The Future of Generative AI is Agentic: What You Need to Know
Free link => Please help to like this Linkedin post
1. Introduction
As usual, I helped my daughter with her Chinese homework during this weekend. I discovered a poem that beautifully mirrors the evolving generative AI agent. The poem titled "人有两件宝" or "We Each Have Two Treasures," elegantly reflected the essence of the large language model Agent concept at its core. The poem speaks of two treasures every person possesses: our hands and brains.
Our hands represent the ability to act – to manipulate tools, craft objects, moving things. Our brains symbolize the capacity for thought, reasoning, planning, reflection, and Memory.
The duality of action and reflection is fundamental in Generative AI agent technologies, much like using hands and brains described in my daughter's Chinese poem:
"If hands alone should do a task,
Without some thought, it's too much to ask.
And brains alone, if they're all we use,
Without our hands, what good are clues?
But use them both, together, strong,
In harmony, they can't go wrong.
With hands and brains, side by side,
There's nothing we can't do with pride!"
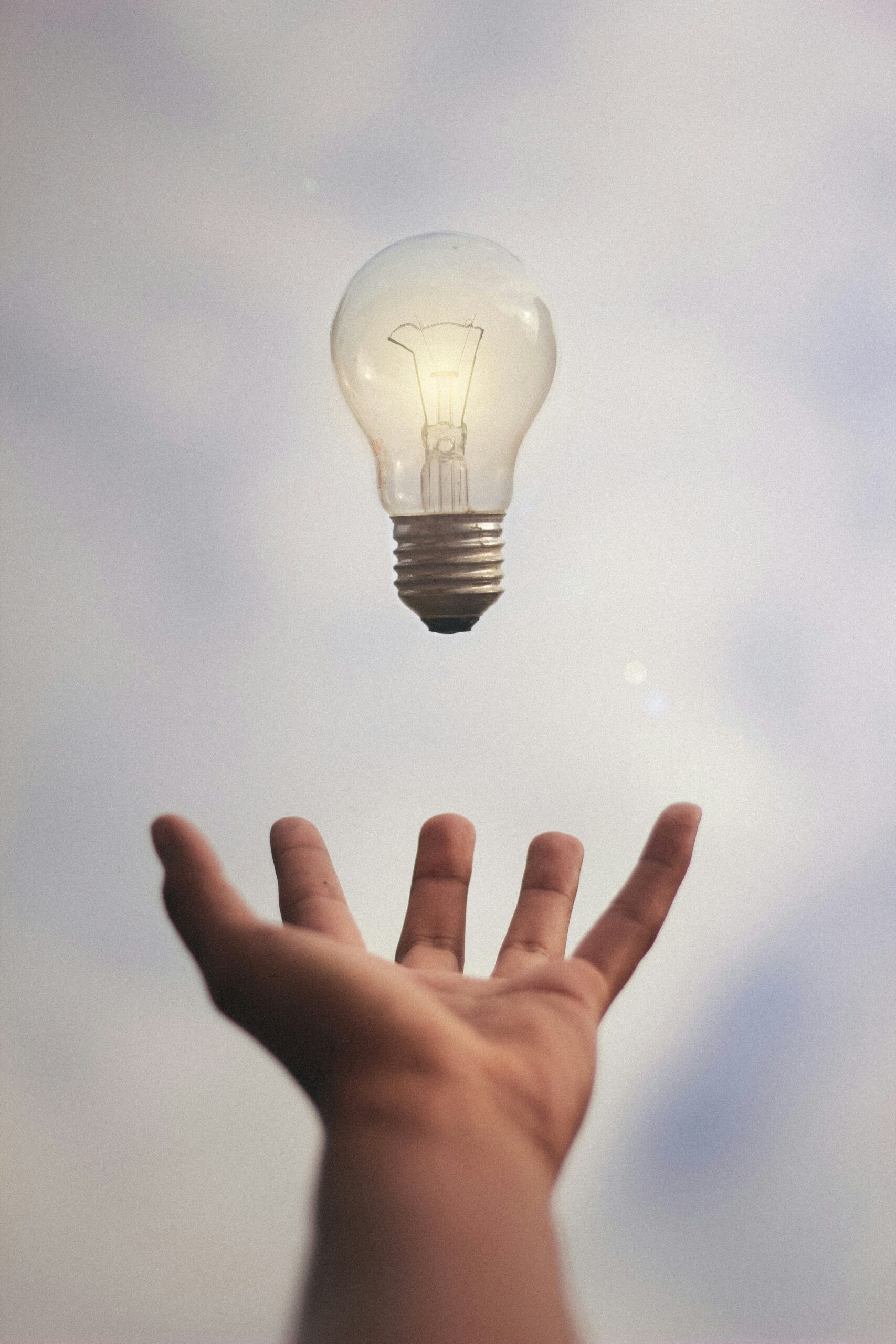
In this article, we will discuss basic concepts of agent technologies, exploring how they plan, utilize tools, incorporate memory, and collaborate within multi-agent systems to enhance their capabilities. By understanding these elements, we can appreciate how agent-based systems are moving forward on the frontier of what's possible in AI.
2. Agent Framework Overview
The agent framework is composed of planning, tools, and memory. Each plays a critical role in the agent's operation and success.
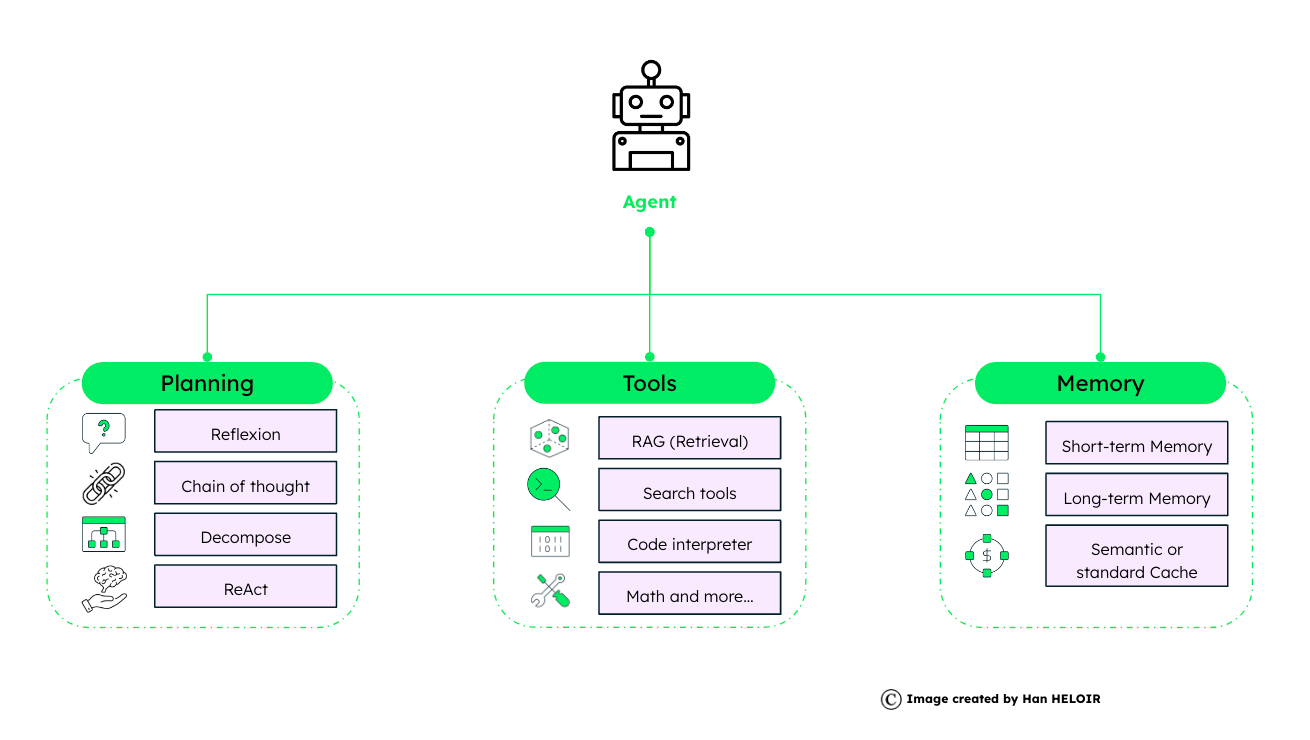
2.1 Planning
Planning is a strategic element for agents, through planning agents determine the steps to achieve a goal. There are several techniques:
Reflexion: Agent think about feedback they get from tasks, then write down their episode memory to help them make better decisions in the next steps. This is a self-improvement mechanism within the agent, allowing it to learn from past actions, reflect on outcomes, and make better decisions in future tasks.
Chain of Thought: We can leverage the prompting technique to ask the Large language model to build a step by step thinking, which is very similar to human-like reasoning, to reach a conclusion or answer.
Recent scholarly works like "Tree of Thoughts" and "Algorithm of Thoughts" have proposed prompting strategies by employing tree-based or graph-based data structures for context management which reduces the number of prompts required.

Decompose: the agent can break down complex problems into smaller, more manageable parts. From there, we can leverage different tools to handle modular issues.
ReAct: this process combines reflection(reasoning) and action, enabling the agent to iteratively think, act, and observe to solve complex tasks dynamically.
This concept is elaborated in the paper "ReAct: Synergizing Reasoning and Acting in Language Models" which is already implemented by LangChain and LlamaIndex in their agent framework.
2.2 Tools
Once we have plans to solve our problems, tools are the functional aspects that enable agents to carry out those plans.
Retrieval / RAG: RAG stands for Retrieval Augmented Generation, a tool that enhances the agent's response by integrating external data, external information from a vector store, or any large corpus of data.

Search Tools: Various utilities can be used by agents to navigate and retrieve information, aiding in their decision-making processes. Such as Wikipedia, Tavily
Code interpreter: external tools that help agents to better understand and execute code which is important for developing generative AI apps.
Math tool: tools dedicated to mathematical computations.
Custom tools: We can leverage any external functions or external API endpoints in custom tools which opens large possibilities to agents.

2.3 Memory
The agent's memory has two categories: short-term and long-term memories.
Short-term Memory: In context memory allows the agent to utilize the short-term memory of the large language model to operate the original problem from the beginning. This capability allows the agent to hold temporarily the information and process it when it is necessary for the task execution.
Long-term Memory: The ability to retain and recall information after the end of the conversations. We leverage often an external database to extend this knowledge which could be precious for further knowledge learning.
Semantic or standard cache: as an extension of long-term memory, it is possible to store the pair of instructions and LLM answers in a database, a vector store, or a database that has vector capability. Before sending the next query to LLM, the agent could check the cache to accelerate the response time and reduce the cost of calling API-based LLM.
3. Agent implementations with accelerators
As people realize that agent is the future of Generative AI, many technical stacks, and clouder propose their way of building AI agents. In this section, let's walk through some main technical stacks and what they propose.
3.1 Agent with LangChain
Planning and Execution with AgentExecutor:
At the execution level, LangChain features the AgentExecutor, which represents the reasoning brain of the agent – the language model and the tools it can call – into a coherent runtime environment.
Here, the agent decides on actions, which the AgentExecutor then carries out. This separation of decision-making and action execution encapsulates a best-practice design pattern in software engineering, enhancing maintainability and scalability.
Defining Agent Tools with LangChain:
The creation of agent tools is a central part of LangChain's utility. For example, it integrated more than 60 tools such as Wikipedia search, YouTube, Yahoo search, Google Scholar, etc. which you can leverage directly in your AI application.
In addition to the integrated tools with LangChain, you can create your tools for example the creation of retrieval tools from your operational database to get your product catalog.
Incorporating Memory:
LangChain also tackles the challenge of agent memory. Although it is only in the beta version, with the mechanisms to incorporate chat history, agents become capable of stateful interactions, remembering and building upon previous exchanges. This capability is crucial for providing continuity and context in conversations or task sequences, resulting in a more human-like interaction with users.
Agent types:
In LangChain, agent types are categorized based on their intended model type, ability to support chat history, handle multi-input tools, and conduct parallel function calling. Here are a few examples: ReAct Agent, Self Ask with Search Agent, and tool calling agent.
The choice of agent type should align with the model's capabilities and the complexity of the intended tasks.
A practical example of Agent creation with Langchain:
This code sets up a custom agent capable of executing tasks and maintaining a conversation state across multiple interactions. It combines tool binding, prompt templating, memory management, and executor logic into a comprehensive setup for a conversational AI model leveraging OpenAI's APIs. This example can serve as a foundational framework for developing more sophisticated agents tailored to specific use cases. (Please refer to this link for the latest code provided by langchain.)
from langchain_openai import ChatOpenAI
from langchain.agents import tool
# Load the language model
llm = ChatOpenAI(model="gpt-3.5-turbo", temperature=0)
# Define a simple tool
@tool
def get_word_length(word: str) -> int:
"""Returns the length of a word."""
return len(word)
# Example tool invocation
get_word_length.invoke("abc")
tools = [get_word_length]
from langchain_core.prompts import ChatPromptTemplate, MessagesPlaceholder
# Create the prompt template
prompt = ChatPromptTemplate.from_messages(
[
("system", "You are very powerful assistant, but don't know current events"),
("user", "{input}"),
MessagesPlaceholder(variable_name="agent_scratchpad"),
]
)
# Bind tools to the LLM
llm_with_tools = llm.bind_tools(tools)
from langchain.agents.format_scratchpad.openai_tools import format_to_openai_tool_messages
from langchain.agents.output_parsers.openai_tools import OpenAIToolsAgentOutputParser
# Create the agent
agent = (
{
"input": lambda x: x["input"],
"agent_scratchpad": lambda x: format_to_openai_tool_messages(
x["intermediate_steps"]
),
}
| prompt
| llm_with_tools
| OpenAIToolsAgentOutputParser()
)
from langchain.agents import AgentExecutor
# Initialize the agent executor
agent_executor = AgentExecutor(agent=agent, tools=tools, verbose=True)
# Example of using the agent
print(list(agent_executor.stream({"input": "How many letters in the word educa"})))
# Adding memory to the agent
MEMORY_KEY = "chat_history"
prompt = ChatPromptTemplate.from_messages(
[
("system", "You are very powerful assistant, but bad at calculating lengths of words."),
MessagesPlaceholder(variable_name=MEMORY_KEY),
("user", "{input}"),
MessagesPlaceholder(variable_name="agent_scratchpad"),
]
)
from langchain_core.messages import AIMessage, HumanMessage
# Setting up memory tracking
chat_history = []
# Updated agent with memory
agent = (
{
"input": lambda x: x["input"],
"agent_scratchpad": lambda x: format_to_openai_tool_messages(
x["intermediate_steps"]
),
"chat_history": lambda x: x["chat_history"],
}
| prompt
| llm_with_tools
| OpenAIToolsAgentOutputParser()
)
agent_executor = AgentExecutor(agent=agent, tools=tools, verbose=True)
# Running the agent with memory
input1 = "how many letters in the word educa?"
result = agent_executor.invoke({"input": input1, "chat_history": chat_history})
chat_history.extend(
[
HumanMessage(content=input1),
AIMessage(content=result["output"]),
]
)
agent_executor.invoke({"input": "is that a real word?", "chat_history": chat_history})
3.2 Agent with LlamaIndex
Data agents, powered by LlamaIndex and enhanced by LLM (Large Language Models) are very similar to what we have explained in section 2. There are three main components:

Planning: features the "Agent Reasoning Loop" logic. While the agent receives a new user message, the agent uses the "Reasing Loop" to decide if it is necessary to fetch history memory, to decide which tools to use, in which sequence, and parameters to call each tool.
The reasoning loop is the core of the agent's operation, various agent types are supported, including:
- Function Calling Agents: Pair well with function-calling LLMs.
- ReAct Agent: Suitable for chat/text completion endpoints.
- Advanced Agents: Such as LLMCompiler, Chain-of-Abstraction, Language Agent Tree Search, and more.
Tools: The agent is provided with a set of tools that it can decide what to use. Based on the current query and conversation history, the agent determines the necessary tools to engage. These could include RAG for information retrieval, specific search tools, or other tailored functionalities.
The selected tools process the input and generate outputs, which may include data retrieval results, computed values, or actions to be taken.
Memory: The agent retrieves the conversation history from memory, ensuring continuity and context for its response. After processing, the agent updates the conversation history in memory for future reference, preserving the flow of interaction.
Data agents in LlamaIndex serve their ability to:
- Execute user queries end-to-end through high-level interfaces.
- Offer low-level APIs for step-wise execution, granting fine control over task progression and analysis.
A data agent can be instantiated and operated as shown in the provided usage patterns, where it initializes from a set of Tools. For example, a ReAct agent can be created with various Tools to support both chat and query functionalities. Additionally, these agents inherit from ChatEngine and QueryEngine, which can provide the.
An example of implementing Data Agent in LlamaIndex
From this cookbook, here is an example of building a custom OpenAI agent in Python, using the OpenAI API with function-calling capabilities. This example includes tool creation, agent setup, and agent interaction within a conversational context.
# Import necessary libraries and packages
import json
from typing import Sequence, List
from llama_index.llms.openai import OpenAI
from llama_index.core.llms import ChatMessage
from llama_index.core.tools import BaseTool, FunctionTool
from openai.types.chat import ChatCompletionMessageToolCall
import nest_asyncio
# Apply necessary async adjustments
nest_asyncio.apply()
# Define computational tools for the agent
def multiply(a: int, b: int) -> int:
"""Multiply two integers and return the result"""
return a * b
def add(a: int, b: int) -> int:
"""Add two integers and return the result"""
return a + b
# Create FunctionTool instances from defined functions
multiply_tool = FunctionTool.from_defaults(fn=multiply)
add_tool = FunctionTool.from_defaults(fn=add)
# Define the custom OpenAI agent class
class YourOpenAIAgent:
def __init__(self, tools: Sequence[BaseTool] = [], llm: OpenAI = OpenAI(temperature=0, model="gpt-3.5-turbo-0613"), chat_history: List[ChatMessage] = []) -> None:
self._llm = llm
self._tools = {tool.metadata.name: tool for tool in tools}
self._chat_history = chat_history
def reset(self) -> None:
"""Reset the conversation history"""
self._chat_history = []
def chat(self, message: str) -> str:
"""Process a message through the agent, managing tool invocations and responses"""
self._chat_history.append(ChatMessage(role="user", content=message))
tools = [tool.metadata.to_openai_tool() for _, tool in self._tools.items()]
ai_message = self._llm.chat(self._chat_history, tools=tools).message
self._chat_history.append(ai_message)
# Handle parallel function calling
tool_calls = ai_message.additional_kwargs.get("tool_calls", [])
for tool_call in tool_calls:
function_message = self._call_function(tool_call)
self._chat_history.append(function_message)
ai_message = self._llm.chat(self._chat_history).message
self._chat_history.append(ai_message)
return ai_message.content
def _call_function(self, tool_call: ChatCompletionMessageToolCall) -> ChatMessage:
"""Invoke a function based on a tool call"""
tool = self._tools[tool_call.function.name]
output = tool(**json.loads(tool_call.function.arguments))
return ChatMessage(name=tool_call.function.name, content=str(output), role="tool", additional_kwargs={"tool_call_id": tool_call.id, "name": tool_call.function.name})
# Create and test the agent
agent = YourOpenAIAgent(tools=[multiply_tool, add_tool])
print(agent.chat("Hi")) # Expected response: Greeting or prompt from the model
print(agent.chat("What is 2123 * 215123")) # Expected response: Result of multiplication
3.3 Agent with AWS bedrock
Here is the process flow of an agent within AWS Bedrock. The process is divided into two main parts: the initial user input processing and the core action loop:

User Input Processing:
- While receiving user input, the agent checks the Prompt Store to fetch augmented prompts, then we check in the session store to fetch conversation history.
- The Foundation Model, which could be the Large Language Model, is invoked to pre-process the prompt to determine its validity and understand the user's intent.
Core Action Loop:
- The Foundation Model is then invoked again, this time to orchestrate prompt / make an action plan, taking into account the previously fetched prompts and history.
- The response is then parsed, and based on this, the agent decides whether to invoke an action or fetch additional documents from the Knowledge Base to inform its response.
- If an action is invoked, the Action Group Lambda function can be triggered to execute the action and generate an observation, which may lead to the RAG/retrieval of documents.
- This loop continues – invoking the model, parsing responses, invoking actions, and generating observations – until the agent either completes the task or asks the user for further information.

How to create an agent in AWS?
Agents in AWS Bedrock consist of several key components:
- Foundation Model (FM): A selected AI model that interprets user input and orchestrates the response flow.
- Instructions and Advanced Prompts: Detailed guidance is written to direct the agent's actions and logic at each step.
- Action Groups: Optional components that include an OpenAPI schema and AWS Lambda functions defining API operations the agent can invoke to complete tasks.
- Knowledge Bases: Optionally associated with agents, several vector databases provide context to augment the agent's responses. Including OpenSearch, Redis, Pinecone, and Amazon Aurora.
You can refer to the following screen captures to have a general idea of the creation of these components in AWS.


3.4 Agent Builder with Gemini
Since April 2024, Google has announced the Vertex AI agent Builder. Agent builder is a tool designed to accelerate the creation of AI agents with GCP.
On the UI, we define easily the agent, and the goal that we want the agent to achieve, provide the instructions, and share conversational examples.


Through agent builder, we can also use enterprise data stored in the GCP to ground the model. We can call functions and connect to applications to perform user tasks.


4. Multi-Agent framework
The AI agent domain is still in its early stages. Each framework or development stack has its method for constructing a standalone agent. However, it has quickly become apparent that when agents work in collaboration, they enhance their functionality and broaden the range of their applications. This collaboration presents two primary challenges:
- Orchestrating workflows involving multiple agents
- The difficulty of establishing communication between agents due to the often disparate interfaces each one utilizes
4.1 Microsoft AutoGen
To tackle the challenge of Agents Orchestration, Microsoft introduced the AutoGen framework in October 2023. AutoGen is designed to simplify the development of multi-agent applications, particularly in orchestrating LLM agents.
AutoGen provides a multi-agent conversation framework as a high-level abstraction. It is an open-source library for enabling next-generation LLM applications with multi-agent collaborations.
By using AutoGen, we can create agents equipped with different Large Language Models. We can have different types of agents: one agent for code generation and execution, and one agent for human feedback and involvement.
Consider a practical example: a user keen on learning Chinese. Through the Autogen we deploy three agents – one engaging with the user, another planning the course, and a third proposing course content. The user interacts with the user proxy agent, giving them the choice to validate the course planning. The teaching agent then sources learning material from vectorDB, constructing a day-to-day course based on user preferences.

If you want to know more about AutoGen, you can refer to my previous article: AutoGen In-depth yet Simple and No Code GenAI Agents Workflow Orchestration: AutoGen Studio with Local Mistral AI model.
4.2 crewAI
The CrewAI system is designed to mimic human teamwork's dynamic and collaborative nature, where each agent's unique skills and attributes contribute towards achieving a common goal. It introduces efficiency and structure to AI-powered task execution.
The CrewAI framework is established as follows:
- Agents: Initialized with specific attributes and capabilities.
- Tasks: Detailed descriptions of objectives and expected outcomes.
- Tools: The agents' toolbox, enables them to perform various actions effectively.
- Processes: The strategic workflow dictates how the crew approaches and completes tasks.

An implementation example with crewAI
Firstly, we install the necessary crewAI package and tools.
# Required installations
!pip install crewai
!pip install 'crewai[tools]'
In this example, there are 4 steps:
- Agent Definition: Configures two agents, a researcher, and a writer, each equipped with specific roles, verbose mode, memory, and a backstory to guide their interactions.
- Task Definition: Outlines specific tasks for the agents, detailing objectives and expected outputs, along with task-related configurations like asynchronous execution and output file specifications.
- Crew Formation: Combines the agents into a crew with defined processes and enhanced configurations like memory usage and task sharing.
- Execution: Initiates the process, feeding input variables into the system for a personalized approach, and prints the result of the crew's collaborative efforts.
# Import necessary libraries
import os
from crewai import Agent, Task, Crew, Process
from crewai_tools import SerperDevTool
# Set up environment variables
os.environ["SERPER_API_KEY"] = "Your Key" # serper.dev API key
os.environ["OPENAI_API_KEY"] = "Your Key"
# Define agents with distinct roles and capabilities
search_tool = SerperDevTool()
researcher = Agent(
role='Senior Researcher',
goal='Uncover groundbreaking technologies in {topic}',
verbose=True,
memory=True,
backstory=("Driven by curiosity, you're at the forefront of innovation, eager to explore and share knowledge that could change the world."),
tools=[search_tool],
allow_delegation=True
)
writer = Agent(
role='Writer',
goal='Narrate compelling tech stories about {topic}',
verbose=True,
memory=True,
backstory=("With a flair for simplifying complex topics, you craft engaging narratives that captivate and educate, bringing new discoveries to light in an accessible manner."),
tools=[search_tool],
allow_delegation=False
)
# Define tasks with specific objectives
research_task = Task(
description=("Identify the next big trend in {topic}. Focus on identifying pros and cons and the overall narrative. Your final report should clearly articulate the key points, its market opportunities, and potential risks."),
expected_output='A comprehensive 3 paragraphs long report on the latest AI trends.',
tools=[search_tool],
agent=researcher,
)
write_task = Task(
description=("Compose an insightful article on {topic}. Focus on the latest trends and how it's impacting the industry. This article should be easy to understand, engaging, and positive."),
expected_output='A 4 paragraph article on {topic} advancements formatted as markdown.',
tools=[search_tool],
agent=writer,
async_execution=False,
output_file='new-blog-post.md'
)
# Form a crew with configured agents and tasks
crew = Crew(
agents=[researcher, writer],
tasks=[research_task, write_task],
process=Process.sequential, # Sequential task execution is default
memory=True,
cache=True,
max_rpm=100,
share_crew=True
)
# Kick off the task execution process
result = crew.kickoff(inputs={'topic': 'AI in healthcare'})
print(result)
4.3 Agent Protocol
Agent Protocol is designed as a single common interface for communicating with different agents which aims to tackle the second challenge that we mentioned for multi-agent systems.
Any agent developer can implement this protocol. The Agent Protocol is an API specification – a list of endpoints, which the agent should expose with predefined response models. The protocol is tech stack agnostic. Any agent can adopt this protocol no matter what framework they use as explained in section 3 (or not).
How does the protocol work? Right now the protocol is defined as a REST API (via the OpenAPI spec) with two essential routes for interaction with your agent:
- POST /ap/v1/agent/tasks for creating a new task for the agent (for example giving the agent an objective that you want to accomplish)
- _POST /ap/v1/agent/tasks/{taskid}/steps for executing one step of the defined task
It has also a few additional routes for listing the tasks, steps and downloading/uploading artifacts.
Here is the agent protocol GitHub if you want to know more.
5. Conclusion
The future of generative AI is exciting. The capability of planning, using different tools, and keeping memory would make agents more performant together with LLM. Agents from systems like LangChain, LlamaIndex, AWS, Gemini, Microsoft AutoGen, and crewAI are changing technology.
Embracing these technologies will enable us to shape a future where AI uses ‘hands and brains,' becoming a helpful partner in what we do every day.