How to Perform Hyperparameter Tuning in R with Python
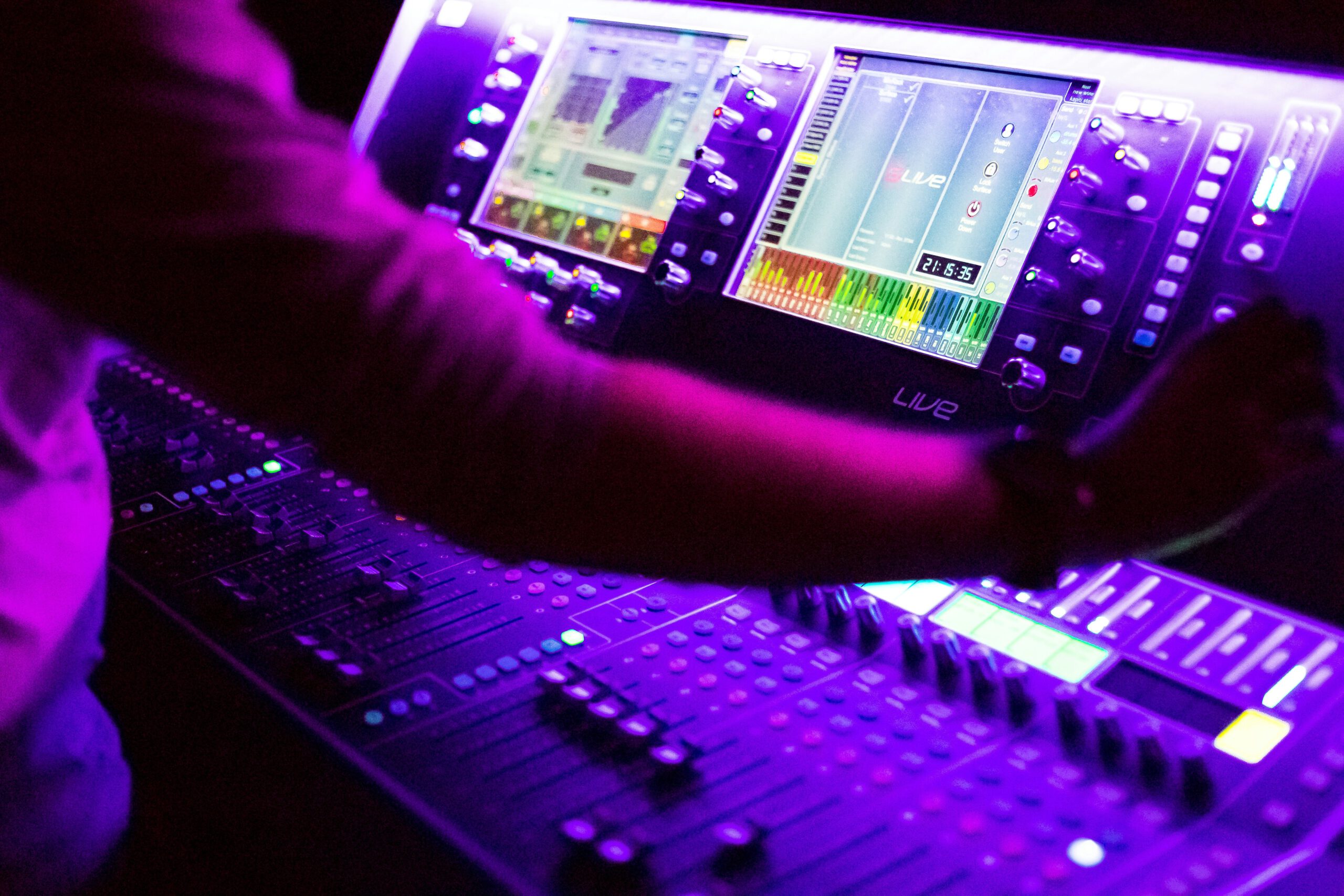
Introduction
Data Science and AI professionals usually spend a significant amount of time gathering data, cleaning it, preparing it, and choosing the perfect algorithm while building a machine learning model to generate predictions. However, the model performance does not always seem to meet the desired expectations. This happens because an important step has not been covered after setting up the baseline model. Yes, you are thinking correctly – tuning the hyperparameters which are the settings that guide our model to learn and make better predictions. Sometimes, even after using powerful machine learning algorithms, the model cannot perform well as its hyperparameters are not fine-tuned. However, manually searching for the best set of hyperparameters and applying them can be boring as well as time-consuming. So what is Hyperparameter Tuning, and why is it important to know when developing ML models?
Why Hyperparameter Tuning Matters in Machine Learning
Hyperparameter tuning improves the performance of machine learning models. Finding the best settings for the model ensures that it learns from the data in the most effective way. This, in turn, increases the accuracy of prediction and makes the model more reliable as well as useful in real-world situations.
A wide variety of domains, such as healthcare, banking, finance, agriculture, etc., can really benefit from highly accurate models. On the other hand, poorly properly tuned ML models may not perform well and can lead to inaccurate predictions. With such outcomes, businesses and organizations can likely end up making potentially expensive errors in decision-making. Additionally, well-tuned models can generalize better to new, unseen data, thereby reducing the risk of overfitting and improving robustness.
Why Do R Users Need Python for Hyperparameter Tuning?
For hyperparameter tuning, some Python libraries tend to perform better than those available in R, particularly for advanced deep-learning models and large-scale optimization.
Let's see some key features of the packages in both languages for hyperparameter tuning requirements.
Python libraries like Optuna, hyperopt, and scikit-learn for hyperparameter tuning perform well, particularly for deep learning and large-scale optimization requirements. These libraries also integrate well with TensorFlow and Keras, handle complex hyperparameter spaces efficiently, and use pruning to save time and resources.
R packages such as caret, mlr3, tune, GA, and ecr also provide strong tools for hyperparameter tuning. They support automated methods like grid and random search, Bayesian optimization, and evolutionary algorithms and include pruning to improve model performance.
Considering the above, we can say that although R packages like GA, ecr, and caret could be a good choice for hyperparameter optimization, Optuna offers advanced features such as dynamic search spaces and pruning strategies, making it highly efficient for complex models and large datasets. Additionally, Optuna has a user-friendly API that can be easily integrated with Python's deep learning libraries, making it more flexible and easy to use.
For optimizing model performance and improving accuracy, automated hyperparameter optimization tools like Optuna from Python can help find the best hyperparameter combinations to maximize model performance.
Why should you use Optuna in R?
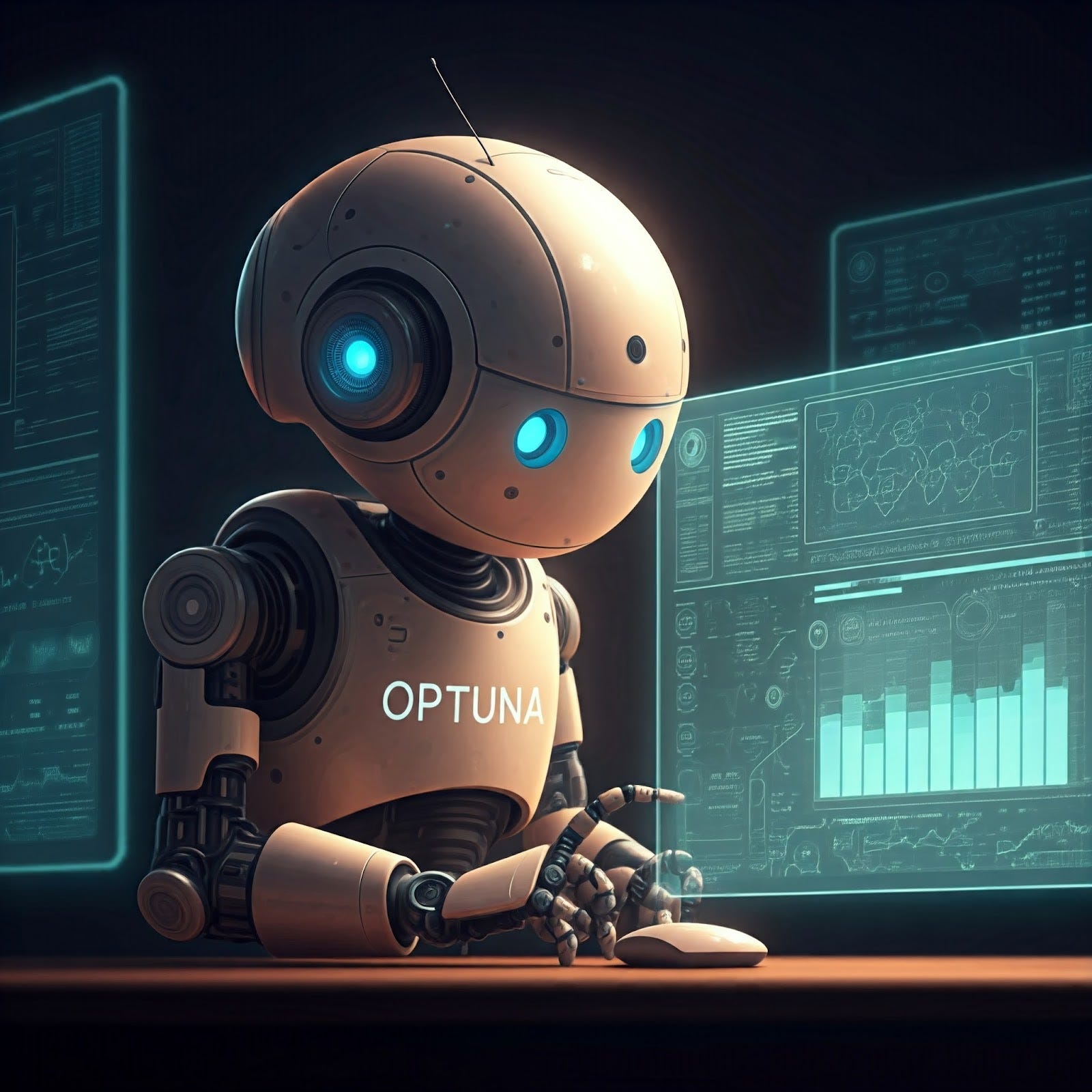
Optuna is a powerful tool for hyperparameter tuning. It uses techniques like the Tree-structured Parzen Estimator (TPE) to explore settings efficiently. You can run multiple trials simultaneously, speeding up the process and improving model performance.
Optuna is capable of handling high-dimensional search spaces well. It employs asynchronous search and pruning to save time and resources. It supports parallel execution, allowing multiple experiments to run at once. Additionally, Optuna's visualization tools help you understand and refine your models better.
Optuna uses Bayesian optimization, which is very effective since it generates a probabilistic model of the objective function and then uses it to choose the most promising hyperparameters to assess next. Because it focuses on the most promising regions of the search space, this method is more effective than random or grid search. Bayesian optimization ensures that the search process improves in accuracy and efficiency over time by constantly updating its model with fresh data.
Bayesian optimization finds its application in healthcare as it can intelligently suggest the best chemical compounds in drug discovery, optimize personalized treatment plans, fine-tune hyperparameters for medical AI models, and calibrate medical devices precisely. These advantages lead to more accurate and reliable models for diagnosing and predicting illnesses.
Ok. But what if you are an R user? Optuna is not directly available in R packages. Don't worry; we can still use Optuna in R.
In this blog post, we will use Python's package Optuna in R with the help of R's package reticulate. Here, we will start with building a baseline model to see how it performs. We will then move to more optimized models, such as implementing grid search in R as well as Python's Optuna, to see how tuning the hyperparameters can improve the accuracy of our model.
This article requires familiarity with R syntax and a basic understanding of hyperparameters in Machine Learning.
Why Reticulate?
Reticulate is a popular package in R that helps us to combine the strengths of both R and Python. We may execute Python code directly in R by using reticulate. When we want to use Python libraries like Optuna without having to convert from R to Python, this is incredibly useful. You can get more information about the reticulate package here.
Setting Up Your Hyperparameter Tuning Environment
First, we will install the reticulate package in R using the following command:
install.packages("reticulate")
After installing the reticulate package, we will use the library()
function to load it:
library(reticulate)
The reticulate package (License: Apache License 2.0) in R uses an isolated Python virtual environment named r-reticulate
. However, we can specify an alternate Python environment using the use_python()
function as shown below:
use_python("/usr/local/bin/python")
We can also enable the specific versions of Python in the conda environment using the following code:
use_condaenv()("myenv")
To enable it in a virtual environment, we can use the use_virtualenv()
function instead of the use_condaenv()
function. Next, to get the Optuna package (License: MIT + file LICENSE) in our R environment, we will use the following commands:
reticulate::py_install("optuna")
optuna <- import('optuna')
Here, the first command, reticulate::py_install()
, allows us to get Optuna installed into our R environment and with the second command, import('optuna')
, we can start using Optuna just like we would in Python.
Case Study Introduction: Leveraging Optuna within R for Healthcare Datasets
In healthcare, accurately diagnosing and predicting illnesses is crucial. Machine learning models help with this, and their performance improves with good hyperparameter tuning. Optuna, a tool for Bayesian optimization, is excellent for this task, especially when used in R.
Bayesian optimization with Optuna is very useful for detecting illnesses like diabetes. It fine-tunes hyperparameters (like learning rate and number of trees) to make models more accurate and reliable. It also customizes models for different groups (such as age, gender, and lifestyle) by efficiently adjusting parameters. For imbalanced datasets, like those with fewer diabetes cases, Optuna finds the best techniques (like class weights and sampling) to improve detection. It also helps identify the most important features (like insulin levels), making models better and easier to understand.
Importing required R packages
Along with Optuna and reticulate, we will require additional libraries to efficiently handle data, build models, and evaluate performance. These packages are tidyverse
(License: MIT + file [[LICENSE](https://cran.r-project.org/web/packages/yardstick/LICENSE)](https://cran.r-project.org/web/packages/tidymodels/LICENSE)) and ** `tidymodels(License: MIT + file LICENSE**). We also require the
yardstick` package (License: MIT + file LICENSE) for measuring model performance and printing metrics.
Let us import them using the library()
function as shown below:
library(lightgbm)
library(tidymodels)
library(tidyverse)
library(mlbench)
library(yardstick)
The Data Source
Here, we will use the built-in PimaIndiansDiabetes
dataset from the mlbench
package (License: GPL-2), where we will predict whether the patient is diabetic or not diabetic. Different categorical features are present in this dataset, such as age, glucose levels, blood pressure, skin thickness, insulin levels, etc.
data(PimaIndiansDiabetes)
diab <- PimaIndiansDiabetes
Here, we have loaded the dataset into our R environment using the data()
function. Also, we have stored the dataset in another object named diab
as a shorter name for our dataset, which will reduce typing errors and improve code readability, making our workflow more efficient. To begin, let us get an overview of this dataset and see if there are any missing values in this dataset using the following commands:
glimpse(diab)

colSums(is.na(diab))

Data Preprocessing and Splitting the Data
There are no missing values in this dataset, and the glimpse of the dataset tells us that there are some entries in the dataset that are mistakenly set to zero, which is not correct for parameters like glucose, insulin, pressure, etc. First, let us find out how many columns in the datasets have such entries with zero values.
zero_cnt <- new_df %>%
summarise(across(everything(), ~ sum(. == 0, na.rm = TRUE)))
print(zero_cnt)

Here, we have used the summarise()
function along with the across()
function, which gives us the output indicating that zero values are present in pregnant, glucose, pressure, triceps, insulin, and mass columns of the dataset. We will replace these values with NA using the mutate()
function from the dplyr
package.
new_df <- diab %>%
mutate(across(c(pregnant, glucose, pressure, insulin, mass, triceps),
~if_else(. == 0, as.numeric(NA), .)))
We have cleaned this incorrect data as it could have a negative impact on our model's performance. By replacing zeros with NA, we have marked these entries as missing values, which we will handle later.
Let us check once again if there are still any zero values remaining.
zero_cnt <- new_df %>%
summarise(across(everything(), ~ sum(. == 0, na.rm = TRUE)))
print(zero_cnt)

There are no zeros in the dataset. Next, we will use the initial_split()
function to split the new data into training and testing sets.
set.seed(123)
df <- initial_split(new_df, prop = 4/5)
df_train <- training(df)
df_test <- testing(df)
Here, we have split the data into an 80:20 ratio, with 80% of the data used for training and 20% for testing. Before moving forward, let us check the sizes of the training and testing datasets to see if the split was performed correctly.
cat("Total data count:", nrow(new_df), "n")
cat("Train data count:", nrow(df_train), "n")
cat("Test data count:", nrow(df_test), "n")

Recipe Creation for Preprocessing
Before we start training our model, let us define a recipe for preprocessing the diabetes data. With the recipe()
function from the tidymodels
framework, we can specify all preprocessing steps in one place, which makes the workflow clean and easy to manage.
df_recipe <- recipe(diabetes ~ pregnant + glucose + pressure + triceps + insulin + mass + pedigree + age,
data = new_df) %>%
step_normalize(all_numeric_predictors()) %>%
step_impute_knn(all_predictors())
We have used the step_impute_knn()
function to handle the NA values and the step_normalize()
function so that all the numeric features in the dataset are scaled to a common range.
Note: We have split the data into training and testing sets before imputing the values. The reason for this is we wanted to make sure that the model does not get any information from the test dataset during training. If we had imputed the entire dataset earlier, we may have got misleading performance metrics. Here, we have fitted the KNN imputer only on the training dataset to help the model learn the relationships between the features of the diabetes dataset without including the test dataset.
Model Building
Next, we will build a baseline model using LightGBM (License: MIT + file LICENSE) without performing any hyperparameter tuning. This will help us to compare the performance of the baseline model with the tuned models we are going to build later.
base_model <- boost_tree() %>%
set_engine("lightgbm") %>%
set_mode("classification")
In the above code, the computational engine is set to lightgbm
using the set_engine()
function, and as our problem task is a classification problem, we have specified it in the set_mode()
function. Next, we will combine our recipe and our defined model into a workflow using the workflow()
function.
base_wflow <- workflow() %>%
add_recipe(df_recipe) %>%
add_model(base_model)
After the baseline workflow is defined, the next task is to fit the model using the train data. This includes training the model on the given data to create a predictive model that we can evaluate later.
base_fit <- base_wflow %>%
fit(data = df_train)
Making Predictions and Performance Metrics
After training the model, we will use it to make predictions on the test dataset. These predictions will tell us how well our model performs on unseen data.
base_pred <- base_fit %>%
predict(new_data = df_test) %>%
bind_cols(df_test)
Here, we have used the predict()
function to generate the model's predictions. Also, we have used the bind_cols()
function that combines the original test dataset predictions for further evaluation. This is an important step as it compares the predicted values with the actual values of diabetes in the target column of the test dataset.
Further, we will print the accuracy, i.e., the percentage of correct predictions for our baseline model, along with the confusion matrix.
# Baseline model accuracy
cat("n=== Baseline Model ===n")
base_mt <- base_pred %>%
metrics(truth = diabetes, estimate = .pred_class)
base_acc <- base_mt %>%
filter(.metric == "accuracy") %>%
pull(.estimate) * 100
cat(sprintf("The accuracy of baseline model is: %.2f%%n", base_acc))
# Baseline model confusion matrix
base_pred %>%
conf_mat(truth = diabetes, estimate = .pred_class) %>%
pluck(1) %>% as_tibble() %>%
ggplot(aes(Truth, Prediction, alpha = n)) +
geom_tile(fill = "#3a86ff", show.legend = FALSE) +
geom_text(alpha = 0.8, size = 5,aes(label = n), colour = "black")+
labs(title = "Baseline Model Confusion Matrix")+theme_bw()+
theme(plot.title = element_text(size = 18, hjust = 0.5))

Here, we have an accuracy of 73.38%, which means our baseline model got 73.38% of the test predictions right. Also, we printed the confusion matrix, which shows that the model correctly predicted 87 non-diabetic cases and 26 diabetic cases. However, it made 15 mistakes in predicting diabetes, i.e., false positives, and did not identify 26 diabetic cases (false negatives). To improve the performance of our model in R, we will move forward with hyperparameter tuning, first with grid search.
Hyperparameter Tuning with Grid Search
One of the popular methods in R to perform hyperparameter tuning is Grid search. This method includes the creation of a grid with hyperparameter values where each combination is evaluated to select the best option based on a chosen metric, such as accuracy.
Let us start by defining a grid search model. Here, we will specify the different hyperparameters, such as the number of trees, learning rate, and tree depth inside the boost_tree()
function with the computational engine set to lightgbm
and the mode to classification
.
grid_model <- boost_tree(
trees = tune(),
learn_rate = tune(),
tree_depth = tune()
) %>%
set_engine("lightgbm") %>%
set_mode("classification")
Next, we will define the workflow and use the grid_regular()
function to define a grid of hyperparameters.
# Workflow
grid_wf <- workflow() %>%
add_recipe(df_recipe) %>%
add_model(grid_model)
# Defining grid
grid <- grid_regular(
parameters(grid_model),
levels = 3
)
With this function, a regular grid is created based on the parameters of our model and the specified number of levels. We will also implement a 5-fold cross-validation to make sure that our model will perform well on unseen data. It will divide the data into multiple parts or folds and will train it on different subsets of data.
df_cv <- vfold_cv(df_train, v = 5)
Now, we will perform the grid search. This is where the model is trained on different parameter combinations and evaluated across each fold of the cross-validation.
# Performing grid search
set.seed(42)
grid_res <- grid_wf %>%
tune_grid(resamples = df_cv, grid = grid, metrics = metric_set(accuracy))
best_grid <- grid_res %>%
select_best(metric = "accuracy")
After performing grid search, we have used the select_best()
function, which helps in identifying the hyperparameter combination that resulted in the highest accuracy. Next, we will update our workflow with the best hyperparameters to move further with the final model fitting.
# Finalizing the workflow with the best parameters
final_grid_wf <- grid_wf %>%
finalize_workflow(best_grid)
Next, by using the fit()
function, we will fit the final grid model on the training data. In addition, we will use the predict()
function to generate predictions on our data.
# Fitting model
final_grid_fit <- final_grid_wf %>%
fit(data = df_train)
# Evaluating model
grid_pred <- final_grid_fit %>%
predict(new_data = df_test) %>%
bind_cols(df_test)
To see the performance of our grid model, let us print the accuracy and create a confusion matrix using the following code:
# Grid search model accuracy
cat("n=== Grid Search Model ===n")
grid_mt <- grid_pred %>%
metrics(truth = diabetes, estimate = .pred_class)
grid_acc <- grid_mt %>%
filter(.metric == "accuracy") %>%
pull(.estimate) * 100
cat(sprintf("The accuracy of grid search model is: %.2f%%n",grid_acc))
# Confusion matrix
grid_pred %>%
conf_mat(truth = diabetes, estimate = .pred_class) %>%
pluck(1) %>% as_tibble() %>%
ggplot(aes(Truth, Prediction, alpha = n)) +
geom_tile(fill = "#3a86ff", show.legend = FALSE) +
geom_text(alpha = 0.8, size = 5,aes(label = n), colour = "black")+
labs(title = "Grid Search Model Confusion Matrix")+theme_bw()+
theme(plot.title = element_text(size = 18, hjust = 0.5))

The accuracy of our grid search model is 75.97%, which shows a significant improvement over the baseline model. The confusion matrix also shows that the number of correct positive predictions has increased, whereas the number of false negatives has decreased.
While our grid search model performance is better than our baseline model, it has certain drawbacks, such as it can be very slow as it tries out every possible combination of hyperparameters. Here, to improve the accuracy of our model further, let us use an advanced method named Optuna to perform more efficient hyperparameter tuning.
Hyperparameter Tuning with Optuna
Next, we will define an objective function to find the best combination of hyperparameters with Optuna.
objective_lgbm <- function(trial) {
trees <- trial$suggest_int("trees", 2000, 2500)
learning_rate <- trial$suggest_loguniform("learning_rate", 0.001, 0.1)
tree_depth <- trial$suggest_int("tree_depth", 3, 15)
In the above code, we have used the suggest_int()
and suggest_loguniform()
functions from Optuna to define the search space for our model's hyperparameters. Next, we will use the boost_tree()
function to define our lgbm model structure with hyperparameters.
lgbm_td <- boost_tree(trees = trees,
learn_rate = learning_rate,
tree_depth = tree_depth) %>%
set_engine("lightgbm") %>%
set_mode("classification")
Similar to our baseline model and grid search model, here we have set the computational engine to lightgbm and the mode to classification. Then, we will create a workflow to combine the preprocessing recipe and the tuned model.
lgbm_wf_td <- workflow() %>%
add_recipe(df_recipe) %>%
add_model(lgbm_td)
Next, we will evaluate the performance of our model using the following code:
cv_res <- lgbm_wf_td %>%
fit_resamples(resamples = df_cv,
metrics = metric_set(accuracy),
control = control_resamples(save_pred = TRUE))
mean_acc <- collect_metrics(cv_res) %>%
filter(.metric == "accuracy") %>%
summarize(mean_acc = mean(mean))
return(-mean_acc$mean_acc)
}
To help with optimization using Optuna, we calculate the average accuracy of the folds and return its negative value. This is important as Optuna tries to minimize an objective function; in simple words, it tries to find the smallest value during optimization. However, our aim is to maximize the accuracy of our model, not minimize it. So, to fit with Optuna's working style, we need to return the negative accuracy. Negative accuracy is simply the accuracy with a negative sign in front of it. For example, if the accuracy is 0.75, we return -0.75.
Thus, by minimizing the negative accuracy, Optuna is actually working to maximize the actual accuracy. This helps Optuna to find the best settings for our model to perform best. Negative accuracy is just a smart way to guide Optuna in maximizing the accuracy of our model.
Next, we will run the optimization for 50 trials to find the best hyperparameters and will store them in an object named best_params
.
# Running 50 trials
op_study <- optuna$create_study(direction = "minimize")
op_study$optimize(objective_lgbm, n_trials = 50)
# Getting the best hyperparameters
best_params <- op_study$best_params
print(best_params)

Using the above hyperparameters, we will again create the LightGBM model and specify the tuned values.
final <- boost_tree(
trees = best_params$trees,
learn_rate = best_params$learning_rate,
tree_depth = best_params$tree_depth,
) %>%
set_engine("lightgbm") %>%
set_mode("classification")
Now, we will build the workflow using the workflow()
function to integrate the preprocessing recipe, i.e., df_recipe
, and the final model, i.e., final
. Next, we will fit this model on the training data and make predictions on the test data.
# Workflow
td_wf <- workflow() %>%
add_recipe(df_recipe) %>%
add_model(final)
# Fitting model
td_fit <- td_wf %>%
fit(data = df_train)
# Evaluating model
td_pred <- td_fit %>%
predict(new_data = df_test) %>%
bind_cols(df_test)
Finally, let us print the accuracy and confusion matrix to see if the tuned model shows improved performance compared to the baseline and grid search model.
# Tuned model accuracy
cat("n=== Optuna Tuned Model ===n")
td_mt <- td_pred %>%
metrics(truth = diabetes, estimate = .pred_class)
tun_acc <- td_mt %>%
filter(.metric == "accuracy") %>%
pull(.estimate) * 100
cat(sprintf("The accuracy of Optuna tuned model is: %.2f%%n",tun_acc))
# Tuned model confusion matrix
td_pred %>%
conf_mat(truth = diabetes, estimate = .pred_class) %>%
pluck(1) %>% as_tibble() %>%
ggplot(aes(Truth, Prediction, alpha = n)) +
geom_tile(fill = "#3a86ff", show.legend = FALSE) +
geom_text(alpha = 0.8, size = 5,aes(label = n), colour = "black")+
labs(title = "Optuna Tuned Model Confusion Matrix")+theme_bw()+
theme(plot.title = element_text(size = 18, hjust = 0.5))

You can find the Kaggle notebook for this tutorial here. If you prefer to try running it locally as an Rscript, you can download the code from my GitHub repository.
Analyzing the Results: Has Optuna Improved the Model Predictions in R?
There is a reasonable improvement in accuracy in the tuned model as compared to the grid search model, as it increased from 75.97% to 77.92%. The confusion matrix also tells us that there is an improvement in correctly identifying the positive cases and reducing the negative cases.
Overall, we started with a baseline model that achieved 73.38% accuracy. By using Grid Search, the performance improved further with an accuracy of 75.97%, and finally, with Optuna, we further improved the model accuracy to 77.92%.
Note: The final accuracy for Optuna can likely vary on different machines.
For simpler models, the observed variations in Optuna model accuracy could usually be smaller, but for complex models like deep learning, they may be larger. So, even if the same hyperparameters are chosen, and a random seed is specified, the final accuracy may likely vary due to certain factors such as :
- Inherent randomness in Machine Learning models during model training
- The adaptive learning and stochastic nature of Optuna
- Differences in hardware (CPU/GPU or memory configurations for the running machine)
- Parallelization differences (numbers of CPU cores or different GPU setups), operation (random number generation, batch processing, etc.), timing, and execution order can vary.
- Data shuffling and cross-validation splits
An important thing to note here is that sometimes Optuna's performance could be further improved by adding pruning options. These enable detecting and stopping unpromising trials early, which saves computational resources and allows for faster convergence. You can refer to the official documentation here.
When to prefer Optuna over GridSearch for optimization
Using Optuna in R could be a better choice over Grid Search for hyperparameter tuning for the following cases:
- When working with large and complex datasets (Medical imaging, genomic data, e-commerce, fraud detection, etc.)
- Handling several hyperparameter models involving xgboost, neural networks, reinforcement learning, etc.
- When cost and resource efficiency are critical (High-dimensional genetic data, large-scale patient data, autonomous driving, marketing data)
- Faster convergence is expected, such as deep learning for medical diagnosis, image recognition, and natural language processing.
- Need a wider search space for accuracy for hyperparameter tuning in cases of cancer detection, stock price prediction, etc.
- Requiring fewer trials for quick tuning (e.g., Time-sensitive diagnostics, real-time patient monitoring, real-time bidding, cybersecurity)
- Tuning complex models (e.g., CNNs, random forests, recommendation engines, credit risk assessment, etc.)
Conclusion
In conclusion, Hyperparameter tuning is an important part of building an ML or DL model that we should not skip.
Python package ‘Optuna' provides dynamic search spaces and pruning strategies, which make it efficient for projects involving complex models and large datasets. It can run multiple trials at once and provides helpful visualizations, quickly finding the best hyperparameters and improving model performance.
While our model performance results for the diabetes prediction case study looked good, there is much scope for improvement. For example, we can use and explore different hyperparameter options or different algorithms, such as random forests, xgboost, etc., or experiment with pruning options. Depending on how big and complex our data is, we can use different methods like Grid Search or advanced methods like Optuna to get better results.
References:
Pima Indians Diabetes Dataset Source
- Original owners: National Institute of Diabetes and Digestive and Kidney Diseases
- Donor of database: Vincent Sigillito ([email protected])
These data have been taken from the UCI Repository Of Machine Learning Databases at
- ftp://ftp.ics.uci.edu/pub/machine-learning-databases
- http://www.ics.uci.edu/~mlearn/MLRepository.html
and were converted to R format by Friedrich Leisch.