Exploring mergekit for Model Merge, AutoEval for Model Evaluation, and DPO for Model Fine-tuning

Let's continue our learning journey of Maxime Labonne‘s llm-course, which is pure gold for the community. This time, we will focus on model merge, evaluation, and fine-tuning.
Maxime has a great article titled Merge Large Language Models with mergekit. I highly recommend you check it out first. We will not repeat the steps he has already laid out in his article, but we will explore some details I came across that might be helpful to you.
High-Level Overview
We are going to experiment with model merge, model evaluation, and model fine-tuning in the following steps:
- Using LazyMergekit, we merge two models from the Hugging Face hub,
mistralai/Mistral-7B-Instruct-v0.2
andjan-hq/trinity-v1
. - Run AutoEval on the base model
mistralai/Mistral-7B-Instruct-v0.2
. - Run AutoEval on the merged model
MistralTrinity-7b-slerp
. - Supervised fine-tune the merged model with QLoRA. Run AutoEval on the fine-tuned model.
- Fine-tune the merged model with DPO. Run AutoEval on the fine-tuned model.

Let's dive in.
First, how do we select which models to merge?
Model Selection Criteria
Determining whether two or multiple models can be merged involves evaluating several key attributes and considerations:
- Model Architecture: Model architecture is a crucial consideration when merging models. Ensure the models share a compatible architecture (e.g., both transformer-based). Merging dissimilar architectures is often challenging. The Hugging Face model card usually details a model's architecture. If you cannot find the model architecture info, you can trial-and-error with Maxime's LazyMergekit, which we will explore later. If you encounter an error, it's usually because of the incompatibility of the model architectures.
- Dependencies and Libraries: Ensure that the dependencies and libraries used in the models are compatible. Conflicts here can make merging models challenging even if the models share compatible architectures. Again, turn to the model card for details such as the library used in a model.
- Parameters: Examine the number of parameters in each model. Merging models with vastly different parameter counts can create computational challenges.
- Compatibility in Objective: The models should share a common or complementary objective. If the goals of the models are too divergent, merging them may not be practical or beneficial.
- Layer Compatibility: If merging specific layers, ensure they operate on compatible data shapes and dimensions. Adapters or additional processing might be needed for seamless integration.
- Output Compatibility: The outputs of the models should be in a format that can be logically combined. For example, if one model outputs probabilities and another outputs classifications, you need a strategy to reconcile these outputs.
- Regulatory and Ethical Considerations: If the models are used in areas with strict regulatory or ethical guidelines (like healthcare or finance), ensure that merging them doesn't violate any of these regulations.
Evaluating these attributes will help determine the feasibility and potential effectiveness of merging two or multiple models. In some cases, adjustments and preprocessing might be required to align the models adequately before a successful merge can be achieved.
mergekit
mergekit is a toolkit for merging pre-trained language models. Developed by Charles Goddard, mergekit supports models such as Llama, Mistral, GPT-NeoX, StableLM, and more. It runs on either GPU or CPU. It supports many merge methods.
Based on mergekit, Maxime developed LazyMergekit, a Colab notebook that allows you to easily merge multiple models using mergekit.
With LazyMergekit, our notebook looks extremely simple using the merge method SLERP (Spherical Linear Interpolation), which is currently the most popular merge method, and it merges two models only:
- Give the new merged model a meaningful name.
- Specify the source models and their layer ranges. If you need more clarification about the layers of your model, check the model's
config.json
in its Hugging Face repo. - Specify the base model.
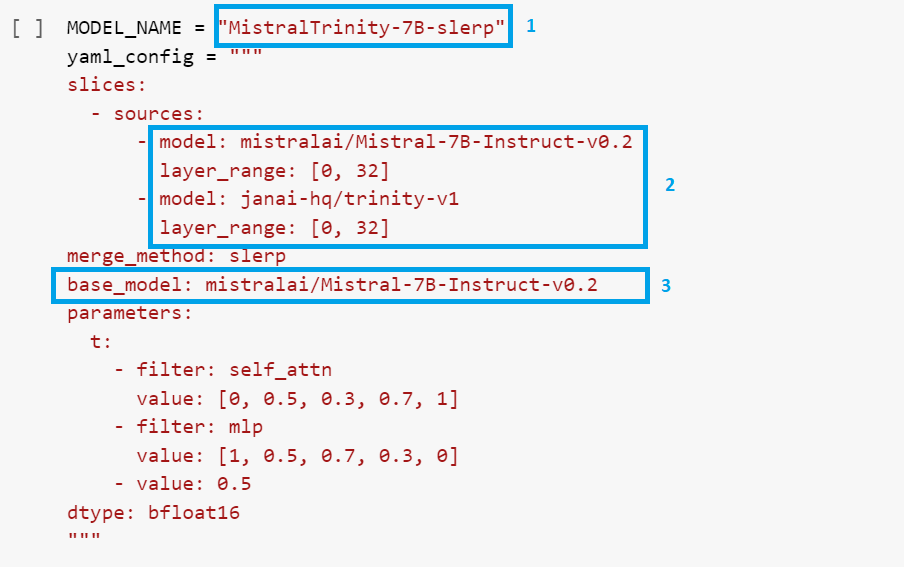
If you run into any error while executing LazyMergekit, first check the issues (both open and closed) in the mergekit GitHub repo; chances are someone else may have already run into the same error you see. In many cases, errors usually stem from the models being incompatible for merge. Try a different model to merge with your base model until you find one compatible.
If you didn't run into any error while executing LazyMergekit, you should see your newly merged model in your Hugging Face hub.
Our newly merged model MistralTrinity-7B-slerp
is now a new born in the Hugging Face hub!

AutoEval
You are excited to see your first merged model born! The question quickly arises: how do you know if this merged model outperforms the base model? Let's prove it.
Model evaluation is an essential aspect of developing and refining models. Various evaluation frameworks and benchmarks have been designed to assess the different capabilities of these models.
Again, Maxime has us covered! He developed LLM AutoEval, a tool leveraging lm-evaluation-harness, and simplifies evaluating LLMs using his convenient Colab notebook. You simply specify the name of your model, a GPU, and press run! It's also available in Hugging Face Space, check it out!

Notice you need to provide two tokens: RunPod and GitHub.
What happens under the hood of AutoEval is three steps:
- Automated setup and execution using RunPod; see more details in the RunPod section below.
- Customizable evaluation parameters for tailored benchmarking.
- Generate a summary and upload it to GitHub Gist for easy sharing and reference.
AutoEval uses the nous
benchmark suite, which contains the following list of tasks:
- AGIEval: a human-centric benchmark designed to evaluate foundation models' general abilities in tasks pertinent to human cognition and problem-solving. AGIEval v1.0 contains 20 tasks, including two cloze tasks (Gaokao-Math-Cloze and MATH) and 18 multi-choice question answering tasks.
- GPT4ALL: a benchmark suite for evaluating the factual language understanding of LLMs. It consists of various tasks designed to assess an LLM's ability to understand and respond to factual queries. Some of the tasks in GPT4All include open-ended question answering, closed-ended question-answering, text summarization, and natural language inference.
- TruthfulQA: a benchmark suite for evaluating the truthfulness of LLMs. It consists of various tasks designed to assess an LLM's ability to distinguish between true and false statements. Some of the tasks in TruthfulQA include multiple-choice questions and textual entailment.
- BigBench: an extensive benchmark suite that aims to evaluate and measure the capabilities of models across a wide range of tasks. It includes tests for reasoning, language understanding, problem-solving, and more. The idea behind BigBench is to provide a comprehensive and challenging set of tasks that can reveal the strengths and weaknesses of AI models in various domains.
In conclusion, these Model Evaluation frameworks offer complementary approaches to assessing model capabilities:
- AGIEval aims for a comprehensive assessment of AGI progress.
- GPT4ALL focuses on language model performance.
- TruthfulQA emphasizes truthfulness in question answering.
- BigBench targets knowledge, reasoning, and learning.
One limitation of AutoEval is that it currently does not support evaluating quantized models yet as of this writing.
RunPod
RunPod is a globally distributed GPU cloud specifically designed for AI and machine learning applications. It offers GPU instances, serverless GPUs, and AI endpoints to developers and researchers in AI and machine learning.
AutoEval uses RunPod to execute the model evaluation. Upon clicking the "Run" button in the AutoEval notebook, you will be instructed that your pod has started on the URL https://www.runpod.io/console/pods, where you will see your running pod instance.
Depending on your model, the evaluation could take hours. In our case, the evaluation for our base model Mistral-7B-Instruct-v0.2
was completed after 11 hours and 26 minutes.
RunPod Tips and Tricks
Here are a few tips and tricks for running evaluation in RunPod:
- You need to prepay your account before you can kick off a run. Without prepaying, your workload terminates after a few seconds without warning or message.
- If you are unsure whether your evaluation succeeded, check your GitHub gist. If you see "Error: File does not exist" in your gist entry, the screenshot below indicates that your evaluation failed. You need to troubleshoot it.

- When you troubleshoot, observe your CPU utilization; if it reaches 100%, see the screenshot below; it usually indicates the existing pod type is insufficient to handle the workload; you need a more powerful pod. Visit RunPod's GPU Cloud page and pick a more fitting pod. Your existing execution will most likely fail without a trace. You will need to enter your new GPU pod type in your AutoEval notebook and re-execute your evaluation.

- You do have the option to turn on "debug" in the AutoEval notebook; see the screenshot below. However, be aware that you need to manually terminate your pod after your execution when you have "debug" turned on. If you don't terminate your pod manually, it will continue running until it drains your account. So be cautious if you have to turn on "debug".

A Word on Costs
The costs of running evaluations in RunPod are dependent on the pod type you select. Just to give you an idea of how much it costs to run the evaluations for our models:
- Evaluation for the base model took 11 hours and 26 minutes on Tesla V100-SXM2–32GB, costing a little less than USD$5.00. This particular instance was discontinued by RunPod just in the last few days.
- Evaluation for the merged model took 2 hours and 12 minutes on NVIDIA RTX A5000, costing less than USD$1.00.

AutoEval Results
Our evaluations for the base model Mistral-7B-Instruct-v0.2
and the merged model MistralTrinity-7B-slerp
succeeded. The eval results were uploaded to my GitHub gists, [here](https://gist.github.com/wenqiglantz/df944610e352c38189c786c4eaaaae86) and here. Due to the length of the results, we are only listing the summaries below:


As we can see, the merged MistralTrinity-7B-slerp
outperformed the base Mistral-7B-Instruct-v0.2
on the AGIEval, GPT4ALL, and BigBench, resulting in a higher average score of 56.82. The base model held a slight advantage on the TruthfulQA task.
Fine-Tuning the Merged Model
We are happy with the merged model. Let's push one step further. How about fine-tuning the merged model to see if we can harvest even better performance?
Training Dataset
The key to fine-tuning a model is finding a good training dataset. As recommended by Maxime, Hugging Face's databricks/databricks-dolly-15k
is a good one. It is an open-source instruction dataset of 15k records generated by thousands of Databricks employees.
We first need to identify the instruction format of our base model. Per the model card for Mistral-7B-Instruct-v0.2
, the instruction needs to be in the following format:
text = "[INST] What is your favourite condiment? [/INST]"
"Well, I'm quite partial to a good squeeze of fresh lemon juice. It adds just the right amount of zesty flavour to whatever I'm cooking up in the kitchen! "
"[INST] Do you have mayonnaise recipes? [/INST]"
The databricks dataset has a different format:

So, we first need to convert the databricks dataset into the desired instruction format our base model can consume. Let's launch a notebook to do the conversion.
We first load the Hugging Face dataset databricks/databricks-dolly-15k
. We shuffle and slice it to take only 1000 records (enough for fine-tuning), transforming them into an instruction dataset to fine-tune our base model. See the code snippet below.
from datasets import load_dataset
import re, json
# Load the dataset
dataset = load_dataset('databricks/databricks-dolly-15k')
# Shuffle the dataset and slice it
dataset = dataset['train'].shuffle(seed=42).select(range(1000))
print(len(dataset))
# Define a function to transform the data
def transform_conversation(example):
# Directly access the data from the example
instruction = example.get('instruction', '')
response = example.get('response', '')
# Convert the input data to the desired format
formatted_output = f"[INST] {instruction} [/INST] {response}n"
return {'text': ''.join(formatted_output)}
# Apply the transformation
transformed_dataset = dataset.map(transform_conversation)
We then push the converted dataset to the Hugging Face hub so we can use it for our fine-tuning step below.
!pip install -q huggingface_hub
from huggingface_hub import create_repo , HfApi
from google.colab import userdata
username = "wenqiglantz" # change to your username
dataset_name = "databricks-dolly-1k"
# Defined in the secrets tab in Google Colab
api = HfApi(token=userdata.get("HF_TOKEN"))
# Create empty dataset repo
api.create_repo(
repo_id = f"{username}/{dataset_name}",
repo_type="dataset", # be sure to use dataset here, not model
exist_ok=True,
)
transformed_dataset.push_to_hub(f"{username}/{dataset_name}")
Supervised Fine-tuning with QLoRA
Now, we are ready to fine-tune our merged model with the newly converted databricks dataset. Check out the fine-tuning notebook for the detailed steps.
We use the parameter-efficient fine-tuning (PEFT) technique QLoRA, Quantized Low-Rank Adapter. It combines a high-precision computing technique with a low-precision storage method. This helps keep the model size small while still making sure the model is highly performant and accurate.
We load the merged model MistralTrinity-7B-slerp
directly in 4-bit precision using the NF4 type and train it for 1 epoch using the databricks dataset.
################################################################################
# bitsandbytes parameters
################################################################################
# Activate 4-bit precision base model loading
use_4bit = True
# Compute dtype for 4-bit base models
bnb_4bit_compute_dtype = "float16"
# Quantization type (fp4 or nf4)
bnb_4bit_quant_type = "nf4"
# Activate nested quantization for 4-bit base models (double quantization)
use_nested_quant = False
...
# Load base model
model = AutoModelForCausalLM.from_pretrained(
model_name,
quantization_config=bnb_config,
device_map=device_map
)
print(model)
model.config.use_cache = False
model.config.pretraining_tp = 1
# Load Mistral tokenizer
tokenizer = AutoTokenizer.from_pretrained(model_name, trust_remote_code=True)
tokenizer.pad_token = tokenizer.eos_token
tokenizer.padding_side = "right" # Fix weird overflow issue with fp16 training
# Load LoRA configuration
peft_config = LoraConfig(
lora_alpha=lora_alpha,
lora_dropout=lora_dropout,
r=lora_r,
bias="none",
task_type="CAUSAL_LM",
target_modules=[
"q_proj",
"k_proj",
"v_proj",
"o_proj",
],
)
...
We train it for 1 epoch, with 250 steps:

The TensorBoard showed a pretty good training loss curve going downward, although that zigzag is not ideal:

AutoEval Supervised Fine-tuned Model
Let's now run AutoEval notebook to find out how the fine-tuned model MistralTrinity-7B-slerp-finetuned-dolly-1k
performed compared to the merged model MistralTrinity-7B-slerp
and the base model. See below the results.

Yikes! Not ideal. I was hoping for better performance by the fine-tuned model. But clearly, its performance is inferior to the merged model in all categories. Although it outperformed the base model in AGIEval, GPT4ALL, and BigBench. It appears that fine-tuning has no positive impact on the merged model.
I was intrigued, so I reached out to Maxime and was informed that supervised fine-tuning doesn't perform very well with the merged models because the merged models have already been fine-tuned. He recommends using DPO (Direct Preference Optimization) instead, which doesn't suffer from this issue. Check out his article for more details on DPO and how to apply it in fine-tuning a model: Fine-tune a Mistral-7b model with Direct Preference Optimization.
Fine-tuning with DPO
Let's press on and experiment with fine-tuning our merged model with DPO.
Traditionally, aligning LLMs with human preferences involved reinforcement learning (RL), a complex and resource-intensive process. DPO takes a different route. It directly optimizes the LLM based on a dataset of explicit human preferences. This dataset often consists of prompts alongside preferred and dispreferred responses. Using this information, DPO fine-tunes the LLM to favor the preferred responses and discourage the dispreferred ones.
DPO is a faster and more elegant way to tune your model than the traditional RLHF way. It drops the reward model and instead uses the preference data directly to fine-tune the model.
Andrew Ng, the founder of DeepLearning.ai, wanted to give the authors of the paper on DPO a "standing ovation" after finishing reading their paper.
Gathering a good set of preference dataset is the most challenging part of DPO fine-tuning. Luckily, Maxime provided his sample preference dataset mlabonne/chatml_dpo_pairs, which we can use for our experiment.
Following Maxime's instructions, we add all DPO fine-tuning steps for our merged model in this Colab notebook:
- Load the preference dataset.
- Define
LoraConfig
. - Load our merged model and a reference model which is the same as the merged model, a step required by DPO training.
- Invoke
DPOTrainer
to train our merged model.
You will have to have Colab Pro+ plan to get access to A100 for this task. DPO fine-tuning took a little over an hour. The details of the run can be found from my Weights & Biases project. The train/loss curve dropped and pretty much plateaued after step 50, interesting.
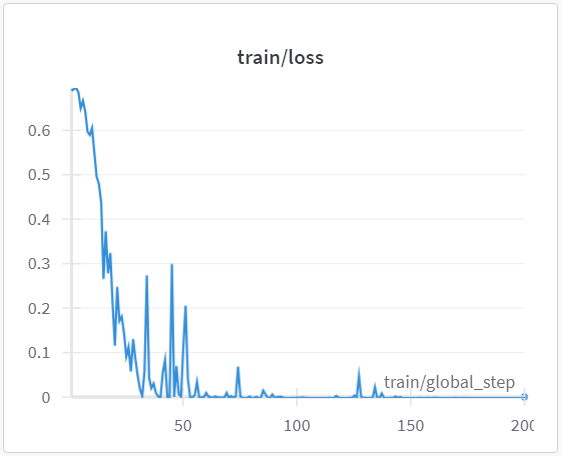
AutoEval Result for DPO Fine-tuned Model
The evaluation of our DPO fine-tuned merged model completed after 2 hours 40 minutes on NVIDIA A5000 in RunPod. The eval result can be found in my GitHub gist. How did it do? Let's compare:

MistralTrinity-7B-slerp-dpo
is the clear winner! With the highest eval scores for all eval tasks, and an average score of 57.89, over 1% above the merged model MistralTrinity-7B-slerp
, and 3% above the base model Mistral-7B-Instruct-v0.2
! Truly impressive. DPO did its magic!
Model Family Tree
Maxime released yet another cool tool, model family tree, and it's also available on his Hugging Face Space. Let's use his notebook to check the family tree for our fine-tuned merged model:

And, here is the beautiful family tree for our merged model MistralTrinity-7B-slerp-dpo
:

Summary
We explored model merge, model evaluation, and model fine-tuning techniques in this article. We applied two tools and multiple notebooks from Maxime's llm-course GitHub repo: LazyMergekit, LLM AutoEval, SFT with QLoRA, and fine-tuning with DPO. We conclude that:
- Our merged model
MistralTrinity-7b-slerp
outperformed its base model on the AGIEval, GPT4ALL, and BigBench tasks, resulting in a higher average score of 56.82, nearly 2 percent higher than the base model. - Supervised fine-tuning on the merged model brought no positive performance gain as the merged model using SLERP merge method has already been supervised fine-tuned.
- Fine-tuning the merged model with DPO revealed the highest eval scores for all AutoEval tasks, with an average score of 57.89, over 1% above the merged model, and 3% above the base model.
It is fascinating to see how much we can manipulate the language models to get the best performance out of them. This is indeed an exciting exploration! The possibility of open-source models surpassing proprietary models is growing exponentially!
See below a list of the notebooks we used in this article. They are also located in my GitHub repo:
- Model merge Colab notebook
- Dataset Colab notebook
- Supervised Fine-tuning Colab notebook
- Fine-tuning with DPO Colab notebook
- AutoEval Colab notebook
Happy coding!
References:
- Merge Large Language Models with mergekit
- mergekit GitHub Repo
- llm-autoeval GitHub Repo
- AGIEval GitHub Repo
- databricks-dolly-15k Hugging Face dataset
- Mistral-7B-Instruct-v0.2 Hugging Face model card
- Fine-Tune Your Own Llama 2 Model in a Colab Notebook
- Fine-tune Llama 2 in Google Colab
- QLoRA Fine-Tuning from mercity.ai