Methods for generating synthetic descriptive data
Generating Synthetic Descriptive Data in PySpark

In a previous article, we explored creating many-to-one relationships between columns in a synthetic PySpark DataFrame. This DataFrame only consisted of Foreign Key information and we didn't produce any textual information that might be useful in a demo DataSet.
For anyone looking to populate an artificial dataset, it is likely you will want to produce descriptive data such as product information, location details, customer demographics, etc.
In this post, we'll dig into a few sources that can be used to create synthetic text data at little effort and cost, and use the techniques to pull together a DataFrame containing customer details.
Why create an synthetic dataset?
Synthetic datasets are a great way to anonymously demonstrate your data product, such as a website or analytics platform. Allowing users and stakeholders to interact with example data, exposing meaningful analysis without breaching any privacy concerns with sensitive data.
It can also be great for exploring Machine Learning algorithms, allowing Data Scientists to train models in the case of limited real data.
Performance testing Data Engineering pipeline activities is another great use case for synthetic data, giving teams the ability to ramp up the scale of data pushed through an infrastructure and identify weaknesses in the design, as well as benchmarking runtimes.
In my case, I'm currently creating an example dataset to performance-test some Power BI capabilities at high volumes, which I'll be writing about in due course.
The dataset will contain sales data, including transaction amounts and other descriptive features such as store location, employee name and customer email address.
Random characters
Starting off simple, we can use some built-in functionality to generate random text data. Importing the random and string Python modules, we can use the following simple function to create a random string of the desired length.

We can run this multiple times to generate enough data for our column, appending the information to a list.
We will review how to add this to our DataFrame further down this post.
Benefits and Limitations
This kind of data generation is very generic, with limited applications in demo datasets. That being said, it can be combined with other string generation techniques (such as concatenation) to give a bit more value at very little effort.
This can be seen below for random usernames, with a base of a first name appended by an underscore and random characters:

Example data points where this could be useful may include:
- Email addresses
- Passwords
- Product codes
- Usernames
APIs
APIs are a great source of information, and will likely be data sources when building your real Analytics platforms!
However, there are also many API endpoints worth querying for generic placeholder data that can give you more meaning for demo dashboards through representative data for various topics, such as currency rates.
If we want to retrieve geographical data for a field for a user's country, we could generate this from the rest countries API endpoint. This API is free to access and requires no sign-up to get started. Using the requests python module we're up and running with a list of countries very quickly.

Note: You should always sense-check your outputs – this API request returns 250 results, which exceeds the real-life number of countries!

Benefits and Limitations
Getting data from APIs can vary in complexity and security requirements can be off-putting. The way data is requested can also vary, as can the format in which it returns. Documentation is produced by the provider themselves, which can also be a bottleneck worth considering.
It is worth mentioning at this point that there are various packages created by developers to interact with APIs in simplified manners – we'll talk about third-party Python libraries in the next section!
With all of that being said, there is plenty of rich data available for you to pull that may be utilised in customer-facing data products as well as for demo purposes.
Third-party packages
There are also some great ready-made packages out there for us to use. This takes some of the heavy effort out of finding a source, processing and formatting the data ready for consumption.
Faker is one such example, with the ability to generate names, addresses, etc.
Install, import and use packages like this at your convenience!
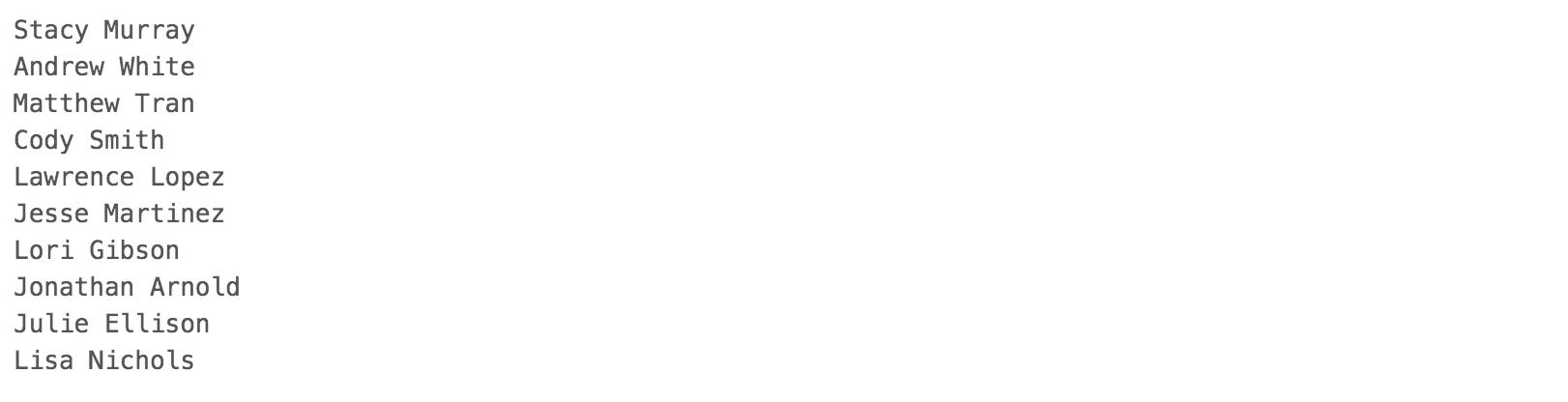
Benefits and Limitations
As much of the leg-work has been done for you by other developers, packages such as Faker can be very high-impact and low-cost (in both price and time).
With just Faker in mind, we've already been able to generate generic and informative user data intuitively. Other libraries are available (often interacting with common APIs) to allow developers to easily pull useful through to their applications.
Blockers could lie within your organisation's regulations around using third-party packages, licensing and maintainability of the package itself, so please be aware of possible restrictions here.
ChatGPT
It wouldn't be fitting to overlook Large Language Models (LLMs) as they are a great asset in data generation.
A simple approach could be to get ChatGPT (or equivalent) to generate you a list of data points, such as possible customer names.

We can do one better and ask for the LLM to help build the random variable function itself. You can include details about data sources and even ask ChatGPT to write a function to interact with an API you have found.
An example here is requesting help with the bored API, extracting the activity field and returning it for your own use:


Note: Always check the output of your result to ensure an LLM generated function is working as expected.
Benefits and Limitations
Generative AI is showing its strengths in rapid data generation (prominently around content creation, as shown by this Gartner report) and I expect to see more grounded approaches for this in populating example datasets next year. As such, it makes creation of various data points at scale accessible to users of various backgrounds and levels of technicality.
With that being said, reliability and consistency of LLMs is being heavily investigated at the same time. Depending on the type of data you need to populate, this may introduce rigorous checks into data quality and anonymity and determine whether or not this is the right tool for specific use cases.
Putting this into a DataFrame
The approach we've taken across the previous sections is to generate our data ready to be processed into the DataFrame column of interest.
There are a few choices of how to implement the synthetic data at this stage. UDFs are a neat way to apply a function for each row of the column and can help define uniqueness.
Databricks Labs Data Generator is another great library that can help speed things up with distributed computing at the core of this data generation.
Manipulating how we generate the lists of values to be populated, we can implement the withColumn PySpark function and combine this with our list data to produce our text column at great speed.
For our test case, we'll use a combination of both of these approaches. We can use Databricks Labs Data Generator to create our DataFrame wireframe, populating 100 rows with a unique Id column, and any values we might not require to be unique, such as Country.
Other columns which we might wish to be distinct, such as the Full Name and Username, we can make use of a UDF to use the id column to access a unique name from the list we've created.
Putting it all together, we can create our DataFrame:

A quick data profile shows that our Full Name and Username columns have completely unique values as desired!

Conclusion
We've outlined a variety of methods to generate textual Synthetic Data quickly, allowing us to accelerate our demonstrative dataset creation.
All the examples above can be extended, refined and tailored to your specific use-case.
Are there any tricks that I have missed? What are you using synthetic datasets for? Let me know in the comments!
Thanks for reading, and as always, feel free to find the code for your own use here.