Small Training Dataset? You Need SetFit
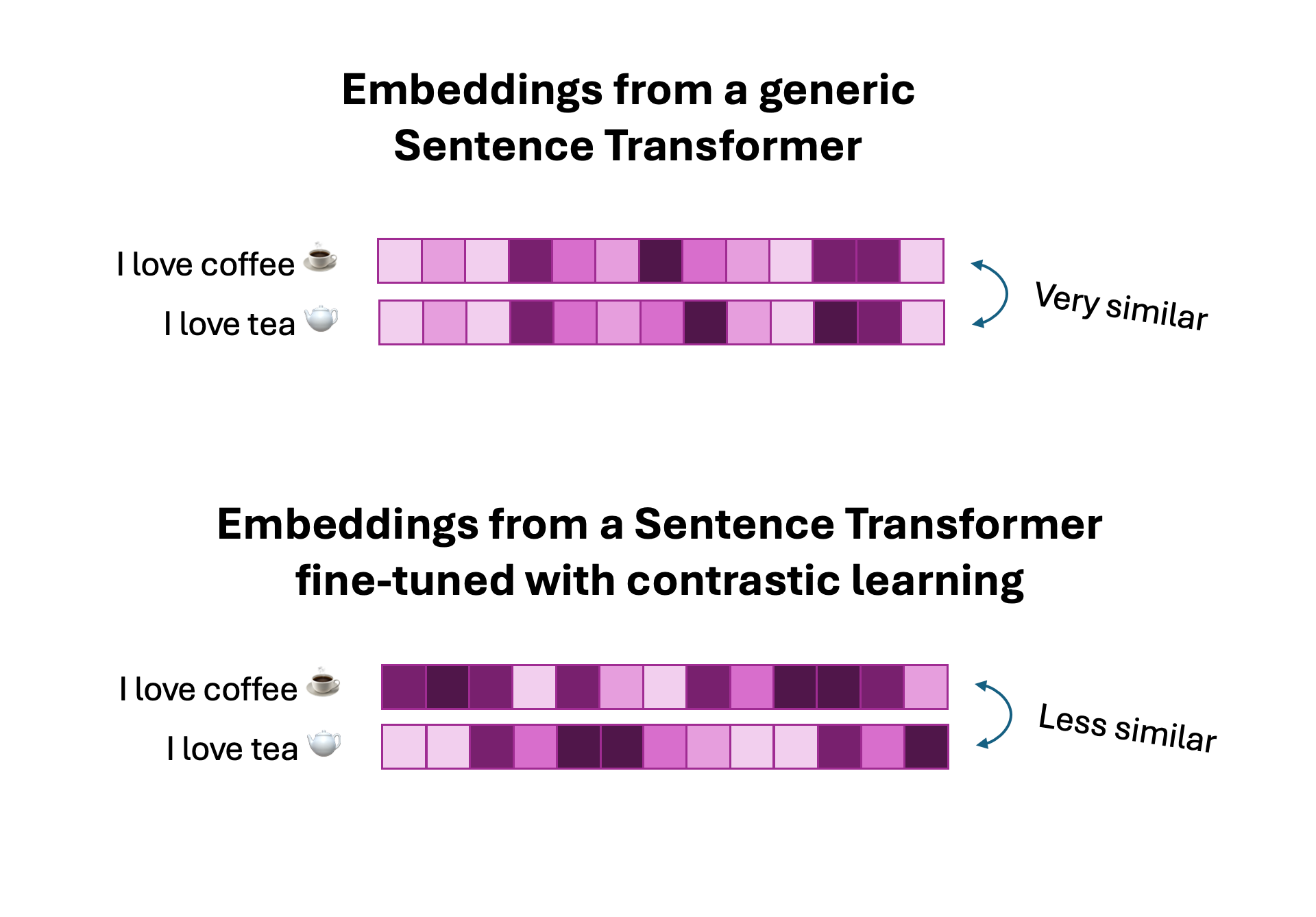
Data scarcity is a big problem for many data scientists.
That might sound ridiculous ("isn't this the age of Big Data?"), but in many domains there simply isn't enough labelled training data to train performant models using traditional ML approaches.
In classification tasks, the lazy approach to this problem is to "throw AI at it": take an off-the-shelf pre-trained LLM, add a clever prompt, and Bob's your uncle.
But LLMs aren't always the best tool for the job. At scale, LLM pipelines can be slow, expensive, and unreliable.
An alternative option is to use a fine-tuning/training technique that's designed for few-shot scenarios (where there's little training data).
In this article, I'll introduce you to a favourite technique of mine: SetFit, a fine-tuning framework that can help you build highly performant NLP classifiers with as few as 8 labelled samples per class.
I first learned about SetFit on a project I delivered for a client in the financial sector
We were trying to build a model that could classify domain-specific texts which had only subtle differences between them. Unfortunately, we had only around 10 samples per class (and ~200+ classes), and weren't having much luck with our arsenal of traditional NLP tools (TF-IDF, BERT, DistilBERT, RoBERTa, OpenAI/Llama 3).
While searching for a solution, I came across SetFit and decided to try it out after seeing this remarkable graph, which shows the results of an experiment run by the original creators of SetFit:

In the experiment, the researchers trained a RoBERTa Large model to classify the sentiment of customer reviews. They started with a training dataset of just 3(!) samples per class/sentiment, and gradually increased the size of the training dataset up to the full sample of 3k, recording the accuracy at each interval. As you can see from the graph (the orange line), the RoBERTa model was highly performant with a large sample size, and terrible at small sample sizes.
Next, the researchers trained a series of models using the SetFit framework (the blue line). And they found that:
SetFit-trained models easily outperform RoBERTa Large with small sample sizes
This blew my mind, but, to understand why it happens, we need to get a grasp on what SetFit actually is.
SetFit is a framework for few-shot fine-tuning of Sentence Transformers (text embedding models). It was developed by researchers at HuggingFace