How is Causal Inference Different in Academia and Industry?

In two months, we finished reading "The Book of Why," which gave us a glimpse into the fascinating world of causality. As promised, I have a bonus article to close my first Read with Me series officially.
Inspired by my own background as an academic researcher who studied Causal Inference in economics during my Ph.D. program, as well as my experience as a data scientist in the industry building causal models to make demand forecasts, for the bonus article, I would like to share my understanding of the concept of causal inference and the similarities and differences in how it is applied in academic and industry settings.
Differences
Due to the difference in the nature and purpose of academic research and industry applications, the causal inference workflows are quite different between the two.
Speed
Academic research usually operates at a slower pace, from forming ideas to drawing final conclusions. It focuses on building trust not only on the causal conclusion itself but also on the data involved, methods used, and robustness of the research. Thus, oftentimes, the research process is extended to validate data eligibility, run sensitivity analysis, test causal structures, etc.
However, for business, time is money. Tech firms are more practical. They would rather focus their resources on building scalable applications that can be put into production and bring benefits quickly. The cost of waiting for a perfect and generalizable model is high. Thus, the industry would prefer to have a benchmark model available as a placeholder first before fine-tuning and making adjustments.
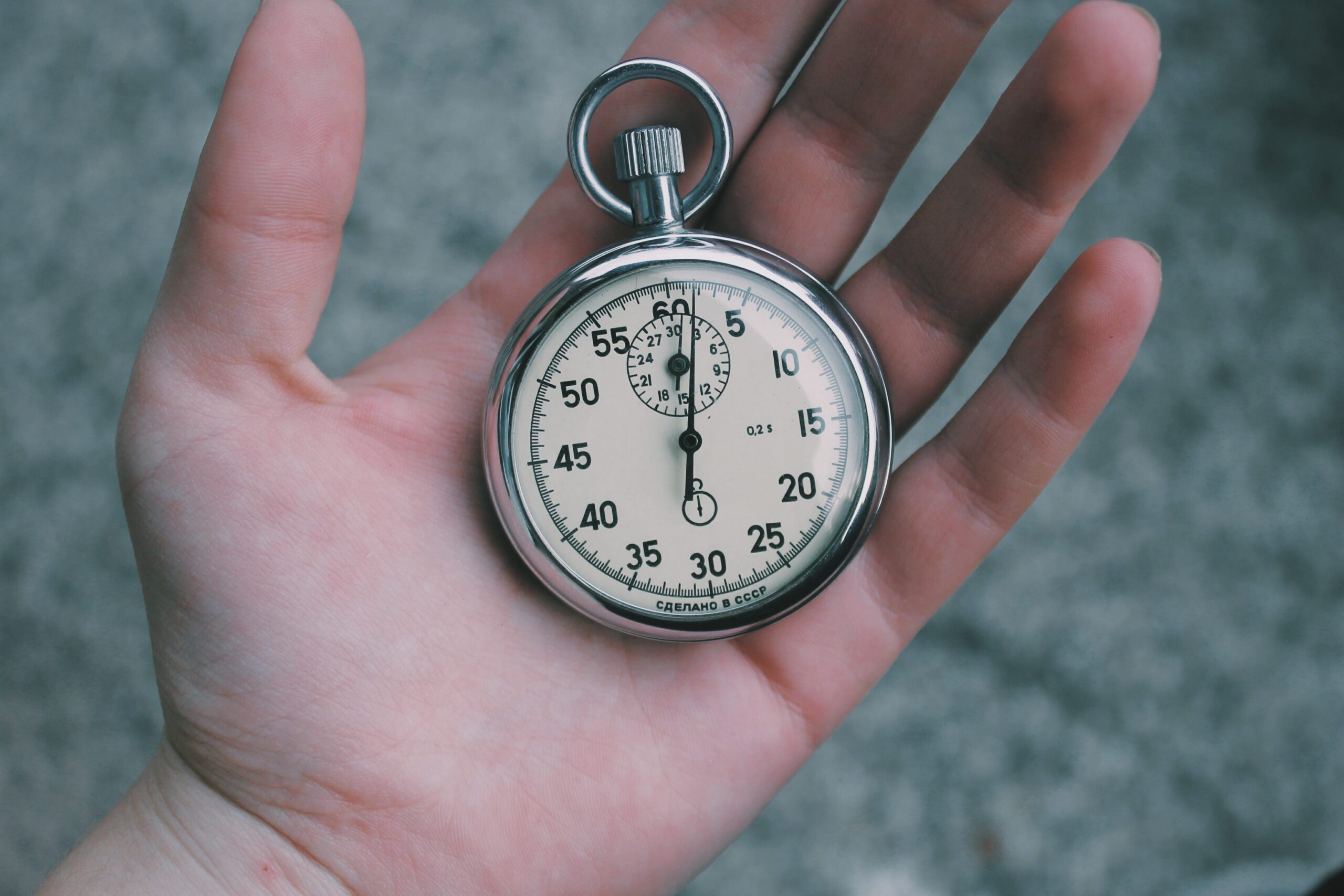
Method
Indeed, academic research is the source of new approaches and mechanisms for theoretical researchers. However, empirical researchers who focus on observational studies or experiments tend to use standard and well-established methodologies. For example, Difference-in-Differences (DID), Instrument Variables (IV), Propensity Score Matching (PSM), etc. Using these widely-recognized methods will attract fewer questions and doubts about the conclusions.
Industries are always open to cutting-edge techniques. The ones with the buzzwords are more acceptable here. A model that works decent and involves the latest and hottest concepts attracts stakeholders' attention, although building bridges between business impacts and technical terms is also very important.
Feedback Loop
When researchers have some initial results, they will attend conferences or other events to network their findings to gather feedback. Publishing a research paper involves enormous amounts of iterations based on feedback from experts and editors.
When tech companies have a causal model with decent performance, they will launch this application into their business decision-making. Their feedback comes from real-time customer reactions to the model or KPIs for a given time period. For example, a company finds a product that is price elastic and decides to drop the price to increase profits. If later it turns out the company's profit actually drops after the price cut, then they would have to reexamine the model.
Average Treatment Effect (ATE) vs. Individual Treatment Effect (ITE)
In academics, it is often the case to evaluate ATE for a population or subpopulation. It is the result of the purpose of academic research as well as the limitations on data availability.
On one hand, academic research aims to inform general policy-making or derive generalizable and trustworthy theories. On the other hand, researchers are often forced to do analysis on more macro-level data – a country's demographic information, a race's income level, a school's test scores, etc.
On the contrary, industrial causal applications are more focused on individual-level decision-making. Thus, ITE is more important. For example, if I send out an email promotion for this customer, will he or she make the purchase or stay with the current subscription?
The individual-level Causality is more meaningful to them because the cost to tailor business decisions at the customer level is minimal, but the gain in profit is huge. Plus, the industry possesses a tremendous amount of data for each customer at each transaction to derive decision-making at a finer granular level.
If you are interested in knowing the difference between ATE and ITE in definitions and mathematic terms, check out this article:
Model Efficiency
Industry applications sometimes need to be put into production to make real-time business decisions. For example, when a customer clicks "cancel subscription," the model needs to make immediate decisions on whether to offer discounts and, if so, how much.
Thus, we need to consider the run time of the model and its scalability when embedding a causal engine into business decision-making, which is less of a concern for academic research.
The Ending
It seems that academic research can go on forever. Even after the research paper has been published, we oftentimes see one paper become a series of research papers by testing causality in different populations or time periods. Research is the process of extending the knowledge frontiers so that any learning along the way can be meaningful.

On the other hand, industrial applications focus on the key KPI improvements. If an uplift model that predicts customer behaviors increases retention rate and profits, then the model will be launched and maintained.
Similarities
Despite the differences, there are also many similarities that academic research and industry share.

Assumptions
The world is not perfect. Thus, both research and industry applications start with assumptions. For example, the relationship between variable X and Y is linear; the unobserved confounder U can be proxied by variable W; variable Z would be a great instrument variable given this causal structure, etc.
We start from somewhere with a set of assumptions and validate whether having each one of them makes sense or whether one of them is causing issues in the conclusions.
Expert Input
Both research and industry applications need expert input to draft the causal structure. Domain knowledge is very important to identify confounders, mediators, proxies for unobserved confounders, instrument variables, etc.
A causal graph cannot be drawn without understanding the underlying mechanism, and hopefully, after the two-month reading series, you will understand the importance of a causal graph.
Transparency
Both research and industry applications embrace transparency when including causality. Just as Pearl mentioned in the last chapter of "The Book of Why", there are many algorithms that perform well in solving a problem, but embedding causality into the systems gives us transparency. Just as @Hoang Pham said in the comment on the first article of this reading series:
"…I always try to find out "Why does it happen?" or "Why do our users have such behavior", so that I can provide treatments for different groups of users based on their needs and our value proposition…"
Understanding why is extremely powerful not only in business decision-making but also in understanding how the world works and how the mechanism is implemented.
Although differences exist due to the data availability and the purpose of the analysis, ultimately, academic research and industry application progress simultaneously through gathering inspiration from each other, just as I demonstrate in the following article:
After 12 weeks of effort, we finally finished the first Read with Me series, reading Judea Pearl's masterpiece, "The Book of Why." I have received many feedback and really enjoyed this reading journey. Although it is a stressful process to keep the updates on time, enforcing output by writing summaries for the chapters helps me complete the learning cycle and pushes me to understand the concepts fully.


Thank you all for signing up my email list to join and provide meaningful feedback. I hope this is also a fruitful journey for you as well, no matter you are reading the book with me, or learning causality through my article summaries. If there are any other books you want to read with a crowd of data enthusiasts and gather collective learning experience, let me know!
If you like this article, don't forget to:
- Check my recent articles about the 4Ds in data storytelling: making art out of science; continuous learning in data science; how I become a data scientist;
- Check my other articles on different topics like data science interview preparation;
- Subscribe to my email list;
- Sign up for medium membership;
- Or follow me on YouTube and watch my most recent YouTube videos: