Fast and Scalable Hyperparameter Tuning and Cross-validation in AWS SageMaker

This article shares a recipe to speeding up to 60% your ** Hyperparameter Tuning with cross-validation in SageMaker Pipelines leveraging SageMaker Managed Warm Pools. By using Warm Pools, the runtime of a Tuning step with 120 sequential jobs is reduced from 10h to 4**h.
Improving and evaluating the performance of a machine learning model often requires a variety of ingredients. Hyperparameter tuning and cross-validation are 2 such ingredients. The first finds the best version of a model, while the second estimates how a model will generalize to unseen data. These steps, combined, introduce computing challenges as they require training and validating a model multiple times, in parallel and/or in sequence.
What this article is about…
- What are Warm Pools and how to leverage them to speed-up hyperparameter tuning with cross-validation.
- How to design a production-grade SageMaker Pipeline that includes Processing, Tuning, Training, and Lambda steps.
We will consider Bayesian optimization for hyperparameter tuning that leverages the scores of the hyperparameter combinations already tested to choose the hyperparameter set to test in the next round. We will use k-fold cross-validation to score each combination of hyperparameters, in which the splits are as follows:
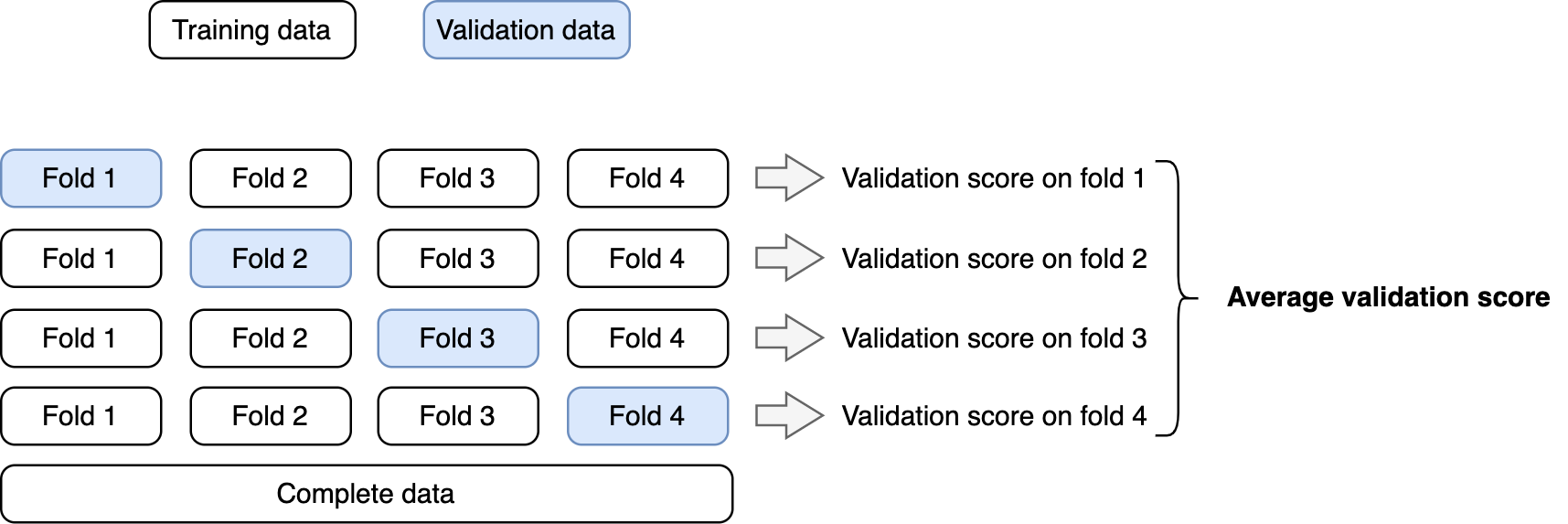