Complex List Comprehensions Can Be Readable!
PYTHON PROGRAMMING
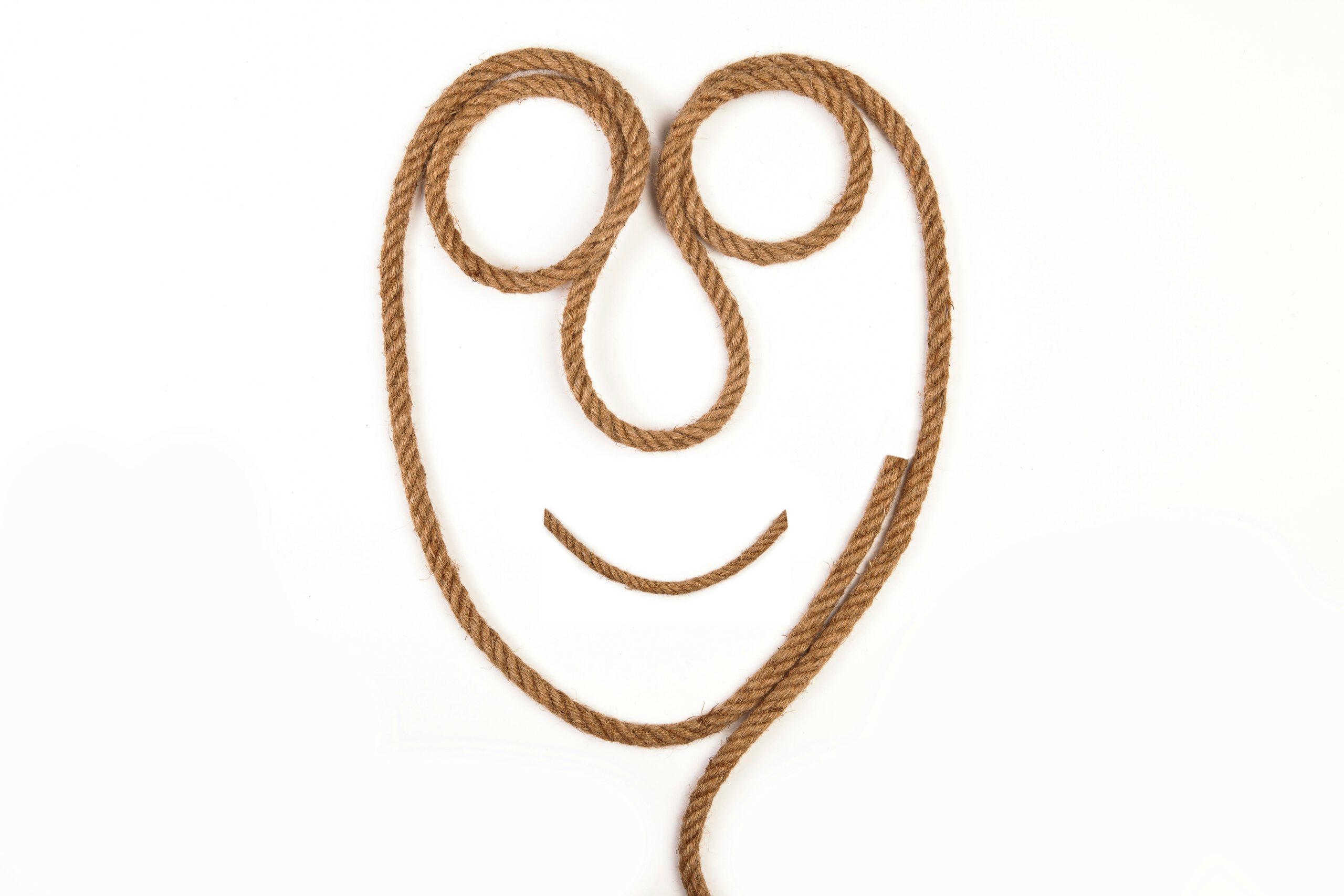
Python comprehensions – including list, dictionary and set comprehensions as well as generator expressions – constitute a powerful Python syntactic sugar. You can read about them in the following articles:
Python comprehensions have two great advantages when compared to the corresponding for
loops: they are faster and they can be much more readable.
Note the phrase "can be much more readable." Indeed, they aren't always more readable. This begs the following question: When are they?
It depends on you. It is you – the developer – who makes a Python comprehension readable. Sloppy implementation can destroy a comprehension's readability, though the same can be said about for
loops.
Comprehensions in Python were designed to be read in a very similar way that you read an English sentence: You can read a comprehension from left to right (or from top to bottom, if it takes several lines) just like you read an English sentence from left to right.
Many say you shouldn't use complex comprehensions because they are difficult to read and comprehend. In this article, we'll discuss this well-known principle – if not a myth. Unfortunately, many people strain this principle by excessively avoiding the use of Python comprehensions in situations where they could be used with success.
You can read a comprehension from left to right (or from top to bottom, if it takes several lines) just like you read an English sentence from left to right.
Reading comprehensions
I like to think of Python comprehensions as algorithms: a data operation is performed in one or more loops, followed by an optional if
condition or several of them. Such viewing of a comprehension largely helps understanding it, even if it's quite long and complicated.
Remember, a Python comprehension always uses the following pattern: data operation → loop(s) → optional condition(s). We'll return to this it every time we'll analyze a comprehension.
There is an exception to this rule. When you use the walrus operator, you have to break this algorithmic pattern a little bit; we'll discuss this later on. Nevertheless, once you get some practice, this change won't pose much of a challenge for you, and the algorithm won't lose much readability.
I like to think of Python comprehensions as algorithms: a data operation is performed in one or more loops, followed by an optional
if
condition or several of them.
Basic use case
Let's consider a very simple example: given a list of numbers x
, we want to create a list of squared elements of x
. We can use a regular for
loop to do this:
>>> x = [1, 2, 5, 100]
>>> x_squared = []
>>> for xi in x:
... x_squared.append(xi**2)
>>> x_squared
[1, 4, 25, 10000]
Let's read the code, starting from the second line:
- We start by creating an empty output list,
x_squared
. The name itself says what the list will contain. - A
for
loop is run, each iteration being run forxi
, the values ofxi
constituting the subsequent elements of thex
list. - In each iteration, we append
xi**2
to the output list,x_squared
.
Let's consider the corresponding List Comprehension:
>>> x = [1, 2, 5, 100]
>>> x_squared = [xi**2 for xi in x]
>>> x_squared
[1, 4, 25, 10000]
As you see, in this simple example, the comprehension needs just one line. We can read it as follows:
- Calculate
xi**2
for eachxi
,xi
being subsequent values of thex
list, and collect the results in the output list.
That's it! It's clear, it's obvious, it's easy to read. Have you noticed the pattern we used? It's as simple as can it be: data operation → Loop.
I know that for beginners it isn't all that clear, obvious and easy to read. But to learn a programming language and to use its strengths, you need practice. Only then can you really benefit from the language's strengths intricacies, including syntactic sugar like Python comprehensions.
Face it: if you want to use Python, you must be able to use and understand comprehensions.
Thus, even if you don't consider Python comprehensions all that natural and easy to read, don't stop trying. Sooner or later, you'll see in them what advanced Pythonistas see: simplicity joined with brevity and readability. Just keep trying.
This was a very simple use case – but the truth is, such comprehensions are very common in the practice of Python. We can make it a little more complex by adding an if
check, another frequent practical scenario.
Thus, even if you don't consider Python comprehensions all that natural and easy to read, don't stop trying. Sooner or later, you'll see in them what advanced Pythonistas see: simplicity joined with brevity and readability. Just keep trying.
We can, for instance, take only odd numbers, so those for which xi % 2 != 0
. Let's refactor the for
loop to achieve this:
>>> x = [1, 2, 5, 100]
>>> x_squared = []
>>> for xi in x:
... if xi % 2 != 0:
... x_squared.append(xi**2)
>>> x_squared
[1, 25]
So:
- Like before, start by creating an empty output list,
x_squared
. - A
for
loop is run, each iteration being run forxi
, the values ofxi
constituting the subsequent elements of thex
list. - In each iteration, we check if
xi
is an odd number. If it is, we appendxi**2
to the output list,x_squared
. Otherwise,xi
is ignored.
Let's use the corresponding list comprehension:
>>> x = [1, 2, 5, 100]
>>> x_squared = [xi**2 for xi in x if xi % 2 != 0]
>>> x_squared
[1, 25]
Let's read it:
- Calculate
xi**2
forxi
,xi
being subsequent values of thex
list. - Collect the results for odd values of
xi
in the output list.
Face it: if you want to use Python, you must be able to use and understand comprehensions.
In both above scenarios, I consider the list comprehension version simpler to read. The for
loop requires reading the whole code, and the different operations are spread across it. The list comprehension is a neat one-liner that collects all the operations in the typical pattern: data operation → loop → condition.
These were simple scenarios. However, it's common knowledge that the readability of Python comprehensions can drop if you make them overly complicated, such as by nesting them in several layers (that is, by creating a loop in a loop). We'll consider such examples in the next section.
Advanced use cases
This time, let's use with a dictionary comprehension, as usually it's a little more complicated to write and read than the corresponding list comprehension. In addition, we'll use one loop and two if
checks.
We'll work with the following data:
>>> products = [
... "Widget", "Gadget", "Thingamajig",
... "Doodad", "Whatsit",
... ]
>>> prices = [19.99, 25.50, 9.99, 20.00, 22.50]
>>> discounts = [0.10, 0.25, 0.05, 0.20, 0.15]
We want to create a dictionary with products and their prices, but only for the products with the discount of at least 15% and the price between $20 and $30.
Let's start with a regular for
loop:
>>> discounted_products = {}
>>> prod_price_disc = zip(products, prices, discounts)
>>> for product, price, discount in prod_price_disc:
... if discount >= 0.15 and 20 <= price <= 30:
... discounted_products[product] = price
>>> discounted_products
{'Gadget': 25.5, 'Doodad': 20.0, 'Whatsit': 22.5}
This is how we can read the code:
- First, we need to initialize an output dictionary,
discounted_products
. It will collect the products that meet the criteria. - Then, we create a
for
loop to iterate over the product names, prices, and discounts simultaneously. For this, we need to create azip
object, using thezip()
function. - Inside the loop, we check two conditions: if the discount for each product is at least 15% (
discount >= 0.15
) and if the price is between $20 and $30 (20 <= price <= 30
). - If both conditions are met, the product and its price are added to the
discounted_products
dictionary, the product being a key while the price being the value.
The way I see it, it's a pretty simple exercise, but the code based on the for
loop isn't proportionally simple. Thus, let's check out the corresponding dictionary comprehension:
>>> discounted_products = {
... product: price
... for product, price, discount
... in zip(products, prices, discounts)
... if discount >= 0.15 and 20 <= price <= 30
... }
>>> discounted_products
{'Gadget': 25.5, 'Doodad': 20.0, 'Whatsit': 22.5}
As you can see, both approaches lead to the same output. Let's read the code:
- The entire process is condensed into a single dictionary comprehension. It doesn't fit in one line, however, unless you're ready to accept a very long line. In my opinion, such a long one-liner could be even less readable than the
for
loop shown above. - This is how we can read the comprehension: Take a product (as a key) and its price (as its value), iterating over the products, prices, and discounts simultaneously, using the corresponding
zip
object using thezip()
function – but only if two conditions are met: the discount for each product is at least 15% (discount >= 0.15
) and the price is between $20 and $30 (20 <= price <= 30
). - Such key-value pairs are kept in the output dictionary,
discounted_products
.
To me, the comprehension code is much more straightforward, as it integrates dictionary construction, data operation, looping and condition checking into a single, readable command. It's not a one-liner anymore – but still, the resulting code is very readable, with the whole process being implemented using the algorithmic pattern we've used before: data operation → loop → conditions. Note that the two conditions are condensed into one if
condition, although we could easily use two if
checks (this applies to both versions of the code).
In other words, in a Python comprehension if a if b
means the same as if a and b
. Which to choose should depend on readability, as benchmarking the two solutions didn't provide conclusive results.
Let's consider an even more advanced scenario, with two nested for
loops. This is the data we're going to use:
>>> products = ['Apples', 'Bananas', 'Cherries', 'Dates']
>>> prices = [25, 15, 22, 35]
>>> discounts = [0.20, 0.10, 0.15, 0.25]
>>> locations = ['East', 'West', 'North', 'South']
>>> available_in = [
... ['East', 'North'],
... ['West'],
... ['South', 'East'],
... ['North']
... ]
Although we have four locations of the stores, the availability of the products is limited to selected locations; they are provided as lists in the available_in
list. So, for example, apples are available in the East and North stores while bananas only in the West store. We need to take this into account while taking products and their prices that follow the conditions.
This is the for
loop:
>>> discounted_products = {}
>>> zipped = zip(products, prices, discounts, available_in)
>>> for product, price, discount, locations in zipped:
... for location in locations:
... cond1 = discount >= 0.15
... cond2 = 20 <= price <= 30
... cond3 = location in ['East', 'North']
... if cond1 and cond2 and cond3:
... discounted_products[(product, location)] = price
>>> discounted_products
{('Apples', 'East'): 25, ('Apples', 'North'): 25, ('Cherries', 'East'): 22}
and the corresponding dictionary comprehension:
>>> zipped = zip(products, prices, discounts, available_in)
>>> discounted_products = {
... (product, location): price
... for product, price, discount, locations in zipped
... for location in locations
... if discount >= 0.15
... and 20 <= price <= 30
... and location in ['East', 'North']
... }
>>> discounted_products
{('Apples', 'East'): 25, ('Apples', 'North'): 25, ('Cherries', 'East'): 22}
We could use three if
conditions instead:
>>> zipped = zip(products, prices, discounts, available_in)
>>> discounted_products = {
... (product, location): price
... for product, price, discount, locations in zipped
... for location in locations
... if discount >= 0.15
... if 20 <= price <= 30
... if location in ['East', 'North']
... }
>>> discounted_products
{('Apples', 'East'): 25, ('Apples', 'North'): 25, ('Cherries', 'East'): 22}
This time, I won't explain the code line by line. Try to do it yourself. However, I'd like to point out the following aspects of the code:
- In the regular
for
loops, we defined the condition variables,cond1
,cond2
andcond3
. Theoretically, this is unnecessary, but I did so for readability. Otherwise, the line with the conditions would have to be very long or split into several lines. - In the dict comprehension, we don't need to do this, as the resulting code with the three conditions is readable – though it's split into three lines. However, this split doesn't decrease readability; rather, it shows that we have three conditions for the data to meet.
- The comprehension follows the same pattern as before: operation, loops, conditions. Again, you can read it from top to bottom, just like a regular sentence from left to right.
- The additional complexity (in both versions) is introduced by the nested
for
loop:for location in locations
. It's enough to understand that we're looping over locations per In my eyes, this line doesn't include too much complexity, at least not the way it does in the nestedfor
loop.
The point of this article is not to claim that Python comprehensions can be simple even in complex situations. Instead, I wanted to show that they can be more readable than the corresponding traditional for
loops even in some complex situations. So, if you decide to give up a comprehension because it's complex, remember about the alternative, which is the corresponding for
loop, and it it can be even less readable than the comprehension we've just gave up on.
Surely, it's on you whether the code is readable or not. In more advanced cases, it's easy to make the code unreadable. Let me rewrite the last example to make the point:
>>> discounted_products = {
... (product, location): price
... for product, price, discount, locations in zip(products, prices, discounts, available_in)
... for location in locations
... if discount >= 0.15 and 20 <= price <= 30 and location in ['East', 'North']
... }
>>> discounted_products
{('Apples', 'East'): 25, ('Apples', 'North'): 25, ('Cherries', 'East'): 22}
This version is less readable than the previous one – but I'd say, it's still pretty readable, especially in comparison with the traditional for
loop.
Is it really the case – and a general one – that nested comprehensions are difficult to read? In my eyes, not really. If you only remember about the powerful zip()
function, you can make things pretty readable – of course assuming that you know how zip
objects work.
Consider the following example, with multiple for
loops. It it really that incomprehensible?
Let's calculate a matrix of values given the row of x
values and the columns of y
values, like in a multiplication table:
>>> multi_table = {(x, y): x * y for x in range(10) for y in range(10)}
>>> multi_table[(5, 6)]
30
We can make this more readable by splitting into several lines:
>>> multi_table = {
... (x, y): x * y
... for x in range(10)
... for y in range(10)
... }
>>> multi_table[(5, 6)]
30
I definitely prefer the latter version, with a one-command, 5-line list comprehension, each line presenting an individual step of the process. Here, this is
- data operation: calculate
x*y
and store as a tuple(x, y)
- loops:
for
given values ofx
,for
given values ofy
This was a simple multiplication, but you can replace the first line with much more advanced calculations, and you will see that such comprehensions can make life easier and the code much simpler and more readable.
Is it not simpler than the following corresponding code?
>>> multi_table = {}
>>> for x in range(10):
... for y in range(10):
... multi_table[(x, y)] = x * y
>>> multi_table
Remember that when you need to calculate a matrix, like here, you need two loops, not the zip
object. This is because the latter doesn't calculate the matrix. Compare:
>>> x, y = range(3), range(3)
>>> [(xi, yi) for xi, yi in zip(x, y)] # the same as list(zip(x, y))
[(0, 0), (1, 1), (2, 2)]
>>> [(xi, yi) for xi in x for yi in y]
[(0, 0), (0, 1), (0, 2), (1, 0), (1, 1), (1, 2), (2, 0), (2, 1), (2, 2)]
I don't want to claim that a comprehension will always be more readable, no matter what. However, when the calculation line doesn't require long code, it usually will.
We must not forget the walrus operator, which can make comprehension code even more powerful. Look here (for Python 3.8 and newer):
>>> {
... (x, y): prod
... for x in range(7)
... for y in range(7)
... if (prod := x * y) % 2 != 0
... if y > x
... }
{(1, 3): 3, (1, 5): 5, (3, 5): 15}
This code should be clear. We're creating a dictionary in the following way:
- data operation: for a tuple of
x, y
we calculate a product ofx
andy
- loops: for
x
values fromrange(7)
, fory
values fromrange(7)
- conditions: if the product is an odd number and if
y > x
Note that this time the condition was on the result of data operation, not on the original data like before. This is why we used the walrus operator.
Using it does introduce some complexity during reading. We need to jump from the line that uses prod
to where it's actually defined, and then we need to return to the same line. So, can we do the same without the walrus operator?
Yes, we can:
>>> {
... (x, y): x * y
... for x in range(1, 7)
... for y in range(1, 7)
... if (x * y) % 2 != 0
... if y > x
... }
{(1, 3): 3, (1, 5): 5, (3, 5): 15}
Have you spotted an issue with this code? This time, it's not about readability, but about optimization: unlike in the walrus version, the product (x * y
) is calculated twice! This means the version without the walrus operator will be slower than that utilizing it.
Let's see how this goes in a regular for
loop. It doesn't need the walrus operator:
>>> multi_table = {}
>>> for x in range(7):
... for y in range(7):
... prod = x * y
... if prod % 2 != 0 and y > x:
... multi_table[(x, y)] = prod
>>> multi_table
{(1, 3): 3, (1, 5): 5, (3, 5): 15}
Honestly, the following part of the comprehension:
... (x, y): x * y
... for x in range(1, 7)
... for y in range(1, 7)
... if (x * y) % 2 != 0
... if y > x
is in my opinion much more readable than this part of the regular for
loop:
>>> for x in range(7):
... for y in range(7):
... prod = x * y
... if prod % 2 != 0 and y > x:
... multi_table[(x, y)] = prod
and this is despite the walrus operator in the former!
Pipelines to simplifying complex comprehensions
Once more, let's return to the general comprehension pattern: data operation → loop(s) → optional conditions. The first step, data operation, can be simple and complex. Sometimes, it can consist of several data operations.
If we need to combine a number of operations in one comprehension, we have several solutions to choose from, the most important being:
Join the operations. For instance, (x + 5)**2
in fact joins two operations, x + 5
and then calculating the square of the output. Let's work with another example, however: we need to join three string operations: str.lower()
, str.strip()
, and str.replace(' ','_')
. In this case, we do this:
>>> texts = [
... "Text 1",
... "the Second text ",
... " and FINALLY, the THIRD text! t"]
>>> output_join = [
... t.lower().strip().replace(' ', '_')
... for t in texts
... ]
This method will work only in simple cases like these, when you can join the operations in such a simple way, without the necessity of performing additional in-between calculations.
Use a function. Instead, we can move all the operations to a function and call it in the comprehension:
>>> def preprocess(text: str) -> str:
... return text.lower().strip().replace(' ', '_')
>>> output_func = [preprocess(t) for t in texts]
This solution can work even in very complex situations, even with many advanced operations on the data that require several steps of computation.
In fact, such a comprehension will itself be very simple, as the data operation logic is moved to the function we used to build the comprehension (here, preprocess()
). While often it doesn't have to be a good idea to define a function that's used only once, it can work great when it helps to organize the code.
If you choose this method, remember to use an informative name for the function. Only then can such a comprehension be readable – even when the data operation logic implemented inside the function is complex.
Use a comprehension pipeline. In that case, we don't use a single comprehension but call a sequence of comprehensions, one after another. This is called a comprehension pipeline. Let's create a generator pipeline:
>>> step1 = (t.lower() for t in texts)
>>> step2 = (t.strip() for t in step1)
>>> output_gen_pipe= (t.replace(' ', '_') for t in step2)
and the corresponding pipeline based on lists (a listcomp pipeline):
>>> step1 = [t.lower() for t in texts]
>>> step2 = [t.strip() for t in step1]
>>> output_list_pipe = [t.replace(' ', '_') for t in step2]
Note that the former version produces a generator, so we need to evaluate it; we'll use a list for this – see below.
Note that all the four approaches lead to the very same results:
>>> (output_join
... == output_func
... == list(output_gen_pipe)
... == output_list_pipe)
True
A comprehension pipeline can constitute a powerful solution. However, not always will it work, only for actual pipelines.
This is an advanced topic, so we'll not cover it here. If you're interested in learning more about the topic, you'll find a lot of related information in the following articles:
Building Generator Pipelines in Python
Conclusion
If you've been using Python for some time already, you've probably heard warnings to use comprehensions only for simple situations, and for
loops otherwise. How to decide if a particular situation is too complex to implement a comprehension?
For this, you need practice and experience. Experienced Python developers almost never hesitate before making such a decision. They usually know which choice is better in the given context.
If you aren't an advanced Python developer, you need to gain such skills. Don't worry if you're not there yet; practice this skill by implementing as many comprehensions as you can – even if your gut feeling suggests that the context is too difficult for a comprehension. Unless the context is indeed very complex and requires multiple operations in each iteration, try to implement both comprehension and a for
loop, and compare them.
Even when each iteration requires multiple data operations, you can use a comprehension, using a simple trick we discussed above: move data operations to a function and call it in each iteration of the comprehension's loop. Such an approach can provide significantly simpler code than a for
loop with all these operations implemented inside the loop's code block.
When a comprehension appears too difficult for you to write, don't give up too early. Try to implement it anyway, and if you succeed, it may occur to be quite a neat solution: the difficulty of a comprehension's code doesn't have to be proportional to the difficulty of implementing it.
Usually, you can choose between a for
loop and the corresponding comprehension. Sometimes, however, you need to use a single command (even if long, since it can be split into several lines), in which case you simply need a comprehension.
Consider, for instance, the parametrization of fixtures in Pytest. You can create a list of parameters, passed as the params
argument, either outside the call to pytest.fixture()
or directly inside it. Often, it's better to do it inside, because this makes the code clearer and better organized. Only when the parametrization code becomes too complex to include it inside the call to pytest.fixture()
, I move it outside.
Python syntactic sugar like comprehensions (including generator expressions), decorators, the walrus operator and others make Python so powerful and so readable. Hence, don't avoid them. They exist to make Programming in Python easier. In addition, comprehensions add a lot of beauty to Python. Learn how to use them, and you'll start enjoying Python much more than you do without them.
Not all agree with such reasoning. Some people claim that you should not use such syntactic sugar because those who don't know Python well won't understand such code. I completely disagree with such an approach. If you decide to use a programming language, why should you not use its native syntax and syntactic sugar, which are often among the most powerful programming tools of this language?
And if you want to use C syntax, use C, not Python. Otherwise, your Python code won't look like idiomatic Python code, even if it will work correctly. Such code can be lengthy, suboptimal and difficult to understand.
The claim that you should limit using comprehensions to the simplest situations has become an overused myth. You should indeed avoid using too complicated comprehensions, but what does this mean? Do two if
conditions or nested loops make a comprehension too complicated? No, they don't!
Therefore, follow this rule: Don't use a Python comprehension if it's too complex to understand compared to the corresponding for
loop. However, if the comprehension is easier to understand than the loop, use it anyway, even if it spans several lines and appears challenging.
In other words, base your decision to use a comprehension on its readability and that of the corresponding for
loop. If performance is a concern, consider it, too; this often means preferring the comprehension over the for loop.