Why Is Python Consuming So Much Memory?

We all know that Python is famous for its flexibility and dynamic nature. However, have you think why it can achieve these and if there are any sacrifices? Of course, the specific mechanism of a Programming language is too complex to be explained in one article like this. Instead, I want to demonstrate today is to show a very simple example in Python regarding memory consumption.
In this article, I will show you how to measure how much memory a variable occupies and some overheads in Python that will cause a relatively higher memory consumption. If you have no idea why Python needs at least 28 bytes for a simple integer number, please don't miss the content of the rest of the sections.
1. How to Measure the Memory Usage for a Variable?

Before we start walking through the examples, we need a solution for measuring memory usage. Otherwise, my examples will not be convincing. Once we know the approach, we can also use it to measure our own implementation in our daily jobs.
The easiest way is definitely using Python built-in method. That is, in the sys
module, there is a function called getsizeof()
. It's very easy to use when we want to check the size of a variable.
import sys
x = [1, 2, 3, 4, 5]
print("Size of list:", sys.getsizeof(x), "bytes")
for item in x:
print("Size of list item:", sys.getsizeof(item), "bytes")

We can see that the function returns the size of objects. You may ask why a number takes 28 bytes and why summing up all the items does not equal the list container. Let me try to give you the answers.
2. Why is the Size of a List ≠ the Sum of the Size of All Its Items?

In fact, the getsizeof()
function only measures the object itself, but not the objects it refers to or contains. So, the 104 bytes only means the size of the "container" of the list. In other words, it doesn't include the items in it.
The container also includes pointers, which are all the "overheads" to maintain the list of objects.

Therefore, the 104 bytes do not even include the numeric elements in this list. The total size of the list should be 104 + 28*5 = 244 bytes. In other words, when we use the sys.getsizeof()
function to get the memory size of an object, it won't give us the full size if the object has some indirect reference to other objects.
There are more questions now. Let me answer one by one.
3. Why does an Integer Need 28 Bytes in Python?

The short answer is that, obviously, the integer objects also have overhead. But what are they? Is that because the number is in a list as an item? We can verify it by defining an integer variable.
import sys
n = 1
size = sys.getsizeof(n)
print(f"Size of the integer {n}: {size} bytes")

Yes, even if we only measure a constant, it is still 28 bytes.

The 28 bytes include the following components:
- Reference count (8 bytes)
- Type pointer (8 bytes)
- Size (8 bytes)
- Integer value (4 bytes)
All the above "fields" that exist for any Python object can be considered overhead and cause the 28 bytes for a simple integer.
Why does Python introduce these overheads? Of course, they must have their reasons for existing. Let's discuss them one by one.
3.1 Reference Count
The first overhead, "Reference Count", is used by Python for garbage collection. That is, every time the object is referenced by something, its reference count will be +1. The Python garbage collection process will release the memory when the reference count becomes 0. That means the current system process has no chance of using the object because there is no reference to it.
Let's see the following example.
import sys
a = [1, 2, 3] # rc = 1
b = a # rc = 2
c = a # rc = 3
print(sys.getrefcount(a)) # rc = 4 (the function created a temp reference)
del b
print(sys.getrefcount(a)) # rc = 3
del c
print(sys.getrefcount(a)) # rc = 2
del a # rc = 0, but we can't show that because there is no reference

In the above code, we created a list and assign it to the variable a
. Then, we define another two variables b
and c
to reference it. After that, we can use the function sys.getrefcount()
to check the reference count of the variable a
. The number should be 3, but since the function itself will create a temporary reference when it is trying to access its reference count, the output reference count will be 4.
Then, we deleted the variable b
, the reference count of a
reduced by 1. After we deleted the variable c
, the reference count of a
was reduced by another 1. At last, we deleted the variable a
. In fact, the reference count of it should be 0 now. However, since our program has removed all the references to it, we cannot access its references anymore.
Now, the variable a
has a 0 reference count. The garbage collection process will realise that the memory allocated for the variable a
can be freed up now.
If you want to know more about the garbage collection mechanism in Python, please check out the article below.
3.2 Type Pointer
Unlike most programming languages that use static typing (e.g., C/C++ and Java), Python is famous for its dynamic typing. Basically, Python allows an object to change its type at runtime flexibly. See the code below.
# Initially, the variable is an integer
my_var = 1
print(f"my_var is an integer: {my_var}, type: {type(my_var)}")
# Change type to string
my_var = "Hello, world!"
print(f"my_var is now a string: '{my_var}', type: {type(my_var)}")
# Change type to list
my_var = [1, 2, 3, 4, 5]
print(f"my_var is now a list: {my_var}, type: {type(my_var)}")
# Change type to a dictionary
my_var = {"key": "value"}
print(f"my_var is now a dictionary: {my_var}, type: {type(my_var)}")
# Change type to a function
def my_function():
return "I am a function"
my_var = my_function
print(f"my_var is now a function: {my_var}, type: {type(my_var)}")
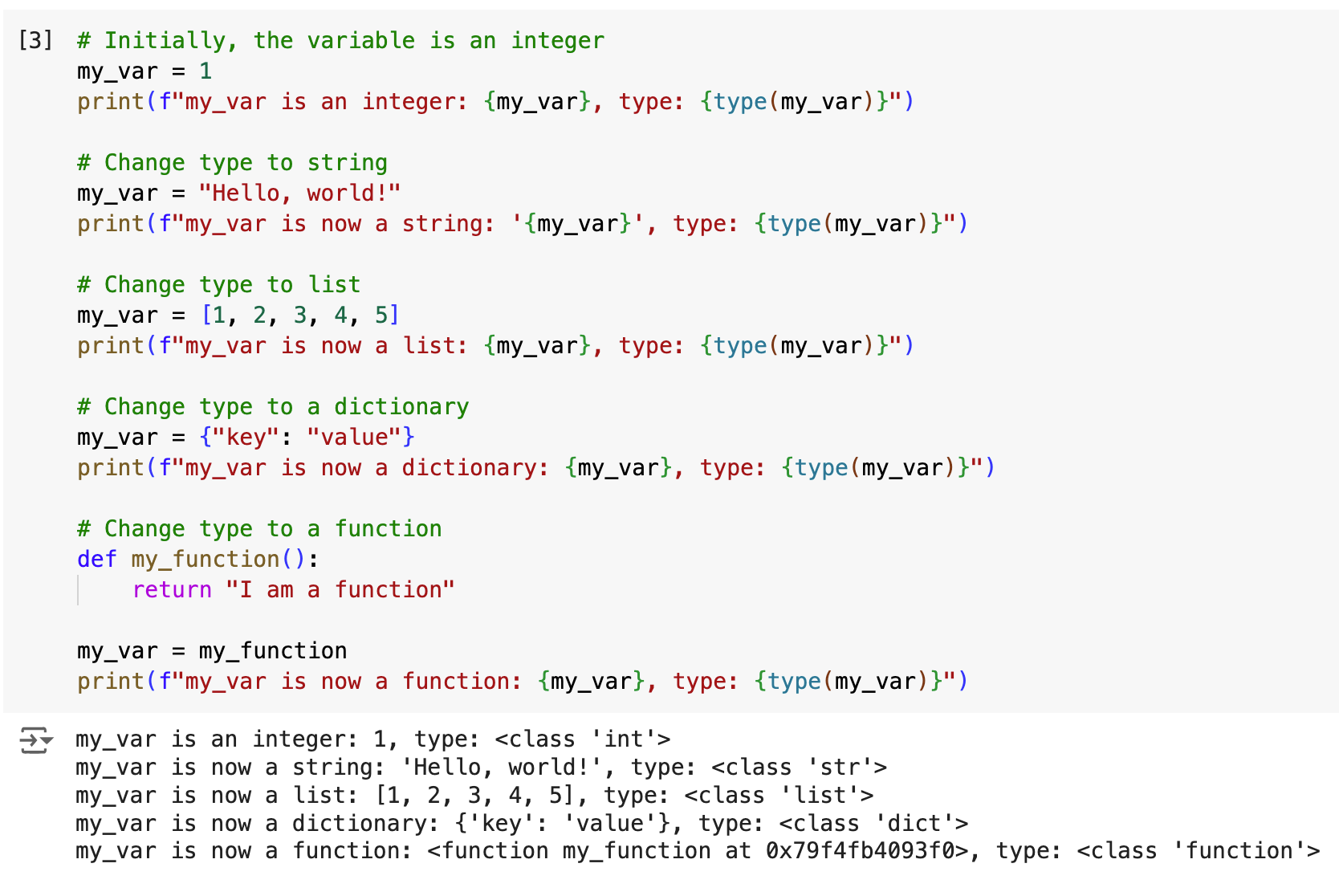
In the above code, we re-defined the variable my_var
multiple times, and the types are different every time. This is totally fine in Python. However, in most of the other programming languages, such as Java, we usually have to define a variable with a fixed type.
// Java Code
int myVar = 1
Therefore, to embrace this flexibility, Python has to use another 8 bytes for this "type pointer" to ensure an object has a dynamic type that can be changed in the runtime. In other words, this "type pointer" can be pointed to other types.
3.3 Size Fields
Again, this is also because of Python's dynamic feature. The precision and length for many types of variables in Python are dynamic. Therefore, Python will need this "overhead" to store this information.
This "size field" will indicate the precision in terms of an integer. Because of the arbitrary precision in Python, the "size" of the variable may have to accommodate the growth of this variable to support larger values or lengths.
3.4 Integer Value
Of course, the 28 bytes must include the number itself. By default, Python allocates 4 bytes to the integer value. In a 64-bit system, which I believe most of us are using right now, this means the number we can have is between -2³⁰+1 and 2³⁰-1. See the example below.
n = 2**30-1
size = sys.getsizeof(n)
print(f"Size of the integer {n}: {size} bytes")
n = 2**30
size = sys.getsizeof(n)
print(f"Size of the integer {n}: {size} bytes")

In the above example, when we tried the number 2³⁰, the total size of the object became 32 bytes. That's because the 4 bytes are not enough to store this integer value, so Python gave it another 4 bytes.
Your homework is to verify the other boundary, -2³⁰+1. Good luck