The Parallels of Generative AI
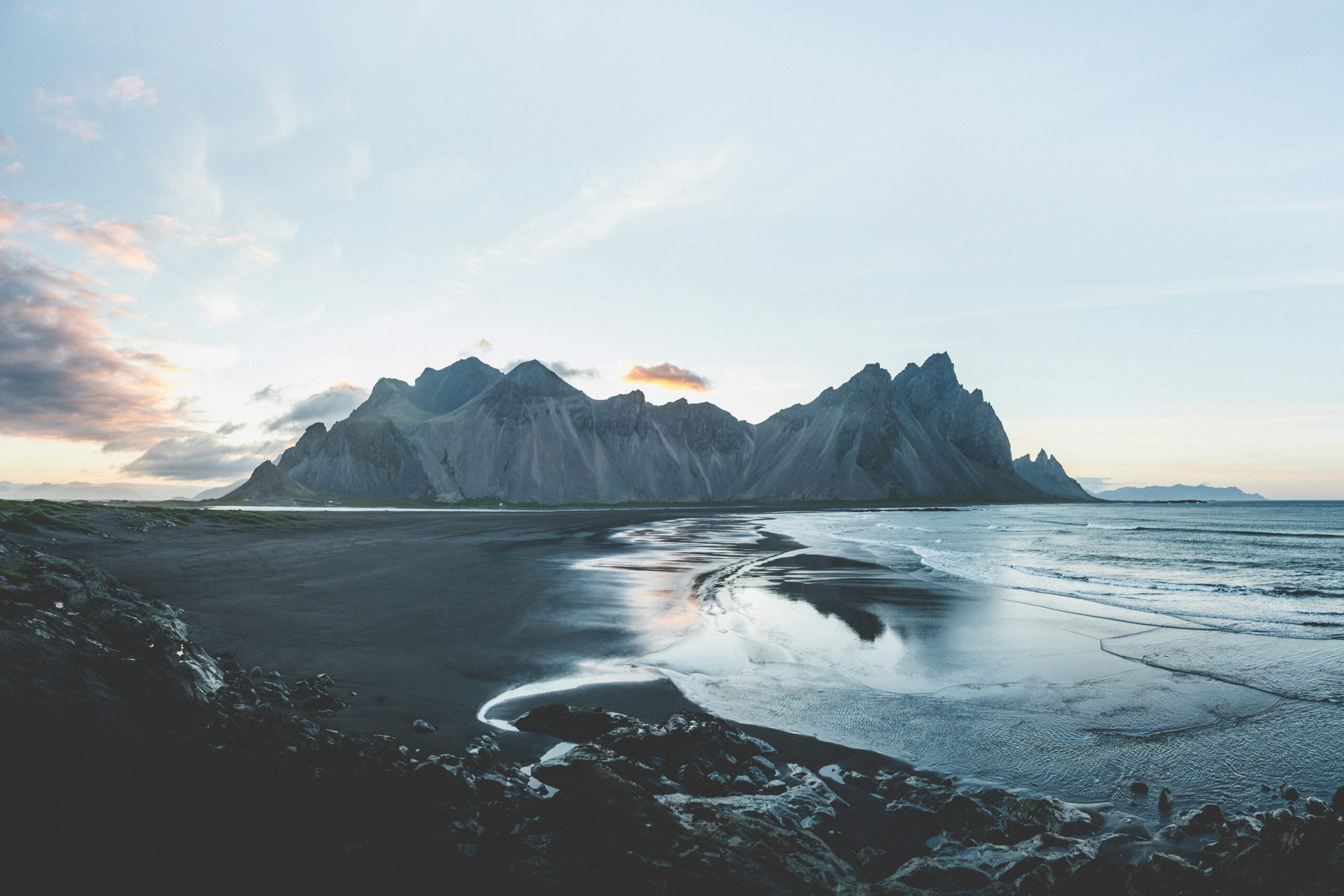
When asked ‘How do you think AI will change us [humans]?‘ Jeff Bezos prefaced his answer with a thought-provoking comment.
"Large language models in their current form are not inventions. They are discoveries."
And to illustrate it with his examples, Galileo invented the telescope to later discover the moons of Jupyter. He anchors LLMs to the far end of the invention-discovery spectrum by arguing that "[as opposed to] a 787 [which] is an engineered object, LLMs are much more like discoveries. We are constantly being surprised by their capabilities".
Large language models – and you can extend it to generative AI as a whole – are already driving excitingly innovative use cases everywhere. They are being wrapped in every shape and form and shipped to users as personalized customer service chatbots, writing assistants, and even embedded in new features at longstanding and widely used tools such as Adobe Photoshop. So it's real technology. They are engineered tools – inventions already – right?
Well, sometimes the wrapper is just too thin. When you stop to reflect on Bezos' comment¹ and start to look at each release of a new GPT by OpenAI or a new state-of-the-art open-source model by Meta solely as the outcome of research, you will notice what might be actually happening – we are getting highly proficient at bridging the gap from discoveries to products.
In this article, I reflect on how a new industry is democratizing generative AI implementation and how byproducts of research and broadly available tools are now closer than ever.
The Parallels
Generative Artificial Intelligence has never been the sole driver of disruptive implementations of AI, nor has it been a ubiquitous field in modern machine learning research. Quite the opposite, until 2020, although deep learning papers dominated Google Scholar's list of most influential publications, computer vision research centred on object detection and image recognition attracted the highest number of citations, and "Attention is all you need", the work that introduced the Transformers architecture, was only the 4th most cited in that year, even though Google was already applying BERT models to search since 2019.
It is a fact, however, that all this work paved the way for generative AI to eventually become mainstream, both in research and in social media. Advancements in deep learning architectures, such as Generative Adversarial Networks (GANs) and Diffusion Models, are driving widespread enthusiasm with each new showcase of potential, like the recent realistic text-to-video demonstrations of Sora by OpenAI.
On the other side, when we look at tools – the ones that make it to the hands of the general public as engineered products – we have pre-ChatGPT examples, such as GitHub Copilot, long proving the potential for productivity increase through generative technology. But it wasn't until the prominent chatbot by OpenAI made the scene in November 2022 that the sheer capabilities of generative AI became evident to the broad public.
Until then, the discovery-tool parallels ran through distinct routes defined by a clear boundary that set them apart: Implementation.

An Urge for Enterprise-Grade Generative AI
If you look for reasons why 2023 was such a wild year for advancements in generative AI, both in research and product offerings, you will probably have a hard time landing into a single denominator. The reason is simple – as time goes by, more players are joining the scene (researchers, companies, and investors), hence more variables are contributing to the equation. A new reason to adopt AI (or at least to admit that you should not be turning your eyes away from it) probably arises every day, regardless of industry. Regardless of where you are in the value chain.
But one thing is undeniable: ChatGPT sparked companies' curiosity plugs about how generative AI could change their businesses. In June last year, a study by McKinsey went over 63 use cases where generative AI can address specific business challenges and estimated that these alone could deliver in value up to $4.4 trillion annually to the global economy. When extrapolated to possible productivity increase by the technology being applied across knowledge workers' activities, the impact can amount to $7.9 trillion².
So, the boundary setting discovery apart from tools could not rest unyieldingly. While the implementation of generative AI for tech companies already leveraging machine learning faces less friction – both in capital allocation, access to a modern data stack and, most importantly, sourcing of talented professionals – the scenario is not the same for the vast majority of businesses. A recent publication by Dataiku concludes it clearly – the massive gap between the potential applications for Generative AI in large enterprises and the number of experts who can potentially build those systems are driving an urge for democratization.
The Burst of Open Source Generative AI
ChatGPT also stirred the research community as much as it did the businesses' C-suite boards by having executives rushing to understand what a Generative Pre-trained Transformer is. But there is also another key contributor to remark.
Arguably, the most important reference to open-source generative AI was the Llama family of large language models introduced by Meta in February 2023. Whether the release of Llama itself can be classified as open-source or not can probably fuel a discussion for a complete medium article. Still, it undeniably provided a foundation for numerous models that followed.
2023 was also the year when open-source artificial intelligence saw a substantial inflow of venture capital through companies like Hugging Face and Mistral, showing the interest of investors in alternatives to OpenAI.
The result of the above is a flourishing research scene that contributes even further to the tension to bridge the implementation boundary that sets discovery and products apart.
Convergence
And that's precisely what is happening.
A whole industry is emerging around the need to reduce the friction of generative AI implementation. Major cloud providers are introducing new capabilities to better support generative AI workflows in their data science and machine learning platforms, such as Google, which announced the general availability of the Generative AI Studio for Vertex AI in June 2023, and Amazon Bedrock, which became generally available in September 2023 for AWS as a service for building generative AI applications.

IBM gives another example, with WatsonX as a whole new division on top of the rebranded IBM Watson to provide companies with a platform to develop and integrate AI into their businesses.
But the list is not solely made up of giants. A whole new roster of dev tools start-ups is raising seed funding with a pitch to take on the task of making the implementation of generative AI as seamless as it can get. Deepset, for example, saw it coming in 2018 after the first waves of adoption of Transformers models and recently raised $30 million to expand its LLM-focused MLOps offerings to help the industry "shift from AI labs to AI factories".
Hugging Face and other community-driven, open-source projects such as LangChain and Llama Index are also empowering independent developers to quickly bring agent-based tools to market.
Vector databases such as Pinecone, Chroma, and Weaviate, thus far known to drive recommender engines, are now central pieces of RAG-based applications. By the first half of 2023, the sector was already seeing a take-off in investments, reflecting its core role in driving generative AI implementation.
Quality Before Quantity
But it's not a consensus that facilitated implementation equals better offering of AI tools. On one side, it enables quick time-to-market of great tools such as Adobe Firefly. On the other hand, the rush to deliver "AI-enabled technology" has also led to a flood of questionable releases.
A recent example was seen this past weekend, as a tribunal ruled in favour of an AirCanada customer who relied on a response by the company's chatbot that failed to retrieve an important piece of the refund policy in its reply. Or the Chevrolet of Watsonville customer service AI assistant that has been fuelling subreddits with memes since December 2023.
The fact that hallucinations are a problem and that securing LLMs from malicious prompts is not a trivial task is not breaking news. These and many other inherent risks of generative AI have been well-known for quite some time. But to see that it keeps attracting investments is another affirmation that its benefits far outweigh the challenges.
Conclusion
As Jeff Bezos also said in his two-hour conversation with Lex Fridman: "Specialized implementations of large language models can become powerful tools". And on the final balance of the equation, it is great to see that we are getting so good at converging the discovery-tool parallels together.
Enjoyed this Story?
You can follow me here on Medium for more articles about artificial intelligence, data science, and machine learning.
You can also find me on LinkedIn and on X, where I share shorter versions of these contents.
Sources
Alongside the hyperlinks throughout this article, I specifically recommend the following sources for long-form content on the subject:
[1] Jeff Bezos: Amazon and Blue Origin | Lex Fridman Podcast #405: https://www.youtube.com/watch?v=DcWqzZ3I2cY
[2] Economic potential of generative AI | McKinsey: https://www.mckinsey.com/capabilities/mckinsey-digital/our-insights/the-economic-potential-of-generative-ai-the-next-productivity-frontier
Unless otherwise noted, all images are by the author.