Exploring the Latest Advances in Foundation Time-series Models

Join AI Horizon Forecast, a blog making complex AI topics as clear as daylight.
Recent advances in foundation time-series models are groundbreaking.
TimeGPT was the first native foundation model. It was released this past August and shook the forecasting community.
Since then, numerous other foundation models have been released, including:
- TimesFM
- MOIRAI
- Tiny Time Mixers (TTM)
- MOMENT
We've covered these models in previous articles, but they've had many updates since their initial release.
In this article, we'll explore these updates – which include new benchmarks and improved model variants.
Let's get started.
TimesFM – Google's Foundation model [1]
New updates: The model weights were recently released on Hugging Face! You can find a project tutorial for TimesFM on the AI Projects folder!
Google entered the race of foundation models with TimesFM, a 200 million parameter model.
The problem with building large time-series models is scarcity of data. It's challenging to find good and diverse publicly available time-series data.
Fortunately, the TimesFM team expanded their training dataset using sources like Google Trends and WikiPage views. The final model was pretrained on 100B billion real-world time points.
Architecturally, TimesFM is Transformer-based model that leverages scale to perform time-series forecasting (Figure 1):

The secret sauce of TimesFM is the usage of patching (which is very beneficial for language models) with the decoder-only style of Generative Pretrained models.
But how does patching work?
Just as text models predict the next word, time-series foundation models predict the next patch of time-points.
Patching is helpful – because it treats a window of time points as a token, creating rich representations with local temporal information. This helps TimesFM capture temporal dynamics more effectively, resulting in more accurate forecasts (Figure 2):

When I first wrote about TimesFM, Google didn't make any promises about open-sourcing the model or its weights. Instead, they stated they would make their model available in Vertex AI (probably via an API client like Nixtla did with TimeGPT)
Fortunately, the authors released the model weights and inference code for the univariate case. They also plan to release newer model variants with an extended API for fine-tuning.
Let's hope they also release the pretraining dataset. Stay tuned!
MOIRAI – Salesforce's Foundation Model [2]
New updates: Salesforce open-sourced the model, the weights, the pretraining dataset and released a new model variant!
You can find a hands-on tutorial of MOIRAI on on the AI Projects folder!
Salesforce released MOIRAI around the same time as TimesFM. MOIRAI stands out due to its unique transformer-encoder architecture – designed to handle the heterogeneity and complexity of time-series data.
Key features of MOIRAI include:
- Multi-Patch Layers: MOIRAI adapts to multiple frequencies by learning a different patch size for each frequency.
- Any-variate Attention: An elegant attention mechanism that respects permutation variance between each variate, and captures the temporal dynamics between datapoints.
- Mixture of parametric distributions: MOIRAI optimizes for learning a mixture of distributions – rather than hypothesizing a single one.

Compared to TimesFM, MOIRAI introduces many novel features for time series. It modifies the traditional attention mechanism (Any-variate Attention) and considers different time-series frequencies.
However, the effectiveness of MOIRAI (and every foundation model) lies in the pretraining dataset. MOIRAI was pretrained on LOTSA, a massive collection of 27 billion observations across nine domains. This dataset was also released.
This extensive dataset, combined with the model's innovative architecture, makes MOIRAI ideal as a zero-shot forecaster – able to predict unseen data quickly and accurately.
Figures 4 and 5 display MOIRAI-large on the task of day-ahead energy forecasting (from the MOIRAI tutorial of the AI Projects folder):

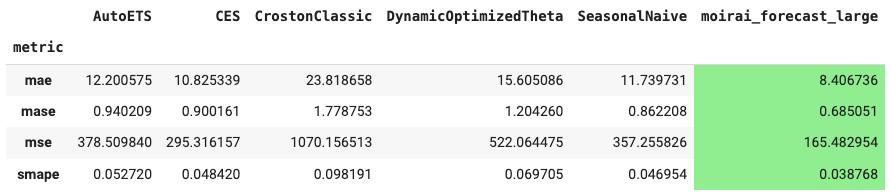
Finally, a strong point of MOIRAI is its capability for multivariate forecasting. We can add past observed covariates or future known inputs (e.g., holidays). This makes MOIRAI ideal for time-series cases that can be enhanced with external information (such as trading, energy demand forecasting, etc.).
Tiny Time Mixers (TTM)— A foundation model by IBM Researchers[3]
New updates: The authors initially open-sourced a fast version, TTM-Q. A few months later they updated the paper, describing better model variants with new features (e.g. explainability) – which they will open-source as well.
You can find a hands-on project of TTM-Q (zero-shotting and finetuning)on the AI Projects folder!
TTM is a unique model, following a different approach from the models mentioned above:
It's not a Transformer model!
Also, it's lightweight and beats other larger foundation models. The main characteristics of TTM are:
- Non-Transformer Architecture: TTM is extremely fast as it uses fully connected NN layers – instead of the Attention mechanism.
- TSMixer Foundation: TTM leverages TSMixer (IBM's breakthrough time-series model) in its architecture.
- Rich Inputs: TTM can handle multivariate forecasting, accepting extra channels, exogenous variables, and known future inputs.
- Fast and Powerful: TTM-quick variant was pretrained on 244M samples of the Monash dataset, using 6 A100 GPUs in less than 8 hours.
Figure 6 shows the top-level view of TTM's architecture:

There are 2 phases, **** pretraining and finetuning:
In the pretraining phase, the model is trained with univariate time series only – leveraging historical information and local seasonal patterns.
In the finetuning phase, the model ingests multivariate data and learns their interdependencies – by enabling the channel mixer process (Figure 6).
During finetuning, the core layers remain frozen – making the process lightweight. The model can optionally use future known covariates by activating the Exogenous Mixer (Figure 7) for extra performance.

Additionally, the authors created new model variants with different parameter sizes, context lengths (sl), and ** forecasting lengths (fl)**:
- TTM-Base (TTM_B): 1M parameters, sl = 512, pl = 64
- TTM-Enhanced (TTM_E): 4M parameters, sl = 1024, pl = 128
- TTM-Advanced (TTM_A): 5M parameters, sl = 1536 and pl = 128
- Quick-TTM (TTMQ): There are 2 variants here: sl/pl = (512,96) and (1024,96)
The authors show these models perform even better in benchmarks. Check my original TTM article for more info.
In the final iteration, the authors addressed explainability. The new variants can provide feature importance (Figure 8):

In general, TTM is an outstanding model. Its approach of not using heavy Transformer operations opens the door to many interesting possibilities. The new variants have not been released yet – but stay tuned as we will benchmark them!
MOMENT [4]
New updates: The authors open-sourced the largest variant, MOMENT-large, and the pretraining dataset, Time-Series Pile
Unlike previous models, MOMENT functions as a general-purpose time-series model. It can handle forecasting, classification, anomaly detection, and imputation tasks.
MOMENT improves upon GPT4TS and TimesNet – 2 models also designed for multiple time-series tasks. Here are the key characteristics of MOMENT:
- LLM-based: Utilizes T5 for five time-series tasks.
- Lightweight execution: Suitable for faster execution with limited resources.
- Zero-shot forecasting: Excels in zero-shot scenarios and can be fine-tuned for better performance.
Like the aforementioned models, MOMENT uses patching.
Here, MOMENT treats sub-sequences of timepoints as tokens – improving inference speed. During pretraining, MOMENT normalizes time points and patches them into embeddings. These embeddings are processed to reconstruct the original time points.
Hence, the pretraining process resembles how BERT is trained (masked language modeling). Parts of the input time series are randomly masked and the model is trained to optimally reconstruct them (Figure 9):

Three model variants were pretrained, using the T5 encoder: T5-Small (40M), T5-Base (125M), and T5-Large (385M). There were pretrained on diverse datasets (the Time-Series Pile), allowing the model to generalize well on unseen data.
How to use MOMENT
Additionally, MOMENT can be used as a zero-shot predictor or finetuned for better performance.
MOMENT-LP, a class of finetuned MOMENT models for specific tasks, has shown promising results in benchmarks – often outperforming larger and more complex models.
So far, the authors have released the largest finetuned version, MOMENT-Large, which achieves the best results on the paper's benchmarks.
At the time of writing, the pretraining and finetuning code have not been released, but the authors plan to release them soon.
MOMENT can be described as a versatile, lightweight time-series model, ideal for multi-objective scenarios. Its performance, especially in resource-constrained environments, makes it a valuable tool in time-series analysis.
Closing Remarks
Foundation NLP models have sparked an interest in LLMs in time-series forecasting.
TimeGPT was released less than a year ago – and major companies and researchers are already investing time and effort in developing these models.
Foundation time-series models will have a significant impact on practical applications. Time series are used in many domains like retail, energy demand, economics, and healthcare. A foundation TS model can be applied to any TS case with great accuracy, similar to how GPT-4 is used for text.
Of course, there is still room for improvement – which is why every model examined in this article is constantly updated.
But the best news so far is that all the aforementioned models are open-source – paving the way for further interest and democratizing research in this field.
Thank you for reading!
- Follow me on Linkedin!
- Subscribe to my newsletter, AI Horizon Forecast!
References
[1] Das et al., A decoder-only foundation model for time-series forecasting __ [2023]
[2] Woo et al., Unified Training of Universal Time Series Forecasting Transformers (February 2024)
[3] Ekambaram et al., Tiny Time Mixers (TTMs): Fast Pre-trained Models for Enhanced Zero/Few-Shot Forecasting of Multivariate Time Series (April 2024)
[4] Goswami et al., MOMENT: A Family of Open Time-Series Foundation Models (February 2024)