What is Data Quality?
.
Summary
I. The Pillars of Data Management
1. Why is it key?
2. Quality vs. Integrity vs. Profiling
II. What are the 6 Dimensions of Data Quality?
1. Completeness: do we have all the data?
2. Uniqueness: are features all unique?
3. Validity: is the format respecting business requirements?
4. Timeliness: up-to-date data
5. Accuracy: does it reflect reality correctly?
III. Next Steps
1. Data Quality for Environemental Reporting: ESG & Greenwashing
2. Data Quality and Accounting Automation
3. Generative AI: Automate Data Quality Check with GTP Agent
4. Conclusion
What are the Pillars of Data Management?
Why is Data Management key?
High-quality data can be the difference between the success and failure of your supply chain operations.
From planning and forecasting to procurement and logistics, every facet of supply chain management relies on data to function effectively.
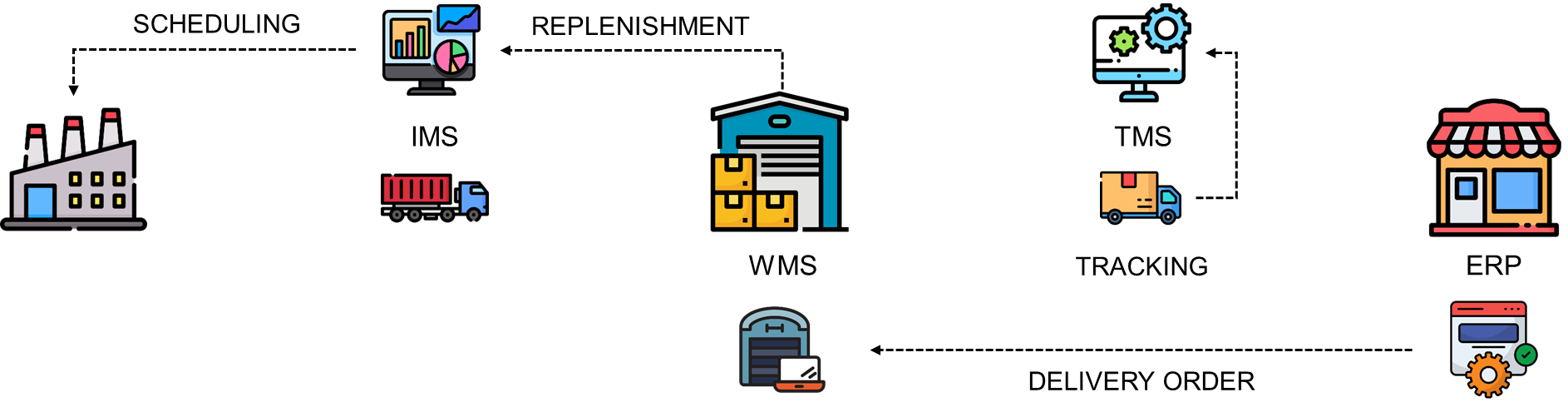
- Planning and forecasting algorithms rely on WMS and ERP data for historical sales, inventory levels and store orders.
- Transportation Management Systems rely on WMS data to properly track shipments from warehouses to stores.
What are the different types of analytics?
Supply Chain Analytics solutions can be divided into four types that provide different levels of insights and visibility.

Descriptive solutions usually represent the first step in your digital transformation: collecting, processing and visualizing data.
It all starts with data collection and processing to analyze your past performance.
What would be the impact of bad data on descriptive solutions?
Let us take the example of the store distribution process of a fashion retail company.

A simple indicator to pilot your operations is the percentage of orders delivered on time: On Time In Full: OTIF.

This indicator can be built by merging transactional tables from
- Enterprise Ressource Planning (ERP) for order creation
- Warehouse Management Systems (WMS) for order preparation
- Transportation Management Systems (TMS) for order delivery
Why do we care about data quality here?
If you don't ensure that the data is correct, it is impossible to
- Measure the actual performance of On-Time Delivery properly
- Implement automated diagnostic by comparing actual time stamps with their target
An indicator you cannot measure confidently cannot be analyzed and improved.
What are the differences between Quality, Integrity and Profiling?
Before we delve into data quality, it is essential to understand how data quality differs from related concepts like data integrity or Data Profiling.

While all three are interconnected, they have distinct areas of focus
- Data integrity is concerned with maintaining the accuracy and consistency of data over its entire life cycle.
- Data profiling involves examining and cleaning data to maintain its quality.
Data profiling should not be mixed with data mining.

- Data profiling will focus on the structure and quality of data, examining outliers, distribution, or missing values.
- Data mining focuses on extracting business and operational insights from data to support decision-making or continuous improvement initiatives.
Now that we have clarified these differences, we can focus on defining Data Quality.
What are the 6 Dimensions of Data Quality?
Data quality is evaluated based on several dimensions that play a crucial role in maintaining the reliability and usability of data.

Data Completeness: do we have all the data?
The objective is to ensure the presence of all necessary data.
Missing data can lead to misleading analyses and poor decision-making.
Example: missing records in the master data
Master Data Management (MDM) is crucial in a company for ensuring consistent and complete data across departments.

Master data specialists enter product-related information into the ERP during the item creation.
- Product information: net weight, dimensions, etc.
- Packaging: total weight, dimensions, language, etc.
- Handling units: number of items per (carton, pallets), pallet height
- Merchandising: supplier name, cost of purchase, pricing per market
These data specialists can make mistakes, and missing data can be found in the master data.
What kind of issues can we face with missing data?
- Missing net weight: issues linked to transportation management as we need the weight for invoicing and customs clearance
- Missing costs: your procurement cannot send purchase orders to the suppliers
Many other issues are along the value chain, from sourcing raw materials to store delivery.