From RAGs to Riches

As large language models (LLMs) have eaten the world, Vector Search engines have tagged along for the ride. Vector databases form the foundation of the long-term memory systems for LLMs.
By efficiently finding relevant information to pass in as context to the language model, vector search engines can provide up-to-date information beyond the training cutoff and enhance the quality of the model's output without fine-tuning. This process, commonly referred to as retrieval augmented generation (RAG), has thrust the once-esoteric algorithmic challenge of approximate nearest neighbor (ANN) search into the spotlight!
Amidst all of the commotion, one could be forgiven for thinking that vector search engines are inextricably linked to large language models. But there's so much more to the story. Vector search has a plethora of powerful applications that go well beyond improving RAG for LLMs!
In this article, I will show you ten of my favorite uses of vector search for data understanding, data exploration, model interpretability and more.
Here are the applications we will cover, in roughly increasing order of complexity:
- Image Similarity Search
- Reverse Image Search
- Object Similarity Search
- Robust OCR Document Search
- Semantic Search
- Cross-modal Retrieval
- Probing Perceptual Similarity
- Comparing Model Representations
- Concept Interpolation
- Concept Space Traversal
Image Similarity Search

Perhaps the simplest place to start is image similarity search. In this task, you have a dataset consisting of images – this can be anything from a personal photo album to a massive repository of billions of images captured by thousands of distributed cameras over the course of years.
The setup is simple: compute embeddings for every image in this dataset, and generate a vector index out of these embedding vectors. After this initial batch of computation, no further inference is required. A great way to explore the structure of your dataset is to select an image from the dataset and query the vector index for the k
nearest neighbors – the most similar images. This can provide an intuitive sense for how densely the space of images is populated around query images.
For more information and working code, see here.
Reverse Image Search

In a similar vein, a natural extension of image similarity search is to find the most similar images within the dataset to an external image. This can be an image from your local filesystem, or an image from the internet!
To perform a reverse image search, you create the vector index for the dataset as in the image similarity search example. The difference comes at run-time, when you compute the embedding for the query image, and then query the vector database with this vector.
For more information and working code, see here.
Object Similarity Search

If you want to delve deeper into the content within the images, then object, or "patch" similarity search may be what you're after. One example of this is person re-identification, where you have a single image with a person of interest in it, and you want to find all instances of that person across your dataset.
The person may only take up small portions of each image, so the embeddings for the entire images they are in might depend strongly on the other content in these images. For instance, there might be multiple people in an image.
A better solution is to treat each object detection patch as if it were a separate entity and compute an embedding for each. Then, create a vector index with these patch embeddings, and run a similarity search against a patch of the person you want to re-identify. As a starting point you may want to try using a ResNet model.
Two subtleties here:
- In the vector index, you need to store metadata that maps each patch back to its corresponding image in the dataset.
- You will need to run an object detection model to generate these detection patches before instantiating the index. You may also want to only compute patch embeddings for certain classes of objects, like
person
, and not others –chair
,table
, etc.
For more information and working code, see here.
Robust OCR Document Search

Optical Character Recognition (OCR) is a technique that allows you to digitize documents like handwritten notes, old journal articles, medical records, and those love letters squirreled away in your closet. OCR engines like Tesseract and PaddleOCR work by identifying individual characters and symbols in images and creating contiguous "blocks" of text – think paragraphs.
Once you have this text, you can then perform traditional natural language keyword searches over the predicted blocks of text, as illustrated here. However, this method of search is susceptible to single-character errors. If the OCR engine accidentally recognizes an "l" as a "1", a keyword search for "control" would fail (how about that irony!).
We can overcome this challenge using vector search! Embed the blocks of text using a text embedding model like GTE-base from Hugging Face's Sentence Transformers library, and create a vector index. We can then perform fuzzy and/or semantic search across our digitized documents by embedding the search text and querying the index. At a high level, the blocks of text within these documents are analogous to the object detection patches in object similarity searches!
For more information and working code, see here.
Semantic Search
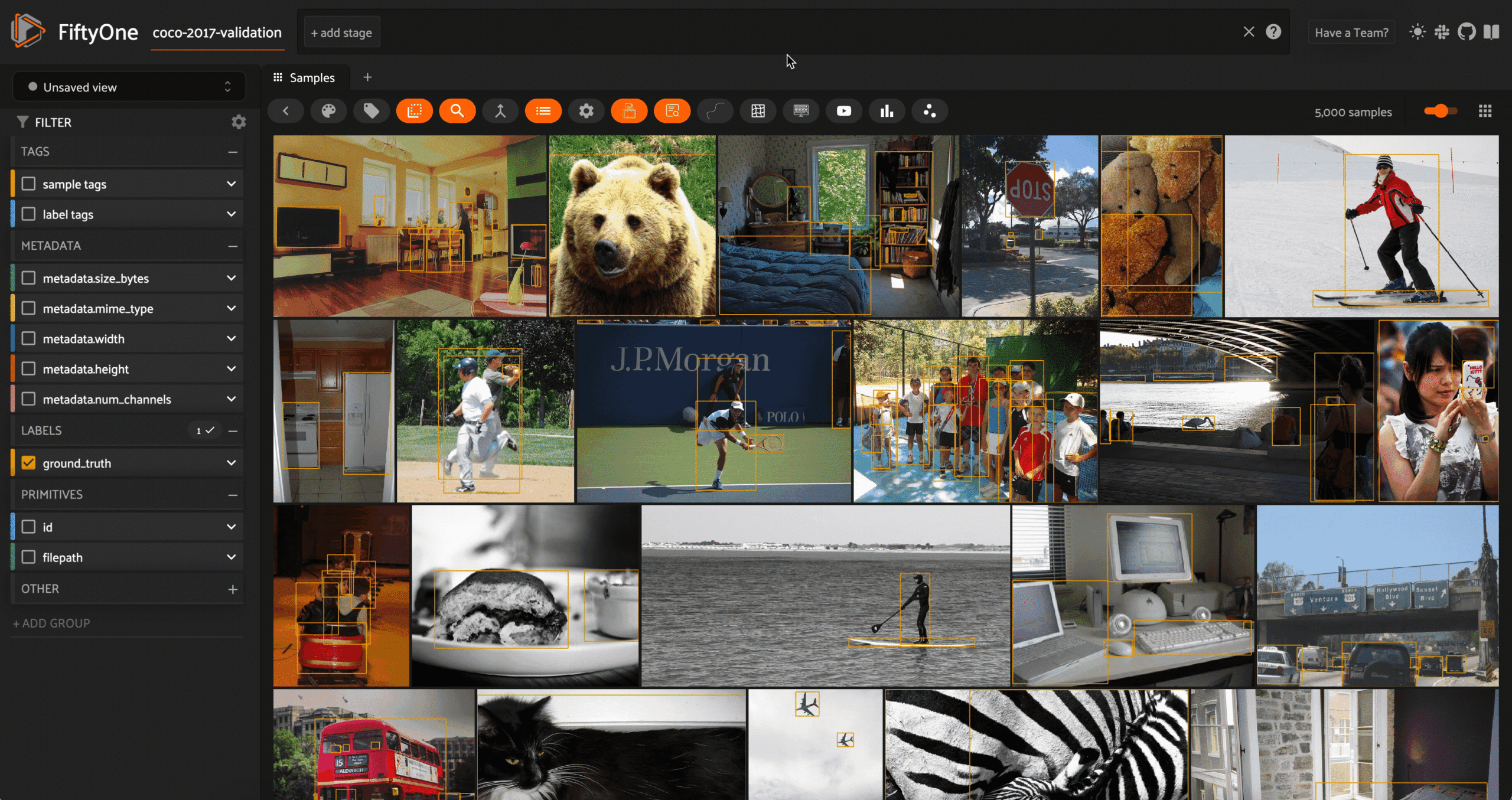
With multimodal models, we can extend the notion of semantic search from text to images. Models like CLIP, OpenCLIP, and MetaCLIP were trained to find common representations of images and their captions, so that the embedding vector for an image of a dog would be very similar to the embedding vector for the text prompt "a photo of a dog".
This means that it is sensible (i.e. we are "allowed") to create a vector index out of the CLIP embeddings for the images in our dataset and then run a vector search query against this vector database where the query vector is the CLIP embedding of a text prompt.