Ensemble of Classifiers: Voting Classifier

The word Ensemble in the context of ML refers to a collection of a finite number of ML models (may include ANN), trained for the same task. Usually, the models are trained independently and then their predictions are combined.
When the predictions from different models differ, it is sometimes more useful to use the ensemble for Classification than any individual classifier. Here, we would like to combine different classifiers and create an ensemble and then use the ensemble for the prediction task. What will be discussed in this post?
- Use Sklearn's VotingClassifier to build an ensemble.
- What is Hard and Soft Voting in VotingClassifier?
- Check individual model performance with VotingClassifier.
- Finally, use GridSearchCV + VotingClassifier to find the best model parameters for individual models.
Let's begin!
Data Preparation:
To see an example of VotingClassifier in action, I'm using the Heart Failure Prediction dataset (available under open database licensing). Here the task is the binary classification for predicting whether a patient with specific attributes may have heart disease or not. The dataset has 10 attributes including their age, sex, resting blood pressure etc., for data collected over 900 patients. Let's check some distributions for different parameters. We check the ‘ClassLabel' counts (1 represents heart disease, 0 represents healthy), i.e. healthy and ill population as a function of Sex.

In general, we see proportionately more Males are ill compared to Females. We can also check individual features such as Cholesterol and Resting BP distribution as below and we see that both the Cholesterol and Resting BP are higher for ill patients, especially for females.

For numerical features, one can also use Boxplots to see distributions where the box represents the range of the central 50% of the data.

We see some issues with the dataset here; There are quite a few data points with zero Cholesterols and RestingBP. Even though it is indeed possible to have really low cholesterol, to have zero for many data points (patients), is it a little too accidental? Anyway, as our main objective is to implement VotingClassifier so we leave those data points as they are and proceed to the next stage.
Train-Test and Classification:
After some preliminary analysis, we prepare the dataset for training and testing different classifiers and compare their performances. First, we split the data into train and test sets, separately standardize the numerical columns (to prevent data leakage) and initialize 3 different classifiers- Support Vector Machines, Logistic Regression and AdaBoost, which itself is an Ensemble Learning method specifically falling under the Boosting method (the other one being known as ‘Bagging'). Next is to train these classifiers using the training set and check predictions for the test set. We check 3 different performance metrics-precision, recall and F1 score. Below is the code block:
Below are the scores:
>>> SVC, LogReg, AdaBoost Precisions: 0.9096562 0.8915564 0.8825301
>>> SVC, LogReg, AdaBoost Recalls: 0.9086956 0.8913043 0.8826086
>>> SVC, LogReg, AdaBoost F1-scores: 0.90831418 0.8910015 0.8825525
We see that on average the F1 scores are around ~89%. One can also check the feature importance for the AdaBoost classifier and expectedly Cholesterol is one of the main important features. Below is the plot:
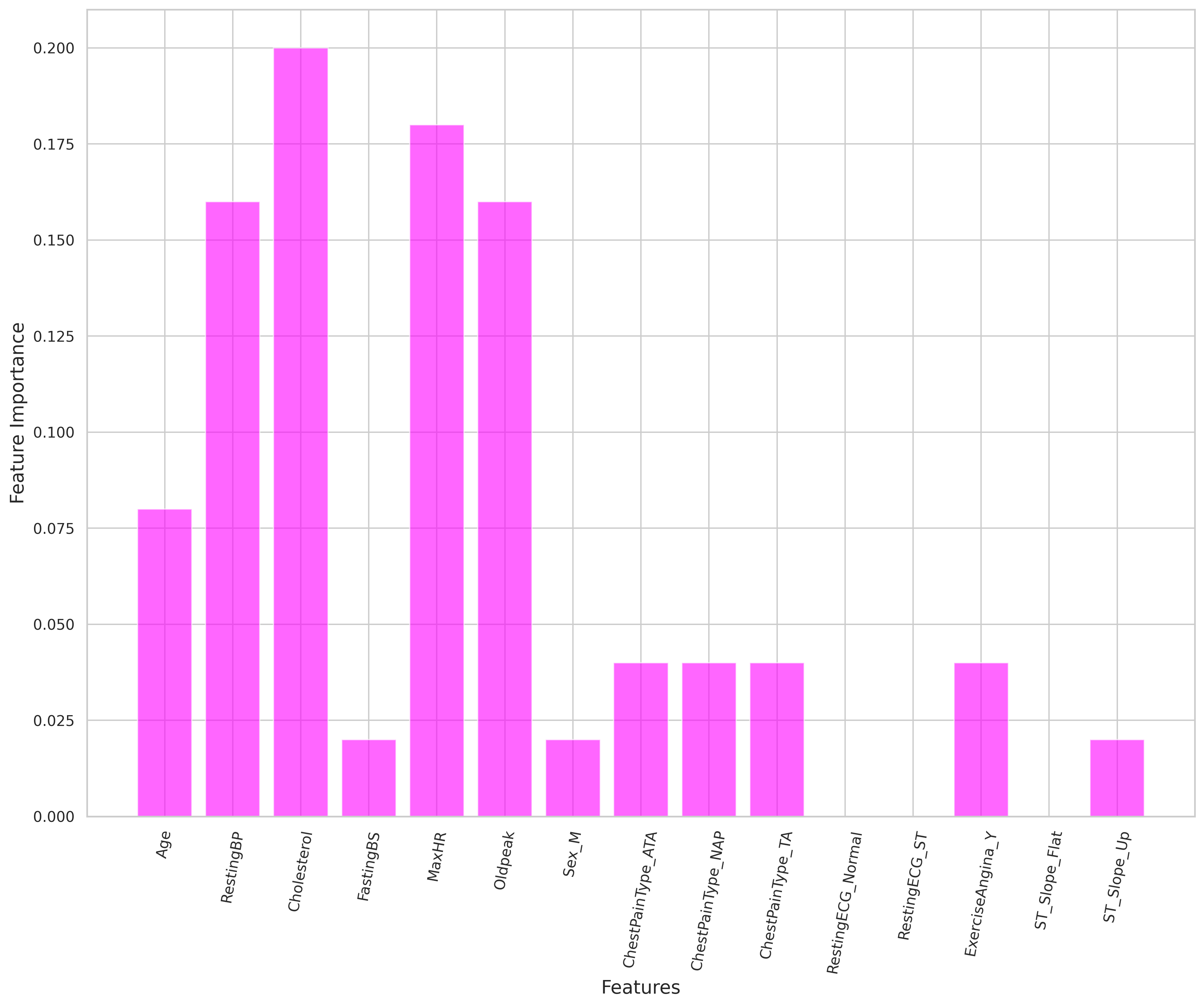
The confusion matrix for the AdaBoost classifier separately looks as below:

VotingClassifier to Form an Ensemble:
VotingClassifier combines different Machine Learning classifiers and uses a voting rule (‘soft' or ‘hard') to predict the class labels.
It balances out the individual weaknesses of models when their performance is almost the same; here we will combine individual classifiers used before to build an ensemble. But what is soft and hard voting?
Majority (‘Hard') Voting: The predicted class label for a particular sample is the class label that represents the majority (mode) of the class labels predicted by each individual classifier. Let's look at the table below for an example of a classification task with 3 classes
+-------------+---------+---------+--------+
| Classifier | Class1 | Class2 | Class3 |
+-------------+---------+---------+--------+
| SVC | 0.2 | 0.3 | 0.5 |
| LogReg | 0.3 | 0.4 | 0.3 |
| AdaBoost | 0.1 | 0.3 | 0.6 |
+-------------+---------+---------+--------+
Here, SVC and AdaBoost both predict Class3 as the output label and in the Hard Voting scenario this will be selected.
Soft Voting: Soft voting returns the class label as argmax of the sum of predicted probabilities; It's also possible to assign a weight array for the classifiers involved in creating the ensemble. Below is an example code block
svc_classifier = SVC(probability=True)
logreg_classifier = LogisticRegression()
adaboost_classifier = AdaBoostClassifier()
estimators = [('svm', svc_classifier), ('logreg', logreg_classifier),
('adaboost', adaboost_classifier)]
voting_classifier_soft = VotingClassifier(estimators=estimators,
voting='soft',
weights=[1, 1, 1])
Once again take a look at the table below for an example classification task with weights [1, 1, 1] i.e. all the classifiers are equally weighted
+ - - - - - - - + - - - - - + - - - - -+ - - - - -+
| Classifier | Class1 | Class2 | Class3 |
+ - - - - - - - + - - - - - + - - - - -+ - - - - -+
| SVC | w1 x 0.2 | w1 x 0.3 | w1 x 0.5 |
| LogReg | w2 x 0.3 | w2 x 0.4 | w2 x 0.3 |
| AdaBoost | w3 x 0.1 | w3 x 0.3 | w1 x 0.6 |
| Weighted Avg. | 0.20 | 0.33 | 0.47 |
+ - - - - - - - + - - - - - + - - - - -+ - - - - -+
Here Class3 will be chosen under the ‘Soft' voting scheme. For more on VotingClassifier you can also check the official Sklearn guide. Let's now build a VotingClassifier and use this ensemble to train and perform predictions on the test set using both ‘Soft' and ‘Hard' schemes.
Using VotingClassifier indeed we could see improvement in the performance metrics compared to the individual estimators. Similarly, we can also plot the Confusion Matrix to further verify the findings.

VotingClassifier and GridSearchCV:
It's also possible to tune the parameters (‘hyperparameters') of individual estimators within VotingClassifier using GridSearchCV. GridSearch exhaustively considers all parameter combinations and uses Cross-Validation to find the best hyperparameters. We have gone through examples of building an analysis pipeline with an estimator like Support Vector Machine, Principal Component Analysis and GridSearchCV before here. We show that it is fairly simple to obtain the best parameters of individual estimators within VotingClassifier and below is an example code block:
For parameter space (in line 18), we scour over SVM ‘C' and ‘gamma' parameters (with a radial basis function kernel) which we have discussed in detail before. For the logistic regression, we check over several values of the inverse of the regularization strength parameter; finally, for the AdaBoost classifier which uses decision trees as base estimators, we check over a few possible values of the number of estimators where boosting is terminated.
Conclusions: In this post through the VotingClassifier, we have discussed one of the important concepts in machine learning, ensemble techniques. We build an ensemble using individual estimators whose predictions are then combined either via ‘Hard' or ‘Soft' voting scheme for an example classification task. It is generally argued that combining several different estimators may or may not beat the performance of the best estimator but the ensemble helps to reduce the risk of selection of a poorly performing classifier [1].
Given the task at your hand, you can certainly consider the VotingClassifier ensemble technique than choosing the best classifier out of many.
Stay strong! Cheers!!
References:
[1] "Ensemble based systems in decision making"; R. Polikar, IEEExplore, DOI: 10.1109/MCAS.2006.1688199.
[2] Sklearn VotingClassifier: User Guide.
[3] "Ensemble-based Classifiers"; L. Rokach, Artificial Intelligence Review 33, 1–39 (2010). Springer.
[4] Codes and Notebooks: GitHub.
[5] Heart Failure Prediction Data; Available Under Open Database License (ODbl); Link.