Causal Validation: A Unified Theory of Everything

Introduction
Causal Inference is an emerging field within machine learning that can move beyond predicting what could happen to explaining why it will happen and it doing so offers the promise of permanently resolving the underlying problem rather than dealing with the potential fallout.
One of the key components of a causal model is a "Directed Acyclic Graph" (DAG) which captures the cause-and-effect relationships between variables and events in a simple visual format but the main issue with DAGs is that they are typically constructed subjectively by the domain experts.
Hence there is no guarantee that the DAG will be correct and if it is incorrect the calculations and conclusions of a causal inference model are likely to be wrong.
Causal Validation is the term used to describe the process of checking a DAG against the underlying data it represents with the objective of identifying any mistakes or inconsistencies and fixing them and if this can be done reliably it will ensure that the conclusions of causal inference and the associated actions and changes will lead to improved impact and outcomes.
The Problem
There are different ways that a DAG can be "wrong" and whilst it is possible to find references in the causal literature to the different types of error, there is little available on how to deal with causal validation holistically.
The Opportunity
If a unified causal validation algorithm can be developed which can find and fix any type of error in a DAG, this would reduce the reliance on the subjective knowledge of domain experts and significantly increase the reliability of causal inference models.
The Way Forward
The individual algorithms for the different categories of DAG errors can be combined, integrated and tested to produce the illusive unified theory of everything for causal validation.
Background
Like all other machine learning problems causal inference projects start with some data which will consist of a set of features …

The purpose of a Causal Inference model is to establish the true effect of a treatment (typically labelled "X") on an outcome (typically labelled "Y") when the effects of all the other variables have been accounted and adjusted for and the starting point is for the domain experts to construct a Directed Acyclic Graph to capture their subjective understanding of the causal relationships …

For the purposes of the exploration of unified causal validation a fictitious example has been selected that is simple enough for an example and complex enough to contain different types of causal relationship and hence the node names (X, Z1, Z3 etc.) have no meaning in the real-world.
However, it might aid understanding to consider that X might indicate the level of taking a new drug, W might be the impact of the drug on blood pressure in the sampled patients and Y may be the effect on patient recovery.
In this example the direction of the arrows make sense because clearly taking the drug could cause changes in blood pressure, but not the other way around and the same goes for changes in blood pressure causing changes in patient outcome with the opposite being very unlikely.
At this stage of our example the data has been captured and a DAG has been constructed that represents the proposed relationships.
As this is a test case designed to illustrate causal validation it is certain that the DAG is correct because the DAG and data were synthetically created together, but what if this were a real world problem?
What if the domain experts had come up with a different DAG that was wrong and what type of mistakes might they have made?
Missing Edge Errors
The first type of error are missing links or edges (note that the terms link and edge are interchangeable and mean the same thing – the connection between one node / feature and another).
It is possible that the domain experts have completely omitted some edges in the DAG that actually exist in the data. For example there could be a causal link between Z3 and Y that exists in the data and the real world that has not been represented in the DAG …

In this example the DAG contains a missing edge error and if the edge or link exists in the data and is missed from the DAG then all the calculations and conclusions of the causal model will be wrong.
Spurious Edge Errors
The second type of error are spurious edges i.e. an edge that has been identified and represented in the DAG that does not exist in the real-world or the data.
For example it may be that the the domain experts have proposed that there is a causal link between Z1 and W and that this link has been created in the DAG but it does not actually exist in the data …

If an additional causal link has be identified in the DAG that is not real and does not exist in the data it will lead to errors and mistakes causing the conclusions drawn from the results of the causal calculations to be wrong.
Reversed Edge Errors
The final type of error are reversed edge errors i.e. the domain experts have correctly identified an association between two features but have got the directionality wrong.
For example the domain experts may have proposed a causal link between X and Z3 that has been captured in the DAG when the correct edge / link is in the opposite direction from Z3 to X …

An edge that is in the wrong direction in the DAG compared to the true direction of causality will also lead to the wrong answers being produced from by the causal calculations.
Causal Validation – Detecting and Correcting Edge Errors
Causal Validation is the concept of detecting errors in the DAG and correcting them such that the DAG is an accurate reflection of the causal relationships that lie hidden in the data.
The first stage is to understand what type of errors can exist in the DAG and as outlined above there are exactly 3 types of error – missing edges, spurious edges and reversed edges.
There are no other categories or types of error so solving the causal validation conundrum for each of the 3 types should lead to a unified theory of everything for causal validation.
The Importance of Independence and Dependence
It turns out that Independence and its opposite expression Dependence are critically important in the detection and correction of all 3 types of errors in a DAG.
This is one proposal for a formal definition of Independence …
Independence between two random variables refers to a fundamental concept where the occurrence or value of one random variable does not influence or provide any information about the occurrence or value of the other random variable
And it can be applied to our initial DAG (the one with no errors in it that is taken to accurately represent the data) …

It is intuitively clear from the DAG that nodes Z1 and Z2 (in the DAG) or features Z1 and Z2 (in the data) are not connected.
It follows that if the value of Z1 changes the value of Z2 will not change as there is no direct or indirect link between them.
Z2 is said to be independent of Z1 in this case and this can be represented in the following expression (noting that the ⫫ symbol is referred to as a "double up-tack") –
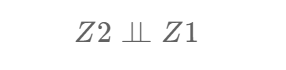
The concept of the independence of Z2 from Z1 can also be visualised and understood by scatter plotting the instances of Z1 and Z2 from the rows in the data and adding a line to the chart that represents the co-efficient of the relationship between the scatter points …

It is easy to see that there is no relationship between Z1 and Z2. The co-efficient is 0.03 (which is close to zero) and it can be seen that increasing Z1 by 1 or 2 or 4 is not going to yield and increase in Z2 because the co-efficient of the line is virtually flat.
Also the pattern of the points in the scatter chart are a cloud rather than a straight line all of which helps to illustrate that Z2 is independent of Z1.
Whilst Z2 is independent of Z1 there are other instances in the DAG of the opposite relationship – dependence …

It is intuitively clear that that W is dependent on X and this means that a change in the value of X will result in a change in W which can be represented by the following expression (noting that the ⫫̸ symbol is referred to as a "slashed double up-tack") …

Just as with independence, understanding the concept of dependence can be aided by visualising the relationship between the features in the data in a scatter chart …

In this instance it is easy to see that there is a relationship between X and Y. Increasing the value of X by 20 or 40 or 60 clearly increases the value of Y and the co-efficient of the line that best fits the scatter points is 6.77 so increasing the value of X by 1 will increase the value of Y by 6.77.
As opposed to the independence example, this time the scatter points clearly form a line rather than a cloud and this is the tell-tale sign of dependence.
A more detailed exploration of independence and dependence is beyond the scope of this article but if you are interested and if you would like to learn how to add an extension function to the pandas DataFrame
object to easily enable the calculation of any independence expression please check out this article …
Understanding Independence and Why it is Critical in Causal Inference and Causal Validation
Detecting Missing Edge Errors
Missing link errors are detected using an algorithm that can be tracked down in several different sources in the causal literature and online that is based on independence …
A node is independent of its predecessors when conditioned on its parents
This can be explained by considering the following DAG that has a mistake in it – the link from Z3 to Y is missing where it is known (because the data was synthetically created) that the link exists in the data …

The missing link validation algorithm will iterate over every node carrying out the independence test.
For example, when the algorithm gets to node Y the statement "A node is independent of its predecessors when conditioned on its parents" becomes Y is independent of nodes X, Z1 and Z3 (the predecessors of Y) when conditioned on nodes W and Z2 (the parents of Y).
This statement can be simplified into the following expression using the independence notation explained above …

Taking one more step the expression can be extended to its full form which is to summarise the statement Y is independent of X, Z1, Z3 given W, Z2 in the graph implies that Y is independent of X, Z1, Z3 given W, Z2 in the data …

This condition can then be tested using the .dependence()
extension method of the DataFrame
class that was introduced in the section above …

The results show that node Y is independent of nodes X and Z1 but Y is not independent of Z3 because the co-efficient is for Z3 in the regression is 3.5012 and the p-value is 0.000.
This means that the independence test for node Y has not only shown that there is an error in the DAG but has also shown exactly where that error exists i.e. that there is a dependency between Z3 and Y in the data that needs adding to the DAG in order to correct it.
The DAG can now be "fixed" as follows …

And just like that a DAG that the domain experts proposed that contained a missing link error has been identified as invalid and fixed using Causal Validation!
This has been a very quick overview of using independence to detect missing links. For a full and detailed explanation please see the following article …
Understanding Independence and Why it is Critical in Causal Inference and Causal Validation
Detecting Spurious Edge Errors
In all the months I have spent researching causal validation I never managed to find a single instance of the literature exploring spurious link errors or any proposal for an algorithm to address this type of error.
In fact I have never found any material stating that there are exactly 3 types of error so the conclusions about the possible error types and the proposed algorithm for spurious links come from my research and lots of trial-and-error using Python code.
The idea for a spurious link algorithm came to me as I completed my Python implementation of the missing link algorithm as I pondered whether that was sufficient to "fix" all errors and I reached the conclusion that a unified theory of everything for causal validation was a long way off, but the spurious link algorithm was fairly straightforward.
I adapted the algorithm for missing links to arrive at this statement …
"A node is dependent on its parents"
Here is an example DAG where a spurious link error (i.e. a link that appears in the DAG but does not exist in the data) has been deliberately introduced …

As in the previous test, the spurious algorithm test will iterate over every node, this time carrying out a dependence test (as opposed to an independence test for missing links).
When the algorithm gets to node W the statement W is dependent on nodes X and Z3 (the parents of W) in the graph implies that W is dependent on X and Z3 in the data …

Again this condition can then be tested using the .dependence()
extension method of the DataFrame
class …

The results show that node W is dependent on node X but W is not dependent of Z3 because the co-efficient is for Z3 in the regression -0.1580 (small) and the p-value is 0.566 (non-zero).
This means that the dependence test for node W has shown that there is an error in the DAG and has shown that that error is a spurious link from Z3 to W which needs to be removed to "fix" the DAG …

Detecting Reversed Edge Errors
A solution to detecting reversed link errors eluded me for a long time and initially I thought it was impossible to solve because if the co-efficient between Z3 and X (for example) where 2.5 then the co-efficient between X and Z3 is the inverse …

… and how is it possible to work out which of these implies directionality and causality?
There are some clues and indications in the available causal literature and online articles, but nothing comprehensive and complete. My research has led to the following conclusions and solution …
All DAGs have a treatment (X in our example) and outcome (Y in our example) and have at least one front-door path (a route through the DAG from the treatment to the outcome). They may also have additional nodes and additional links, but they must be acyclic (non-cyclical) and no nodes may be orphaned (cut off).
The various paths can be broken down into a series of lower level units called junctions which consist of a start, middle and end node with exactly 2 links, one from the start to the middle and another from the end to the middle. Junctions have exactly 3 types – chains (X -> Z -> Y), forks (X <- Z -> Y) and colliders (X -> Z <- Y).
Of these 3 types colliders are critically important because they can be detected using a dependency test whilst chains and forks cannot be differentiated as they both produce exactly the same set of results.
This can be summarised as follows …

… which reads as follows …
- In a collider Y (the outcome) is independent of X (the treatment)
- In a chain or a fork Y (the outcome) is dependent on X (the treatment)
The available literature usually stops at this point but there is one further step. In a collider Y is independent of X but only if there are no direct or indirect connections between X and Y. If there are any connections then "messages" or changes in X leak through to cause changes in Y which makes them dependent again.
This gives rise to a sub-set of colliders called "v-structures" which are simply colliders where the start and end nodes are not connected. This can be easily visualised by considering all of the colliders that exist in the example DAG …

There are 5 colliders but two of them have direct connections between the start and end nodes (Z3 ->Y <- Z2 and <- Z1 -> X <- Z2) which is termed "adjacency".
Removing the "adjacent" colliders gives a visualisation of the 3 v-structures in the example DAG …

The 3 v-structures can be alternatively represented as follows …
- W -> Y <- Z2
- W -> Y <- Z3
- Z1 -> Z3 <- Z2
The reason for taking the time to understand v-structures is that they canbe detected in the data and this enables the construction of an algorithm built on those detections that can indicate the directionality of links and hence the detection of reversals. Here is the pseudo-code for the algorithm …
- Iterate around all the edges in the DAG and for each edge …
- Create a new DAG by reversing the current edge.
- If a v-structure has been destroyed and that v-structure does not exist in the data then the current DAG is wrong and the edge needs reversing.
- For each v-structure in the DAG that has been destroyed by the edge reversal execute this dependency test …

- If this test passes then the edge that is currently being tested is wrong in the DAG and needs to be reversed.
- If a v-structure has been created and that v-structure exists in the data then the current DAG is wrong and the edge needs reversing.
- For each v-structure in the DAG that has been created by the edge reversal execute this dependency test …

- If this test fails then the edge that is currently being tested is wrong in the DAG and needs to be reversed.
By implementing this algorithm in Python using the DataFrame
.dependency()
extension method reversed links can be detected and corrected.
For example if, during the iteration of edges / links to test for reversal, the current edge selected for testing is Z1 -> Z3, the following steps would be followed …
- Reversing Z1 to Z3 destroys exactly one v-structure in the DAG (Z1 -> Z3 <- Z2)
-
A dependency test can then be carried out against the data as follows …
Output from Python Code for Dependence, Image by Author
The results show that Z2 is not dependent on Z1 in the data because the co-efficient is very small (-0.0253) and the p-value is non-zero (0.485).
The dependency test has not passed (i.e. has not returned True
), hence it can be inferred that Z1 -> Z3 has the correct directionality in the DAG and should not be reversed.
If steps 1–4 are iteratively applied to every edge / link in the DAG then the directionality of all edges and hence the entire DAG can be validated.
If you would like to know more this article contains a more detailed explanation of v-structures with more code samples …
Understanding V-Structures and the Role They Play in Causal Validation and Causal Inference
Combining Missing, Spurious and Reversed Link Errors
The previous section showed that there are exactly 3 types of errors in a DAG – missing edges, spurious edges and reversed edges – and also showed how dependency tests can be implemented in Python to detect all 3 types.
In theory then a "Unified Theory of Everything" for causal validation can be achieved simply by sequentially combining all 3 types of tests, so let's start by proposing a unified causal validation algorithm as follows …
- Test for Missing Edge Errors
- Test for Spurious Edge Errors
- Test for Reversed Edge Errors
Intuitively this algorithm should work but when it is implemented in practice and robustly tested it consistently fails and the reason is as follows …
Let's start by assuming that the DAG contains an error – a missing edge between nodes Z2 and Z3 …

The first step i.e. test for missing edges will identify the missing edge but on step 3 the directionality of the v-structure at Z1 -> Z3 <- Z2 cannot be tested because it does not exist because of the missing edge.
This is easily resolved by amending the unified algorithm as follows …
- Test for any Missing Edge Errors
- Make a note of any errors and fix them
- Test for Spurious Edge Errors
- Make a note of any errors and fix them
- Test for and Fix Reversed Edge Errors
- Make a note of any errors
The first issue has now been resolved. Step 1 will re-instate the missing edge at Z2 -> Z3 and then the reversals at step 3 will be correctly tested, but there are more serious issues.
Let us now assume that the DAG has a different error. In this example the edge between X and W has the wrong direction and is reversed …

Step 1 which tests for missing links will iterate over all nodes executing the condition "A node is independent of its predecessors when conditioned on its parents".
If the DAG were correct (i.e. the edge between X and W were X -> W) then this condition resolves as follows …

However, because this DAG contains an error X is no longer a parent of W (in fact W is now a parent of X). That means that any missing links between the predecessors Z1, Z2, Z3 and W will not be detected and in fact the set of missing link tests executed for all nodes in the iteration based on the new sets of parents and predecessors will all be completely wrong.
In this particular instance a satisfactory outcome can be obtained by changing the order of the sequential tests to identify and fix any reversed edge errors before the missing and spurious edge tests. However, this only works because, in this test, there is prior knowledge of the errors, i.e. a single reversed edge, because that was how the test was created.
In a real-world causal inference problem the data team would be completely unaware …
- If any errors are present in the DAG and …
- The types of errors (missing, spurious or reversed).
For example in one DAG there could be a single spurious edge error and in another there could be a missing edge and a reversed edge etc.
This invalidates any attempt to produce a unified theory of everything for causal validation by changing the order of the sequential tests.
Another solution must be sought.
A Proposed Unified Theory of Everything for Causal Validation
At this point in my research I came close to giving up on a unified theory of everything for causal validation.
I had developed algorithms for successfully detecting each of the 3 types of errors which worked with usable reliability provided the DAG being validated only had the types of errors that matched the algorithm and no others. In reality this is useless as it can never be known beforehand what the errors are.
When the sequential algorithm had failed I began to suspect that a recursive solution might work. I have always loved recursion as a programming construct; it is both elegantly simple and fiendishly complex at the same time but once the complexity is mastered there are a certain class of problems that can be solved in a few lines of elegant code that could take 100's of lines of non-recursive code.
Recursion in programming simply refers to a function that calls itself. It is very common to write functions that call other functions, much less so for functions to call back to themselves.
Recursive solutions are often used in algorithms that traverse, parse or modify tree-like structures (like a family tree) and I have found the best way of understanding them is to consider each call back to itself to be a call to a new function that is an exact copy.
If that still does not work in achieving and understanding of recursion, spending time debugging a recursive function and stepping through all the calls will help.
This then is the pseud-code for the proposed unified theory of everything for causal validation …
fix_dag(DAG):
- If DAG is valid or if the maximum number of recursive calls have been reached terminate the recursion and return DAG
- If any missing edges are detected fix them and call fix_dag(FIXED_DAG)
- If any spurious edges are detected fix them and call fix_dag(FIXED-DAG)
- If any reversed edges are detected fix them and call fix_dag(FIXED-DAG)
At first glance this looks almost identical to the sequential algorithm but it is very different.
It no longer relies on the order of the tests even though their is an order insider the recursion because the recursive algorithm will continue trying a fix and evaluating if the "fixed" dag is valid until the accumulation of changes produces a valid DAG (or the maximum number of recursions is reached).
The errors in the original DAG can then be inferred by simply comparing the final fixed DAG with the original DAG and identifying the differences.
The Python Code for the Unified Theory
It is impractical to show all of the code behind the scenes as it runs to over 2000 lines, but here is the code for fix_dag
and difference
which implements the algorithm …
Testing the Unified Theory
Before testing the proposed algorithm to destruction there is one more issue to consider.
The previous articles that explore the 3 types of errors and their individual detection algorithms built a case for providing "hints".
Hints are assertions passed into the algorithms that certain edges within the DAG are correct and instruct the algorithm not to check them.
It turns out that hinting is critical to the operation of the 3 types of test because in reality there can be different DAGs that cannot be distinguished between with any amount of checking and testing.
The causal literature refers to a set of DAGs that cannot be differentiated as "Equivalence Classes" and the only defence is to provide enough hints to eliminate the equivalent classes from the search space.
There is another upside to hints in that they significantly reduce the permutations that must be checked.
Part of the reversed edge algorithm searches for all combinations of reversed edges to identify a valid dag. With 11 edges the search space is 2 to the power 11 = 2048 but increasing the number of edges by just 1 give 2 to the power 12 = 4096 and so on.
Python is an interpreted language and performance will degrade exponentially as the number of edges increased, hence hinting edges will significantly reduce the processing time.
Hinting Edges in the Example Test DAG
There are some principles relating to DAGs that help to develop a set of hinted edges.
Firstly any edge that would make the DAG cyclic must by definition be excluded and the best approach is not to bother testing for any set of changes that would result in a cyclic DAG.
Also there are clues in the nature of the treatment and effect. Generally the paths must flow from the treatment towards the effect and by definition the effect should not be causing events. Hence edges should flow into the effect node and not the other way around. There are some exceptions to this but it is a good rule of thumb and likewise causality and hence edges should be flowing away from the treatment.
Further clues can also be obtained by considering the exogenous nodes. These are Z1 and Z2 in our example. Exogenous nodes are the "inputs" to the DAG and the causal model and all other nodes are built from an un-seen set of structural equations (e.g. Z3 = 3 xZ1 + 1.5 x Z2+ ε).
It is possible that the identification of exogenous nodes and variables is incorrect but it is unlikely that the data and domain experts will not know what variables are the inputs to the DAG and the model.
Hence it is highly likely that causality and edges flow away from the exogenous variables / nodes.
Lastly there is the concept of transitivity. In our example DAG X causes W and W causes Y. If X were a drug, W blood pressure and Y the patient outcome it is unlikely that the drug directly causes the patient outcome.
It is much more likely that the drug changes something in patients that in turn improves outcomes. It follows that it is generally likely that where the domain experts have identified any transitive causal relationship like Z2 -> Z3 -> X it is unlikely that Z2 causes X directly whatever Z2, Z3 and X represent.
In summary these properties can give clues that help identify hints …
- Acyclicity
- Exogeneity
- Transitivity
This can also be augmented by trial-and-error as follows …
- Run the algorithm
- Review the results
- If the algorithm has suggested an error that the domain experts disagree with, add it as a hint and go back to step 1
Using the approach of Acyclicity, Exogeneity, Transitivity (because the example DAG is fictitious removing any possibility of help from domain experts), the following is a reasonable set of hints to provide to the proposed algorithm implementing the "Unified Theory of Everything for Causal Validation" that have been used in the tests.
Note that where a highlight overlays an edge (e.g. W -> Y) it hints that the edge is correct and where a highlight overlays a space (e.g. Z1 -> Z2) it hints that the absence of an edge is correct. Note also that absent edges can be hinted in both directions which is why some edges appear to be hinted twice (e.g. Z1 -> Z2 and Z2 -> Z1).

Testing the Algorithm
The next stage is to robustly and exhaustively test the algorithm for different combinations of the 3 types of errors and to measure performance.
The test harness works as follows:
Preparation
- Choose the types and numbers of errors (e.g. 1 missing edge, 0 spurious edges, 1 reversed edge)
- Generate all possible combinations of valid DAGs containing this combination (i.e. all DAGs with exactly 1 missing edge and 1 revered edge) excluding the hints
- Choose n tests from the valid combinations (for example 10 tests)
Execution
- For each chosen test …
- Generate an error DAG by starting with the test / example DAG and applying the changes to make it invalid (i.e. remove 1 missing edge, reverse 1 reversed edge)
- Generate a new set of data FOR THE VALID DAG (i.e. not the one with the errors)
- Call the recursive fix_dag function to correct the error DAG and to identify the errors given the generated data
- Compare the identified errors that were used to create the error DAG
Evaluation
- Count the percentage of missing, spurious and reversed edges that were individually correctly detected
- Count the percentage of tests were all 3 – missing, spurious and reversed edges were all correctly detected
Test Examples
Here is an example of 4 edges that can be deleted to produce valid missing edge tests …

And here is an example of 3 non-existent edges have been added to produce valid spurious edge tests …

Lastly here is an example of 3 edges that have been reversed to produce reversed valid edge tests …

In the above examples the term "valid" test is deliberately used. There are other edges that can be removed, added or reversed but any changes that would make the DAG cyclic or that have been included in the hints are removed.
Test Results
Here are the test results for a range of combinations of the 3 types of errors .
1 of Each Type Separately
The first batch of tests is to try 1 each of missing, spurious and reversed edges. The results are as follows …

The results are that in a DAG containing a single missing edge the single error is detected with 100% accuracy. The same accuracy is found for a DAG with a single reversed edge and a DAG containing a single spurious edge is detected with 66.7% accuracy.
The spurious edge result does not sound promising but the number of valid tests for a single spurious link is only 3 and the algorithm has difficulty detecting a spurious link from Z1 -> W so put another way there is only a single failure for spurious edge detection and no failures for the other types.
2 Errors of Different Types
Testing for 2 different errors performs as follows …

If a DAG has 1 missing edge error and 1 spurious edge error, the unified validation algorithm correctly identifies the errors in 100% of test cases. 1 missing edge error and 1 reversed edge error are correctly identified in 78% of tests and 1 spurious and 1 reversed error are correctly identified in 78% of tests.
These results are impressive. They demonstrate that the key issue with the non-recursive solution i.e. that the the individual tests must be ordered with some prior knowledge of what errors the DAG contains has been completely solved by the recursive algorithm.
Also these results suggest that the algorithm will be useful in real-world causal inference problems. It is a reasonable assumption that the domain experts can produce a DAG that is close to the actual causality found in the real-world but that they may make a small number of mistakes.
Given that assumption these results show that the algorithm can correct an invalid DAG and that is a pre-requisite for obtaining indicative, useful and usable results from the subsequent causal modelling stage.
2 of Each Type Separately
Testing for 2 of each type of error performs as follows …

The accuracy starts to deteriorate when the test DAG contains 2 of each type of error. 2 missing edges retains a high level of accuracy at 83.3% but 2 spurious edges are only accurately detected 33.3% of the time with 25% accuracy for 2 reversed edges.
1 of Each Type in the same DAG
The next stage is to make the testing more varied and try a set of error DAGs that each contain one of each type of error i.e. 1 x missing, 1 x spurious, 1 x reversed = 3 errors in each test DAG …

This test shows that DAGs that contain 1 of each type of error are accurately detected in 80% of cases and that is a remarkable test result!
A DAG can contain 3 errors, one of each type, and this combination of errors will be correctly identified 80% of the time!
2 of each Type in the same DAG
Lastly here are the test results for DAGs that contain 2 of each type of error …

When the example DAG that contains 6 errors in total, 2 x missing, 2 x spurious and 2 x reversed will not have those errors reliably detected. However that is unsurprising as the example DAG has just 8 edges and in this test 6 out of 8 or 75% of the edges are wrong.
Conclusion
The "Unified Theory of Everything" Causal Validation algorithm is not infallible and the more complex, varied, and numerous the errors you get the more the accuracy drops off.
However the accuracy is high enough to be usable and useful. In a real-world project the errors identified by the algorithm would be reviewed by the domain experts, then incremental changes would be made to the DAG and then the validation would be re-executed in an iterative manner until a final DAG is agreed.
It is also true that the more effort is put into reviewing, critiquing and correcting the DAG using domain expertise before the validation algorithm is run against it, the better the results will be.
Even the unified algorithm with all the improvements delivered by recursion and hinting has its limitations but it is accurate enough to provide an invaluable tool to aid in the critical step of ensuring that a proposed DAG accurately captures the causal relationships inherent in the data and the real world which in turn is critical to producing useful, usable and reliable results from causal inference machine learning models.
Further Research
The main reason that the various types of error cannot be detected with 100% reliability is the performance of the dependency test.
The dependency test must be flexible enough to evaluate an expression like Y ⫫ X, Z1, Z3 | W, Z2 to implement the missing link test and must be able to evaluate the results for each variable separately.
As explained in the earlier article (link above) looking at the regression results for p-value alone is unreliable and hence my solution was to look at a combination of the co-efficient and p-value and the thresholds I chose were based on trial-and-error in many tests.
The end result is that my implementation of the dependency test is not 100% reliable but it is as close to 100% as I could get it. It is possible that there are options for improvements
- Is there is another method of evaluating the p-value and co-efficient to improve performance?
- Is there a totally different approach to the regression solution with better performance?
- Can the proposed unified theory of everything for causal validation be completely replaced with something fundamentally different with better performance?
After many months of trawling through the books and online articles and piecing together a solution from many different sources, I have arrived at the very best performance I can achieve.
If there is anyone out there with any ideas for improvement based on one of my 3 options above, or using an option I have not thought of, please get in touch – I would love to hear from you.
In the meantime this article, the last of 5, represents the culmination of what I can achieve in terms of a unified theory of everything for causal validation and I will now move on to explaining how I have used this and other techniques to solve real-world causal inference problems to drive impact and outcomes in organisational settings.
Connect and Get in Touch …
If you enjoyed this article please follow me to keep up to date with future articles.
If you have any thoughts or views, especially on causal inference and where this exciting new branch of Data Science is going I would love to hear from you – please leave a message and I will get in touch.
My previous articles can be reviewed by Taking a quick look at my previous articles and this website brings together everything I am doing with my research and causal inference – The Data Blog.