Climate Change in the Countryside
Quick Success Data Science
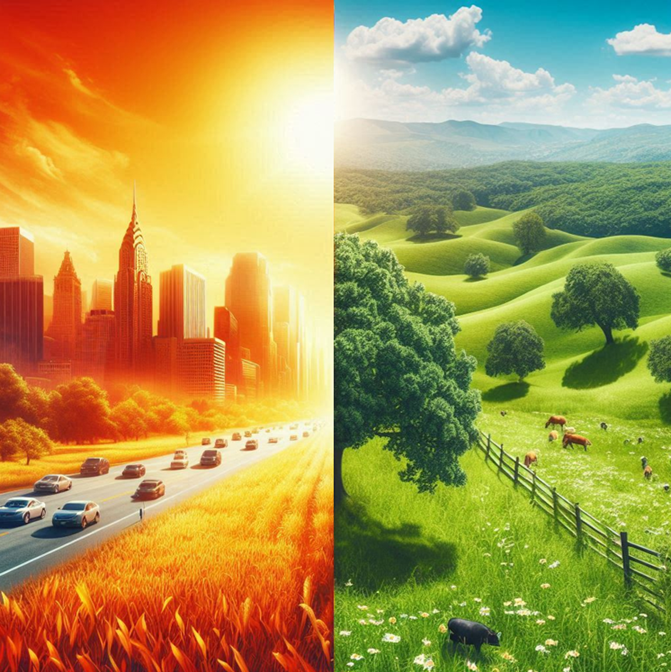
I have a relative who believes rising temperatures are just a "heat island" effect. That is, rapidly growing cities of steel and concrete heat up faster and retain heat longer than green rural areas. He says, "Much of global warming goes away if you focus on temperature measurements taken in the cooler countryside."
The nice thing about data science is that you can be your own fact-checker. In this Quick Success Data Science project, we'll test the previous assumption using temperature data from the great state of Texas.
What's a Heat Island?
According to the EPA, heat islands are urbanized areas that experience higher temperatures than outlying areas. Artificial structures, such as buildings and roads, absorb and re-emit the sun's heat more than natural landscapes, such as forests and lakes. Human activity, including driving cars and cooling buildings, generates additional heat. In big cities, where these structures and activities are highly concentrated, "islands" of higher temperatures form relative to the surrounding countryside.

Because of this urban heat island effect, cities are typically hotter during the day and warmer at night. Daytime temperatures are about 1–7°F higher than temperatures in rural areas, and nighttime temperatures are about 2–5°F higher.
If you only show the temperature data from large growing cities, the impression will be that global temperatures are higher and rising faster than the true global averages. To test this, we simply need to compare historical temperature profiles for large urban areas with much smaller rural towns.
The Strategy
We'll look at two large urban areas and four rural towns, three in northern Texas and three in southern Texas. In the north, we'll use Dallas, flanked by Albany to the west and Sulphur Springs to the east.

To the south, we'll use San Antonio, flanked by Hondo to the west and Luling to the east.

The population of these cities in 1950 and 2023, based on publicly available data from the U.S. Census, is summarized in the following table. Dallas and San Antonio represent the large urban areas.

A goal here is to use relatively closely grouped cities along roughly the same line of latitude. The prevailing winds in Texas are from the west and cold fronts tend to move down the plains from the north. Keeping the cities fairly close together and at the same latitude means they should experience similar weather.
With the data in hand, we can plot and compare the temperature profiles of the big cities with the small ones to see if they follow similar trends.
The Data
In his book, Unsettled: What Climate Science Tells Us, What it Doesn't, and Why it Matters, Steven Koonin uses the US government's 2017 Climate Science Special Report to show that the number of daily record cold measurements has been decreasing over time at a higher rate than daily record warms have been increasing. Thus, a significant Climate Change signal lies hidden in low temperatures, so we should look at both high and low measurements.
We can find the average yearly low and high temperatures for our selected cities in public records managed by the National Oceanic and Atmospheric Administration (NOAA). We'll look at the period between 1950 and 2023. This will give us almost 75 data points and include a glimpse of the years before the rapid rise in temperatures starting around 1980.
For convenience, I've already collected this data and stored it in a Gist. We'll access it programmatically using URL addresses.
The Code
The following code was written in Jupyter Lab.
Importing Libraries
We'll need only three third-party libraries for the main part of this project: Matplotlib, pandas, and GeoPandas.
Matplotlib is Python's most popular plotting library. You can find installation and use instructions here.
Pandas is Python's main data analysis library. You can find its installation and quick start guides here.
GeoPandas extends pandas to handle geospatial data. You can find its installation and user guides here.
import matplotlib.pyplot as plt
import pandas as pd
import geopandas as gpd
Loading the Geospatial Data for the Cities
Studies like these always include an "index map" that shows the area of interest. So, we'll start by plotting an outline of Texas with the cities in their proper geospatial location and scaled by their population.
The following code loads the city latitude, longitude, and population data from a URL using pandas' read_csv()
method. It then displays the full DataFrame.
# Load the city data:
df_map = pd.read_csv('https://bit.ly/3XgMpIu')
df_map.head(6)

Creating the GeoDataFrame
Next, we convert the pandas DataFrame into a GeoPandas GeoDataFrame with a new column for "geometry." This column holds the longitude and latitude data in "point" geometry format. Later, we'll use these points to plot the cities on the map.
gdf = gpd.GeoDataFrame(df_map, geometry=gpd.points_from_xy(df_map.Longitude,
df_map.Latitude))
gdf.head(6)

Plotting the Index Map
The following code uses Matplolib to plot the index map. Before we can do this, however, we'll need the outline of Texas, or more specifically, a shapefile of Texas.
A shapefile is a common geospatial vector data format for geographic information system (GIS) software. While we used a point geometry for the city locations, we'll use a polygon for the state boundary.
A handy place to find shapefiles is the Natural Earth public domain dataset. Just navigate to this site and click the download link highlighted in yellow below (the version number may change over time):

Move the zipped file into the folder containing your Python script or notebook, then run the following code (you don't need to unzip the folder).
# Set-up the index map figure:
fig, ax = plt.subplots(figsize=(7, 6))
# Load the states shapefile as a GeoDataFrame:
states = 'ne_110m_admin_1_states_provinces.zip'
usa = gpd.read_file(states)
# Make a new GeoDataFrame with just Texas:
tx = usa[(usa.name == 'Texas')]
# Plot the outline of Texas:
tx.boundary.plot(ax=ax,
linewidth=1,
edgecolor='black')
# Plot the cities scaled by population:
gdf.plot(ax=ax,
markersize=gdf['Population'] / 2000,
color='firebrick')
# Annotate city names centered over the marker:
for x, y, label in zip(gdf.geometry.x, gdf.geometry.y, gdf['City']):
if label == 'Hondo':
horiz_align = 'right'
vert_align = 'bottom'
else:
horiz_align = 'left'
vert_align = 'center'
ax.annotate(label,
xy=(x, y),
xytext=(5, 2),
textcoords="offset points",
fontsize=8,
ha=horiz_align,
va=vert_align)
plt.title('Texas Cities Used in Study (Scaled by Population)')
plt.xlabel('Longitude')
plt.ylabel('Latitude')
plt.show()

Again, the marker size represents a city's population, not its city limits.
For more on shapefiles, visit this article:
Loading the Temperature Data
Now we'll load the temperature data for each city – stored online as a CSV file – as a pandas DataFrame.
# Read in city temperature data:
df_dallas = pd.read_csv('https://bit.ly/3WVtW2R')
df_albany = pd.read_csv('https://bit.ly/476rQSw')
df_sulphur = pd.read_csv('https://bit.ly/3Mhg6Tp')
df_san_antonio = pd.read_csv('https://bit.ly/3AKLQOa')
df_luling = pd.read_csv('https://bit.ly/4dSZ5e6')
df_hondo = pd.read_csv('https://bit.ly/4cCaMVC')
As a check, display the first five records in the Dallas dataset:
df_dallas.head()

Defining a Function to Plot the Yearly Average High Temperatures
We'll need to make multiple plots, so next we'll define a function that takes two DataFrames as arguments and plots the high-temperature measurements for each as line charts in the same figure. I'm only using two DataFrames because I find it hard to understand three or more curves in the same figure.
def plot_highs(df1, df2):
plt.plot(df1.Year, df1.High, color='firebrick', label=df1.City[0])
plt.plot(df2.Year, df2.High, color='grey', label=df2.City[0])
plt.title('Average Yearly High Temperatures (F)')
plt.xlabel('Year')
plt.ylabel('Average Yearly High Temperature (F)')
plt.legend()
plt.grid()
plt.legend();
Defining a Function to Plot the Yearly Average Low Temperatures
Next, we repeat the previous code for the low-temperature measurements.
def plot_lows(df1, df2):
plt.plot(df1.Year, df1.Low, color='firebrick', label=df1.City[0])
plt.plot(df2.Year, df2.Low, color='gray', label=df2.City[0])
plt.title('Average Yearly Low Temperatures (F)')
plt.xlabel('Year')
plt.ylabel('Average Yearly Low Temperature (F)')
plt.grid()
plt.legend();
Comparing the Highs in Dallas and Sulphur Springs
First, we'll look at Dallas and the rural town of Sulphur Springs about 80 miles (128 km) to the northeast.
plot_highs(df_dallas, df_sulphur)

Several things stand out in this plot. Dallas tends to be a few degrees hotter, in line with the heat island effect. Temperatures were very high during the historic droughts of the 1950s and then cooled somewhat in the 1960s and 70s (attributable to high concentrations of industrial and volcanic-related sulfate aerosols reflecting sunlight into space). After around 1980 there's an inexorable climb to the present.
The temperature data for both cities show the same general trends. If the warming were due to the heat island effect, we would expect to see the Sulphur Springs curve flatten after 1980 rather than follow the Dallas curve.
Comparing the Highs in Dallas and Albany
Now let's look at Dallas and the rural town of Albany about 150 miles (240 km) to the west.
plot_highs(df_dallas, df_albany)

The curves are similar and follow the same trends.
Comparing the Highs in San Antonio and Hondo
Now let's move about 270 miles (432 km) south and look at San Antonio and the rural town of Hondo about 44 miles (70 km) west.
plot_highs(df_san_antonio, df_hondo)

Oddly, the Hondo temperatures tend to be higher than those in the much larger city of San Antonio. They show the same trends, however, suggesting there's no heat island effect biasing the results.
Comparing the Highs in San Antonio and Luling
Here's the result for San Antonio versus the rural town of Luling about 57 miles (91 km) to the northeast.
plot_highs(df_san_antonio, df_luling)

These curves are very similar and, except for a few aberrations, track each other nicely.
Comparing the Lows in Dallas and Sulphur Springs
Now let's look at the low temperature data. Remember, one aspect of climate change seems to be that the number of record-low temperatures is decreasing over time.
Here's the comparison of Dallas and Sulphur Springs:
plot_lows(df_dallas, df_sulphur)

The temperature separation here is significant but still within the observed behavior for the heat island effect. The key takeaway is that both curves increase at the same approximate rate following the early 1980s.
Comparing the Lows in Dallas and Albany
Here's the low-temperature comparison for Dallas and Albany:
plot_lows(df_dallas, df_albany)

This plot is more like what I would expect to see if climate change-related temperature changes were heavily influenced by the heat island effect. After around 1980, the yearly average low temperatures in Dallas increased overall while those in rural Albany tended to flatline, though in the last ten years or so they have mimicked Dallas more closely.
Comparing the Lows in San Antonio and Hondo
Here's the low-temperature comparison for San Antonio and Hondo:
plot_lows(df_san_antonio, df_hondo)

Both these curves tend to track each other fairly well, though the Hondo curve appears to be fairly flat from 1980 to 2010.
Comparing the Lows in San Antonio and Luling
Here's the low-temperature comparison for San Antonio and Luling:
plot_lows(df_san_antonio, df_luling)

Even more so than the San Antonio-Hondo curves, the rural measurements in Luling diverge from the San Antonio readings between about 1999 to 2013. After this, they quickly recover and closely follow the larger city's behavior.
Comparing San Antonio to Dallas
For fun, let's compare San Antonio to Dallas. Being much farther south, we should expect San Antonio to be warmer, and it is. The curves also rise with similar slopes.
plot_highs(df_san_antonio, df_dallas)

plot_lows(df_san_antonio, df_dallas)

These last two curves don't help address the urban versus rural issue, but they demonstrate that temperature changes in the previous 73 years have been similar across Texas.
Quantifying Increasing Temperatures (1980–2023)
A more quantitative way of looking at this is to fit separate regression lines to the northern and southern rural datasets after filtering them to the interval 1980–2023. This represents the period when the recent warming trend was established.
We'll need the NumPy (numerical Python) and scikit-learn (machine learning) libraries. You can find installation instructions in the previous links.
Here's an example code snippet for combining and plotting the Hondo and Luling DataFrames in the south:
import numpy as np
from sklearn.metrics import r2_score
# Filter southern rural Dfs to 1980 and after:
df_hondo_80 = df_hondo[df_hondo['Year'] >= 1980]
df_luling_80 = df_luling[df_luling['Year'] >= 1980]
# Merge the DFs into one:
rural_south_df = pd.concat([df_hondo_80, df_luling_80],
ignore_index=True)
# Plot a Scattergram:
plt.scatter(rural_south_df['Year'],
rural_south_df['Low'],
color='grey',
label='Rural South Low Temps')
# Fit a regression line with NumPy:
slope, intercept = np.polyfit(rural_south_df['Year'],
rural_south_df['Low'], 1)
regression_line = slope * rural_south_df['Year'] + intercept
plt.plot(rural_south_df['Year'],
regression_line,
color='firebrick',
label='Regression line')
# Calculate R-squared value with sklearn:
r_squared = r2_score(rural_south_df['Low'], regression_line)
# Add equation and R-squared value to the plot:
equation_text = f'y = {slope:.2f}x + {intercept:.2f}n$R^2$ = {r_squared:.2f}'
plt.text(0.05, 0.95,
equation_text,
transform=plt.gca().transAxes,
fontsize=12,
verticalalignment='top',
bbox=dict(boxstyle='round,pad=0.5',
edgecolor='black',
facecolor='white'))
# Add labels and title:
plt.xlabel('Year')
plt.ylabel('Low')
plt.title('Rural South Average Yearly Low Temperatures (1980-2023)')
plt.legend(loc='lower right')
# Show plot
plt.show()
Here are the results for both the high and low temperatures. Both regression lines have positive slopes, indicating increasing temperatures over this period:

The two rural cities in the north show similar, though more muted, results:

These regression lines confirm the increase we observed in the line plots.
The Recap
Based on a cursory examination of only six cities, it is apparent that both urban and rural areas show similar responses to climate change. Global warming is not an urban data "head fake" caused by only showing data from heat islands.
Bonus Project: Comparing Temperature and Population Trends
As a bonus project, compare a city's temperature trend with its population growth. Here's an example for Dallas, using the population of the Dallas-Fort Worth metroplex:


According to the Environmental Protection Agency, a city with a population of only one million can behave as an urban heat island. That means the DFW metroplex was a heat island as far back as the early-1950s.
More Climate Change Projects
If you enjoy using Python to explore climate change topics, be sure to check out the following projects:
Tell a Climate Story with Plotly Express
Map an Urban Heat Island With PyGMT
Build Beautiful Ridgeline Plots with joypy
Visualize Data Ranges with Matplotlib
Thanks!
Thanks for reading and please follow me for more Quick Success Data Science projects in the future.