than for you to construct a Cypher statement.
Do not include any text except the generated Cypher statement.
Return every node as whole, do not return only the properties.
The question is:
{question}"""
CYPHER_GENERATION_PROMPT = PromptTemplate(
input_variables=["schema", "question"], template=CYPHER_GENERATION_TEMPLATE
)
chain = GraphCypherQAChain.from_llm(
llm,
graph=graph,
verbose=True,
allow_dangerous_requests=True,
return_intermediate_steps=True,
cypher_prompt=CYPHER_GENERATION_PROMPT
)
If you are using Gemini, make sure to switch to a chat model by uncommenting the llm
variable at the top.
In the code above we provide a prompt to the LLM to help it with the generation, and the schema of the graph passing the graph variable.
Finally, let's ask it something:
chain.run("Give me an overview of the movie titled David Copperfield.")
In my case, the output is:
Generated Cypher:
MATCH p=(n:Title {id: 'David Copperfield'})-[*1..2]-()
RETURN p
Full Context:
[{'p': [{'id': 'David Copperfield'}, 'TITLE', {'id': 'David Copperfield'}]}, {'p': [{'id': 'David Copperfield'}, 'TITLE', {'id': 'David Copperfield'}, 'CAST_IN', {'id': 'Florence La Badie'}]}, {'p': [{'id': 'David Copperfield'}, 'TITLE', {'id': 'David Copperfield'}, 'RELEASE_YEAR', {'id': '1911'}]}, {'p': [{'id': 'David Copperfield'}, 'TITLE', {'id': 'David Copperfield'}, 'GENRE', {'id': 'Drama'}]}, {'p': [{'id': 'David Copperfield'}, 'TITLE', {'id': 'David Copperfield'}, 'DIRECTED', {'id': 'Theodore Marston'}]}, {'p': [{'id': 'David Copperfield'}, 'TITLE', {'id': 'David Copperfield'}, 'ORIGIN_ETHNICITY', {'id': 'American'}]}, {'p': [{'id': 'David Copperfield'}, 'TITLE', {'id': 'David Copperfield'}, 'CAST_IN', {'id': 'Mignon Anderson'}]}, {'p': [{'id': 'David Copperfield'}, 'TITLE', {'id': 'David Copperfield'}, 'CAST_IN', {'id': 'William Russell'}]}, {'p': [{'id': 'David Copperfield'}, 'TITLE', {'id': 'David Copperfield'}, 'CAST_IN', {'id': 'Marie Eline'}]}]
INFO:httpx:HTTP Request: POST http://127.0.0.1:11434/api/generate "HTTP/1.1 200 OK"
> Finished chain.
'David Copperfield is a 1911 American drama film directed by Theodore Marston. The movie stars David Copperfield, Florence La Badie, Mignon Anderson, William Russell, and Marie Eline in various roles. It provides an overview of the life and adventures of David Copperfield through the eyes of his various companions and experiences.'
By setting verbose=True
we output the intermediate step: generated Cypher query, and context provided.
Conclusion
This article served as an introduction to a modern approach to building a knowledge graph. First, we explored a traditional approach and had an overview of the Cypher language, then created a Naïve LLM graph builder to automate the Graph-building process matching the performances achieved during the manual process. Lastly, we went a step further and introduced LLMGraphTransformer
by LangChain which significantly improved our KG. However, this is just the beginning of our Graph RAG journey specifically our graph builder journey. There are many more modern approaches we need to explore and build from scratch, and we will do that in future articles.
Moreover, the strategies we talked about above are still not considering the second component of Graph-RAG: the graph retriever. Although improving the actual graph will also improve retrieval performances, we are still not thinking of a retrieval point of view. For example, following the direction we have taken for now, the more labels, nodes, edges, and properties, the better. However, that's actually making it harder for the retriever function to identify the right information to retrieve. Indeed, modern approaches also consider following a tree structure in the knowledge graph, by creating macro-areas and further breaking them down into micro-areas to help the retrieval.
As of now, I would like you to test all this code on a different dataset, and get creative with the customization of our Naïve LLM graph-builder. Although LLMGraphTransformer
seems a very convenient choice to speed up a flexible graph-builder with a minimum amount of code, this method required much more time to build our KG. Moreover, building it from scratch will make sure you are grasping and fully understanding every component behind Graph-RAG.
Get ready for more Graph-RAG in future articles!