Shifting Tides: The Competitive Edge of Open Source LLMs over Closed Source LLMs
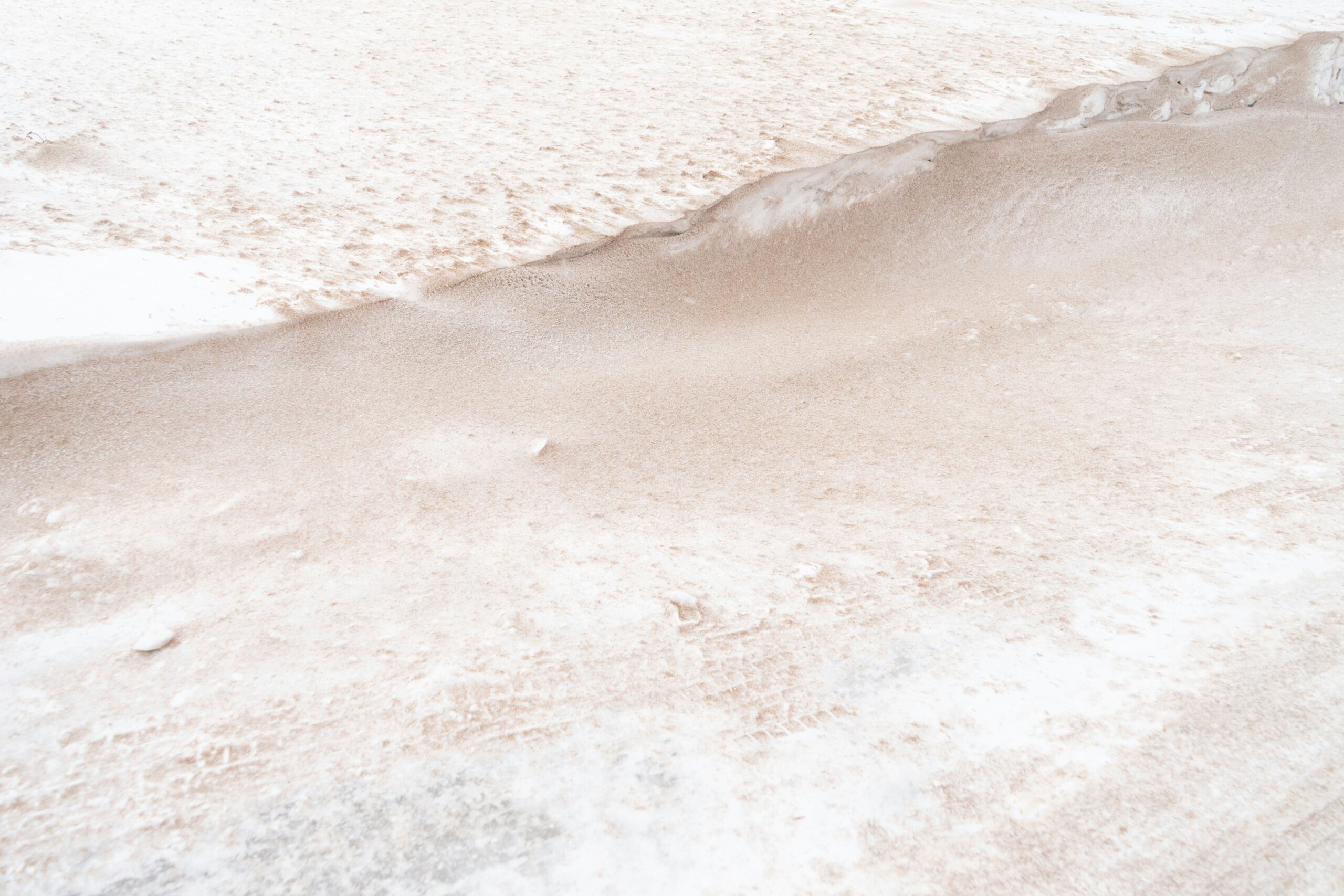
Since the release of ChatGPT has sparked developers' interest in building applications with Large Language Models (LLMs), proprietary closed source foundation models, especially by OpenAI, have been dominating the market. OpenAI's foundation model, gpt-3.5-turbo, which powers ChatGPT, is often the default LLM in programming tutorials. A survey conducted by a16z [1] in 2023 among 70 enterprise AI leaders showed that roughly 80% of enterprise market share was closed source – with the majority going to OpenAI.
However, smaller open source models have been gaining popularity and could soon replace larger closed source ones. Mainly because the capabilities of open source LLMs are catching up. The main advantage of closed source models, performance, is now quickly diminishing. Additionally, the aforementioned survey respondents listed other factors, such as data security, customizability, and cost, which are making open source LLMs a more attractive alternative to closed source LLMs in enterprises. Some enterprise AI decision makers are even targeting a 50/50 split for the coming year, according to the same survey [1]. Thus, we can already expect a significant shift toward use cases deployed on smaller open source models in 2024.

Performance
Closed source proprietary foundation models have been outperforming open source models so far, but this main advantage is diminishing quickly. While proprietary models were more capable than open source models at first, open source models have become more advanced, and the performance gap is closing quickly. This is because the barrier to entry for training and experimentation has dropped from large research organizations to smaller research institutions and even to individuals. Because cost-efficient fine-tuning strategies, such as Low-Rank Adaptation (LoRA), have been developed, making high-quality foundation models publicly available makes it possible for almost anyone with an idea, some time, and a performant laptop to generate and distribute a new variant of it.

A great example of how quickly open source models are closing the performance gap is Meta's Llama model. In March 2023, Meta decided to open source the Llama model. This has propelled advancements in the model's capabilities: Within a month of Llama's release, a community of tinkerers and researchers had improved the model and created various customized versions, many of which were built on top of each other. Almost one year later, Meta released Llama 3, which now ranks among the top 10 on the LMSYS leaderboard.
Data security
Open source models can be self-hosted and thus offer a clear advantage of control and data security, which is especially important for enterprises. Productionizing Generative AI applications in enterprises requires addressing data security concerns for sensitive use cases. Especially businesses operating in heavily regulated industries, such as banking or health care and life sciences, might even have regulatory requirements. Thus, many enterprises aren't comfortable sharing their sensitive or proprietary data with closed source model providers, especially if it isn't guaranteed that the data will be not used to re-train the LLM, thus risking data leakage.
These concerns can either be addressed with virtual private cloud (VPC) environments or an entirely local self-hosted environment. While many closed source model providers offer VPC integrations, self-hosting is only possible with open source models. However, the rapidly increasing interest in frameworks for running LLMs locally, such as Ollama, is already the first sign of developers building local pipelines.

Customizability
Another factor to be considered is the customizability of foundation models through fine-tuning to a specific industry, business, or use case. In the future, we can expect that most organizations will develop customized models to improve accuracy and reduce latency and costs by reducing the number of required tokens, thus making the solution more scalable. While there are customized models that are trained from scratch, such as BloombergGPT, for financial use cases, this is not a feasible solution for many organizations. Developing so-called foundation models requires technical expertise and computational resources, which are often only available to large research institutions. Instead, fine-tuning a pre-trained foundation model for a specific use case by adding stackable improvements, such as instruction tuning or LoRA, is a more feasible approach.
While many proprietary models can be fine-tuned, open source models offer more flexibility than closed ones. When fine-tuning open source models, you can even use smaller, more lightweight LLMs, which can achieve similar performance as large proprietary models after being fine-tuned to a specific use case. Additionally, smaller models can be iterated upon more quickly during the development of LLM-powered applications, thus reducing time-to-market.
Cost
Lastly, open source models can be more cost-effective than closed source ones, especially for production use cases at scale. While the main cost factor of closed source models is inferencing costs, it is the cost for self-hosting for open source models, which are generally free to use. Although the setup and maintenance of a self-hosted infrastructure can initially lead to higher costs, it can be more cost-effective at scale. Additionally, being able to utilize smaller fine-tuned models gives enterprises more control over hosting costs because they are less resource intensive and can thus reduce cost.
On the other hand, the inferencing costs for proprietary models increase linearly with utilization. Being exposed to the risk of unexpected changes in pricing policy could also be a reason why enterprises often want to avoid lock-in with closed source model providers, according to the a16z survey [1]. While "getting an accurate answer is worth the money," as one respondent in the a16z survey [1] stated, it is clear that proprietary models will become less attractive when free, unrestricted alternatives are available.

As a blog post on Hugging Face has called 2023 "a year of open releases", we can already see the first signs of closed source model providers pivoting in strategy. As Meta's release of Llama 3, which ranks among the top 5 on the leaderboard after its release, proves that model providers can benefit from open sourcing their models. As OpenAI is still leading the leaderboard with GPT-4, we have not seen any move from them towards open sourcing their models. Instead, they have enabled the customization of smaller models. Meanwhile, Google is trying to replicate what Meta has done with its Llama model. As the leaked internal Google memo [2] foreshadowed, Google aims to establish a platform where innovation happens to cement itself as a thought leader and direction-setter. As a result, we can see that Google has released an open source alternative, Gemma, alongside their proprietary Gemini model. Additionally, they have pushed its development via Kaggle competitions to get a community to publish variants of Gemma.

To summarize, we can expect a shift in popularity from closed source models towards open source models in the near future. As discussed, the four key factors are performance, data security, customizability, and cost.
Enjoyed This Story?
Subscribe for free to get notified when I publish a new story.
Find me on LinkedIn, Twitter, and Kaggle!
References
Literature
[1] Sarah Wang and Shangda Xu in Andreessen Horowitz (2024). 16 Changes to the Way Enterprises Are Building and Buying Generative AI. (accessed April 20th, 2024).
[2] Dylan Patel and Afzal Ahmad in (2023). Google "We Have No Moat, And Neither Does OpenAI".
Images
If not otherwise stated, all images are created by the author.