Simplify Your Machine Learning Projects
Many businesses are eager to adopt Machine Learning to improve their products and services. However, many data scientists get too caught up in creating the perfect model and using state of the art techniques. By doing this, they forget the most important thing: delivering a functional minimum viable product (MVP). In this post, I'll discuss three reasons why it's better to focus on getting a working MVP first before spending too much time on creating a complex model. To end the post, I give three tips for creating a MVP.
When I started as a data scientist around six years ago, I wasn't really interested in topics like Naive Bayes, linear regression, and statistics. Maybe it was because of my mathematical background, and I had already learned these topics during my studies. Instead, I was way more interested in neural networks, language models, computer vision, and reinforcement learning. These topics intrigued me, and I took courses to learn them as quickly as possible. When I had to deal with real business problems at companies, I always tried complex models and solutions that I found fun to work on, often involving deep learning, datasets scraped from the web, and complex architecture. Unfortunately, my code was messy and hard to read.
I remember one project that I spent months working on. I had weekly meetings with the business, but I was the one who was talking, and the end result was too complex and hardly used. Important takeaways from that period are to not overcomplicate machine learning solutions and to talk less. With this post, I hope I can prevent you from making the same mistakes I made and explain what to do instead.
Don't focus on complex techniques
Why shouldn't the focus of a project be on using complex techniques? In my opinion there are three main reasons, which I will explain here.
Reason 1. The business doesn't care
The first and most important reason is that the business doesn't care! Your stakeholders are not interested in the technical details of your model. Whether you used boosted trees or a neural network, to them, it's all the same. What they want to know is how your model is helping them achieve their business goals. If the model needs to be retrained often, you can justify your decision to use a simple model like logistic regression over a neural network because it's super fast to train.
Often, the main goal of a machine learning model is not to reach 100% accuracy. Instead, a machine learning model helps with business processes. Spending too much time optimizing the model will delay the time it takes to deliver a working product to the market. It's better to create an MVP, ensure it meets the business requirements, and get it into production. It's essential to take not only performance but also interpretability, computation speed, development costs, robustness, and training time into account. These factors are important too and can be as relevant to business people as performance.
Besides yourself, there are other people who care about a complex model and state-of-the-art methods. Those people are often researchers or data science colleagues. If you work too closely with them instead of with the business, you can get to the point where you believe modeling is the main goal. To overcome this, try to work closer with business people. Demo your product after every new feature implementation and ask the business if your assumptions are correct. Decisions that seem small can be really important for business people.
Reason 2. A complex model adds less value than a working MVP
The more time you spend on the model, the less time you have for good engineering principles, such as writing modular code, testing, architecture, logging, and monitoring. Setting these things up in a good way at the beginning saves a lot of time later. You can easily add new features to a solid codebase. This is more valuable than having a complex model in a Jupyter Notebook that performs slightly better but doesn't run in production. Another benefit of a simple model is interpretability, which can help convince stakeholders because they can see the predictions make sense.
Especially in the beginning, focus on creating a product that works and has robust code and a well-crafted CI/CD pipeline. This makes it easier to improve the solution later on. If the business doesn't feel the urge to improve the current solution, you can move on to another project. You didn't waste your time creating a ‘perfect' model.
What relates to this is the Pareto principle. It is a rule that states that 80% of results can be achieved through 20% of our efforts (aka the 80/20 rule). Often, creating a complex model that performs slightly better than a simple model doesn't fall into the 80% of the results but is a task that is hard and takes a lot of time. The complex model is that last hard-to-reach 20% that takes 80% of the effort. Before you start, convince yourself it is worth it.
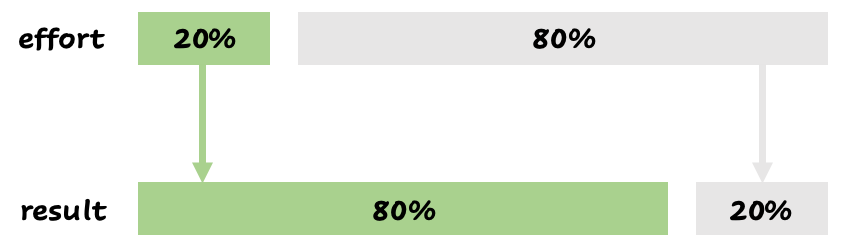
Reason 3. Complex projects require more maintenance
The more complex the project, the more resources and time are needed to maintain it. This means that you'll spend more time fixing bugs, optimizing the model, keeping the data up to date, and less time adding new features or improving the product. A simple project, on the other hand, requires less maintenance, which means that you can spend more time iterating on the MVP and adding new features to improve the product.
An important thought to keep in mind is that the best solution is often the simplest solution that fits the requirements. This can help you determine if that deep learning state-of-the-art model is truly worth the extra work that comes with it! If there are two models that perform equally well, and one is simple and the other is complex, go with the simple one.
One example from my work at a company: I tried to solve a scheduling problem with reinforcement learning. It was quite complex, and we were progressing slowly. The business became a bit annoyed and disappointed because we couldn't show good results. When we switched our solution method to (good old) mathematical optimization, it went much faster! It was less interesting, but we gained the trust of the business and could implement new features and constraints easily.
Creating an MVP
It can be hard to decide what should be covered by the MVP. Here are three tips to help you determine (and protect) the scope.
Tip 1. Questions you need an answer to before writing code
Before writing code, start with the following questions. Make sure you get answers from business experts and that everyone involved is present at this meeting.
-
Why should the product be built? This question shows the importance of the product. With this question, you can start thinking about metrics to calculate the value of the product.
-
What should the product do? Rather than focusing on the "how," you should focus on the "what." The "how" of the new product is something you can figure out later within the data science team. For now, you should focus on what the business finds important and what they think the output should be. Don't forget to ask what they think a perfect solution would look like. This can help determine must-haves and nice-to-haves. For the MVP, you need to focus on the must-haves.
-
How is it done right now? The business experts can tell you all about it! You can use this information to determine critical features for the product.
- Who is the business expert to reach out to?Besides engineers and data scientists, at least one business expert should be involved. If possible, an end-user who knows exactly what the product should do in the end. You need a person you can go to at any time during development to help you make the right decisions.
These questions are the bare minimum but are often overlooked. Directly jumping to the "how" and focusing on ML techniques without listening to what the stakeholder wants is a recipe for project failure. Make sure to listen without interruption and don't flag product features as impossible during this meeting.
Tip 2. Create a living document with functionality and ideas
A document containing all ideas and a diagram with functionality that is understandable for everyone is a great way to communicate with different stakeholders. It can be updated as the project progresses, and you can keep track of features and add new ones. The diagram shouldn't be too technical or architectural, but instead, it should contain all business features in plain English (or any other language, of course). Below is a simple example of a diagram for a chatbot:

Tip 3. Don't change the scope before the MVP is delivered
If you work with creative and inventive people, and probably you are one yourself, you might find it hard to stick to the initial plan. You showcase your product and people can respond: "Wouldn't it be cool to add a computer vision feature here?" You think, "Hell yeah, that sounds fun!" But that is the moment where the problems begin because before you know it, you are building a complex system, and it becomes really hard to deliver on time. A better way to handle this is to say thank you and note it down. After MVP delivery, you can revisit those ideas and decide if you should go for it. You can also decide to set time limits on the experimentation phase. This can help you focus on the core problem and try only a certain number of strategies. After the experimentation phase, you can continue with the most successful solution.
Conclusion
While creating a complex machine learning model may seem like the best way to deliver a high-performing product, it's better to focus on delivering a working MVP first. A working MVP demonstrates the value of your product to stakeholders, and it's easier to iterate on a simple model. Remember that the business cares more about how your product can help them achieve their goals than the technical details of your model. Therefore, focus on delivering a working MVP that meets the requirements, and worry less about creating the perfect machine learning model.
And to nuance this post a bit: of course there can be exceptions, e.g. when performance is the main business goal, or when ML experts with experience in the type of problem all agree a new solution should be created. In that case, lucky you! You can try all the cool new stuff that is out there.
Related
These are the Steps You Need to Take to Create a Good Data Science Product
Three Essential Soft Skills for Practical Data Scientists
How to Create a Web App in Minutes that Makes Predictions and Explains them