Difference-in-Difference 101
What is Difference-in-difference (DiD or DD or diff-in-diff)? Why do we care about DiD? Today I will answer all the questions about one of the most popular methods in econometrics to study a policy effect.

DiD is a widely used econometric technique that estimates causal relationships by comparing the changes in outcomes over time between a treatment group and a control group. The question is what are treatment and control? Treatment is a policy intervention that affects a specific group due to a policy or change. Control is a group that receives no intervention. Causal relationships mean cause and effect relations.
We care about this method because it is useful in evaluating the effects of policy changes or interventions when randomized experiments are not feasible. This means that sometimes, experiments are targeted to a particular group, implying the people who received the treatment are not random. DiD will help to isolate the impact of intervention even without randomization.
This article will delve into the concepts, assumptions, implementation, and examples.
What is DiD
Our research question is: what is the effect of treatment D on outcome y? DiD allows us to estimate what would have happened to the treatment group if the intervention had not occurred. This counterfactual scenario is essential for understanding the true effect of the treatment. Every job or work revolves around answering similar questions like the effect of interventions, policy changes, or treatments across various fields. In economics, it assesses the impact of tax cuts on economic growth, while in public policy, it evaluates the effects of new traffic laws on accident rates. In marketing, DiD analyzes the influence of advertising campaigns on sales.
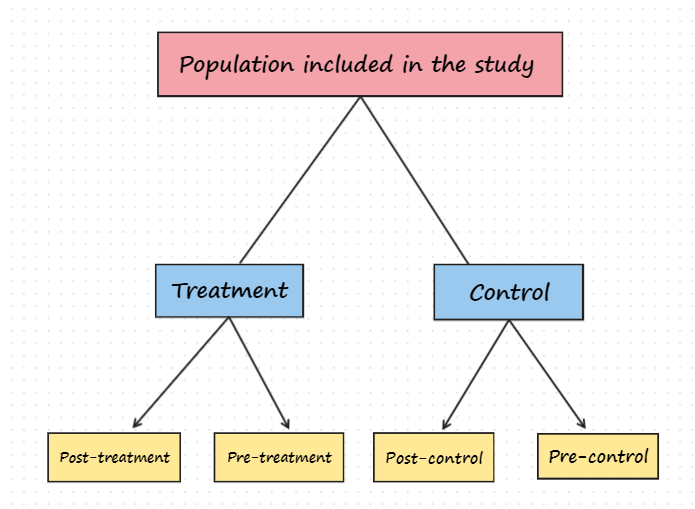
For example, in the diagram above, we have population data in our sample. We will divide the data into treatment and control where the treatment received the intervention. We can observe post and pre-variables for both groups.
How to do DiD
Simple Treatment/Control Difference Estimator

This equation will calculate the treatment effect by comparing the changes in the outcome over time between the treatment and control groups.
I have created a fake example to help understand the math.

The DiD coefficient would be 9 using the formula mentioned above.
DiD Estimator: Calculation using a regression
DiD helps to control for time-invariant characteristics that might bias the estimation of treatment effects. This means that it removes the influence of variables that are constant over time (eg., geographical location, gender, ethnicity, innate ability, etc.). It can do so because these characteristics affect both pre-treatment and post-treatment periods equally for each group.
The core equation for a basic DiD model is:

where:
- y is the outcome variable for individual