promptrefiner: Using GPT-4 to Create a Perfect System Prompt for Your Local LLM

In this tutorial, we will explore promptrefiner
: A tiny python tool I have created to create perfect system prompts for your local Llm, by using the help of the GPT-4 model.
The python code in this article is available here:
https://github.com/amirarsalan90/promptrefiner.git
Crafting an effective and detailed system prompt for your program can be a challenging process that often requires multiple trials and errors, particularly when working with smaller LLMs, such as a 7b language model. which can generally interpret and follow less detailed prompts, a smaller large language model like Mistral 7b would be more sensitive to your system prompt.
Let's imagine a scenario where you're working with a text. This text discusses a few individuals, discussing their contributions or roles. Now, you want to have your local language model, say Mistral 7b, distill this information into a list of Python strings, each pairing a name with its associated details in the text. Take the following paragraph as a case:

For this example, I would like to have a perfect prompt that results in the LLM giving me a string like the following:
"""
["Elon Musk: Colonization of Mars", "Stephen Hawking: Warnings about AI", "Greta Thunberg: Environmentalism", "Digital revolution: Technological advancement and existential risks", "Modern dilemma: Balancing ambition with environmental responsibility"]
"""
When we use an instruction fine-tuned language model (language models that are fine-tuned for interactive conversations), the prompt usually consists of two parts: 1)system prompt, and 2)user prompt. For this example, consider the following system and user prompt:
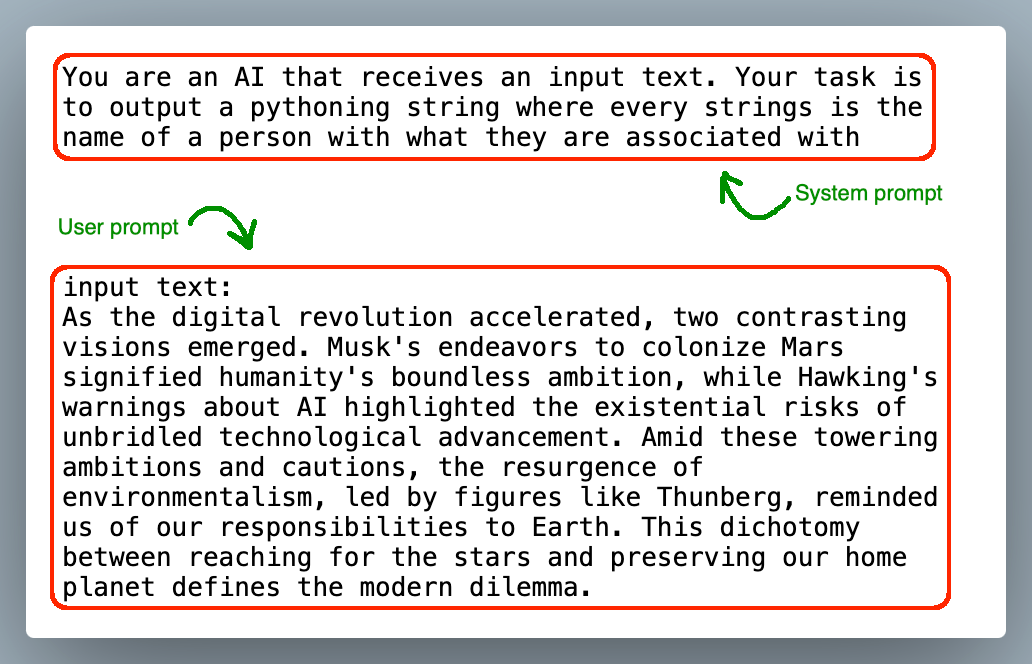
You see the first part of this prompt is my system prompt that tells the LLM how to generate the answer, and the second part is my user prompt, which is the input text. Using the above prompt with the Mistral 7b model gives me the following output:
```python persons = { "Musk, Elon": "space_exploration", "Hawking, Stephen": "ai_ethics",
"Thunberg, Greta": "environmentalism" } print(f"Elon Musk is known for {persons['Musk, Elon']}")
print(f"Stephen Hawking is known for {persons['Hawking, Stephen']}") print(f"Greta Thunberg is known
for {persons['Thunberg, Greta']}") ``` This Python code creates a dictionary called `persons` where
each key-value pair represents a person and their associated field. The `print` statements then
display the person's name and their field of expertise.
Clearly, the result isn't what we want. While we could manually trim away the parts we don't need, think about scaling this up – processing, say, 10,000 texts. That's when the manual approach becomes unfeasible, leading us towards more sophisticated solutions like the proprietary GPT models, which can handle such volume without the need for constant manual adjustments.
It turns out that the following combination of system prompt and user prompt, will give me something very close to what I want!:
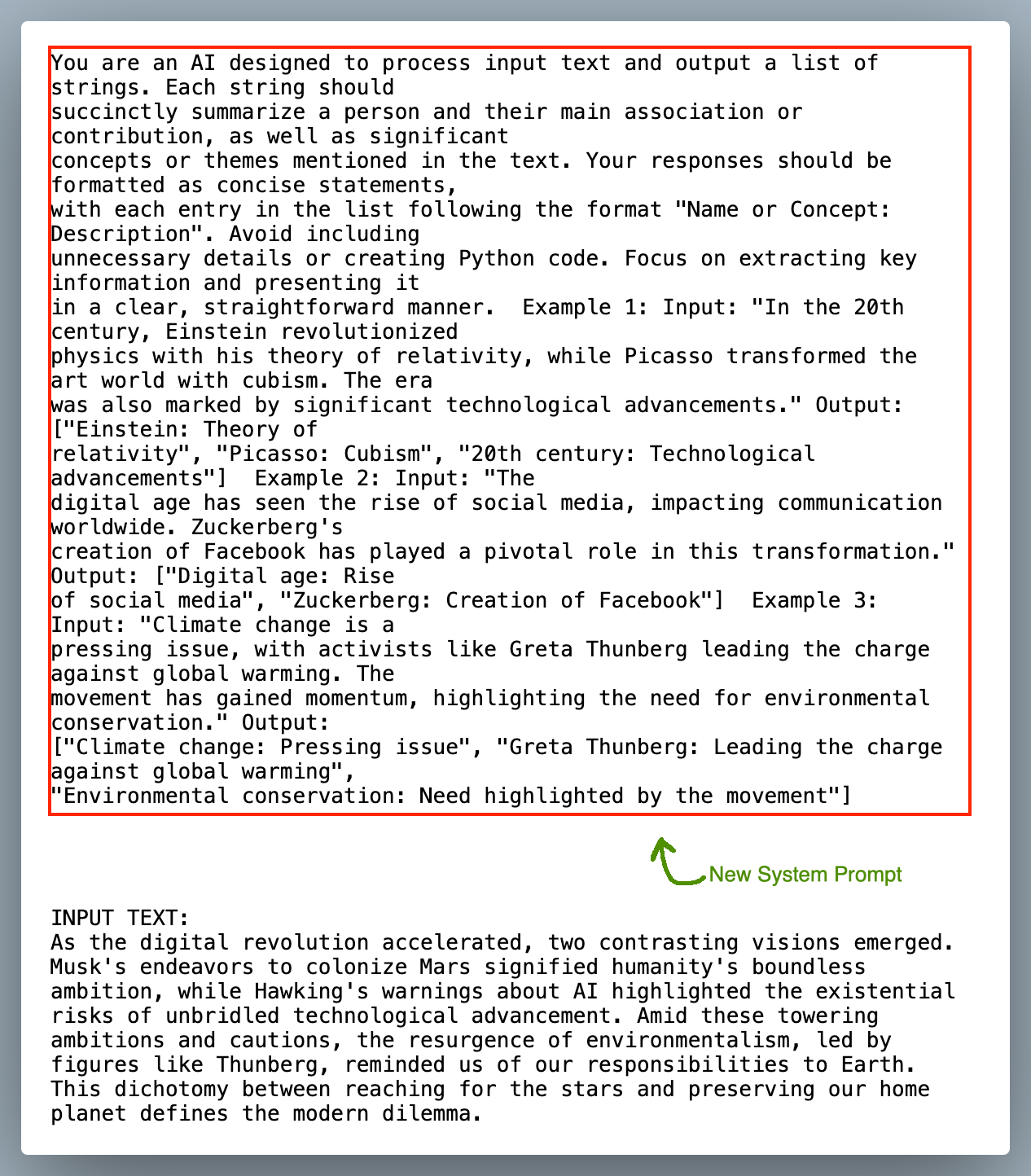
As you see, the new system prompt includes a detailed instruction on what to do and how to do it, with system prompt including three examples. I don't know about you, but writing this detailed and thought-about system prompt is a challenging task for me. The result of running Mistral LLM on the new system prompt+ user prompt is the following:
["Musk: Colonizing Mars", "Hawking: Warnings about AI", "Modern dilemma: Reaching for the stars vs
preserving Earth", "Thunberg: Environmentalism"]
Pretty close to what we want!
In what follows, I will explain the small python approach I created to implement this, which is provided in this repo, along with a demo notebook:
Using promptrefiner
Using promptrefiner is quite simple. All you need are a few examples, much like the Elon Musk scenario we discussed earlier. For each, you'll provide the text you want evaluated, alongside the output you're hoping for.
In the demo notebook, we should import openai
and dotenv
modules. openai
is to communicate with OpenAI GPT API and dotenv
is used to read our OPENAI_API_KEY environment variable from a text file in our root folder.
from dotenv import load_dotenv
from openai import OpenAI
load_dotenv("env_variables.env")
client = OpenAI()
We also have to import the primary promptrefiner classes:
from promptrefiner import AbstractLLM, PromptTrackerClass, OpenaiCommunicator
Here AbstractLLM
will be a base class that the local LLM Class inherits from, PromptTrackerClass
will keep evaluation prompts and system prompts in each iteration inside it, and OpenaiCommunicator
is responsible for communication with OpenAI API GPT models. I recommend using GPT-4 models to get the best results.
The somewhat tricky part is to create your LLM class that inherits from the AbstractLLM
class. In my example, I am using llama-cpp-python LLM, which is a python wrapper around llama.cpp . We can serve GGUF models with llama-cpp-python, which are lightweight and available in different quantized versions. You can find a more detailed explanation of how to use and serve a GGUF model with llama-cpp-python in one of my previous posts here:
Building Your Own Personal AI Assistant: A Step-by-Step Guide to Build a Text and Voice Local LLM
After running the llm engine server, I can communicate with my local mistral model using openai
package. The following code shows how to create the LLM class that inherits from AbstractLLM class:
class LlamaCPPModel(AbstractLLM):
def __init__(self, base_url, api_key, temperature=0.1, max_tokens=200):
super().__init__()
self.temperature = temperature
self.max_tokens = max_tokens
from openai import OpenAI
self.client = OpenAI(base_url=base_url, api_key=api_key)
def predict(self, input_text, system_prompt):
response = self.client.chat.completions.create(
model="mistral",
messages=[
{"role": "system", "content": system_prompt}, # Update this as per your needs
{"role": "user", "content": input_text}
],
temperature=self.temperature,
max_tokens=self.max_tokens,
)
llm_response = response.choices[0].message.content
return llm_response
I have named the class LlamaCPPModel
and as you see it is inheriting from AbstractLLM
class. You are free to include as many arguments as you want when defining __init__
functions and predict
functions. for example I have base_url, api_key, temperature=0.1, max_tokens=200
arguments in my __init__
function. The important thing to remember is that 1) you MUST define a predict
function in your class, and 2) The predict function MUST include these two arguments: input_text
, and system_prompt
.
Next, we will create an object from LlamaCPPModel
class:
llamamodel = LlamaCPPModel(base_url="http://localhost:8000/v1", api_key="sk-xxx", temperature=0.1, max_tokens=400)
Next thing to do is to create evaluation inputs and outputs. In this example, we are giving two evaluation input outputs.:
input_evaluation_1 = """In an era where the digital expanse collided with the realm of human creativity, two figures stood at the forefront. Ada, with her prophetic vision, laid the groundwork for computational logic, while Banksy, shrouded in anonymity, painted the streets with social commentary. Amidst this, the debate over AI ethics, spearheaded by figures like Bostrom, questioned the trajectory of our technological companions. This period, marked by innovation and introspection, challenges us to ponder the relationship between creation and creator."""
input_evaluation_2 = """As the digital revolution accelerated, two contrasting visions emerged. Musk's endeavors to colonize Mars signified humanity's boundless ambition, while Hawking's warnings about AI highlighted the existential risks of unbridled technological advancement. Amid these towering ambitions and cautions, the resurgence of environmentalism, led by figures like Thunberg, reminded us of our responsibilities to Earth. This dichotomy between reaching for the stars and preserving our home planet defines the modern dilemma."""
output_evaluation_1 = """
["Ada Lovelace: Computational logic", "Banksy: Social commentary through art", "Nick Bostrom: AI ethics", "Digital era: Innovation and introspection", "AI ethics: The debate over the moral implications of artificial intelligence"]
"""
output_evaluation_2 = """
["Elon Musk: Colonization of Mars", "Stephen Hawking: Warnings about AI", "Greta Thunberg: Environmentalism", "Digital revolution: Technological advancement and existential risks", "Modern dilemma: Balancing ambition with environmental responsibility"]
"""
input_evaluations = [input_evaluation_1, input_evaluation_2]
output_evaluations = [output_evaluation_1, output_evaluation_2]
Next step includes creating an initial system prompt. This initial system prompt can be something short and straightforward, that GPT4 will start from and build upon. It must contain the essence of what you want the LLM to do, but doesn't need to be very detailed. We will also create a promptTracker object from PromptTrackerClass
class.
init_sys_prompt = """You are an AI that receives an input text. Your task is to output a pythoning string where every strings is the name of a person with what they are associated with"""
promptTracker = PromptTrackerClass(init_system_prompt = init_sys_prompt)
promptTracker.add_evaluation_examples(input_evaluations, output_evaluations)
Next step includes running the LLM once. In this step, the local LLM will take your initial system prompt and evaluation examples, and run the LLM on evaluation examples using our initial system prompt (GPT-4 will look into how the local LLM performs on the evaluation inputs and change our system prompt later on).
promptTracker.run_initial_prompt(llm_model=llamamodel)
We can check the results:
print(promptTracker.llm_responses[0][0])
print(promptTracker.llm_responses[0][1])
In the expression promptTracker.llm_responses[0][1]
, the first [0]
represents the initial result, obtained using system_prompt[0]
– that's our starting point. The [1]
that follows indicates we're looking at the outcome from the second evaluation instance. If you go back to the examples I provided at the first part of the article, you will see that this is how the LLM gives us the output:
```python persons = { "Musk, Elon": "space_exploration", "Hawking, Stephen": "ai_ethics",
"Thunberg, Greta": "environmentalism" } print(f"Elon Musk is known for {persons['Musk, Elon']}")
print(f"Stephen Hawking is known for {persons['Hawking, Stephen']}") print(f"Greta Thunberg is known
for {persons['Thunberg, Greta']}") ``` This Python code creates a dictionary called `persons` where
each key-value pair represents a person and their associated field. The `print` statements then
display the person's name and their field of expertise.
Which is definitely not in the format that we want.
Next we have to create our openaicommunicator
object from OpenaiCommunicator
class:
openai_communicator = OpenaiCommunicator(client=client, openai_model_code="gpt-4-0125-preview")
I am using gpt-4–0125-preview
model. You can see a full list of OpenAI models here .
Now it is time for the main part where we start the refinement process:
openai_communicator.refine_system_prompt(prompttracker=promptTracker, llm_model=llamamodel, number_of_iterations=3)
Feel free to experiment with the number_of_iteration
parameter. In my experiments, I usually don't need more than 3 iterations. GPT-4 is able to craft a perfect system prompt within 3 iterations. What happens in each iteration is:
- We send the outcomes of previous rounds to the GPT-4 model. This includes the system prompts we've tested and the performance of our local LLM when applying these prompts to our evaluation texts.
- Based on this information, GPT-4 proposes an improved system prompt.
- We then apply this newly suggested prompt to our local LLM for another round of testing.
This cycle repeats for the number_of_iterations
specified. Once complete, you're able to review the outcomes of each iteration to understand how the LLM responded to the evaluation texts under different system prompts. For example, if you wish to examine the performance of the model using GPT-4's first suggested prompt on your second test case, simply check:
print(promptTracker.llm_responses[1][1])
To see which system prompt was used for this iteration, you can use the following command:
print(promptTracker.llm_system_prompts[1])
Essentially, our goal is to identify the first instance where the responses from our LLM (llm_responses[i][j]
) align with our expectations. Once we find that moment, the corresponding llm_system_prompts[i]
becomes the golden system prompt we ought to adopt for future use!
In conclusion, what we basically did was leveraging GPT-4 to find a detailed and optimized system prompt for a smaller LLM, in this example a quantized mistral 7b model, using promptrefiner
. You can use any local LLM serving tool with promptrefiner, for example Huggingface models, by creating a class that inherits from AbstractLLM
class. In this process, GPT-4's deep understanding of context and instruction significantly improved the prompt's detailedness and relevance, therefore enhancing the smaller LLM's output accuracy on varied inputs.
Don't forget to follow for more AI content. More hands-on articles are on the way!