Emergent Abilities in AI: Are We Chasing a Myth?
Opinion

The emergent properties of a model
Emergent properties are not only a concept that belongs to Artificial Intelligence but to all disciplines (from physics to biology). This concept has always fascinated scientists, both in describing and trying to understand the origin. Nobel Prize-winning physicist P.W. Anderson synthesized the idea with "More Is Different." In a certain, sense it can be defined as an emergent property, a property that appears as the complexity of the system increases and cannot be predicted.
For example, you can encode information with a small molecule, but DNA (a large molecule) is encoding a genome. Or a small amount of Uranium is not leading to a nuclear reaction.

Recently the same behavior has been observed with artificial intelligence models, one of the most commonly used definitions being: "An ability is emergent if it is not present in smaller models but is present in larger models."
What does this mean and how is it observed?
OpenAI stated in an article that the performance of a model follows a scaling law: the more data and parameters, the better the performance. In the case of emergent properties, what is expected is a particular pattern: as the number of parameters increases, performance is almost random until at a certain threshold a certain property is observed (performance begins to improve noticeably). Basically, we see a sharp turn of the curve (called phase transition). This also is called emergent, because it is impossible to predict by examining a small-scale model.

So in short, we can say that a property is considered emergent if it satisfies these two conditions:
- Sharpness, the transition is discontinuous between being present or not present.
- Unpredictability, its appearance cannot be predicted as parameters increase
In addition, scaling a transformer mainly takes into consideration three factors: the amount of computation, the number of model parameters, and the training dataset size.
All three factors make a model expensive. On the other hand, these properties are particularly sought after and have also been used as a justification for increasing the number of parameters (despite the fact that models are not trained optimally).

Several studies have also focused on why these properties emerge, why they do so in this way, and why at a particular threshold. According to some the emergence of some properties can be predicted:
For instance, if a multi-step reasoning task requires l steps of sequential computation, this might require a model with a depth of at least O (l) layers. (source)
Alternative explanations have been proposed such as that the larger number of parameters aids memorization. The model gains knowledge as the data increases and at some point reaches critical mass to be able to support that property
In addition, some authors have proposed that different architectures and better data quality could lead to the appearance of these properties in even smaller models.
This was noted with LLaMA, where a significantly smaller model of GPT-3 showed comparable properties and performance.
META's LLaMA: A small language model beating giants
In any case, the question remains, why do these properties appear?
Anthropic in one study states that:
large generative models have a paradoxical combination of high predictability – model loss improves in relation to resources expended on training, and tends to correlate loosely with improved performance on many tasks – and high unpredictability – specific model capabilities, inputs, and outputs can't be predicted ahead of time (source)
In simpler words, for an LLM there are things we can predict and things we cannot predict. For example, the scaling law allows us to predict that increasing the number of parameters will improve performance in scale, but at the same time, we cannot predict the emergence of certain properties that instead appear abruptly as the parameters increase.
So according to this principle, we should not even try to predict them.


Why are we so interested in predicting these properties?
The first reason is pure economics: if a property emerges only at a certain number of parameters, we cannot use a smaller model. This significantly increases the cost of both training and hardware. On the other hand, if a property cannot be predicted, we cannot even estimate the cost of obtaining it.
Second, it justifies the inordinate increase in parameters in the search for new properties that appear at trillions of parameters. After all, this may be the only way to be able to obtain certain properties.
Moreover, this presents a safety problem, as we cannot predict what property a model will have at a certain scale. A model may develop problematic properties and may not be safe for deployment. Also, models this large are more difficult to test for bias and harm.
Moreover, scaling law and emergent properties have been one of the reasons for the rush to large models.

This opens a scary scenario, on the one hand, we have an explosion of open-source models, a reduction in the cost of their training, and an increase in the use of chatbots. But on the other hand, we have no way to predict the properties of these models.
What if emerging properties were a mirage?

In 2020, Google researchers realized the potential of LLMs and predicted that they would be transformative. So they asked the community to provide examples of tasks that were both different and difficult and that could then be used to test the capabilities of an LLM. Thus was born the Beyond the Imitation Game Benchmark (BIG-bench) project.
This project was actually also focused on studying emergent and surprising properties and trying to be able to understand their origin.
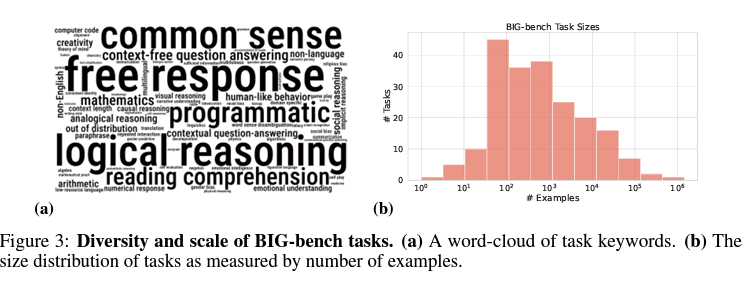
Indeed, the dataset and article discussed the emergence of probabilities and tried to provide explanations. For example, models over ten billion parameters could solve three-digit addition or two-digit multiplication problems.
Building on this article, researchers at Stanford questioned in a recent paper the very concept of emergent property for a language model.
In fact, the authors noticed that emergent properties seemed to appear only with metrics that were nonlinear or otherwise discontinuous.
The authors provided an alternative hypothesis to the emergence of properties. according to them is the choice of performance measurement. In other, words the error per-tokens grows smoothly, continuously, and predictably with increasing model scale. But then the study authors measure performance for tasks using discontinuous metrics, and so it appears that the model performs the task abruptly.
In other words, a small model performs decently on a task but we can't detect it because the chosen metric is discontinuous, and only under a certain error (achieved over a certain model size) can we observe performance in the tasks.

According to the authors, it is also the small number of examples for the test that leads to small models not being properly evaluated.
To demonstrate this, the authors started with the scaling law, according to which performance (or error) increases as a function of the number of metrics and which is indeed shown to be consistent at different magnitudes. As the authors note, many metrics require all tokens in the sequence to be correct, especially when dealing with long sequences leads to seeing sharp increases.
They were able to do these experiments using InstructGPT/GPT-3 because models such as LaMDA, Gopher, and Chinchilla are unfortunately not accessible. This prevented them to do an extensive evaluation of the different models. Since LLMs are trained only on text (and GPT is trained on predicting the next word), one of the surprising abilities of LLMs is integer arithmetic tasks. As showed from the GPT-3 introduction article, this property is defined as emergent in function of the scale/

As seen in the image (top) when performance is measured with a non-linear metric we see an emergent property. when a linear metric is used (bottom) on the other hand we see a continuous and predictable increase in performance as a function of scale.

In addition, the authors noted that by increasing the data for small model evaluation even with nonlinear metrics the effect was not as pronounced. In other words, if the test dataset is larger even with nonlinear metrics we do not observe such a dramatic effect.
In fact, with low resolution (few test data) is more probable to assist in zero accuracies for small models, which support the claim that a property emerges just after a certain threshold.

The authors then decided to extend to a meta-analysis on emerging properties, using BigBench (since it is public and is also well documented). In addition, this dataset offers more than one evaluation metric. When the authors look at nonlinear metrics (Exact String Match, Multiple Choice Grade, ROUGE-L-Sum) emergent properties could be observed. On the other hand, using linear metrics no emergent properties are observed.
The most surprising finding is that 92 % of claimed emergent abilities come from using two discontinuous metrics Multiple Choice Grade and Exact String Match.

So if indeed the cause of emergent properties is the use of discontinuous metrics, just changing metrics would be enough to make them disappear. Keeping the model and task fixed, just change the rating metrics and the emergent properties disappear. In this case, the authors simply reused the outputs of the LaMDA family of models and changed the metrics from discontinuous (Multiple Choice Grade) to continuous (Brier Score).

one final question remains: but if emergent properties appear by choosing discontinuous metrics can we create emergent properties using discontinuous metrics?
The authors take as an example the classification ability of the handwritten digits dataset (MNIST or the data scientist's favorite dataset). Anyone who has tried to train a convolutional network on this dataset has noticed that even with a few layers a decent result is obtained. Increasing the number of layers can improve the accuracy. If it were an emergent property, we would expect that at first, the accuracy would be near zero, and by increasing the parameters above a certain threshold the accuracy would start to increase significantly.
The authors used the LeNet family (several models with increasing numbers of parameters). They simply chose a new metric called subset accuracy: "1 if the network classifies K out of K (independent) test data correctly, 0 otherwise."
While using test accuracy we notice the classic increase in accuracy with a sigmoidal trend, with the new discontinuous metric it seems that the ability to classify handwritten digits is an emergent property.

The authors provide another example: image reconstruction with autoencoders. Just by creating a new discontinuous metric, the ability to reconstruct autoencoders becomes an emergent property.

The authors conclude:
Emergent abilities may be creations of the researcher's choices, not a fundamental property of the model family on the specific task (source)
In other words, if someone wants an emergent property all they have to do is choose a discontinuous metric and magically they will see a property appear over a certain threshold of parameters.
The authors conservatively state, "This paper should be interpreted as claiming that large language models cannot display emergent abilities." They merely claim that the properties seen so far are instead produced by choice from the metric.
Now it is true that until you see a black swan, all swans are white. But the next time an emergent property appears, though, one must check under what conditions it appears. Also, this is another call to rethink benchmarks that may now be unsuitable for measuring the quality of a model. Second, LLMs should be open-source, because any claim could simply be due to a choice of evaluation.
Parting thoughts
For a long time, emergent properties have been considered among the most surprising behaviors of Large Language Models (LLMs). The fact that beyond a certain number of parameters, an ability would emerge was a fascinating but at the same time terrifying concept. Indeed, on the one hand, it was further justification to look for larger and larger models. On the other, the emergence of potentially dangerous abilities without warning was problematic.
This article surprisingly shows how the choice of evaluation metrics leads to the emergence of properties. This prompts a rethinking of benchmarks with a new focus on the choice of evaluation metrics. Second, emergent properties may not exist.
More broadly, all along, many authors choose the evaluation metric that makes their data shine. Thus, we can only be sure of a claim when a model and its outputs are open to the public for independent scientific investigations.
If you have found this interesting:
You can look for my other articles, you can also subscribe to get notified when I publish articles, you can become a Medium member to access all its stories (affiliate links of the platform for which I get small revenues without cost to you) and you can also connect or reach me on LinkedIn.
Here is the link to my GitHub repository, where I am planning to collect code and many resources related to Machine Learning, artificial intelligence, and more.
GitHub – SalvatoreRa/tutorial: Tutorials on machine learning, artificial intelligence, data science…
or you may be interested in one of my recent articles:
PMC-LLaMA: Because Googling Symptoms is Not Enough
Welcome Back 80s: Transformers Could Be Blown Away by Convolution
Looking into Your Eyes: How Google AI Model Can Predict Your Age from the Eye