How to Program a Neural Network

Not a Medium member? Access this article for free here.
In this article, we will build a neural network from scratch and use it to classify handwritten digits.
Why reinvent the wheel/neural network, I hear you say? Can't I just use my favourite machine learning framework and be done with it? Yes, there are many off-the-shelf frameworks that you can use to build a neural network (Keras, PyTorch, and TensorFlow to name a few). The thing with using one of these is that they make it easy for us to treat neural networks like black boxes.
This isn't always a bad thing. Often we need this level of abstraction so that we can get to work on the problem at hand, but we should still strive to at least have a basic understanding of what is going on under the hood if we are to use neural networks in our work.
Building a neural network from scratch is, in my opinion, the best way to foster a deep understanding of how they work.
By the end of this article, you will have learned about the feedforward and backpropagation algorithms, what an activation function is, what the difference between an epoch and a batch is, and how to train a neural network. We will finish with an example by training a neural network to recognise handwritten digits.
All code used in this article is available here on GitHub [1].
What is a neural network?
Neural networks, or artificial neural networks, are a type of machine learning algorithm. They form the core of many Deep Learning and artificial intelligence systems like computer vision, forecasting and speech recognition.
The structure of artificial neural networks is sometimes compared to the structure of biological neural networks in the brain. I would always urge caution not to draw too much from this comparison. Sure, artificial neural networks look a bit like biological neural networks but it is quite a big leap to start comparing them to something as complex as a human brain.
A neural network is made up of several layers of neurons. Each layer of neurons is activated based on the activations in the previous layer, a set of weights connecting the previous layer to the current layer and a set of biases applied to the neurons in the current layer.

The first layer is the input layer. Input layer activations come from the input to the neural network. The final layer is the output layer. The activations in the output layer are the output of the neural network. The layers in between are called hidden layers.
A neural network is a generalized approximation of a function. Like any other function, when we give it an input it returns an output.
The novel thing about Neural Networks is how they get from the input to the output. The process is driven by how network weights and biases influence neuron activations and how these propagate through the network to eventually arrive at the output layer. The feedforward algorithm is used by neural networks to transform from our input into an output.
For a neural network to provide useful output we must first train it. When we train a neural network all that we are doing is iteratively adjusting the weights and biases to improve the accuracy of the output. We work out which direction and by how much we need to nudge the weights and biases using backpropagation and gradient descent.
Feedforward algorithm
The feedforward algorithm transforms our neural network input into meaningful output. As its name suggests, the algorithm "feeds forward" information from one layer to the next.
To understand how it achieves this, let's first zoom in and look at how information is passed from one layer to a single neuron in the next layer.

The activation of the first neuron in the second layer, _a_₀⁽¹⁾, is calculated by taking a weighted sum of the activations from the previous layer plus a bias and passing this through an activation function, σ(x):
The superscript with round brackets denotes the layer index, starting from 0 for the input layer. Activation (a), and bias (b) subscripts _ denote neuron index. The first and second numbers in the weight (_w) subscripts denote the index of the neuron the weight connects to (in the current layer) and from (in the previous layer) respectively.
The activation function determines if a neuron should be activated based on the input it receives. Common activation functions include sigmoid, tanh, rectified linear unit (ReLU), and softmax. To keep things simple, in our implementation, we will always use the sigmoid activation function.
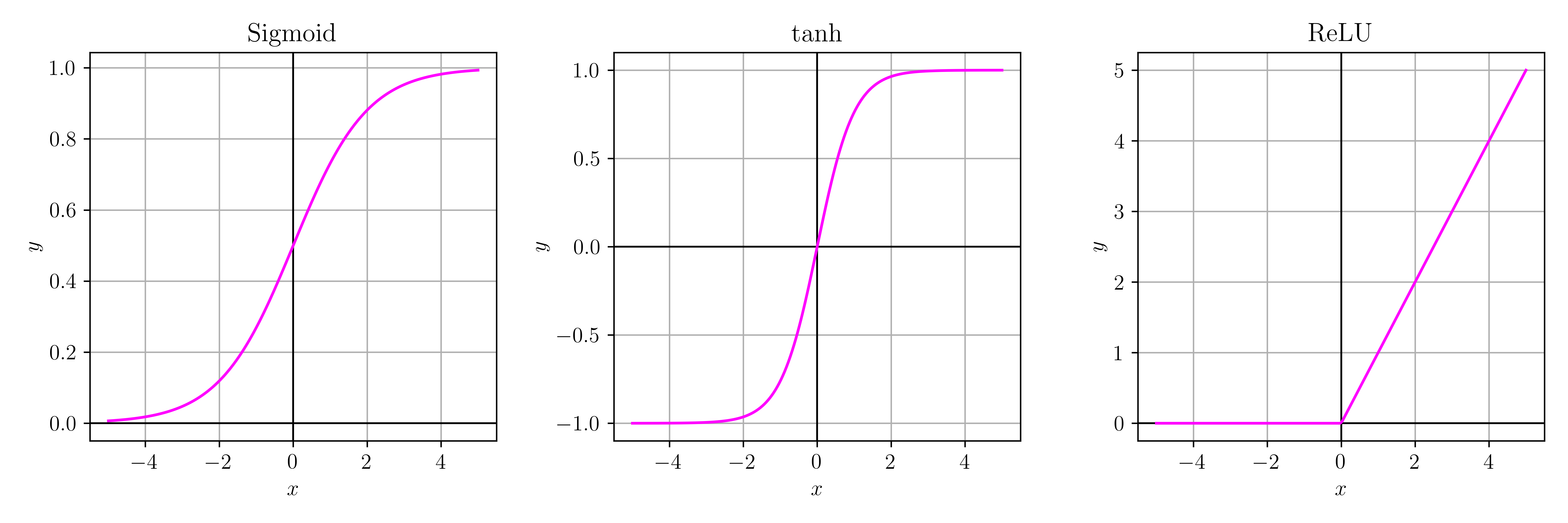
The equation we used to calculate _a_₀⁽¹⁾ can be vectorised so that we can calculate all activations in the second layer:
Now we have neuron activations for the second layer a⁽¹⁾, we can use the same calculation to find a⁽²⁾ and again for a⁽³⁾ and so on…
Let's look at how this can be implemented in Python:
import numpy as np
import math
class Network:
def __init__(self, layers):
self.layers = layers
self.activations = self.__init_activations_zero()
self.weights = self.__init_weights_random()
self.biases = self.__init_biases_zero()
def __init_activations_zero(self):
activations = []
for layer in self.layers:
activations.append(np.zeros(layer))
return activations
def __init_weights_random(self):
weights = []
for i in range(0, len(self.layers) - 1):
weights.append(np.random.uniform(-1, 1, (self.layers[i+1], self.layers[i])))
return weights
def __init_biases_zero(self):
biases = []
for i in range(1, len(self.layers)):
biases.append(np.zeros(self.layers[i]))
return biases
def sigmoid(self, x):
return 1 / (1 + np.exp(-x))
def feedforward(self, input_layer):
self.activations[0] = input_layer
for i in range(0, len(self.layers) - 1):
self.activations[i+1] = self.sigmoid(np.dot(self.weights[i], self.activations[i]) + self.biases[i])
The Network
class contains all the information about our neural network. We initialise it by passing a list of integers relating to the number of neurons in each layer. For example, network = Network([10, 3, 3, 2])
will create a network with ten neurons in the input layer, two hidden layers each containing three neurons and an output layer with two neurons.
The __init_*
methods initialise values for activations, weights and biases. Activations and biases are initially all zero. Weights are given a random value between -1 and 1.
The feedforward
method loops through the layers calculating activations in each subsequent layer.
Below is an example using feedforward
to calculate the output of our [10, 3, 3, 2]
network given a random input. The output is inspected by printing the activations in the final layer:
network = Network([10, 3, 3, 2])
input_layer = np.random.uniform(-1, 1, (10))
network.feedforward(input_layer)
print(network.activations[-1])
...
[0.29059666 0.5261155 ]
That's it! We have successfully implemented the feedforward algorithm! Lets turn our attention to backpropagation.
Backpropagation algorithm
The backpropagation algorithm is the process through which a neural network learns from its mistakes.
In the above implementation of the feedforward algorithm, we initialise network weights with a random number between -1 and 1 and set all biases to equal 0. With this initial setup, the output produced by the network for any given input will be essentially random.
What we need is a way to update the weights and biases so that the output of the network becomes more meaningful. To do this we use gradient descent:
Where aₙ **** is a vector of input parameters. The subscript _ n denotes iteration. f(aₙ) is a multi-variable cost function and ∇f(a) is the gradient of that cost function.