Matrix Approximation in Data Streams

Matrix approximation is a heavily studied sub-field in data mining and Machine Learning. A large set of data analysis tasks rely on obtaining a low rank approximation **** of matrices. Examples are dimensionality reduction, anomaly detection, data de-noising, clustering, and recommendation systems. In this article, we look into the problem of matrix approximation, and how to compute it when the whole data is not available at hand!
The content of this article is partly taken from my lecture at Stanford -CS246 course. I hope you find it useful. Please find the full content here.
Data as a matrix
Most data generated on web can be represented as a matrix, where each row of the matrix is a data point. For example, in routers every packet sent across the network is a data point that can be represented as a row in a matrix of all data points. In retail, every purchase made is a row in the matrix of all transactions.

At the same time, almost all data generated over web is of a streaming nature; meaning the data is generated by an external source at a rapid rate which we have no control over. Think of all searches users make on Google search engine in a every second. We call this data the streaming data; because just like a stream it pours in.
Some examples of typical streaming web-scale data are as following:

Think of streaming data as a matrix A containing n rows in d-dimensional space, where typically n >> d. Often n is in order of billions and increasing.
Data streaming model
In streaming model, data arrives at high speed, one row at a time, and algorithms must process the items fast, or they are lost forever.
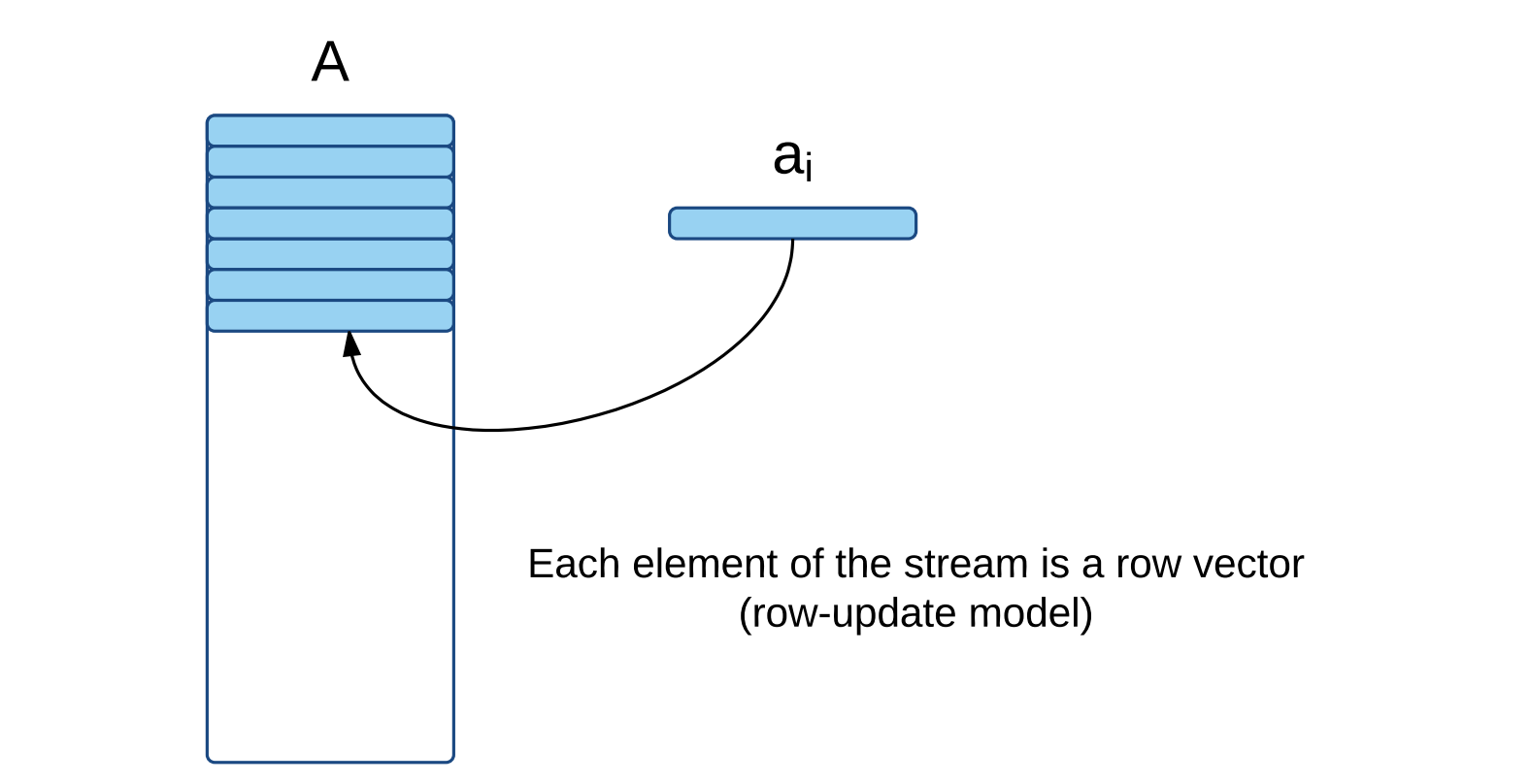
In data streaming model, algorithms can only make one pass over the data and they work with a small memory to do their processing.
Rank-k approximation
Rank-k approximation of a matrix A is a smaller matrix B of rank k such that B approximates A accurately. Figure 2 demonstrates this notion.

B is often called a sketch of A. Note, in data streaming model, B will be much smaller than A such that it fits in memory. In addition, rank(B) << rank(A). For example, if A is a term-document matrix with 10 billion documents and 1 million words then B would probably be a 1000 by million matrix; i.e. 10 million times less rows!
The rank-k approximation has to approximate A "accurately". While accurately is a vague notion, we can quantify it via various error definitions:
1️⃣ Covariance error:
The covariance error is the Frobenius norm or L2 norm of differences between covariance of A and covariance of B. This error is mathematically defined as following:

2️⃣ Projection error:
The projection error is the norm of the residual when data points in A are projected onto the subspace of B. This residual norm is measured as either L2 norm or Frobenius norm:

These errors assess the quality of the approximation; the smaller they are the better the approximation is. But how small can they be?
When we compute these errors, we must have a baseline to compare them against. The baseline, everyone uses in field of matrix sketching is the rank-k approximation created by Singular Value Decomposition (SVD)! SVD computes the best rank-k approximation! Meaning it causes the smallest error on both "covariance error" and "projection error".
The best rank-k approximation to A is denoted as Aₖ. Therefore, the least error caused by SVD is :

SVD, decomposes a matrix A into three matrices:
- The left singular matrix U
- The singular values matrix S
- The right singular matrix V
U and V are orthonormal, meaning that their columns are of unit norm and they are orthogonal; i.e. the dot product of every two columns in U (similarly in V) is zero. The matrix S is diagonal; only entries on the diagonal are non-zeros and they are sorted in descending order.

SVD computes the best rank-k approximation by taking the first k columns of U and V and the first k entries of S:

As we mentioned, the Aₖ computed in this way has the lowest approximation error among any matrices B with rank k or lower. However, SVD is a very time-consuming method, it requires runtime O(nd²) if A is n-by-d, and it is not applicable to matrices in data streaming fashion. In addition, SVD is not efficient for sparse matrices; it does not utilize sparsity of the matrix in computing approximation.
❓Now the question is how do we compute matrix approximation in streaming fashion ?
There are three main family of methods in streaming matrix approximations:
1️⃣ Row sampling based
2️⃣ Random projection based
3️⃣ Iterative sketching
Row sampling based methods
These methods sample a subset of "important" rows with respect to a well-defined probability distribution. These methods differ is in how they define the notion of "importance". The generic framework is that they construct the sketch B as following:
- They first assign a probability to each row in the streaming matrix A
- Then they sample l rows from A (often with replacement) to construct B
- Lastly, they rescale B appropriately to make it an unbiased estimate of A

Note, the probability assigned to rows in step 1, is in fact the "importance" of the row. Think of "importance" of an item as the weight associated to the item e.g. for file records, the weight can be size of the files. Or for IP addresses, the weight can be the number of times the IP address makes a request.
In matrices, each item is a row vector, and its weight is the squared of its norm; also called L2 norm. There is a row sampling algorithm that samples with respect to L2 norm of rows in a one pass over data. This algorithm is called "L2-norm row sampling", and its pseudocode is as following:

This algorithm samples l = O(k/ε²) rows with replacement and achieves the following error bound:

Note, this is a weak error bound, as it is bounded by total Frobenius norm of A, which can be a large number! There is an extension of this algorithm that performs better; let's take a look at it.
Extension: There is a variation of this algorithm that samples both rows and columns! It is called "CUR" algorithm and it performs better than "L2-norm row sampling" method. The CUR method creates three matrices C, U and R by sampling rows and columns of A. Here is how it works:
Step 1: CUR first samples few columns of A, each with the probability proportional to the norm of the columns. This makes the matrix C.

Step 2: Then CUR samples few rows of A, each with probability proportional to the norm of the rows. This forms matrix R.
Step 3: The CUR then computes the pseudo-inverse of the intersection of C and R. This is called matrix U.
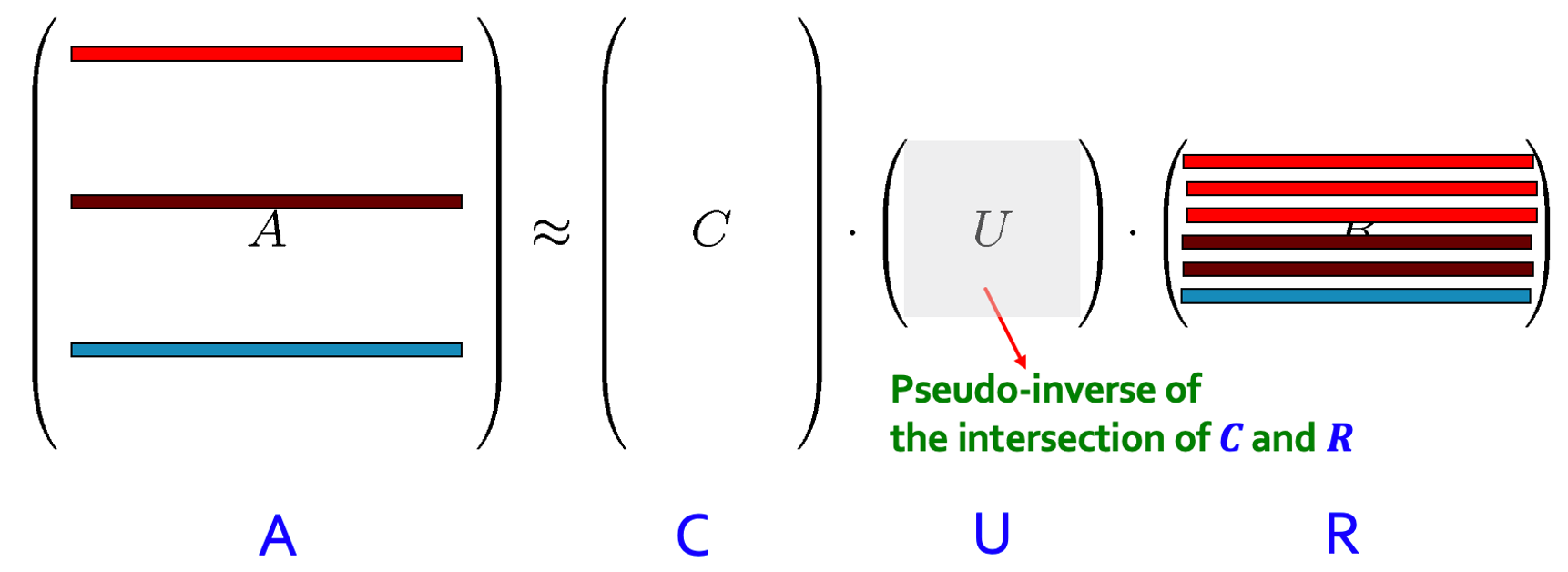
Finally, the product of these three matrices, i.e. C.U.R approximates A, and provides a low-rank approximation. This algorithm achieves the following error bound sampling l = O(k log k/ε²) rows and columns.

Note, how this is a much tighter bound as compared to L2-norm row sampling.
Summary: The row sampling family of methods (CUR included) samples rows (and columns) to form a low-rank approximation; therefore they are very intuitive and form interpretable approximations.
In the next section, we see another family of methods that are data oblivious.
Random projection based methods
The key idea of these group of methods is that if points in a vector space are projected onto a randomly selected subspace of suitably high dimension, then the distances between points are approximately preserved.
Johnson-Lindenstrauss Transform (JLT) specifies this nicely as following: d datapoints in any dimension (e.g. n-dimensional space for n≫d) can get embedded into roughly log d dimensional space, such that their pair-wise distances are preserved to some extent.
A more precise and mathematical definition of JLT is as following:

There are many ways to construct a matrix S that preserve pair-wise distances. All such matrices are called to have the JLT property. The image below, shows few well-known ways of creating such matrix S:

One simple construction of S, as shown above, is to pick entries of S to be independent random variables drawn from N(0,1), and rescale S by √(1/r):

This matrix has the JLT property [6], and we use it to design a random projection method as following:

Note the second step, projects data points from a high n-dimensional space into a lower r-dimensional space. It's easy to show [6]that this method produces an unbiased sketch:

The random projection method achieves the following error guarantee if it sets r = O(k/ε + k log k). Note that they achieve better bound than row sampling methods.

There is a similar line of work to random projection that achieves better time bound. It is called Hashing techniques [5]. This method take a matrix S which has only one non-zero entries per column and that entry is either 1 or -1. They compute the approximation as B = SA.

Summary: Random projection methods are computationally efficient, and are data oblivious as their computation involves only a random matrix S. Compare it to row sampling methods that need to access data to form a sketch.
Iterative Sketching
These methods work over a stream A=

State of the art method in this group is called "Frequent Directions", and it is based on Misra-Gries algorithm for finding frequent items in a data stream. In what follows, we first see how Misra-Gries algorithm for finding frequent items work, then we extend it to matrices.
Misra-Gries algorithm for finding frequent items
Suppose there is a stream of items, and we want to find frequency f(i) of each item.

If we keep d counters, we can count frequency of every item. But it's not good enough as for certain domains such IP addresses, queries, etc. the number of unique items are way too many.

So let's Let's keep l counters where l≪d. If a new item arrives in the stream that is in the counters, we add 1 to its count:

If the new item is not in the counters and we have space, we create a counter for it and set it to 1.

But if we don't have space for the new item (here the new item is the brown box), we get the median counter i.e. counter at position l/2:

and subtract it from all counters. For all counters that get negative, we reset them to zero. So it becomes as following:

As we see, now we have space for the new item, so we continue processing the stream