Through the Uncanny Mirror: Do LLMs Remember Like the Human Mind?
|LLM|AI|HUMAN MIND|MEMORY|COGNITION|

The limits of my language are the limits of my mind. – Ludwig Wittgenstein
The true art of memory is the art of attention. – Samuel Johnson
Language is one of the most important capabilities of human beings; it enables us to communicate and transfer knowledge, and it is considered a pillar of civilization. That is why the incredible capabilities displayed by Large Language Models (LLMs) have astounded the world, and made it ask the question: are they intelligent?
All this has been achieved by huge amounts of text and a simple learning function: predicting the next word in a sequence. The model behind this success is the Transformer, and today modern derived LLMs are currently used by a large segment of the population for tasks such as translation, summarization, question answering, or generating articles.
All these elements show the great versatility of the transformer. At the same time, despite the extensive use of transformers in both research and production, several open questions remain. For example, most of the research on the model has focused on how to increase its performance or applications. These studies though tell us little about how it works and how it achieves its abilities.
One of the neglected topics is how the memory of LLMs works. Memory is as fundamental to us as language is. Without memory, we cannot perform any of our daily skills. LLMs learn from a huge body of text and can show incredible knowledge, so they seem to have memory. Several open questions remain:
- Do LLMs have a memory?
- If so, in what form?
- How does this differ from that of humans?
In general, the concept of memory is discussed for LLMs only at the application level. For example, a transformer limitation is context length, so an LLM cannot use information that does not fit into its context length. Therefore, one line of research focuses on extending the context memory [1–2]. These approaches are training-free and provide external memory to allow the model to retrieve information:
In this paper, we propose a training-free memory-based approach, named InfLLM, for streamingly processing extremely long sequences with limited computational costs. Specifically, InfLLM incorporate the sliding window attention with an efficient context memory, where each token only attends to local contexts and relevant contexts from the memory. [2]
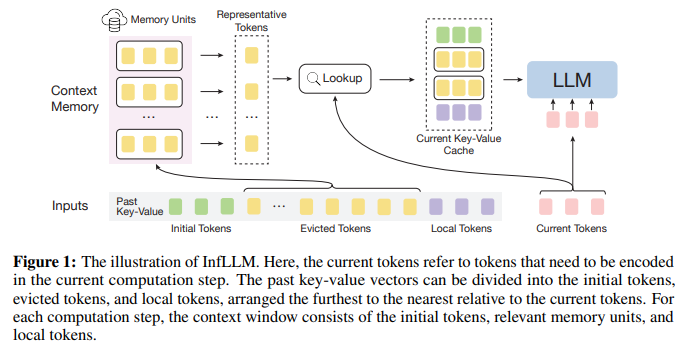
A second line of research instead investigates the possibility of adding external memory to the LLM. Indeed, training an LLM is expensive but its knowledge becomes outdated quickly. Conducting fine-tuning is an equally expensive process, so a method is sought to be able to allow the model to continue to learn and edit its memory. This external memory should be used to learn new knowledge but also to reinforce/delete certain information [3–4].

AI Hallucinations: Can Memory Hold the Answer?
Forever Learning: Why AI Struggles with Adapting to New Challenges
These studies focus on improving model performance and tell us nothing about the parametric memory of the LLM.
How is LLM memory different from human memory?
To make a comparison one would have to start with the definition of memory, according to Wikipedia:
Memory is the faculty of the mind by which data or information is encoded, stored, and retrieved when needed. It is the retention of information over time for the purpose of influencing future action.
This definition is generic and does not explain how human memory works. In the human brain, we can define the passage of information as an electrical signal, thus memory will be encoded as an electrical signal. The problem arises when talking about "storage" and "retrieval."
Where is this memory located in the human brain? What does a single neuron encode (a word, a sentence, or a concept)? How does the human brain handle the enormous amount of daily information?
Storage thus turns out to be more complex than it seems, as evidenced by different studies:
There is not a single part of the brain that stores all the memory; instead, the storage location is defined by the type and use of memories. Explicit memories (information about events where a person was present, general facts, and information) are stored in the hippocampus, the neocortex, and the amygdala. For implicit memories, also referred to as unconscious or automatic memories, the most crucial brain regions are the basal ganglia and cerebellum. [6]
Similarly, information recall is complex, and it is difficult to identify how this occurs, which regions are involved, and the role of individual neurons.
Even mathematically modeling such a definition of memory is complex, to make it simpler we can define memory as consisting of two components:
- Input. To trigger a memory, the input must be the same or similar to information that a brain (or electronic brain) has previously encountered.
- Output. The result is based on the output (this can be forgotten, incorrect, or correct). When the output is correct must be aligned with the information previously encountered.
This is a more dynamic definition of memory allowing us to verify it in the LLMs. A person may or may not know a mathematical theorem but until he is asked and answers we will not know if he has memory of it. After all, if memory is diffuse we have no way of knowing if memory exists until there is an input.

Since we are defining memory as the relationship between input and output, in the transformer this process is modeled by the transformer block:
By using attention, however, a model can simply memorize facts (e.g. function definitions) by storing them as (key, value) pairs in long-term memory, and then retrieve those facts later by creating a query that attends to them. [4]
So the memory capacity of an LLM must have something to do with the transformer block.
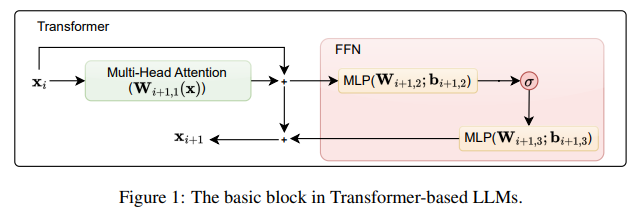
From the Universal Approximation Theorem (UAT) it can be shown that the transformer block can approximate any function and its parameters are dynamically modified in response to the input. So can we suggest that the memory of LLMs is to fit specific outputs based on the input?
In this paper [5], the authors conduct fine-tuning of a set of models (Qwen family) on a series of poems in both Chinese and English. The results show that:
- Larger models perform better.
- Given a title or other partial information, the models can regenerate the full poem.
- Although sometimes the prediction is incorrect, the output aligns with the information.

Based on these experiments the LLMs possess a memory and it functions by fitting the input to a specific output. That is, one can only determine whether an LLM possesses a specific memory only if one provides a question:
Based on the definition of memory and the experimental results, we believe that LLMs indeed possess memory capabilities, and this ability shows no fundamental difference from human memory. [5]
This is a bold comparison. Is there really all this similarity between LLM memory and human memory?
It may sound strange, but there are other similarities between human and LLM memory. The memory of LLMs and humans is diffuse. We cannot find a single unit that stores a specific memory in either a brain or an LLM. In addition, LLMs have a problem with rare knowledge. Once a fact is encountered during training it is stored. Reencountering the same information during training strengthens its memorization, while its absence reduces its knowledge. Also, repetitions work best after some delay, just as in humans.

An LLM Student's Handbook: Mastering the Art of Learning and Retaining Knowledge
Human memory manifests the so-called primacy and recency effects. Simply put, objects appearing at the beginning or end of the list are more easily remembered. Thus there is more memory of items at the extremes and less of those in the middle of the list. The same phenomenon is observed in LLMs: there is the same positional bias. LLMs also have better recall of elements positioned at the beginning and end (this is one of the problems with long-context LLMs) [10–11].

There are two possible mechanisms of forgetting: memory traces fade with time (memory decay) or new memories are rewritten over previous ones (memory interference). Some psychological studies show that humans forget more by interference than by the simple passage of time [12]. In a study [10], they showed that the same is true for LLMs. In forgetting, memory decay is a less important mechanism than memory interference. This effect is more prominent when the pattern is presented with new information similar to the stored information (e.g., we forget a person's name more easily after being presented with several other people).

To claim that the memory of an LLM and a transformer function equally is more of a provocation than an accepted fact. Mainly because how human memory works is not yet clear to us. There are similarities, and these might come from the fact that we structure our narratives in a way that is compatible with the characteristics of our biological memory. LLMs are then trained on these written narratives, subtly inheriting this imprinting. This also means that the relationship between language and the human brain is even closer than thought.
The similarities and differences between LLMs and the human brain can guide us to create new and better LLMs in the future. At the same time, these similarities allow us to use LLMs to study human memory (as done in this study [13]). Either way, exciting prospects open up.
What do you think? Do you think there are other similarities or differences with human memory? Let me know in the comments
If you have found this interesting:
You can look for my other articles, and you can also connect or reach me on LinkedIn. Check this repository containing weekly updated ML & AI news. I am open to collaborations and projects and you can reach me on LinkedIn. You can also subscribe for free to get notified when I publish a new story.
Here is the link to my GitHub repository, where I am collecting code and many resources related to machine learning, Artificial Intelligence, and more.
GitHub – SalvatoreRa/tutorial: Tutorials on machine learning, artificial intelligence, data science…
or you may be interested in one of my recent articles:
OpenAI's New ‘Reasoning' AI Models Arrived: Will They Survive the Hype?
Graph ML: How Do you Visualize Large network?
How the LLM Got Lost in the Network and Discovered Graph Reasoning
A Brave New World for Scientific Discovery: Are AI Research Ideas Better?
Reference
Here is the list of the principal references I consulted to write this article, only the first name of an article is cited.
- Chen, 2023, LongLoRA: Efficient Fine-tuning of Long-Context Large Language Models, link
- Xiao, 2024, InfLLM: Training-Free Long-Context Extrapolation for LLMs with an Efficient Context Memory, link
- Modarressi, 2024, MemLLM: Finetuning LLMs to Use An Explicit Read-Write Memory, link
- Wu, 2022, Memorizing Transformers, link
- Wang, 2024, Schrodinger's Memory: Large Language Models, link
- Psychology writing, Human Memory: The Current State of Research, link
- Tirumala, 2022, Memorization Without Overfitting: Analyzing the Training Dynamics of Large Language Models, link
- Chang, 2024, How Do Large Language Models Acquire Factual Knowledge During Pretraining? link
- Robinson, 1926, Effect of Serial Position upon Memorization, link
- Zhang, 2024, A Survey on the Memory Mechanism of Large Language Model based Agents, link
- Liu, 2023, Lost in the Middle: How Language Models Use Long Contexts, link
- Oberauer, 2008, Forgetting in immediate serial recall: decay, temporal distinctiveness, or interference? link
- Georgiu, 2023, Using large language models to study human memory for meaningful narratives, link