Embedding Markdown Files in a Streamlit Dashboard
PYTHON PROGRAMMING

Streamlit offers a simple yet efficient tool for creating interactive dashboards in Python:
This interactivity is what makes dashboards so great. Sometimes, however, some parts of a dashboard aren't interactive. To format them, you can use various functions, and among them is st.Markdown()
(st
originating from import streamlit as st
):
It allows for using typical Markdown syntax to format text, so to me it's the most useful text-formatting Streamlit function.
If a large part of the dashboard is to be formatted that way, you may simplify the dashboard's app code by moving this part to a Markdown file. That way, the app code will be shorter and simpler. It's actually very easy to do, and this article shows how to do it.
How to
Let's create a very simple Streamlit dashboard. It'll contain two tabs, one with the contents of a Markdown file (say, tab1.md
) and the other with just a string. This is the contents of the tab1.md
file:
# Streamlit and Markdown
You can embed Markdown files in Streamlit dashboards.
It will render inline `code`, code blocks:
```Python
GLOBAL_VARIABLE = 10
class FooException(Exception):
...
def foo(x):
return x**2
if __name__ == "__main__":
print(foo(2))
text formatting, like italics or bold font.
Try yourself!
This is what we need to do:
1. Read the contents of the Markdown file to an object. We'll define a function to do that. Thanks to this, it'll be easy to reuse it for as many Markdown files as you need.
2. Create tabs in Streamlit using `st.tabs()`.
3. Embed the Markdown content in the first tab, using `st.markdown()`.
Here's an example implementation:
```python
# app.py
import streamlit as st
def read_markdown_file(file_path):
with open(file_path, "r") as file:
return file.read()
tab1_content = read_markdown_file("tab1.md")
tab1, tab2 = st.tabs(["Markdown Tab", "Other Tab"])
with tab1:
st.markdown(tab1_content)
with tab2:
st.write("This is tab2!")
Let's run the app:
(venv-streamlit) > streamlit run app.py
You will see this:

Example: Play with the Iris dataset
Above, I showed you a tool. Now, let's use it to create an interactive presentation using Streamlit.
The full code I used to create the dashboard is available from the following GitHub repository:
GitHub – nyggus/play_with_iris: Use Markdown files in Streamlit dashboards
The code uses two Markdown files, intro.md
and play.md
. The first one is used to show the whole contents of one of the tabs, "Introduction". The other one is used to present a fragment of the third tab, "Play with the data". This is something we didn't do above – this tab first shows the explanation of what the user can do on the tab, and it uses a Markdown file for that.
First, let's analyze the dashboard. This is its default view:

The second tab just show the data, so I will omit it here. The third tab offers much more – see the explanation on the screenshot below.

Everything below the header "Play with the Iris data" and above the data editor comes from the play.md
file.
The remaining part of the tab shows:
- the data editor, in which the user can change the data
- SPLOM (a scatterplot matrix) of the data:

- boxplots of a trait selected by the user using a dropdown menu:
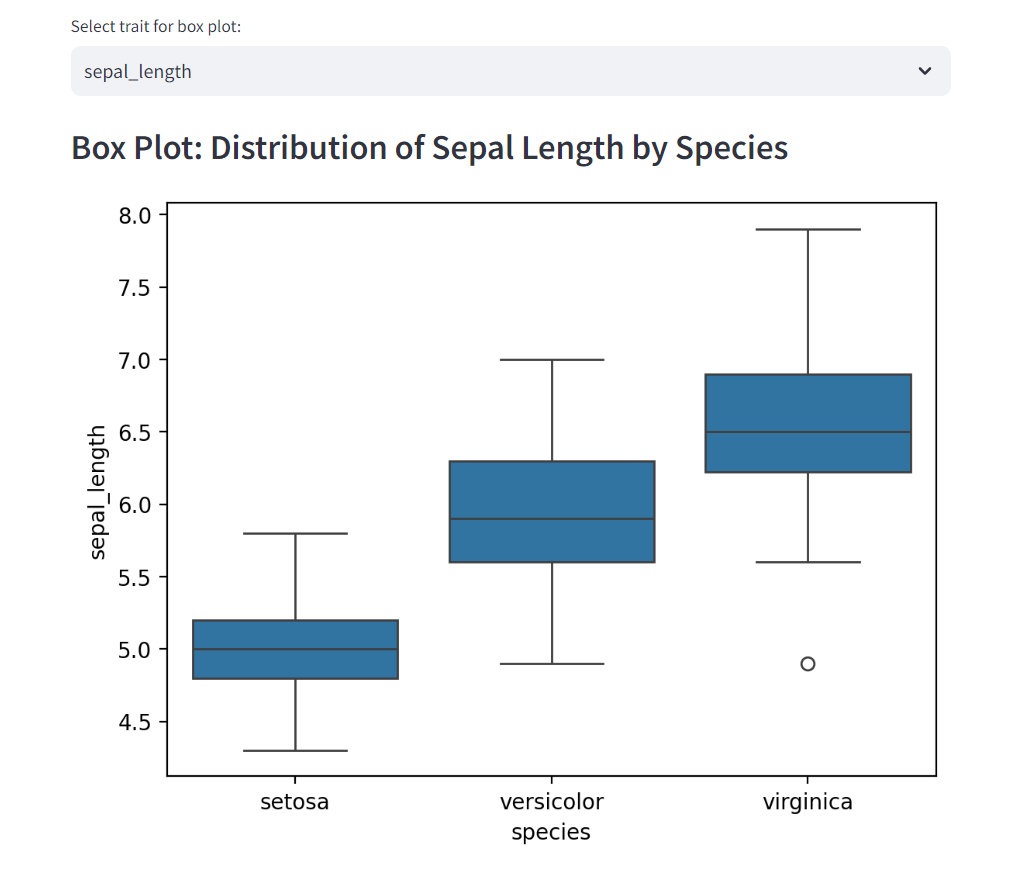
- the "Refresh" button
- logs of the changes since the last click of the "Refresh" button:

All of these elements of the tab are included directly in the app.py
file, as they couldn't've been included in the Markdown file.
Now, let's have a look at some of the code – that in which we use the two Markdown files:
st.title("The iris dataset")
contents = {}
contents["intro"] = read_markdown_file("intro.md")
contents["play"] = read_markdown_file("play.md")
tab_intro, tab_data, tab_play = st.tabs(
[
"Introduction",
"See the data",
"Play with the data",
]
)
iris = pd.read_csv("iris.csv")
# Initialize session state if not already done
if "iris_edit" not in st.session_state:
st.session_state["iris_edit"] = iris.copy()
if "log" not in st.session_state:
st.session_state["log"] = []
def reset_data():
st.session_state["iris_edit"] = iris.copy()
st.session_state["log"] = []
with tab_intro:
st.markdown(contents["intro"])
with tab_data:
st.write("This is the famous iris dataset:")
st.dataframe(iris)
with tab_play:
st.subheader("Play with the Iris data")
st.markdown(contents["play"])
# Editable dataframe
old_data = st.session_state["iris_edit"].copy()
iris_edit = st.data_editor(
st.session_state["iris_edit"], num_rows="dynamic"
)
st.session_state["iris_edit"] = iris_edit
You can see the remaining part of the code in the GitHub repository.
As you can see, the code for the first tab – which presents quite long text – is very short, just two lines:
with tab_intro:
st.markdown(contents["intro"])
The third tab uses the play.md
file:
with tab_play:
st.subheader("Play with the Iris data")
st.markdown(contents["play"])
We could, actually, move the subheader to the file, but I decided to keep it here for readability – but I don't think it would make much of a difference.
Imagine that we included the contents of these two Markdown files directly in the code, using the st.markdown()
function. This would make the code much, much longer and thus much less readable. In addition, the code would be less readable, as we would have to add the text as strings. It's much easier to create a Markdown file. Note only it makes the code shorted and more readable, but also it makes the text itself more readable – it's easier to read it in the file than inside the st.markdown()
function. Compare:

with, for instance, this:

Conclusion
This was simple, wasn't it? All you need to do is put the Markdown content in a file, read the file's contents into an object, and pass this object to the st.markdown()
function.
This tool is especially handy when you need to include longer fragments of Markdown content in your Streamlit dashboard. For shorter snippets, it's easier to include them directly in the st.markdown()
function.
I've used this method to create quite complex interactive dashboards, reading from multiple Markdown files. I've also found Streamlit to be useful for creating interactive presentations. Streamlit is great for such purposes. However, if you include all the Markdown content inside the code, it can quickly become difficult to read – and I hate code that's difficult to read. When one day I saw my own code for a Streamlit dashboard full of Markdown content, I thought there must be a better way of doing this.
And indeed there is. But this method has one more advantage that you may sometimes consider even more important than clear code: not only is such code better organized, but you can also reuse these Markdown files. For instance, you can create a single Markdown file that joins the contents of all of them. Often, Markdown files are used to write documentation – you can use the very same files in a dashboard, without the necessity of copy-pasting their contents. Any update to such a file will be visible in both the dashboard and the documentation.
Finally, I'd like to invite you to clone the repository and build the dashboard on your machine. It contains more than just the presentation of how to use Markdown files. But the tools I used there are a different story, I will leave it for another day and another article.