Running Python Wheel Tasks in Custom Docker Containers in Databricks

Data engineers design and build pipelines to run ETL workloads so that data can be used downstream to solve business problems. In Databricks, for such a pipeline, you usually start off by creating a cluster, a notebook/script, and write some Spark code. Once you have a working prototype, you make it production-ready so that your code can be executed as a Databricks job, for example using the REST API. For Databricks, this means that there usually needs to be a Python notebook/script on the Databricks file system already OR a remote Git repository is connected to the workspace*. But what if you don't want to do either of those? There is another way to run a Python script as a Databricks job without uploading any file to the Databricks workspace or connecting to a remote Git repository: Python wheel tasks with declared entrypoints and Databricks Container Services allow you to start job runs that will use Docker images from a container registry.
Hence, this tutorial will show you how to do exactly that: run Python jobs (Python wheel tasks) in custom Docker images in Databricks.
*either a syncing process uploads the Git files to the Databricks workspace before code execution or the remote git ref notebook/script is provided for job runs
Why would you want to do this?
You might have a "build, ship and run anywhere" philosophy, so you may not be satisfied with the conventional way of using DataBricks.
Let me explain.
Databricks suggests some CI/CD techniques for it's platform.
Continuous integration and continuous delivery/continuous deployment (CI/CD) refers to the process of developing and delivering software in short, frequent cycles through the use of automation pipelines.
Typically, a commit to the deafult branch or a release starts a pipeline for linting, testing etc. and ulitmately results in an action that interacts with Databricks. This can be a REST API call to trigger a job run in which the notebook/script is specified OR a deployment package is deployed to a target environment, in the case of Databricks, this can be the workspace.
The first option usually needs Databricks to be connected to the remote Git repository to be able to use a remote Git ref, for example, a specific notebook in main branch of a Github repository to trigger the a job run.
The second option uploads files to its workspace but does not necessarily need Databricks to be connected to the remote Git repository. A visual summary for this workflow option is shown here.
Where a deployment package can be a notebook, a library, a workflow etc. The Databricks CLI or the REST API is commonly used to deploy packages to the Databricks workspace. In essence, an automation pipeline syncs the changes in the remote git repository with the Databricks workspace.
My goal for this blog post is to explore a different CI/CD workflow, one in which there is no interaction with Databricks (decoupling the code from the Databricks workspace). The workflow suggested, just creates a Docker image and pushes it to a container registry and leaves the execution of job runs up to the service. This can be anything, a web app, function, a cron job or Apache Airflow.
Please bear in mind that doing it like this is not for all use cases but I think some workloads (e.g. ETL) can benefit from it. Use common sense to decide what fits best to you. Nonetheless, it's worth to explore the options a platform such as Databricks offers. So let's get started.
TLDR
Databricks (standard tier*) will be provisionied on Azure**. A single Python wheel file with defined entrypoints and dependencies will be created using Poetry. The wheel file will be installed in a Databricks compatible Docker image that is pushed to a container registry. Job runs will be created and triggered with the Databricks workspace UI portal and REST API.
*Provisioning the Azure Databricks Workspace with Standard tier should not incur any costs
**alternatives include AWS or GCP
Prerequisites
- Poetry
- Docker
- Azure or AWS Account
- Container registry (e.g. DockerHub, ACR, ECR)
Structure
- Apache Spark & Databricks
- Provisioning Databricks on Azure
- Enable Databricks Container Services
- Create a Personal Access Token (PAT)
- Options to execute jobs runs (Python)
- Create a Python wheel with entrypoints (feat. Poetry & Typer CLI)
- Build a Databricks compatible Docker image
- Create and trigger a job run (UI)
- Create and trigger a job run (REST API)
Apache Spark & Databricks
In the introduction, I already talked about Databricks and mentioned a common use case for data enigneers. But if you need a short definition on what Apache Spark and Databricks is, here you go:
Spark is an open-source engine for processing large-scale data. By distributing the data and the computation to multiple nodes in a cluster, it achieves parallelism and scalability.
Databricks is a cloud-based platform that leverages Spark to run various data-related tasks, such as data processing, data analysis, machine learning, and AI workloads.
Provisionig Databricks on Azure
It is assumed that you have an Azure account and a subscription at this point, if not, create a free Azure account, or just follow along.
Let's provision the Databricks resource (workspace) on Azure. No costs should occur at this stage.
We create a resource group in which we will provision the Databricks resource: databricks-job-run-rg

Within this resource group, we can create the Azure Databricks workspace and give it a name: databricks-job-run

For the pricing tier, select **Standard***. You can leave the rest as suggested. Managed Resource Group name can be left empty.
*Please note that only with Premium, we will have proper Role based access control (RBAC) capabilites. But for the sake of this tutorial, we don't need it. We will use a Personal Access Token (PAT) that allows us to create and trigger job runs using the Databricks REST API.
After the deployment, our resource group now contains the Azure Databricks workspace:

And we can launch the workspace,

which will open a friendly user interface (UI) like this:

So far so good.
Enable Databricks Container Services
Databricks does not allow custom Databricks Docker images by default, we must enable this feature first. The steps for this are described here.
In the Admin Settings (drop-down menu at the top right corner), we must enable the Container Services field:

Also make sure Personal Access Token is enabled, we will create one in the next section.
Create a Personal Access Token (PAT)
In the User Settings (drop-down menu at the top right corner), a button allows us to generate a new token:

Store this token somewhere safe as this can be used for secure authentication to the Databricks API. We're going to need it later.
Please note: We use a PAT, because the standard tier does not come with RBAC capabilites. For this, we would need to upgrade to Premium.
Options to execute jobs runs (Python)
Before we create a Python wheel for a Databricks job, I'd like to focus on the options that we have to create and run Databricks jobs (Python). There are different ways to execute scripts in a cluster.
Creating a task in the Workflows/Jobs or Workflows/Job runs pane, reveals our options. Alternatively, we find out about them reading the docs.

As mentioned earlier, we can basically specify 2 types of sources from where the job gets the notebook/script to be executed: The Databricks Workspace OR a **remote Git repository*. For Python wheels**, we can't select the source, but instead we must enter the Package name and the Entry Point. The wheel package should exist either on a the DBFS (Databricks File System) or an index such as PyPi. I think the tutorial in the docs does a poor job of explaining the source options (May 2023), or maybe I'm just unable to find this information. But there's a neat blog post that shows how to do it: How to deploy you Python project to Databricks
Anyway, it's not really mentioned (even though it makes sense) that if you provide a custom docker image that has your Python wheel task already installed, you can also specify it and it will be executed. And that's what we're going to do.
*For Python scripts, there is also the option: DBFS (Databricks File System)
Create a Python wheel with entrypoints (feat. Poetry & Typer CLI)
I've setup a project with **Poetry*** in src layout, that contains the code and commands to build a wheel. You can find the full code here:
https://github.com/johschmidt42/databricks-python-wheel-job-run
./src
├── dbscript1
│ ├── __init__.py
│ └── script.py
└── dbscript2
├── __init__.py
└── __main__.py
In the pyproject.toml, these scripts are defined as follows:
[tool.poetry]
name = "dbscripts"
version = "1.0.0"
...
packages = [
{include = "dbscript1", from = "src"},
{include = "dbscript2", from = "src"},
]
[tool.poetry.scripts]
dbscript1 = "dbscript1.script:main"
dbscript2 = "dbscript2.__main__:main"
...
And within the two packages dbscript1 and dbscript2, we find some code:
and
They pretty much do the same thing. The only noticeble difference between them is that script1's name is just "script" and the other's name is "main". You will see the impact of this difference in a bit. Both scripts use the typer
** library to create a command-line interface (CLI) for the script1
or script2
function.
When the script1
or script2
function is called with an argument (required), it prints the name of the current file (__file__
) and the value of the passed in argument
parameter.
From these files, we can use Poetry
to create a package:
> poetry build --format wheel
Building dbscripts (1.0.0)
- Building wheel
- Built dbscripts-1.0.0-py3-none-any.whl
which will create a wheel in the dist directory
dist
└── dbscripts-1.0.0-py3-none-any.whl
We can use pip
to install this wheel into a virtual environment to see what will happen:
> pip install dist/dbscripts-1.0.0-py3-none-any.whl
Successfully installed dbscripts-1.0.0
If we restart the shell (bash, zsh etc.), we now have two new functions that we can call:
(dbscripts-py3.9) databricks-python-wheel-job-run % dbscript [TAB]
dbscript1 dbscript2
Both can be used as functions, such as black or isort:
> dbscript1 Databricks
/Users/johannes/learnspace/databricks-python-wheel-job-run/.venv/lib/python3.9/site-packages/dbscript1/script.py
Your argument is: Databricks
> dbscript2 Databricks
/Users/johannes/learnspace/databricks-python-wheel-job-run/.venv/lib/python3.9/site-packages/dbscript2/__main__.py
Your argument is: Databricks
And here comes the benfit of naming the entrypoint function "main":
> python -m dbscript1 abc
/Users/johannes/learnspace/databricks-python-wheel-job-run/.venv/bin/python: No module named dbscript1.__main__; 'dbscript1' is a package and cannot be directly executed
compared to
> python -m dbscript2 abc
/Users/johannes/learnspace/databricks-python-wheel-job-run/.venv/lib/python3.9/site-packages/dbscript2/__main__.py
Your argument is: abc
If you're baffled how both scripts can be executed as functions in the shell, just take a look into your venv's bin directory: .venv/bin
# .venv/bin
.
├── activate
...
├── black
├── blackd
├── dbscript1
├── dbscript2
├── dotenv
├── flake8
...
The codes looks like this:
These are called consol_scripts entry points. You can read up about them in the Python documention.
*Poetry is a dependency, environment & package manager
**Typer is wrapper that is based on the popular Python lib click. It allows us to build CLIs from only Python type hints!
Build a Databricks compatible Docker image
We now have a Python wheel file that comes with two console script entry points when installed. Let's containerize it with docker:
This Dockerfile defines a multi-stage build for our Python application. The Stages are seperated by #----#
in the Dockefile.
The first stage is the base image that uses **Databricks Runtime Python 12.2-LTS*** as the base image and sets the working directory to /app
. It also updates pip.
*We can also build our own base image, as long as we have certain libraries installed: Build your own Docker base
The second stage is the builder image that installs Poetry, copies the application files (including pyproject.toml
, poetry.lock
, and README.md
) and builds a wheel using Poetry.
The third stage is the production image that copies the wheel from the build stage and installs it using pip (We don't want Poetry in our production image!).
We can build a Docker Container from this with
> docker build --file Dockerfile --tag databricks-wheel-scripts:latest --target production .
When going inside (bash), we can execute our console_script as we did before:
> docker run -it --rm databricks-wheel-scripts:latest /bin/bash
> root@bca549fdcb50:/app# dbscript1 some_value
/databricks/python3/lib/python3.9/site-packages/dbscript1/script.py
Your argument is: some_value
Or we do this in one line:
> docker run -it --rm databricks-wheel-scripts:latest /bin/bash -c "dbscript1 hello_world"
Please note, that the docker entrypoint is /bin/bash, as this shell contains the dbscript1 and dbscript2 in the $PATH variable.
This docker image can be now pushed to a container registry of our choice. This can be e.g. DockerHub, ACR, ECR etc. In my case, I choose Azure Container Registry (ACR), because the Databricks workspace is on Azure as well.
To push the image I run these commands:
> az login
> az acr login --name databricksjobrunacr
> docker tag databricks-wheel-scripts:latest databricksjobrunacr.azurecr.io/databricks-wheel-scripts:latest
> docker push databricksjobrunacr.azurecr.io/databricks-wheel-scripts:latest
Create and trigger a Dabricks job run (UI)
In the Databricks workspace (UI), we can create a job (Workflows tab) and define a new cluster for it:

Here, I create the smallest available single node cluster, the Standard_F4 that consums 0,5 DBU/h. In the Advanced options section, we can specify the Docker settings:

So that the cluster can pull the image from the container registry. We provide a container registry username and password but we could also use the "Default authentication" method (e.g. Azure).
In the jobs UI, we can then create a job:

where we define the package name and entry point.
Please note, in the UI, we create a job first and then trigger it for a job run. The REST API allows us to create and trigger a one-time job run with one call only! We'll see this in the next section.
In the Job runs tab, we can see the status of our job run:

In a matter of minutes, the cluster was ready and ran our console script in the docker image of our choice:
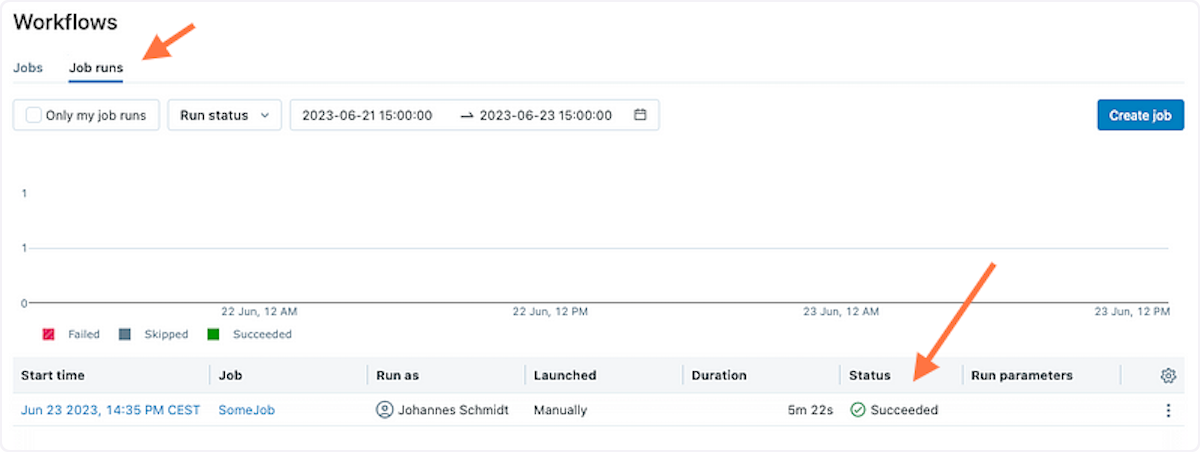
We can get the logs (stdout, stderr) by clicking on the run:

Fantastic!
Create and trigger a job run (REST API)
A service such as an Azure Function cannot use the UI to initiate job runs. Instead, it has to use the Databricks REST API (Jobs API 2.1). We could use Airflow's Databricks Connectors to do this, but writing some Python code that sends a single request to the REST API is probably faster to set up. So let's to write some Python code that allows us to create and trigger job runs. I will enclose this code in a class called Databricks service:
The script has two components: a SecretsConfig
class and a DatabricksService
class.
The SecretsConfig
class is used read and store config settings and secrets such as Databricks URL, Databricks Personal Access Token (PAT)*, Azure Container Registry (ACR) username and password. These are the same basic parameters that we had to specify using the UI.
*If you have Databricks deployed with the Premium Tier, you don't need to use the PAT, but can get yourself a token with OAuth.
The DatabricksService
class is used to interact with the Databricks API. It allows to create and trigger a one-time job run using an existing cluster or a new cluster. The API documentation can be found in the jobs API 2.1. The service itself has only two variations of the same call to this submit endpoint: The create_job_run_on_existing_cluster()
method is used to create a job run on an existing cluster, while the create_job_run_on_new_cluster()
method is used to create a job run on a new cluster.
Let's briefly examine the create_job_run_on_new_cluster()
function:
The method takes several arguments, such as _imageurl, _packagename, entrypoint etc and calls the submit endpoint to create and start a job run on a new cluster.
The python_wheel_task_payload
dictionary is used to specify the package name and entry point of the Python package to use. The positional and named arguments are also specified in this dictionary if they are provided.
The cluster
dictionary is used to specify the cluster settings for the new cluster. The settings include the number of workers, Spark version, runtime engine, node type ID, and driver node type ID.
Having that, we now need some code to call the Databricks REST API using our DatabricksService:
After running the script, we observe that we get a status code 200 back and our job run completes successfully after a while:

And we see the output:

Easy!
If we wanted to use an identity to access resources in Azure, we'd provide the credentials of a service principal as environment variables when calling the submit endpoint (new cluster!).
Conclusion
Running Python jobs in custom Docker images in Databricks is not only possible but also practical and efficient. It gives you more flexibility, control, and portability over your code and workflows. You can use this technique to run any Python script (or any other code) as a Databricks job without uploading any file to the Databricks workspace or connecting to a remote Git repository.
In this short tutorial, you've learned how to create Python wheel tasks in custom Docker images and trigger job runs with the Databricks UI or the REST API.
What do you think of this workflow? How do you run your Python jobs in Databricks? Let me know in the comments.