The Hardest Part: Defining A Target For Classification
What Is a Target Variable?
A target variable is the variable or metric you're trying to predict with a supervised machine learning model. It is also often called the dependent variable, the response variable, the ‘y' variable, or even simply the model output.
Regardless of which term you prefer (or were made to use in statistics class), this is the most important variable in any supervised modeling project. While this is obvious to most people who have machine learning experience – it's worth reiterating why for newcomers.
From a technical perspective, the data type of your target variable determines what type of modeling project you are working on. Numeric target variables are the domain of regression models, while categorical variables mean you are working on a classification model.

But even more importantly than model type, your target variable is the entire reason why you are building a model.

Defining A Target Variable for Classification
On the surface, defining a target variable for classification might seem easy. But your opinion might change if you get asked to build a model for data that's never been modeled before.
When people begin learning machine learning, they are usually given relatively clean datasets that have clearly defined 0s and 1s they can use for classification modeling. But in my experience, it's extremely rare to find a column in a relational database that perfectly aligns with what your final target variable will look like.
Of course, this makes perfect sense in the context of learning the ins and outs of ML algorithms, but I certainly had a rude awakening when I saw the SQL query that generated the first "real life" modeling dataset I had the opportunity to work on in my first data scientist job.
Let's look at a few examples of the types of transformations we might need to make to our data to generate a target variable, in the context customer churn for a subscription product. This could be an insurance policy, Netflix subscription, whatever. I've created some fake data that mimics data I've seen in production, but forgive me if it doesn't align with how a data engineer would structure these tables.
Example 1: Cancellation Date

There is no clear 1 or 0 indicating a customer has churned (subscription ended here), but in this example, it's pretty straightforward to engineer one. If subscription_end_date is null, we might be able to assume the customer is still active. So we can apply some basic logic to assign a 1 if subscription end date is not null, and a 0 if it is, so that our target variable's positive class is a churner.
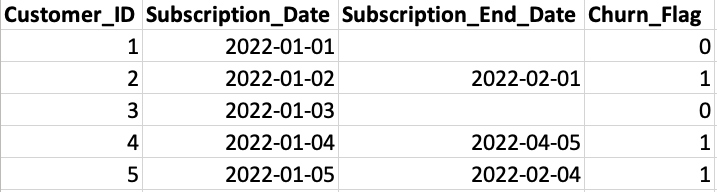
Easy enough. Now let's look at a more complex example.
Example 2: Renewal Date

Now imagine we have another column in our subscription table. If a customer becomes a paying subscriber again, we have a column to capture when that occurred.
Our first customer who had a subscription end never came back, so we can safely say they churned.
But what about customer 4? They resubscribed one day after their subscription ended. They may have had a monthly subscription end, or had a credit card expire, and as soon as they realized this reactivated their subscription. Is a one day lapse in membership enough to say this customer churned? Probably not. But what about a 7 day or 30 day lapse?
Customer 5 did resubscribe, but they were not a customer for over a year. It is probably fair to say they churned, but this is where talking to your stakeholders and subject matter experts is critical. We might settle on logic that defines a churner as someone who has lapsed for a period of a longer than 30 days, and end up with the following:

This is not a trivial difference. Your target definition can have downstream impacts on which features successfully predict your target, the overall accuracy of your model, and ultimately the success of your project.
For example, if customer 4 was a very active user of our platform (they noticed immediately when they lost access), but the other two were not, the predictive power features that correlate to churn will be dampened by our shoddy target definition.
I considered adding more examples, but I think the broader point is well illustrated by these two. As food for thought, the logic of target creation is even more susceptible to error when we have transactional tables that have one row for each monthly payment, differing subscription types and lengths, customers who got a free subscription due to a promotion (we may want to filter them out altogether), and more.
A Bit of Advice
Your model's target is not simply a 0 or 1, it represents the foundational unit of success for your classification modeling project. As such, I have a few pieces of general advice for how to approach defining the target variable in your next project:
- Your target variable likely won't be defined cleanly in a table for you
- Take time, and I'm talking several days, if not weeks, to think critically about how your target variable can be defined from the data at hand and how that definition relates to business goals. This is rarely a trivial task, and you are much better off putting in the time and effort up front than needing to shift the definition later.
- Your target variable may not have an agreed on definition conceptually in your organization. "Churn" can be defined in many ways, and you may never have a perfect definition. But it's important to get consensus on how you, as the modeler, define it, by talking to stakeholders. At the very least, you're covering your own you-know-what in the case that it turns out the definition needs to change, and at best, you have set the foundation for building a successful model.
Thank you for taking the time to read my thoughts – I know they are probably not groundbreaking to seasoned data scientists, but my hope is that folks new to this field gain awareness about the gaps between the classroom and the job, and understand that target variables shouldn't be taken for granted – it's tempting to move as quickly towards modeling as possible, but if you do – you might miss the… target.
If you enjoyed this article, give me a follow! I write regularly about topics like Python, Pandas, and transitioning from data Analytics to data science. I also have courses on these topics available on the Maven Analytics Platform and Udemy – would love to see you there!