Why Do You Need to Use SQL Grouping Sets for Aggregating Data?
Although it's called a query language, SQL is capable of not only querying databases but also performing efficient data analysis and manipulation. It is not a surprise that SQL is embraced by the Data Science community.
In this article, we will learn about a very handy SQL feature, which allows for writing cleaner and more efficient queries. This I-wish-I-knew-this-earlier feature is the GROUPING SETS
, which can be considered as an extension of the GROUP BY
function.
We will learn the difference between them as well as the advantage of using GROUPING SETS
over the GROUP BY
function but first, we need a dataset to work on.
I created a SQL table from the Melbourne housing dataset available on Kaggle with a public domain license. The first 5 rows of the table looks as follows:

The GROUP BY function
We can use the function to calculate aggregate values per group or distinct values in a column or multiple columns. For instance, the following query returns the average price for each listing type.
SELECT
type,
AVG(price) AS avg_price
FROM melb
GROUP BY type
The output of this query is:

Multiple groupings
Let's say you want to see the average price for each region in the northern area, which can be achieved by using the GROUP BY
function as follows:
SELECT
regionname,
AVG(price) AS avg_price
FROM melb
WHERE regionname LIKE 'Northern%'
GROUP BY regionname
The output:

Consider a case where you want to see the average price of different house types in these two regions in the same table. You can achieve this by writing two groupings and combining the results with UNION ALL.
SELECT
regionname,
'all' AS type,
AVG(price) AS average_price
FROM melb
WHERE regionname LIKE 'Eastern%'
GROUP BY regionname
UNION ALL
SELECT
regionname,
type,
AVG(price) AS average_price
FROM melb
WHERE regionname LIKE 'Eastern%'
GROUP BY regionname, type
ORDER BY regionname, type
What the query does is to calculate the average price for each region first. Then, in a separate query, it groups the rows by both region name and type and calculates the average price for each group. The union combines the output of these two queries.
Since the first query does not have the type column, we create it manually with a value of "all". Finally, the combined results are ordered by the region name and the type.
The output of this query:
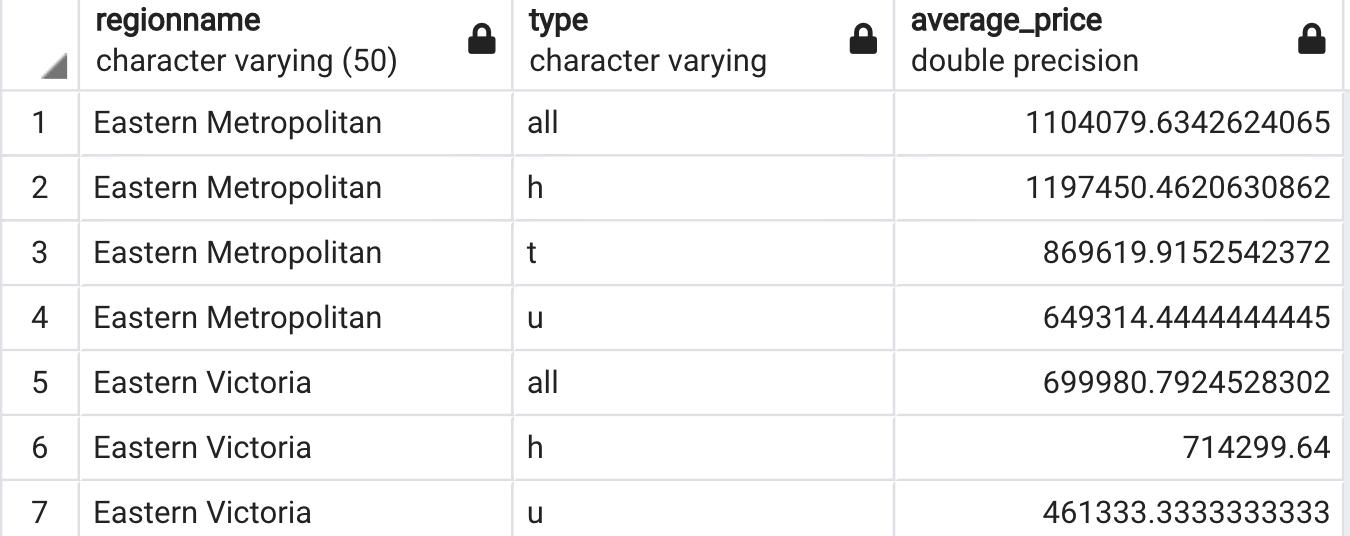
The first row for each region shows the region average and the following rows show the average price for different house types.
We had to write two separate queries because we cannot have different queries in a GROUP BY
statement unless we use GROUPING SETS.
GROUPING SETS
Let's rewrite the previous query using GROUPING SETS.
SELECT
regionname,
type,
AVG(price) as average_price
FROM melb
WHERE regionname LIKE 'Eastern%'
GROUP BY
GROUPING SETS (
(regionname),
(regionname, type)
)
ORDER BY regionname, type
The output:

The output is the same except for the null values in the type column which can easily be replaced with "all".
Using the GROUPING SETS
has two main advantages:
- It is shorter and more intuitive which makes the code easier to debug and manage
- It is more efficient and performant than writing separate queries and combining the results because SQL scans the tables for each query.
Final thoughts
We often disregard query readability and efficiency. We are happy if the query returns the desired data.
Efficiency is something we always need to keep in mind. The impact of writing bad queries may be tolerated when querying a small Database. However, when the data size becomes large, bad queries may lead to serious performance issues. In order to make ETL processes scalable and easy-to-manage, we need to adapt best practices. The GROUPING SETS
is one of these best practices.
You can become a Medium member to unlock full access to my writing, plus the rest of Medium. If you already are, don't forget to subscribe if you'd like to get an email whenever I publish a new article.
Thank you for reading. Please let me know if you have any feedback.