The 4 new trendy AI concepts, and their potential in digital products

Headlines keep buzzing with updates on cutting-edge new model versions of Large Language Models (LLMs) like Gemini, GPT, or Claude. In parallel to all this core AI progress, there is also a lot of discovery and work from many other companies on how to actually leverage these models to innovate, bring further value, and reduce costs. It is easy to feel overwhelmed and pressured to keep up with all this progress, I can say it happens to me a lot! In this blog post, I'm packing some of the most important concepts and their potential to products and companies to help you keep up.
There are some common trendy concepts around how companies are achieving the integration of LLMs and other GenAI models into their products or processes. These concepts are: prompting, fine-tuning, retrieval augmented generation (RAG), and agents. I'm sure you'll have heard about several or all of these concepts before, but I feel sometimes the differences between the concepts are unclear, and most importantly we are still unaware of the potential they can provide to our companies or products.
In this blog post, we'll have an overview of each of these concepts, with the aim that by the end of it you understand what they are, how they work, the differences between them, and their revolutionary potential for companies or digital products. There is no better way to understand a technology's potential than through analyzing its use in specific examples. That's why I'll walk you through these concepts pivoting around one single use case -publishing an ad in a marketplace- to illustrate how each of these trending concepts can be leveraged to generate further value and efficiency.
The use case
In most marketplaces, users are able to publish ads or products, and the platforms provide a standardized publishing process. Let's consider the scenario where this process involves multiple steps:
- "Publish new item" button: signals the user's intent to list an item, and initiates the publishing process,
- Information tab: users are asked to provide specific details about the item. Let's imagine in this case the user is asked to provide a title, description, category, picture, and price.
- "Confirm publication" button: finalizes the publishing process, submitting the item for publication in the platform.

Up until now, users have navigated through these steps manually, inputting information as required. This can be time-consuming and even confusing for new users, who might ask: what's the appropriate price? Which category best fits my item? Marketplaces have a clear opportunity to make these publishing functionalities faster and less confusing to users.
Using LLMs through prompting
Prompting is the process of structuring an instruction for an LLM to obtain the desired generated results from it.
By now, many people are already familiar with prompting: using ChatGPT on OpenAI's website is a good example of it. As users, we input a specific question to ChatGPT, like "Write me a rap song about how LLMs will change digital products". OpenAI constructs this input within a prompt and feeds it into the GPT-x model to generate a response. This construction allows OpenAI to manage responses: how the tone should be, decline to answer questions that need recent information, warn about the dangers of nuclear weapons if asked how to produce them, or concatenate all the previous conversation for responses to take into account all of it and not just the last input from the user.
So, how can prompting enhance our "Publish new item" use case? By integrating an API call to a LLM into our functionality, we can construct prompts based on specific user inputs. For instance, we could ask the user to simply input the title of the new item, and construct a prompt to generate appropriate content to fill the description and the category of the publication. If the prompted model is multimodal (accepts multiple types of input data, text, images, sound…), the constructed prompt could include the picture of the product, allowing for more accurate descriptions and categories.

We would need to play with the prompt (prompt engineering) in order to obtain results that are accurate and useful. A nice resource to learn about prompt engineering can be found here.
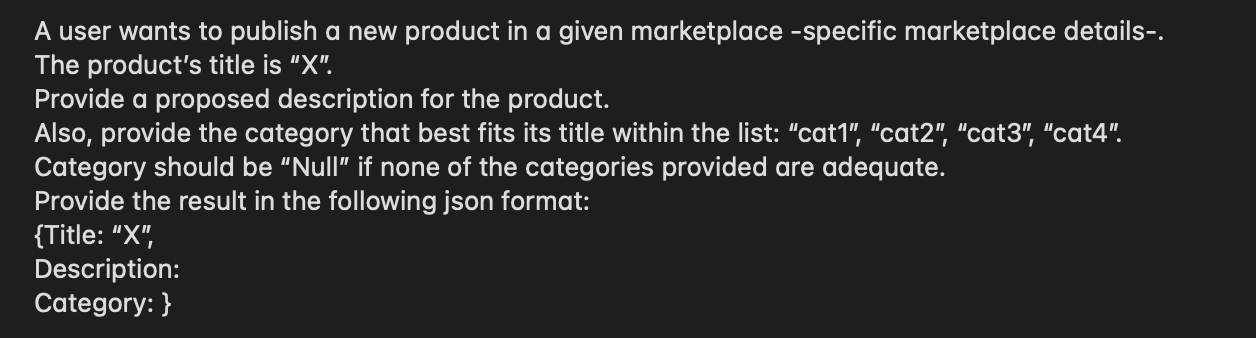
While there are a lot of techniques to improve prompts, such as one-shot / few-shot learning, chain of thought, and ReAct, it's crucial to acknowledge that generated text will never be perfect. Issues like hallucinations, inaccuracies in descriptions or classifications, inappropriate tone, and general lack of context from the marketplace and the product itself can arise.
Because of these limitations, it will be important to enable users to review and edit the suggested information from the system. Still, this new functionality can already provide added value: accelerates the writing process, mitigates the "white page syndrome", suggests a relevant category for the item, and improves the quality of listings of the marketplace.
Using LLMs through fine-tuning.
While improving a prompt can bring better results, there might be a point where further refinement is necessary. This is where fine-tuning can be a good option, as it allows us to adapt the model to the context of our marketplace, by feeding it data from it (e.g. all the history of product information published in our platform). This way, we enable it to generate text that aligns more closely with the linguistic style, and communication norms within the platform.
Fine-tuning is the process of taking a pre-trained model and refining it further with specific datasets or tasks, to adapt better to specialized contexts or domains.
Fine-tuning an LLM is not as straightforward as prompting, and it requires the expertise of a Data Science team to implement the fine-tuning and deploy the fine-tuned model in production, as well as curated data from the context to feed it. As the model needs to be deployed in-house, costs can also vary (and grow) compared to the costs related to querying an API.
Fine-tuning can be done by applying methods that update all the weights of the initial model. However, there are also more cost-effective approaches, Parameter Efficient Fine Tuning (like LoRA or QLoRA). A really good, hands-on resource to learn further about fine-tuning is the course "Generative AI with Large Language Models" by deeplearning.ai. Once the fine-tuned model is deployed, the workflow remains similar to the prompting schema we saw before. The only difference: we now run the fine-tuned model instead of the base model.

Through fine-tuning we bring added value to users, as the generated descriptions and categories are likely to be more accurate and valuable, requiring less manual editing. As the fine-tuned model has seen marketplace data, it could even be that the model is able to suggest a price item that makes sense. In this case, though, it is important to balance the benefits of fine-tuning with the increased costs and complexity of the solution.
Retrieval augmented generation
Both prompting and fine-tuning encounter limitations inherent in the model's cutoff, with it's knowledge confined to the data available up to the point of its training or fine-tuning. This was seen as a huge limitation for LLMs, leaving users wondering about their utility if they couldn't access information real time, or details on products currently available in their marketplace. Retrieval Augmented Generation (RAG) addresses this limitation.
Retrieval Augmented Generation (RAG), is a technique that retrieves relevant information to enrich the prompt.
In practical terms, imagine in our example the user inputs "Harry Potter book 1, almost new". From this input oyr system would take the following steps:
- Search results in the marketplace for "Harry Potter Book 1, almost new"
- Obtain the most relevant results. This step can be done through semantic search, or through whatever sorting logic the marketplace has implemented. Let's suppose these results are other examples of Harry Potter first book and some examples of other books of the series.
- Construct the prompt, by enriching a predefined template with all the information available from the retrieved relevant results.

Through this enriched prompt, the LLM is now able to generate a more valuable description, one that might include specific characteristics of the Harry Potter book obtained from similar products. Since it also has access to pricing data from comparable items, the model may even be able to suggest an appropriate price for the new item. It has also been proven RAG mitigates hallucination risks of LLMs (reference). To deep dive on how RAG can be implemented, a recommended short course is "Building and Evaluating Advanced RAG Applications", also from deeplearning.ai.
Agents
Up until now, we have seen the potential of prompting, fine-tuning and RAG to facilitate and bring quality to the ad publication process. However, two minor limitations persist:
- Lack of price explainability: the model generates price autonomously, offering little insight or control over the result
- Manual process: the user still needs to input, confirm, and navigate manually through various steps.
Agents are processes based on LLMs that can proactively take decisions, access tools, and execute actions.
With agents, we'd be able to introduce an additional layer of autonomy to our system, enabling it to plan, access tools, and execute actions. This translates into equipping our system with the capability to compute prices. Rather than relying solely on model-generated process, agents can access runnable environments or calculators to compute the suggested price based on a logic that makes sense (e.g. mean of all prices of similar items). Moreover, we could further automate the publishing process, by having the agent directly access the publishing API. For further information about agent strategies, I recommend checking these posts from The Batch.
Wrapping it up
In this blog post, we've gone through the trendy AI concepts shaping today's innovation: prompting, fine-tuning, RAG, and agents. We've covered what each concept means, exploring relationships and differences, benefits and downsides, while focusing on their transformative potential.
Prompting is the most straightforward way to bring value through AI-generated suggestions. Fine-tuning takes it a step further, customizing models to better suit the company or product's context and deliver more accurate results. RAG breaks down the barriers of knowledge cutoff, enabling the system to access information in real time to enrich the generated suggestions. And finally, agents introduce a new level of automation and task execution.
Understanding wheter these functionalities improve user satisfaction, reduce user pains (like friction, confusion), and impact product metrics is key to learning, iterating and truly bringing value through innovation. UX surveys can give qualitative feedback on how users perceive the new features. Are users finding the ad creation process more intuitive? Do they find the suggestions relevant? Moreover, a/b testing and checking on specific product metrics can help quantify this impact. How much time do users need to publish a new item? How many users start the publishing process and finalize it? How many users edit the suggestions placed by the system? Has AI-generated content better quality or conversion than other content?
By analyzing and monitoring this impact, we can validate the effectiveness of our AI products, and identify areas for further optimization and innovation. Bear in mind that we have only covered the opportunity, but not related risks to these solutions: hallucinations, security issues, bias, misalignment… It is important to be aware of these risks when implementing Genai solution (I'm thinking about writing about this next!).
This is just the beginning of how GenAI can be leveraged by companies and digital products. Hopefully, this post was useful in understanding the current usage of this technology and generating some ideas on related use cases where LLMs can be valuable.