Llama-2 vs. Llama-3: a Tic-Tac-Toe Battle Between Models
About a week before the time of writing this story, new open Llama-3 models were released by Meta. As claimed by Meta, these are "the best models existing today at the 8B and 70B parameter scales." For example, according to a HuggingFace model page, Llama-3 8B got a 66.6 score compared to 45.7 for Llama-2 7B in the MMLU (Massive Multitask Language Understanding) benchmark. A Llama-3 also got a 72.6 vs. 57.6 score in CommonSense QA (dataset for commonsense question answering). An instruction-tuned Llama-3 8B model got a 30.0 compared to a 3.8 score in a math benchmark, which indeed is an impressive improvement.
Academic benchmarks are important, but can we see the real difference "in action"? Apparently, we can, and it can be fun. Let's program a tic-tac-toe game between two models and see which one wins! During the game, I will test all 7B, 8B, and 70B models. In parallel, I will also collect some data about model performance and system requirements. All tests can be run for free in Google Colab.
Let's get started!
Loading The Models
To test all models, I will use the Llama-cpp Python library because it can run on both CPU and GPU. We will need to run two LLMs in parallel. Both 7B and 8B models can easily run on a free 16GB Google Colab GPU instance, but 70B models can be tested only using the CPU; even the NVIDIA A100 does not have enough RAM to run two models at the same time.
First, let's install Llama-cpp and download 7B and 8B models (for 70B models, the process is the same; we only need to change URLs):
!CMAKE_ARGS="-DLLAMA_CUBLAS=on" FORCE_CMAKE=1 pip3 install llama-cpp-python -U
!pip3 install huggingface-hub hf-transfer sentence-transformers
!export HF_HUB_ENABLE_HF_TRANSFER="1" && huggingface-cli download TheBloke/Llama-2-7B-Chat-GGUF llama-2-7b-chat.Q4_K_M.gguf --local-dir /content --local-dir-use-symlinks False
!export HF_HUB_ENABLE_HF_TRANSFER="1" && huggingface-cli download QuantFactory/Meta-Llama-3-8B-Instruct-GGUF Meta-Llama-3-8B-Instruct.Q4_K_M.gguf --local-dir /content --local-dir-use-symlinks False
When the download is complete, let's start the model:
from llama_cpp import Llama
llama2 = Llama(
model_path="/content/llama-2-7b-chat.Q4_K_M.gguf",
n_gpu_layers=-1,
n_ctx=1024,
echo=False
)
llama3 = Llama(
model_path="/content/Meta-Llama-3-8B-Instruct.Q4_K_M.gguf",
n_gpu_layers=-1,
n_ctx=1024,
echo=False
)
Now, let's prepare a function to execute a prompt:
def llm_make_move(model: Llama, prompt: str) -> str:
""" Call a model with a prompt """
res = model(prompt, stream=False, max_tokens=1024, temperature=0.8)
return res["choices"][0]["text"]
Prompts
Now, it is time to code the tic-tac-toe game process. The goal of the game is to draw "X" and "O" on the board, and the player who first makes the horizontal, vertical, or diagonal line wins:

As we can see, the game is easy for humans, but it can be challenging for a language model; making the right move requires an understanding of the board space, relations between objects, and even some simple math.
First, let's encode the board as a 2D array. I will also make a method to convert the board to a string:
board = [["E", "E", "E"],
["E", "E", "E"],
["E", "E", "E"]]
def board_to_string(board_data: List) -> str:
""" Convert board to the string representation """
return "n".join([" ".join(x) for x in board_data])
The output looks like this:
E E E
E E E
E E E
Now, we are ready to create model prompts:
sys_prompt1 = """You play a tic-tac-toe game. You make a move by placing X,
your opponent plays by placing O. Empty cells are marked
with E. You can place X only to the empty cell."""
sys_prompt2 = """You play a tic-tac-toe game. You make a move by placing O,
your opponent plays by placing X. Empty cells are marked
with E. You can place O only to the empty cell."""
game_prompt = """What is your next move? Think in steps.
Each row and column should be in range 1..3. Write
the answer in JSON as {"ROW": ROW, "COLUMN": COLUMN}."""
Here, I created two prompts for models 1 and 2. As we can see, the sentences are the same; the only difference is that the first model is making moves by placing Xs, and the second model is placing Os.
The prompt formats for Llama-2 and Llama-3 are different:
template_llama2 = f"""[INST]<>{sys_prompt1}< >
Here is the board image:
__BOARD__n
{game_prompt}
[/INST]"""
template_llama3 = f"""<|begin_of_text|>
<|start_header_id|>system<|end_header_id|>{sys_prompt2}<|eot_id|>
<|start_header_id|>user<|end_header_id|>
Here is the board image:
__BOARD__n
{game_prompt}
<|eot_id|>
<|start_header_id|>assistant<|end_header_id|>"""
Let's also make two methods to use these prompts:
def make_prompt_llama2(board: List) -> str:
""" Make Llama-2 prompt """
return template_llama2.replace("__BOARD__", board_to_string(board))
def make_prompt_llama3(board: List) -> str:
""" Make Llama-3 prompt """
return template_llama3.replace("__BOARD__", board_to_string(board))
Coding the Game
We have prepared the prompts; now it's time to code the game itself. In a prompt, I asked the model to return an answer in JSON format. Practically, the model can answer this:
My next move would be to place my X in the top-right corner, on cell (3, 1).
{
"ROW": 3,
"COLUMN": 1
}
Let's make a method to extract JSON from this kind of string:
def extract_json(response: str) -> Optional[dict]:
""" Extract dictionary from a response string """
try:
# Models sometimes to a mistake, fix: {ROW: 1, COLUMN: 2} => {"ROW": 1, "COLUMN": 2}
response = response.replace('ROW:', '"ROW":').replace('COLUMN:', '"COLUMN":')
# Extract json from a response
pos_end = response.rfind("}")
pos_start = response.rfind("{")
return json.loads(response[pos_start:pos_end+1])
except Exception as exp:
print(f"extract_json::cannot parse output: {exp}")
return None
It turned out that a LLaMA-2 model does not always make a valid JSON; pretty often, it generates a response like "{ROW: 3, COLUMN: 3}". As we can see in the code, in that case, I update quotes in the string with a proper one.
After getting the row and column, we can update the board:
def make_move(board_data: List, move: Optional[dict], symb: str):
""" Update board with a new symbol """
row, col = int(move["ROW"]), int(move["COLUMN"])
if 1 <= row <= 3 and 1 <= col <= 3:
if board_data[row - 1][col - 1] == "E":
board_data[row - 1][col - 1] = symb
else:
print(f"Wrong move: cell {row}:{col} is not empty")
else:
print("Wrong move: incorrect index")
We also need to check if the game is finished:
def check_for_end_game(board_data: List) -> bool:
""" Check if there are no empty cells available """
return board_to_string(board_data).find("E") == -1
def check_for_win(board_data: List) -> bool:
""" Check if the game is over """
# Check Horizontal and Vertical lines
for ind in range(3):
if board_data[ind][0] == board_data[ind][1] == board_data[ind][2] and board_data[ind][0] != "E":
print(f"{board_data[ind][0]} win!")
return True
if board_data[0][ind] == board_data[1][ind] == board_data[2][ind] and board_data[0][ind] != "E":
print(f"{board_data[0][ind]} win!")
return True
# Check Diagonals
if board_data[0][0] == board_data[1][1] == board_data[2][2] and board_data[1][1] != "E" or
board_data[2][0] == board_data[1][1] == board_data[0][2] and board_data[1][1] != "E":
print(f"{board_data[1][1]} win!")
return True
return False
Here, I check the horizontal, vertical, and diagonal lines in a loop. Maybe a shorter solution is available, though it works well enough for the task.
All our components are ready. Let's combine them together:
num_wins1, num_wins2 = 0, 0
times_1, times_2 = [], []
def run_game():
""" Run a game between two models """
board = [["E", "E", "E"],
["E", "E", "E"],
["E", "E", "E"]]
moves_limit = 20
for step in range(moves_limit):
print(f"Step {step+1}")
# Move: Model-1
t_start = time.monotonic()
prompt = make_prompt_llama2(board)
result_str = llm_make_move(llama2, prompt)
times_1.append(time.monotonic() - t_start)
new_data = extract_json(result_str)
if new_data is not None:
make_move(board, new_data, symb="X")
if check_for_win(board):
print('**Model 1 Won**')
num_wins1 += 1
break
if check_for_end_game(board):
break
# Move: Model-2
t_start = time.monotonic()
prompt = make_prompt_llama3(board)
result_str = llm_make_move(llama3, prompt)
times_2.append(time.monotonic() - t_start)
new_data = extract_json(result_str)
if new_data is not None:
make_move(board, new_data, symb="O")
if check_for_win(board):
print('**Model 2 Won**')
num_wins2 += 1
break
if check_for_end_game(board):
break
print()
Here, I also saved an execution time for each step and the number of wins for every model.
Results
As for the results, they are interesting.
For 7B and 8B models, the Tic-Tac-Toe game is challenging. A 7B Llama-2 model has some understanding of the rules, but it was not able to grasp the idea of the board coordinates and often placed the "X" into the wrong position.
Some requests and responses look like this:
>>> Prompt:
[INST]<>You play a tic-tac-toe game. You make a move by placing X,
your opponent plays by placing O. Empty cells are marked with E.
You can place X only to the empty cell.< >
Here is the board image:
X X O
X O E
E E E
What is your next move? Think in steps.
Each row and column should be in range 1..3.
Write the answer in JSON as {"ROW": ROW, "COLUMN": COLUMN}.
[/INST]
>>> Response:
Since there are no empty cells on the board, I will place my X in the
center cell: { "ROW": 2, "COLUMN": 1 }.
As we can see, there are several errors in this response. First, there are enough empty cells on the board; second, a (2,1) position does not represent a center; and third, this position is not empty anyway.
A 70B LLaMA-2 model was generally better, but it also made enough errors. This is a typical request and response:
>>> Prompt:
[INST]<>You play a tic-tac-toe game. You make a move by placing X,
your opponent plays by placing O. Empty cells are marked with E.
You can place X only to the empty cell.< >
Here is the board image:
O E E
E X E
E E E
...
>>> Response:
My next move would be to place my X in the center cell, which is empty.
Here is the updated board:
O E E
E X X
E E E
JSON representation of my move: {"ROW": 2, "COLUMN": 2}
As we can see, the 70B model correctly determined a "center" position, but the move itself was wrong; the model still did not "understand" that the central cell was not empty. The model also tried to "draw" a new board, and the "drawing" was also incorrect.
Funny enough, ChatGPT 3.5 also gave the wrong answer to the same prompt and produced the same {"ROW": 2, "COLUMN": 2} result. But the LLaMA-3 70B did it right. However, it still made similar errors and sometimes tried to place symbols in occupied cells. I did not log the total number of errors per model, though it can be a useful improvement.
In the bar chart form, results for the 7B and 8B models look like this:
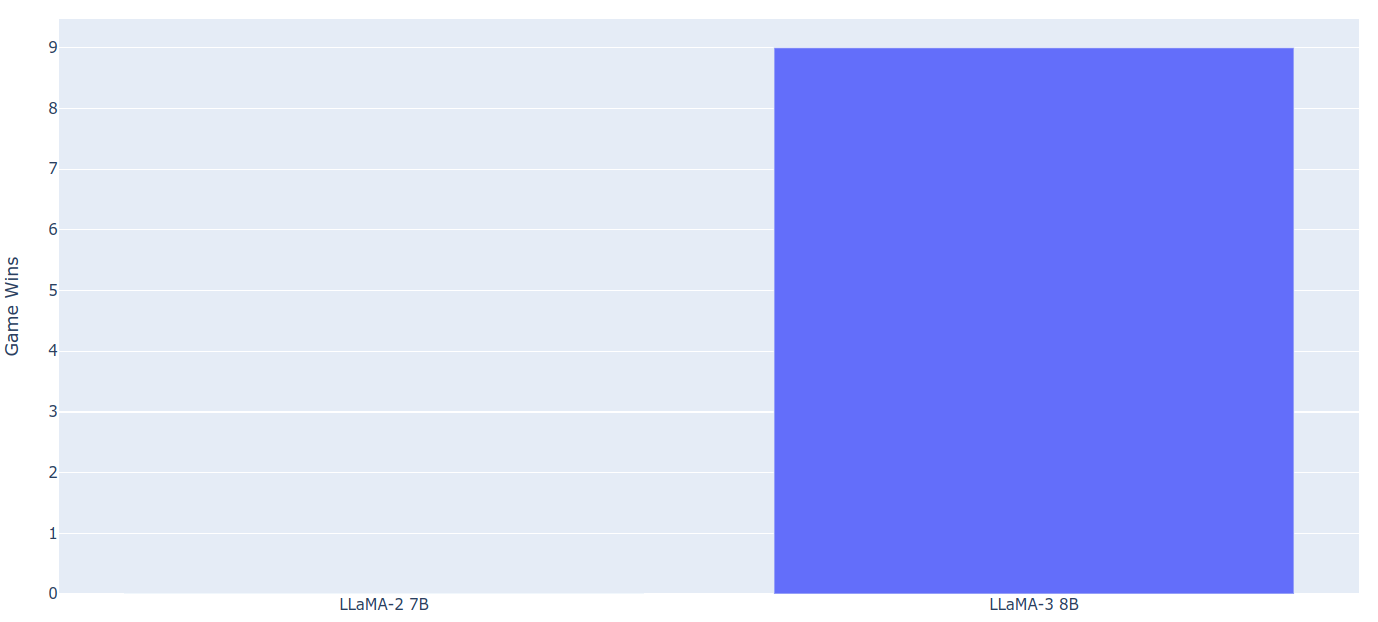
The winner is clear: a score of 10:0 for Llama-3!
We can also see the inference time for both models, running on a 16 GB NVIDIA T4 GPU:


As a slight disadvantage, a Llama-3 is slower compared to a previous model (2.5 vs. 4.3 seconds, respectively). Practically, 4.3s is good enough because, in most cases, streaming is used and nobody expects an immediate answer.
A Llama-2 70B performed better. It could win twice, but Llama-3 still won in almost all cases. A score of 8:2 for Llama-3!

As for the CPU inference for a 70B model, it is, naturally, not fast:

The batch of 10 games took about an hour. This speed is not suitable for production, but it is okay for testing. Interestingly, Llama-cpp uses a memory-mapped file to load a model, and even for two 70B models in parallel, the RAM consumption did not exceed 12 GB. It means that even two 70B models can be tested on a PC with only 16 GB of RAM (alas, this trick does not work for a GPU).
Conclusion
In this article, I made a tic-tac-toe game between two language models. Interestingly, this "benchmark" is challenging. It requires an understanding of not only game rules but also coordinates and some sort of "spatial" and "abstract thinking" in representing a 2D board in a string form.
As for the results, LLaMA-3 was a clear winner. This model is definitely better, but I must admit that both models made a lot of errors. This is interesting, and it means that this small, unofficial "benchmark" is hard for LLMs and can be used for testing future models as well.
Thanks for reading. If you enjoyed this story, feel free to subscribe to Medium, and you will get notifications when my new articles will be published, as well as full access to thousands of stories from other authors. You are also welcome to connect via LinkedIn. If you want to get the full source code for this and other posts, feel free to visit my Patreon page.
Those who are interested in using language models and natural language processing are also welcome to read other articles:
- GPT Model: How Does it Work?
- 16, 8, and 4-bit Floating Point Formats – How Does it Work?
- Process Pandas DataFrames with a Large Language Model
- A Weekend AI Project (Part 1): Running Speech Recognition and a LLaMA-2 GPT on a Raspberry Pi
- A Weekend AI Project (Part 2): Using Speech Recognition, PTT, and a Large Action Model on a Raspberry Pi
- A Weekend AI Project (Part 3): Making a Visual Assistant for People with Vision Impairments