Yes, you still need old-school NLP skills in "the age of ChatGPT"
Yes, You Still Need NLP Skills in "the Age of ChatGPT"
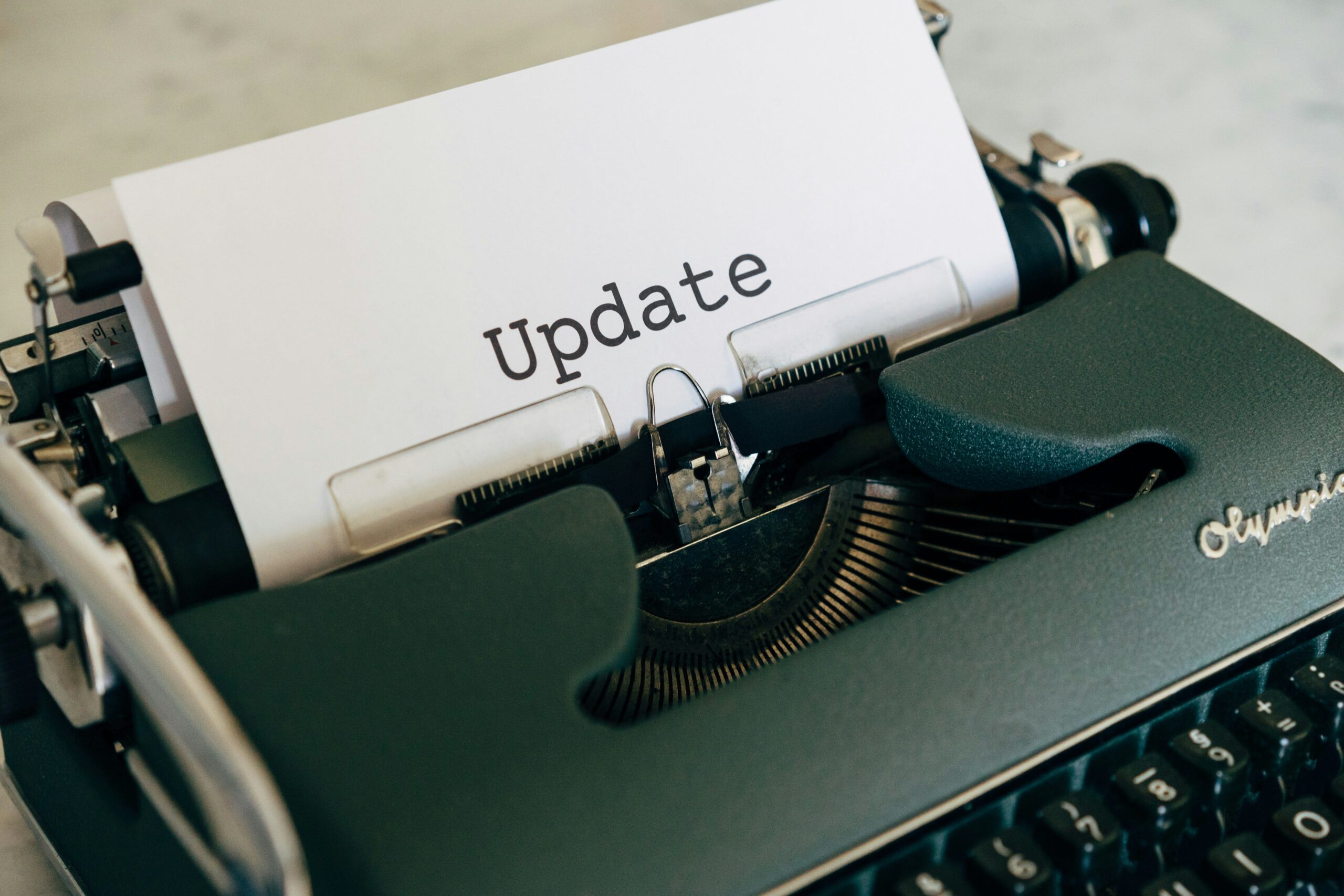
Back when I started a masters of Computational Linguistics, no-one I knew had even the faintest idea what Natural Language Processing (NLP) was. Not even me [1]. Fast forward four years, and now when I say I work in NLP, I only get blank looks about half of the time [2]. Thanks to masses of media hype, most people know that there are things called Large Language Models, and they can do a lot of amazing and very useful stuff with text. It's become a lot easier for me to explain my job to people (provided I tell them "it's basically ChatGPT"). But recently, this also gave me pause.
I'm the editor of a Data Science and AI textbook, published waaaay back in 2022 (seriously, in AI years that's about 20). In preparation for the third edition, coming this year, I needed to update my NLP chapter. And as I sat down to read what I wrote back then about neural networks, sequence to sequence models, and this damn-fangled new technology called "Transformers," I noticed something remarkable: it all felt so old school. All that stuff on statistical Machine Learning approaches? Quaint. And my little code snippets explaining how to do text preprocessing with Python? Like a curious artifact from a bygone time.

I started to worry, would anyone find my old passion relevant? Or would I have to scrap it all in favour of our new chapters on Generative AI and foundation models [3]? I'm convinced that fundamental NLP skills are still important, and that anyone who's actually worked in "traditional" NLP would agree. But what about a novice reader? What about a total programming newbie, or perhaps a statistician or software developer wanting to go where all the cool kids are? Would they want to read about the joys of web scraping, tokenization and writing regex parsers [4]?
In my opinion, they should.
Why you still need to learn "traditional" NLP
Not everyone needs a chatbot
LLMs are great at using their vast world "knowledge" and creativity to generate novel, long-form content, where multiple correct solutions are possible. But many data use cases seek the exact opposite of this. They require extracting specific, concrete information from unstructured data, and usually, there's only one correct answer. Sure, LLMs can do this too: if I copy a customer inquiry email into a chatbot and ask it to extract the customer details and inquiry topic into a JSON string, it'll manage. But so could a Named Entity Recognition (NER) model, and it'll probably have lower latency, be easier to evaluate, and potentially be more interpretable. Its output format will also be guaranteed, whereas with the LLM, it'll be up to me to validate that the response is, indeed, valid JSON [5].
Thus, while LLMs could be useful in prototyping a pipeline which performs entity recognition as one of its stages, the final result may be more practicable with a traditional supervised learning model. Of course, you'd need a labelled training dataset for this, but here's a saving grace for the LLM: it can generate that data for you [6]!
Not everyone is using ChatGPT (yet?)
Outside of the headlines and press releases by the world's largest research organizations, who are using LLMs to solve NLP problems end-to-end, many companies aren't there yet, even if their use case could benefit from an LLM. Some of them are figuring out what this technology can do, others are even building their first LLM-powered solutions, but many are realising the challenges with bringing such a product into production.
Best practices and established design patterns don't yet exist for developers to turn to. Many new tools designed to help build LLM systems are not yet robust enough to be relied upon. Issues like complexity and latency when making multiple LLM calls, and security when connecting LLMs to external tools, can massively slow the pace of development. Finally, difficulties figuring out how to evaluate an LLM's outputs make it harder to measure the value of the solution, and thus, harder for some companies to justify the continued R&D effort on solving particular problems with LLMs [7].
You can't throw out all the old solutions at once
You know the saying, "if it ain't broke, don't fix it?" Plenty of companies have NLP systems which are working just fine. Those companies have no incentive to start over with Gen AI, and if they do decide to experiment with LLMs, it'll likely be to tackle brand new problems first (problems which proved unsolvable with traditional methods, perhaps). Thus, it will take quite some time (if it happens at all) before existing solutions using "traditional" NLP techniques become entirely obsolete. And in the meantime, these companies will __ need to maintain their existing NLP systems in production. That means they'll still need employees who know how to debug text preprocessing pipelines, evaluate NLP models, and maybe even extract new features from text data, to continually improve their existing systems.
LLMs need NLP too, ok?
Training or fine-tuning your own LLM will require text data which has been gathered, cleaned, and formatted consistently. You'll need NLP skills for that. If you want to filter the input data, prompts, or model outputs for toxic content, you'll need NLP for that, too, as you'll be implementing something like keyword filters or content classification models. The same goes if you want to apply quality control to an LLMs responses, pulling a human into the loop in cases where the quality is detected as low: tasks like this are still sometimes done with traditional NLP techniques and supervised models.
How about building a Retrieval Augmented Generation (RAG) application? In this architecture, you provide a language model with a document knowledge base, from which it will draw information to create its answers. For this, you'll likely need to experiment with embedding methods, document segmentation strategies (known as "chunking"), and how much overlap should be allowed between document chunks, such that all the relevant information is preserved for the LLM, but the content window isn't immediately filled. NLP skills can help you figure out those issues, too [8].
How NLP and LLMs can work together
I actually dislike the term "age of GPT." To me, it's indicative of the narrow focus so many people have on Large Language Models, and the blind spots this casts over a robust toolkit of tried and tested NLP methodologies. It seems to imply that, for the foreseeable future, LLMs will be all we need to solve all our problems. But I think this attitude comes from a place of solutions looking for problems, and that's how you end up with AI-powered coffee machines that don't actually need any AI, and genuine (as in, I definitely did not make this up) articles asking whether AI will change the way we fridge forever [9]?
In the end, nobody knows for sure how this is all going to play out. But my money's on LLMs becoming just one useful tool of many for working with text data. So for anyone dreaming of getting into NLP, I've got some mixed news for you. Yes, you'll be able to play with all the new toys, but you're also going to have to learn regex, too [10].
Some final thoughts, and useful resources
1. Turns out that, by sheer luck, I stumbled into NLP at the perfect time. The entire field was having its own ImageNet moment. Maths with words was cool, neural networks had (very helpfully) developed selective amnesia, and everyone was raving about Sesame Street. It was fascinating, fun, and flattering to see how everyone wanted to learn what I was learning, and every company was hiring for skills like mine. I started teaching, speaking and writing about NLP, and even wound up trying to explain chatbots to Australia's most famous comedian, Jim Jeffries. But nevertheless, people like my parents still couldn't wrap their heads around what I was doing. Now they hear about this stuff in the news almost daily.
2. Hey, as long as no-one thinks I mean the other NLP, I'm happy with that.
3. By the way, I'm also responsible for our new chapters on Foundation Models, Generative AI, and LLMs, and they're going out of date faster than I can finish writing them! (See, for example, this pesky paper introducing a fast and performant new architecture for foundation models, or this one, proposing combining LLMs with SymbolicAI, which will probably be out of date before I even finish reading it!).
4. Of course, by "writing regex parsers," I mean, "rewriting and debugging regex parsers, and generally questioning the life choices which landed you in that situation in the first place."
5. Entity recognition is generally formulated as a sequence labelling task, and the model architecture will be fixed to ensure that output is one label per token in the original input sequence. An LLM, by contrast, returns a string, which must be parsed and validated. Here's a tutorial from a former coworker demonstrating exactly that that, i.e. how to "Enforce and Validate LLM Output with Pydantic."
6. Matthew Honnibal of NLP library spaCy makes a great case for using LLMs to train their own replacements, and even provides step-by-step recommendations for doing so, in "Against LLM maximalism". Highly recommended reading.
7. For a more concrete example of the challenges of bringing an LLM-based system into production, consider the case of function-calling LLMs, or agent systems. These can be incredibly useful, but bring plenty of operational hurdles with them, including managing complex workflows, maintaining satisfactory levels of security, and evaluating the effectiveness of the system. Ben Lorica of Gradient Flow covered all these and more in this piece, "Expanding AI Horizons: The Rise of Function Calling in LLMs."
8. Another great article, "Driving Operational Efficiency at Adyen through Large Language Models," talks about exactly this: using NLP techniques to support LLM-based applications. Check out the section on RAG, for example.
9. I've actually seen some really practical use cases for AI in fridges. It's just this phrasing which makes me cringe.
10. As ChatGPT so eloquently put it, b(?:[Ss][uc]{2}[kx]s?stos[bB]es[yY]ou)b