Building a marketing data science team from scratch
It is not about fancy ML. Assess what the biggest problem is. Solve 20% of it. The rest will follow.
In 2022, I had been working at Skyscanner for a couple of years and as a manager around 4. I got a sudden opportunity of moving completely outside of my domain knowledge and tasked with building a marketing Data Science team from scratch. This was no small ask, as Data Science hadn't had a team working under the marketing umbrella before. This came with a few daunting implications for me:
- Who are the main decision makers and how do I establish a good working relationship with them?
- Where do I even start? What could the first projects be?
- I don't have a team, so how do I add value to influence more headcount growth?
- I have no Marketing domain knowledge, what can I do to accelerate this learning?
In this blog, I want to share with you what did I do over the course of 2 years to build a data science team in marketing from only myself to a team of myself + 6 data scientists.
Historical context: Why did the company want to finance a new Data Science Marketing team?
At the time the request of building a marketing data science team came, our Data Science discipline at Skyscanner had 4 main teams focusing on (1) recommendations and ranking – primarily on flights, our core product (2) optimising our search engine (3) experimentation (4) helping grow our internal ads platform. We were roughly a team of 20 Data Scientists, and had proven our value in these four areas. The company wanted to expand the impact we were having, and marketing was the main candidate.
Why did Skyscanner want to add Data Scientists into the marketing tribe?
Marketing is one of the key pillars for growth. If your product is loved by travellers and users return, you have a solid baseline for growth, because "all you have left to do", is bring more travellers through the door.
Now, you can't just simply splash money to the problem. There is a reason why our conversion rates and return rates were high. The segment user base who used our products had certain characteristics. When you go into the wild to look for more customers, the customer mix changes.
What marketing wanted to do was togrow sustainably. And that is literally what I was told: "Hey, we need help building a marketing data science team to aid us in our journey of sustainable growth". Off you go…
Phase 1. Figuring out the biggest problem to focus on.
In phase 1, my goals where:
- To understand the whole marketing ecosystem and map possible projects that Data Science could help on.
- Build a template to assess how much off was each team from targets, how difficult would it be for Data Science to help and the potential opportunity that us helping could unlock.
- Once a project was selected, build the relationship with the primary stakeholders on that area.
- Finally, finish with a mission statement for the new data science marketing team, so that the marketing tribe knew what Data Science would be focused for the next 2 years.
Understanding the marketing ecosystem
At Skyscanner, the marketing tribe had 4 main pillars: SEO, Paid, Affiliate growth and Lifecycle marketing (Retention). Each of these pillars had a wide range of projects. We are talking here about well into the 100+ people working in the area if you count engineers, marketeers, designers, product and analysts. Below you can see a summary diagram audit I created to paint the marketing ecosystem picture.

Opportunity size template
One thing was clear in my mind: I wanted a focused and deeply knowledgable team at the end of these 24 months.
Building a marketing data science team was for me a creating a centre of excellence for a specific area of marketing, not building a marketing data science team to cover all possible marketing areas.
Given these many projects, I knew I couldn't serve them all, and if I wanted to scale this new team to 6 people, I wanted the 6 of them leaving and breathing under the same pillared umbrella. The time to expand to other areas in marketing would come, but adding value and making a reputation of what we could do was the most important thing for me at the beginning.
This is why I had to really nail down what wouldn't we support. The statement " would't we support" is very important, because, once I had committed to helping one of these pillars, I had to also run an exercise of explaining why the others wouldn't have data science resources in, at least 24 months (that can piss a few people off…).
For this, I used a really simple framework to guide me in my decisions.

What was my criteria for t-shirt sizing these 3 dimensions?

Note that the "Potential opportunity" and "DS efforts" column include figures such as 1m or 2 quarters. Take these as "orders of magnitude" references. My goal was to differentiate a S or XL t-shirt size easily.
Data Science in Paid marketing
With the sizing exercise you have read above, the area which had the highest potential for us was helping in Paid marketing. Let me show you some thinking around this decision using the described T-shirt sizing.
- Help required (XL). Skyscanner was (and is) great at SEO. Our SEO traffic is amazing, but we hadn't optimised our paid traffic. Paid marketing had many open fronts, but all of them faced the same problem: "How can we automate bidding to grow paid traffic in a sustainable way".
- Potential opportunity (XL). Paid marketing represented an annual cost in the tens of millions. Imagine if Data Science could optimise this spend to make it profitable and set it on growth mode.
- DS efforts (XL). At this point in time, any project was a massive effort from our side. We were new to the space and didn't have Data Science tooling for marketing solutions. We knew we had to be here for the long run to get a good ROI on our work.
Why weren't SEO or Lifecycle marketing chosen?
- SEO was a super complex space too. But it was well resourced from a technical point of view. SEO were on target with their goals and whilst a 1% improvement in SEO traffic would translate to the millions of pounds, paid marketing had bigger bottlenecks we could help with.
- Lifecycle marketing was an area we could have helped faster. Other data science teams had already integrated some of our models into Lifecycle marketing's tech stack, so Lifecycle marketing requests could potentially be picked up by other colleagues. In addition, the reality is that, paid's tens of millions spend dwarfed the potential opportunity we had if we compared it to Lifecycle marketing.
How to build the relationship with our Paid marketing stakeholders?
Having chosen paid marketing as our data science focus, it was really important for me to get a solid working relationship with the relevant stakeholders. What worked really well for me was:
- Organise 1–1s every 2 to 3 weeks with the most senior people in the whole paid marketing organisation (or in reality, get to the people making the hard trade-offs). For example, the Senior Directors of product, marketing and engineering.
- Organise weekly project calls with product, engineering and marketing. Show them a 1 quarter roadmap and delivery against it. This helped get everyone aligned on delivery and flag where we needed help.
Our mission statement then became super clear
Our Data Science Marketing team mission is to optimise our most important bidding solutions to grow our Skyscanner audience in a sustainable way.
Phase 2. Proving our value in Hotel Price Ads
In phase 2, my main goals where:
- Hire the first 2 data scientists.
- Emphasize to stakeholders that the first quarter's worth of work would be on tooling, foundations and learnings. Be up-front that positive results would not happen until the following quarter. It helps so much being clear. Stakeholders normally understand this and are supportive.
- Get the tooling and testing ready. Can Data Science be self-sufficient on deploying our solutions? If not, this will hamper future accelerated growth as would always have a dependency to go to production.
- Proof value in our PoC. If we can proof and demonstrate value, this opens the door to getting more headcount to support more projects.
What was the chosen project?
Data Science was tasked with growing our acquisition of hotel users through Google and Tripadvisor. We had to develop:
- A bidding algorithm that decided "how much should we bid for a specific hotel & itinerary"
- A supply selection algorithm that decided "which hotels & itineraries should we be bidding on?"

All of this had to done programatically. If we proved value by doing manual changes in 2 markets, it wouldn't have mattered. At Skyscanner we work with the premise of scalability. Therefore, we needed programatic deployment and machine led algorithms to take decisions for us.
We proved value!
In the following screenshot, you can see a snapshot of how our work looked like in terms of transactional growth coming from Tripadvisor. With this, I want to show you how the project evolved, and why it was important to keep constant communication with stakeholders.
- Phase 1. ROAS and volumes all over the place. But expected as we were learning to integrate with different systems and testing ML ideas.
- Phase 2. Clarity on what we wanted to deliver in the following quarter. You can see that we ended the quarter with great success both on aligning with our target ROAS whilst increasing conversions.
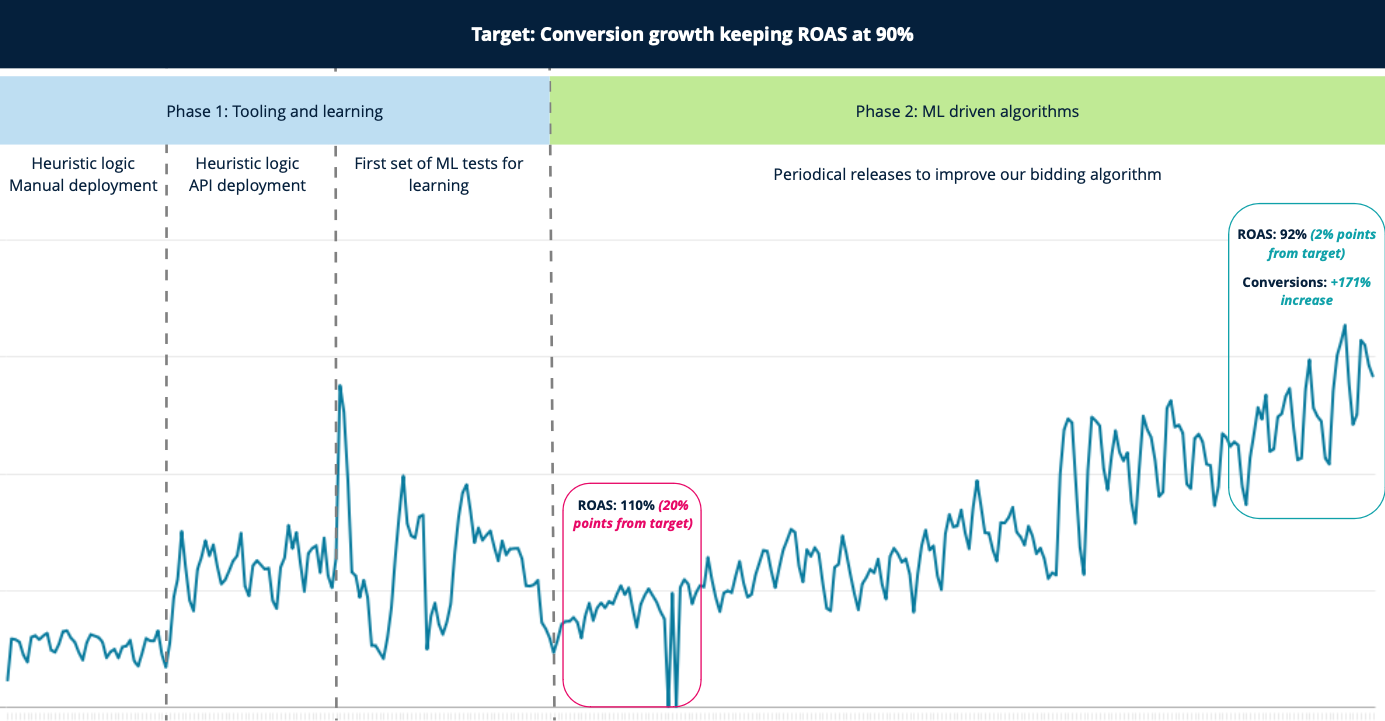
Phase 3. 3x team growth to own 3 projects.
In phase 3, my main goals were:
- Grow the team from 2 Data Scientists to 6
- Scale our existing hotel bidding project with best MLOps practices.
- Open 2 new projects in the bidding space.
Having proven that Data Science could add value to the paid marketing space, and being much more knowledgeable in the area, we were ready to open new projects and scale the team to 6 Data Scientists.
My goal was to ensure that no data scientist worked on their own, so we paired up to work on 3 different projects:
- Optimising bids for hotel price ads (existing project, but keep improving our bidding algorithms whilst opening new partnerships).
- Optimising bids for SEM. This was super big, and conceptually, similar to our first project. This allowed us to hit the ground running on the tooling we had. But, we did approach SEM problems differently to hotel price ads, so it took some time to show the same sort of growth results.
- Optimising paid APP installs. This is a massive project, because we are not only dealing here with a bid optimisation problem, but with a privacy problem. iOS and Android were tackling the privacy world, so we could not pin down the relationship between our internal transaction and the specific upstream click we were buying. This is still an ongoing project and most probably one which we will need more DS brain power behind.
Summary
As you can see, the key element in me growing the team was identifying a problem space which Data Science could own, and which had multiple similar projects. This way, whilst our beginnings were slow, we accelerated massively later on because we were experts in the area of bidding.
In addition, keep in mind the power of your network. I managed to establish a great relationship with very senior stakeholders, and when things were not going as expected or we needed to remove blockers, they were there to help. Without them, this team growth wouldn't have existed.
Further reading
Thanks for reading the article! If you are interested in more of my written content, here is an article capturing all of my other blogs posts organised by themes: Data Science team and project management, Data storytelling, Marketing & bidding science and Machine Learning & modelling.
Stay tuned!
If you want to get notified when I release new written content, feel free to follow me on Medium or subscribe to my Substack newsletter. In addition, I would be very happy to chat on Linkedin!
Get notified with my latest written content about Data Science!