Automating Research Workflows with LLMs
Recently, I had the awesome opportunity to give a workshop at the Open Data Science Conference in London, and I discussed what I consider to be a potentially interesting role for LLMs in augmenting academic and non-academic researchers by automating certain groups of tasks.
In this article I want to dive into the core concepts discussed during that workshop, and discuss what I consider a fascinating emerging role for AI through integration with researchers in different fields.
Augmenting What?
The workshop I presented explored the question:
how can we leverage LLMs to enhance or augment research workflows without diminishing the cognitive engagement of researchers?
Touching on this topic of augmentation is always tricky and can lead to some cringy conversations about how AI will replace humans in the near future. Therefore, for the purpose of clarity I want to start by defining it a bit more concretely:
Augmentation = Enhancing Capability Through Tools
The concept of augmentation is deeply rooted in the work of Douglas Engelbart, who somewhat pioneered a version of this idea that technology should enhance human capabilities in an essay entitled: _Augmenting Human Intellect: A Conceptual Framework._
He suggests that tools – whether mechanical or cognitive – should amplify a person's ability to approach complex problems, not replace the process of understanding.
By augmenting human intellect we mean increasing the capability of man to approach complex problem situation to gain comprehension to suit his particular needs and to derive solutions to problems
In the same way, LLMs can be effectively used as amplifying tools to help researchers manage, synthesise, and navigate vast amounts of data and information, enabling deeper comprehension and faster problem-solving.
LLM Tangibles
The term "LLM tangibles" refers to the real-world applications and capabilities that LLMs can provide in research settings. This term was inspired by this quote from Engelbart from the same essay:
We refer to way of life in an integrated domain where hunches cut-and-try intangibles and the human feel for situation usefully coexist with powerful concepts streamlined terminology and notation sophisticated methods and highpowered electronic aids
But I call it tangibles to emphasize the atomic and practical nature of their usage.
I think LLMs are incredibly versatile and can reduce cognitive load by performing granular research tasks such as generating hypotheses, extracting key findings from papers, and organizing data in usable formats.
My contention is that rather than to think of LLMs as potential replacements for human effort in the broad sense, we should look at them atomically, in terms of research primitives, where __ LLMs can perform on demand small-scale repetitive tasks or semantic-like procedures and therefore, amplify a researcher's ability to perform effective knowledge work and problem solving.
Cool LLM-Based UI Patterns
The inspiration for talking about this came from a bunch of really interesting UI patterns I've seen recently that leverage LLMs in this way, namely I would mention:
- Elicit Table UI

Elicit is a fascinating tool designed to help researchers automate their workflows by allowing them to ask research questions and get structured, data-driven responses.
The app organizes data from multiple research papers in a well-thought out table ui experience that presents them accompanied by helpful columns such as main findings , summary, methodology and so on. In the backend, LLMs are used to generate these data points across multiple papers, allowing for effective cross-paper comparison in one interface.
2. Semantic Zooming Inspired by Amelia Wattenberger's awesome talk _Climbing the ladder of abstraction:_
semantic zooming is this concept of using LLMs to view information at different levels of complexity. By using an LLM's ability to summarize a book for example you can explore it at different levels, for example getting just one sentence glimpse per chapter, before you dive into the book.
3. Latent Space Exploration of Ideas with Luminate The last example I'll mention is Luminate, which is this paper that recently came out, accompanying a really cool demo where you can explore ideas for writing stories across different semantic dimensions such as tone, character development, style, etc..
This tool uses LLMs to create this latent space-like mapping of ideas, packed into a super intuitive UI that offers a unique way to explore concepts visually and conceptually, allowing you to zoom in and out of different ideas, compare generations across semantic dimensions of interest and more.

Level 0: Prompting for Better Research Workflows
Ok, so all of these ideas carry in common the fact that they make use of very simple LLM capabilities, but by integrating them as primitives into an intuitive and useful app, they lead to some pretty impressive results.
My biggest pitch with this article is that LLMs can be effectively leveraged to compose semantically meaningful primitives that can be chained together into powerful workflows.
The first level of this amplification (what I call Level 0) can be very easily understood and refers to the most basic application of LLMs for improving research workflows: good prompts.
More specifically, system messages (the messages that describe the overall desired behavior from models like ChatGPT) can be used as utility functions to perform specific, granular tasks.
When researchers use well-structured prompts, they can extract more meaningful data, organize information more effectively, and ensure that the LLM's outputs are reliable and verifiable.
Key principles for Level 0 prompting:
- System messages as utility functions
- _Prompts should perform actions that are
- Reliable
- Easily verifiable
- Produce one meaningful data transformation_
I show below a few examples in the context of research using Python code:
- Argument Construction
from openai import OpenAI
client = OpenAI()
MODEL='gpt-4o-mini'
def get_response(prompt_question, sys_msg):
response = client.chat.completions.create(
model=MODEL,
messages=[{"role": "system", "content": sys_msg},
{"role": "user", "content": prompt_question}]
)
return response.choices[0].message.content
SYS_MSG_ARG_CONSTRUCT = """
# Argument Construction Utility
You are a research expert specialized in constructing arguments. Your task is to build a logical and persuasive argument based on the given topic or claim. Follow these steps:
1. Identify the main claim or thesis.
2. List key supporting points.
3. Organize points in a logical flow.
4. Anticipate potential counterarguments.
5. Provide a concluding statement that reinforces the main claim.
Respond with a structured argument without additional commentary.
"""
prompt_question = """
Claim: Artificial Intelligence (AI) will increase job opportunities rather than eliminate them.
"""
response = get_response(prompt_question, SYS_MSG_ARG_CONSTRUCT)
print(response)
- Hypothesis Generation
from openai import OpenAI
client = OpenAI()
MODEL='gpt-4o-mini'
def get_response(prompt_question, sys_msg):
response = client.chat.completions.create(
model=MODEL,
messages=[{"role": "system", "content": sys_msg},
{"role": "user", "content": prompt_question}]
)
return response.choices[0].message.content
SYS_MSG_HYP_GEN = """
You are a research expert specialized in generating relevant, valid, and robust hypotheses. You will:
1. Analyze the given research question or problem.
2. Generate multiple plausible hypotheses.
3. Rank hypotheses by plausibility and testability.
4. Suggest potential experiments or data collection methods to test each hypothesis.
5. Identify potential confounding variables for each hypothesis.
Provide a list of generated hypotheses with brief explanations and testing suggestions.
"""
prompt_question = """
Research question: How does remote work affect employee Productivity in software development teams?
"""
response = get_response(prompt_question, SYS_MSG_HYP_GEN)
print(response)
These 2 examples simply leverage the OpenAI API via some simple Python function, to call the gpt-4o-mini
model, with well considered system messages to perform common Research procedures.
Level 1: Research Primitives with Pydantic and Structured Outputs
Level 1 takes LLM-augmented research workflows to the next level by combining structured outputs with Python's Pydantic library (data validation framework) to capture these research primitives as _semantic data structures_ (not 100% sure about this name but it is the best I could come up with).
These primitives are atomic components of research workflows, such as: hypothesis generation, evidence gathering and assessment, etc..
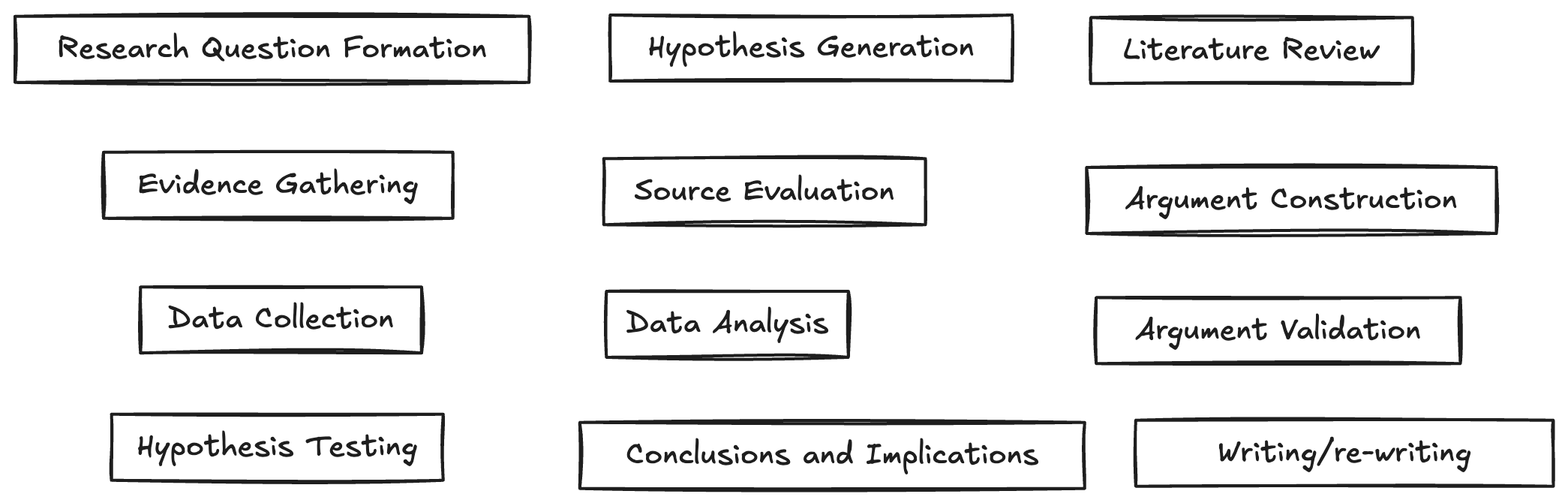
The core insight here is that these structures can have human-written validation procedures and then be used and composed for more complex workflows that leverage LLMs in the backend.
By defining data structures for these research components, researchers can maintain control over their workflows, ensuring that LLMs produce verifiable, reliable, and reusable statements, data and so on.
Examples of Structured LLM Based Research Workflows
In practice, LLMs can automate large parts of literature reviews by generating structured outputs that help researchers identify relevant papers, analyze the findings, and formulate hypotheses.
import arxiv
from datetime import datetime
SYS_MSG_KEYWORD_SEARCH = """
You are a search engine that translates search topics into a list of relevant keywords for effective search.
take in a research topic and you output.
"""
SYS_MSG_SEARCH_QUERIES = """
You are a search engine that takes in a research topic and you output a list of relevant
that perfectly encapsulate that topic.
"""
SYS_MSG_RELEVANCY_JUDGE = """
You are a research expert and an evalation engine for research results given a research topic of interest.
Given a research topic you output a binary score yes|no to determine if a paper summary is strictly relevant to the topic
or not.
"""
from openai import OpenAI
from pydantic import BaseModel, Field
from typing import List, Literal
client = OpenAI()
class Keywords(BaseModel):
keywords: List[str] = Field(..., description="List of relevant keywords to search for")
class SearchQueries(BaseModel):
queries: List[str] = Field(..., description="List of queries to search for")
def generate_keywords_for_search(research_topic):
"""
"""
response = client.beta.chat.completions.parse(
model=MODEL,
messages=[{"role": "system", "content": SYS_MSG_KEYWORD_SEARCH},
{"role": "user", "content": research_topic}],
response_format=Keywords
)
return response.choices[0].message.parsed
def generate_search_queries(research_topic, num_queries=5):
response = client.beta.chat.completions.parse(
model=MODEL,
messages=[{"role": "system", "content": SYS_MSG_SEARCH_QUERIES},
{"role": "user", "content": f'Generate {num_queries} search queries for this research topic: {research_topic}'}],
response_format=SearchQueries
)
return response.choices[0].message.parsed
class RelevantPaper(BaseModel):
relevancy_score: Literal['yes', 'no'] = Field(description="A binary score yes|no if a paper is relevant given a research topic.")
justification: str = Field(description="A short one sentence justification for the relevancy score.")
def filter_paper_relevancy(research_topic, paper_summary):
paper_relevancy_score = client.beta.chat.completions.parse(
model='gpt-4o-mini',
messages=[
{
'role': 'system', 'content': SYS_MSG_RELEVANCY_JUDGE,
'role': 'user', 'content': f'Given this research topic: {research_topic}, score the relevancy of this paper:nn {paper_summary}'
}
],
response_format=RelevantPaper
)
return paper_relevancy_score.choices[0].message.parsed
def filter_out_search_results(research_topic: str, search_results: dict):
filtered_results = []
for paper in search_results:
relevancy = filter_paper_relevancy(research_topic, paper.summary)
if relevancy.relevancy_score=='yes':
filtered_results.append((paper, relevancy.justification))
elif relevancy.relevancy_score=='no':
continue
else:
raise ValueError("Invalid relevancy score")
return filtered_results
arxiv_client = arxiv.Client()
def search_arxiv_papers(keywords, year=2024, MAX_NUM_PAPERS=30):
query = ' '.join(keywords)
# Define the start and end dates for the specified year
start_date = datetime(year, 1, 1)
end_date = datetime(year, 12, 31)
# Append the date range filter to the query
date_filter = f'submittedDate:[{start_date.strftime("%Y%m%d%H%M%S")} TO {end_date.strftime("%Y%m%d%H%M%S")}]'
full_query = f'{query} AND {date_filter}'
# Perform the search
search = arxiv.Search(query=full_query, max_results=MAX_NUM_PAPERS)
# Fetch the results
results = list(arxiv_client.results(search))
return results
research_topic = "LLMs for enhancing human's ability to research and learn"
keywords = generate_keywords_for_search(research_topic)
structured_results = search_arxiv_papers(keywords.keywords)
filtered_papers = filter_out_search_results(research_topic, structured_results)
full_search_result = []
for query in search_queries:
keywords = generate_keywords_for_search(query)
structured_results = search_arxiv_papers(keywords.keywords)
full_search_result.extend(filter_out_search_results(research_topic, structured_results))
full_search_result
For example, in the code above I use tools like the arxiv
API integrated with LLMs to pull and filter relevant papers, making the literature review process faster and more thorough by leveraging LLMs to help me assess the relevance of different papers.
LLMs can also help with evidence assessment and inspection:
from pydantic import BaseModel, Field, field_validator
from typing import List, Literal
class Evidence(BaseModel):
positions: List[Literal['yes', 'no', 'neutral']] = Field(description="A List with 1-3 ternary scores presenting the positions of the paper regarding the query/hypothesis in question as yes|no|neutral.")
position_descriptions: List[str] = Field(description="List with 1-3 statements presenting the position for, against or neutral regarding the query/hypothesis n question.")
evidence: List[str] = Field(description="List of DIRECT QUOTES serve as evidence to support each position.")
@field_validator('positions', 'position_descriptions', 'evidence')
def validate_list_size(cls, values):
if len(set(map(len, values))) != 1:
print("Uncertainty in results, retrying...")
return values
SYS_MSG_EVIDENCE = """
You are a helpful research assistant. Given a research question or hypothesis you
will inspect the contents of papers or articles for evidence for or against
the respective hypothesis in question. Your output will be 2 fields:
- positions: List of ternary scores (yes|no|neutral) presenting the positions of the paper regarding the query/hypothesis in question.
- positions_descriptions: List of a short one sentence statements summarizing the position from the text regarding the user's query or hypothesis, like: "Yes this paper validates this idea by pointing out...." etc..
- evidence: a list with DIRECT QUOTES from the paper containing information that supports and validates each position. It should be one quote to validate each position.
All 3 fields should be lists with the same size.
"""
MODEL = "gpt-4o-2024-08-06"
from openai import OpenAI
client = OpenAI()
def inspect_evidence(prompt_question):
response = client.beta.chat.completions.parse(
model=MODEL,
messages=[{"role": "system", "content": SYS_MSG_EVIDENCE},
{"role": "user", "content": prompt_question}],
response_format=Evidence,
)
return response.choices[0].message.parsed
# It's actually the entire first page but bare with me.
abstract = pdf_pages[0]
# source: https://www.semanticscholar.org/paper/Improvements-to-visual-working-memory-performance-Adam-Vogel/e18bbb815cf36aa75ef335787ecd9084d418765e
hypothesis = "Working memory training in humans might lead to long-term far transfer improvements in some cognitive abilities."
prompt = f"""
Does this abstract:
{abstract}nn
presents evidence for the following statement:
{hypothesis}
"""
output = inspect_evidence(prompt)
output
for p,pd,e in zip(output.positions, output.position_descriptions, output.evidence):
print(f"Position: {p}nPosition Description: {pd}nEvidence: {e}nn")
print("****")
def inspect_evidence_for_hypothesis(hypothesis: str, source_content: str):
"""Inspects the evidence for, against or neutral regarding a research hypothesis."""
prompt = f"""
Does this abstract:
{source_content}nn
presents evidence for the following statement:
{hypothesis}
"""
output = inspect_evidence(prompt)
return output
def display_output_evidence(output):
for p,pd,e in zip(output.positions, output.position_descriptions, output.evidence):
print(f"Position: {p}nPosition Description: {pd}nEvidence: {e}nn")
print("****")
# source for this abstract: https://pubmed.ncbi.nlm.nih.gov/35107614/
hypothesis_fluid_intelligence = "Working memory training might lead to long lasting improvements in fluid intelligence."
abstract_with_argument_for_improvement_in_fluid_intelligence = """
Abstract
Process-based working memory (WM) training in typically developing children usually leads to short- and long-term improvements on untrained WM tasks. However, results are mixed regarding far transfer to academic and cognitive abilities. Moreover, there is a lack of studies jointly evaluating the different types of transfer, using an adequate design and considering motivational factors. In addition, evidence is needed about how pre-training performance is related to individual differences in training-induced transfer. Therefore, this study aimed to implement and evaluate the efficacy of a computerized process-based WM training in typically developing school-age children. Near and far transfer effects were evaluated both immediately after training and after 6 months, as well as individual differences in training-induced transfer. The sample was composed of 89 typically developing children aged 9-10 years (M = 9.52, SD = 0.30), who were randomized to a WM training group or an active control group. They were evaluated at pre-training, post-training, and follow-up phases with measures of visuospatial and verbal WM, reading comprehension, math computation, and fluid intelligence. Results showed that the training group significantly improved performance in verbal WM and fluid intelligence compared to the active control group, immediately after training and after 6 months. Trained children with lower initial performance in verbal WM or fluid intelligence showed greater transfer gains. No group differences were found in motivational factors. Findings of this study suggest that process-based WM training may promote transfer to cognitive abilities and lead to compensation effects of individual differences in typically developing school-age children.
"""
output = inspect_evidence_for_hypothesis(hypothesis_fluid_intelligence, abstract_with_argument_for_improvement_in_fluid_intelligence)
display_output_evidence(output)
In this piece of code, actually inspired by this amazing talk by Jason Liu, creator of pydantic, I created these "semantic data structures" to read through a paper and inspect whether or not they provide sensible evidence given a hypothesis, accompanied by quotable evidence to validate and verify their assessment.
Level 2 Agentic Workflows and Iterative Research Reports
For this section, I want to note an interesting yet experimental workflows that leverage agents for iterative research reporting.
Using tools like GPT Researcher, researchers can generate, refine, and verify draft research reports iteratively, allowing them to get the ‘lay of the land', when tackling more unknown topics.
These reports are based on multiple sources, and each step can be checked and cross-checked against the original source, making it a powerful tool for generating high-quality research documents.



Composability: LLM in the Terminal
Before I go I'd like to mention a very cool tool by one of my favorite people to follow in this space of LLM+tools: Simon Willison.
He made a tool called: LLM (I know, how lucky right?)—it essentially allows you to to interact with LLMs directly from the terminal, running queries and managing tasks in a command-line environment.
This level of control allows you to pipe regular command and llm prompts together, giving the user a level composability that enables them to create some mind-boggling automations in single one-liners in bash.
If you prefer video form, check out my Youtube video on this topic here:
Final Thoughts
I like framing this discussion as a search towards the best ways for humans and LLMs to interface. In the future I'd like to research more about integrating evaluation mechanisms at the individual usage level to mitigate the negative effects of hallucinations and detect incoherences, ensuring reliable results at the real-time usage level.
Some key questions for the future:
- How can evaluation be integrated seamlessly into LLM-powered workflows for non-experts, meaning, when we can't implement engineering infrastructure to tackle hallucinations for example.
- What principles will guide the most effective real-time collaboration between humans and LLMs?
That's it! Thanks for reading!
If you want to support me check out my course on prompt engineering:
References
- Augmenting Human Intellect
- LLMs as Zero-Shot Hypothesis Proposers
- Machine-assisted mixed methods: augmenting humanities and social sciences with AI
- Elicit tool
- Luminate: Structured Generation and Exploration of Design Space
- Luminate paper
- Climbing the ladder of abstraction by Amelia Wattenberger
- OpenAI Structured Outputs blog post
- GPT Researcher