Three Fundamental Flaws In Common Reinforcement Learning Algorithms (And How To Fix Them)
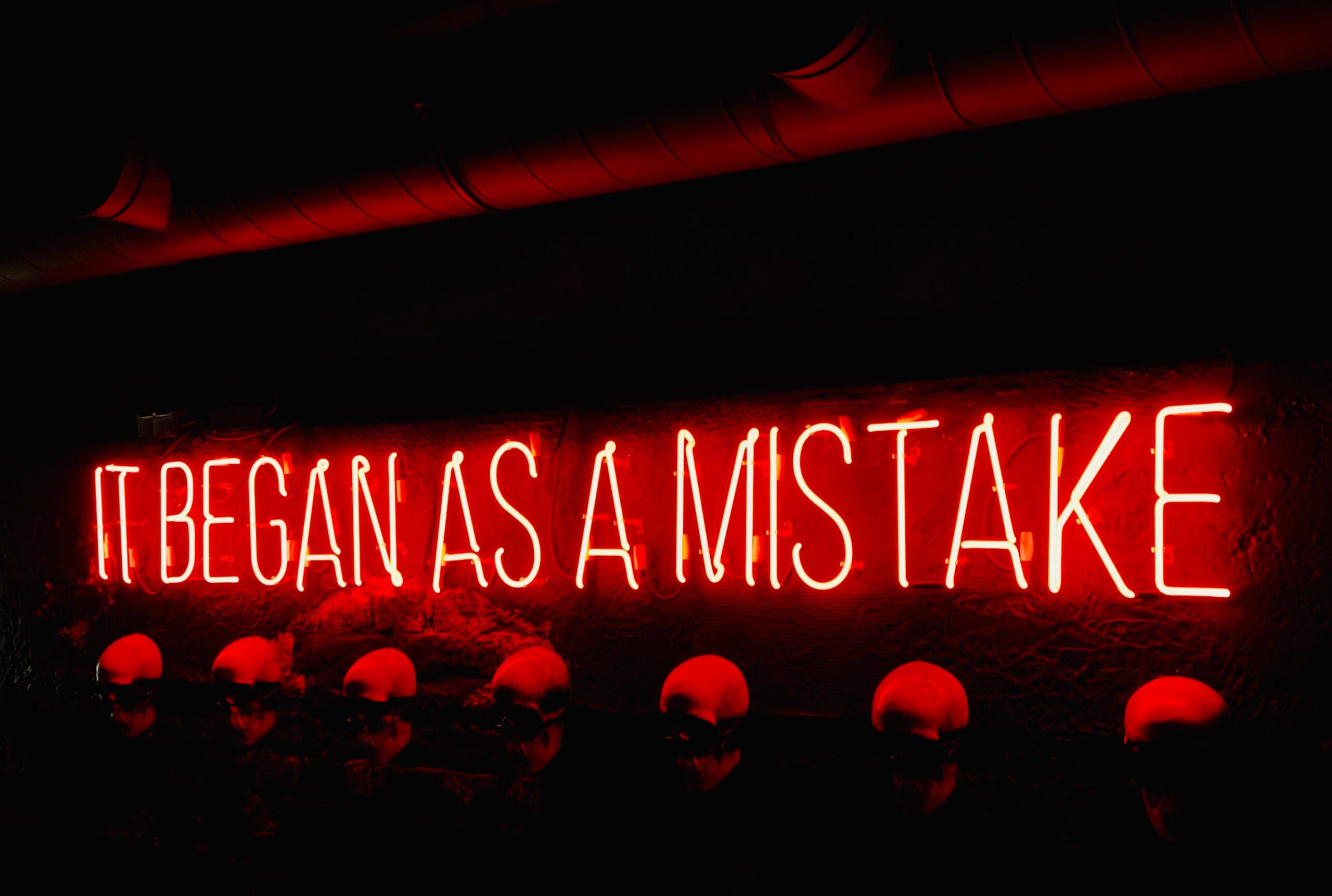
Reinforcement Learning algorithms such as Q-learning and REINFORCE have been around for decades and their textbook implementations are still widely used. Unfortunately, they exhibit some fundamental flaws, which greatly increase the struggle of learning a good policy.
This article addresses three major shortcomings of classical Reinforcement Learning algorithms, along with solutions to overcome them.
I. Selecting overvalued actions
The problem
Most RL algorithms use some notion of value functions to capture downstream rewards, many of them based on the well-known Q-learning algorithm. The mechanism driving Q-learning is that it selects the action that yields the highest expected value. Depending on initialization, this mechanism might get stuck at the first action that is tried, so we also select random actions with probability ϵ, typically set at 0.05 or so.
In the limit, we would explore each action infinitely often and the Q-values would converge to the true values. In practice, however, we work with limited samples and biased Q-values. As a result, Q-learning consistently selects actions with overestimated values!
Imagine a setting in which we play two identical slot machines. Machine A happens to give above-average rewards in the early iteration, so its Q-value is higher and we keep playing A. As machine B is only rarely selected, it would take a long time before we figure out the Q-values are actually identical.
More generally speaking, value functions will always be imperfect, and RL has a penchant to perform overvalued actions. In a sense, RL "rewards" poor estimates, which is obviously not a desirable property.

Solutions
The problems of Q-learning can be traced back to the practice of sampling and updating with the same observations. We can decouple these steps by sampling with one policy and updating another. This is precisely what Double Q-learning (Van Hasselt, 2010) does.

More generally speaking, it is good practice to work with target networks. The target network is a periodical copy of the policy, used to generate target values that we train upon (rather than using exactly the same policy to generate both observation and target). This approach reduces the correlation between target and observation.
Another solution angle is to take into account the uncertainty of our Q-value estimates. Rather than solely tabulating the expected values of actions, we can also keep track of the variance of observations, indicating how for we might be off from the true value. Working with uncertainty bounds and knowledge gradients are two ways to achieve this purpose. Instead of simply selecting the action with the highest expected Q-value, we also consider how much we can learn from a new observation. This approach favors exploring actions with high uncertainty, while still sampling with intelligence.
Seven Exploration Strategies In Reinforcement Learning You Should Know
II. Poor policy gradient updates
The problem
Policy gradient algorithms have been in swing for decades, and are at the root of all modern actor-critic models. The vanilla policy gradient algorithms – e.g., REINFORCE – rely on gradients to determine the direction of weight updates. A combination of high rewards and high gradients yield a strong update signal.

The idea seems natural. If the slope of the reward function is steep, you take a big step in that direction. If it is small, there is no sense in performing big updates. Compelling as it may seem, this logic is also fundamentally flawed.

A gradient only provides local information. It tells us how steep the slope is, but not how far to step in that direction; we might overshoot. Furthermore, policy gradients do not consider that a lack of gradient signal might get us stuck on a sub-optimal plateau.
To make matters worse, we cannot control this behavior by forcing the weight updates to be within a certain parameter region. In the figure below, for example, weight updates of the same magnitude have very different effects on the policy.

Solutions
A simple start is to experiment with various learning algorithms. The traditional stochastic gradient descent (SGD) algorithm only considers first moments. Modern learning algorithms (e.g., ADAM) consider second moments, often substantially enhancing performance. Although not fully resolving the problem, the performance increase may be remarkable.
Entropy regularization is a common way to prevent premature convergence of vanilla policy gradient algorithms. Loosely stated, entropy in RL is a metric for the unpredictability of action selection. Entropy regularization adds a bonus for exploring unknown actions, which is higher when we have learned less about the system:

More sophisticated extensions of policy gradient algorithms also consider second-order derivatives, which provide information on the local sensitivity of the function. At a plateau, we can safely take big steps without consequence. At a steep but curved slope, we would prefer cautious steps. Algorithms such as natural policy gradients, TRPO and PPO take into account the sensitivity towards updates, either explicitly or implicitly considering second-order derivatives. At the moment, PPO is the go-to policy gradient algorithm, striking a fine balance between ease of implementation, speed and performance.

Natural Policy Gradients In Reinforcement Learning Explained
III. Underperformance off-policy learning
The problem
Certain algorithms (e.g., these rooted in Q-learning) rely on off-policy learning, meaning that updates may be performed with a different action than actually observed. Whereas on-policy learning requires a tuple (s,a,r,s',a') – indeed, like its algorithm namesake SARSA— off-policy learning uses the best known action a* instead of a'. Consequently, we only store (s,a,r,s') for the weight updates and learn the policy independent of the agent's actions.
Due to its setup, off-policy learning can re-use prior observations by drawing them from an experience replay buffer, which is particularly convenient when creating observations is (computationally) expensive. We simply feed state s' to our policy to obtain an action a*, using the resulting value to update Q-values. The transition dynamics from s to s' do not need to be recomputed.
Unfortunately, even after extensively training an off-policy Reinforcement Learning algorithm on a large dataset, it often does not work nearly as well when deployed. Why is that?
The problem boils down to a common statistical caveat. The assumption is that the training set is representative for the true data set. When this is not the case – which it often isn't, as updated policies generate different state-action pairs – the policy is fit to a dataset that does not reflect the environment the agent ultimately operates in.
Solutions
True off-policy learning – e.g., learning good policies solely from a static dataset – might be fundamentally infeasible in Reinforcement Learning, as updating policies inevitably alters the probability of observing state-action pairs. As we cannot exhaustively explore the search space, we inevitably extrapolate values towards unseen state-action pairs.
The most common solution is to not train on a fully static dataset, but continuously enrich the dataset with observations generated under the new policy. It may help to also remove older samples, which no longer represent data generated under recent policies.
Another solution is Importance Sampling, which is essentially reweighs observations based on their likelihood of being generated under the present policy. For each observation, we can compute its ratio of the probability being generated under the original and present policy, making observations stemming from similar policies more likely to be drawn.

If you continue to struggle to get your off-policy algorithm to perform well out-of-sample, a switch to an on-policy algorithm should be considered. Especially when generating new observations is cheap, the loss in sample efficiency might be offset by the enhanced policy quality.
How To Model Experience Replay, Batch Learning and Target Networks
Summary
This article addressed three common flaws encountered in traditional RL algorithms, along with strategies to address them.
I. Overvalued actions
Problem:
- Algorithms based on value function approximation systematically select actions with overestimated values.
Solutions:
- Use target networks to decrease correlation between target and observation (e.g., as in Double Q-learning).
- Incorporate uncertainty of value estimates in action selection (e.g., uncertainty bounds, knowledge gradients.
II. Poor policy gradient updates
Problem:
- Policy gradient algorithms often perform poor update steps, e.g., minor steps when stuck in a local optimum or large ones that overshoot and miss the reward peak.
Solutions:
- Use learning algorithm that includes, e.g., ADAM – which tracks momentum in addition to first-order gradients – instead of the standard Stochastic Gradient Descent.
- Add an entropy bonus to the reward signal, encouraging more exploration of unknown regions.
- Deploy algorithms that include second-order derivatives (either explicitly or implicitly), such as natural policy gradients, TRPO or PPO.
III. Underperformance off-policy learning
Problem:
- The experiences in the replay buffer may not be representative for out-of-sample experiences, such that values are incorrectly extrapolated and performance drops.
Solutions:
- Update replay buffer, adding new experiences and removing older ones.
- Perform importance sampling to increase probability of selecting experiences stemming from policies closer to the target policy.
- Switch to on-policy learning (if sampling observations is cheap).
References
Problem I: Overvalued actions
Hasselt, H. (2010). Double Q-learning. Advances in neural information processing systems, 23.
Matiisen, Tambet (2015). Demystifying deep Reinforcement Learning. Computational Neuroscience Lab. Retrieved from neuro.cs.ut.ee/demystifying-deep-reinforcement-learning/
Problem II: Poor policy gradient updates
Mahmood, A. R., Van Hasselt, H. P., & Sutton, R. S. (2014). Weighted importance sampling for off-policy learning with linear function approximation. Advances in Neural Information Processing Systems, 27.
Cornell University Computational Optimization Open Textbook. (2021). ADAM. URL: https://optimization.cbe.cornell.edu/index.php?title=Adam
Problem III: Underperformance off-policy learning
Fujimoto, S., Meger, D., & Precup, D. (2019, May). Off-policy deep reinforcement learning without exploration. In International conference on machine learning (pp. 2052–2062). PMLR.