Deploying your Llama Model via vLLM using SageMaker Endpoint
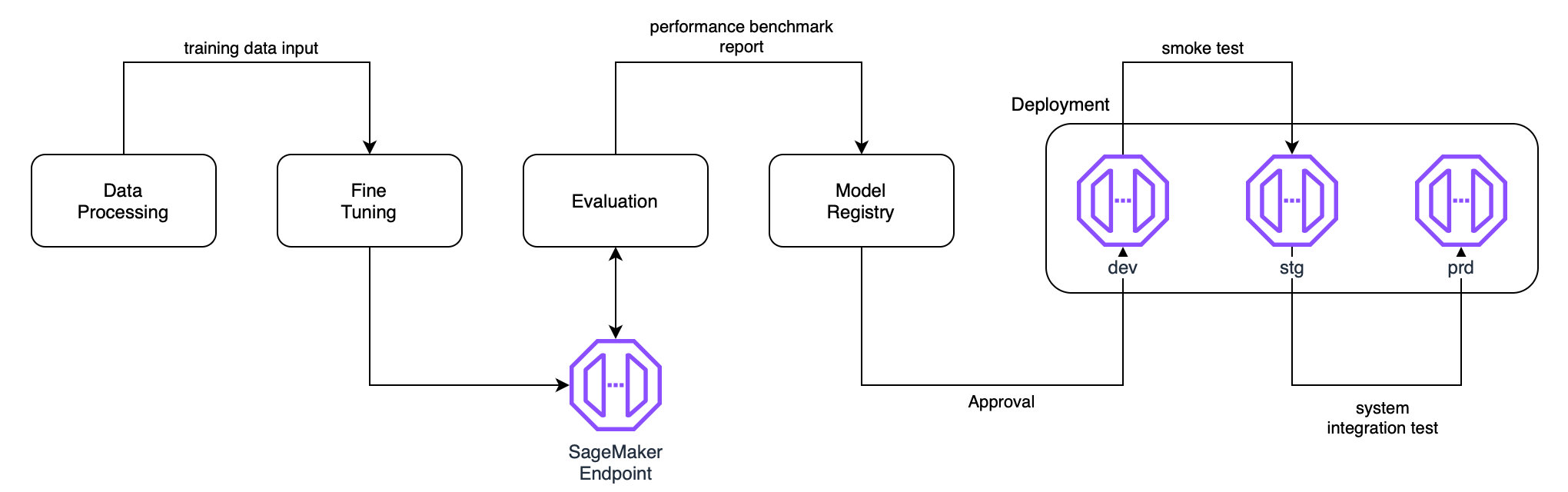
In any machine learning project, the goal is to train a model that can be used by others to derive a good prediction. To do that, the model needs to be served for inference. Several parts in this workflow require this inference endpoint, namely, for model evaluation, before releasing it to the development, staging, and finally production environment for the end-users to consume.
In this article, I will demonstrate how to deploy the latest Llm and serving technologies, namely Llama and vLLM, using AWS's SageMaker endpoint and its DJL image. What are these components and how do they make up an inference endpoint?

SageMaker is an AWS service that consists of a large suite of tools and services to manage a Machine Learning lifecycle. Its inference service is known as SageMaker endpoint. Under the hood, it is essentially a virtual machine self-managed by AWS.
DJL (Deep Java Library) is an open-source library developed by AWS used to develop LLM inference docker images, including vLLM [2]. This image is used in the SageMaker endpoint.
vLLM, introduced in 2023, is an open-source inference framework optimized for serving LLM models [1]. It uses an innovative GPU memory management which enables fast inference and reduces memory usage. It is prebuilt in one of the DJL images.
Contents
Installation Endpoint Creation Prediction Endpoint Update & Deletion Summary
Installation
Only two Python libraries are required. boto3
is AWS's low-level API used to manage all types of AWS resources. sagemaker
is their high-level API developed to manage only AWS's SageMaker resources. They are updated frequently, hence future versions may have backward compatibility issues.
pip install boto3~=1.35.5 sagemaker~=2.229.0
Creating an Endpoint
The basics
from sagemaker import image_uris
from sagemaker.model import Model
# 1) variables
aws_region = "us-east-1"
instance_type = "ml.g5.12xlarge"
model_data = "s3-mymodel-bucket/model/"
infer_instance_role = "iamr-vllm-inference"
name = "my-vllm-endpoint"
# 2) obtain inference ECR image uri
container_uri = image_uris.retrieve(
framework="djl-lmi",
region=aws_region,
version="0.29.0"
)
# 3) create model object
model = Model(
name=name,
image_uri=container_uri,
role=infer_instance_role,
# model_data=model_data,
env={
"HF_MODEL_ID": model_data,
"TENSOR_PARALLEL_DEGREE": "max",
"OPTION_ROLLING_BATCH": "vllm",
"OPTION_MAX_ROLLING_BATCH_SIZE": "64",
}
)
# 4) deploy model endpoint
model.deploy(
instance_type=instance_type,
initial_instance_count=1,
endpoint_name=name,
wait=False
)
You just need the above code to deploy an LLM model. Breaking down each part:
- Variables are defined, including the AWS region name, instance type, and S3 dir path to the LLM model. An instance role also needs to be created, minimally allowing access to the S3 path, ECR image, and pushing to the Cloudwatch Logs. Note that the model needs to be in HuggingFace's format to be served using vLLM [3].
- The DJL prebuilt inference image in ECR's URI is then retrieved, using the framework
djl-lmi
(large model inference). You may find the latest and full list of images at [4]. - A model object is then specified to specify the instance role and image URI. Of particular importance is to set the environment variables using the
OPTION_ROLLING_BATCH
with the value ofvllm
and theHF_MODEL_ID
with the S3 bucket. - Last,
model.deploy()
will create the model object, an endpoint configuration, and deploy a live endpoint.wait=False
will stop the script after it completes, otherwise, it will continuously poll SageMaker till it obtains a success or failure status of the endpoint creation.
Model object, endpoint configuration, endpoint
The three earlier stated components are viewable on SageMaker's page and they each serve a different purpose as defined below. In particular, you may treat the model object and endpoint configuration to be constructs of the live endpoint.


Alternative model download path
There is one important part regarding how to retrieve the LLM model from your S3 bucket not covered in AWS's existing documentation. The previous example assumes the model or models are stored within a directory in a bucket. All contents within that directory will be downloaded to the endpoint server. However, if you have compressed the files in a .tar.gz
format, you may call the file directly and then include the path in the model_data
argument in the Model()
object instead.
# to specify a compressed tar.gz model file
model_data = "s3-mymodel-bucket/model/model.tar.gz"
# use model_data arg instead of env var
model = Model(
name=name,
image_uri=container_uri,
role=infer_instance_role,
model_data=model_data,
env={
# "HF_MODEL_ID": model_data,
"TENSOR_PARALLEL_DEGREE": "max",
"OPTION_ROLLING_BATCH": "vllm",
"OPTION_MAX_ROLLING_BATCH_SIZE": "64",
}
)
Endpoint Architecture

This is how a SageMaker endpoint is related to its associated resources. At the initial launch, the prebuilt inference image is pulled into a managed instance, and the model is also downloaded from the S3 bucket. All logs will be pushed to an automatically created Cloudwatch log group.
Optionally, you can also define the subnets, and security group of the managed instance to enhance its security by defining them in the model object.
# specify subnets and security groups
vpc_config = {
"Subnets": ["subnet-12345668", "subnet-23456789"],
"SecurityGroupIds": ["sg-12345678"]
}
# add to model object
model = Model(
name=name,
image_uri=container_uri,
role=infer_instance_role,
vpc_config=vpc_config,
env={
"HF_MODEL_ID": model_data,
"TENSOR_PARALLEL_DEGREE": "max",
"OPTION_ROLLING_BATCH": "vllm",
"OPTION_MAX_ROLLING_BATCH_SIZE": "64",
}
)
Additionally, an auto-scaling group can also be attached to a deployed endpoint to dynamically adjust its availability based on schedules or traffic conditions. Creation of such resources like this, S3, ECR, etc., are better managed through infrastructure-as-code like Terraform, which is a separate topic on its own, and hence will not be covered here.
Prediction
SageMaker SDK
import json
from sagemaker import serializers
from sagemaker import Predictor
# payload
input_data = {
"inputs": "What is LLM?",
#add your params
"parameters": {
"max_tokens": 2400,
"temperature": 0.01
}
}
# predictor class
name = "my-vllm-endpoint"
predictor = sagemaker.Predictor(
endpoint_name=name,
serializer=serializers.JSONSerializer(),
)
# post request
response = predictor.predict(input_data)
response_data = json.loads(response)
print(response_data)
Sending a request to the created endpoint is simple as shown above using the Predictor
class from SageMaker's SDK [5].

HTTPS Request
There will be cases whereby using a high-level SDK to send a send request like the above is not possible as services calling it require more traditional methods through a POST request via an HTTPS URL.

You may locate the URL in the AWS Management Console > SageMaker > Endpoints > [endpoint-name].
import json
import boto3
import requests
from botocore.auth import SigV4Auth
from botocore.awsrequest import AWSRequest
from botocore.session import Session
# payload
payload = {
"inputs": "What is LLM?",
"parameters": {
"max_tokens": 2400,
"temperature": 0.01
}
}
# variables
region = "us-east-1"
endpoint = "my-vllm-endpoint"
endpoint_url = f"https://runtime.sagemaker.{region}.amazonaws.com/endpoints/{endpoint}/invocations"
# generate signed header
credentials = Session().get_credentials()
request = AWSRequest(
method='POST',
url=endpoint_url,
data=json.dumps(payload),
headers={"Content-Type": "application/json"}
)
SigV4Auth(credentials, 'sagemaker', region).add_auth(request)
signed_header = dict(request.headers)
# post request
response = requests.post(
request.url,
headers=signed_header,
json=payload
)
print(response.json())
With that, you then need to create an AWS-signed header using boto3
and send it together with the payload using third-party libraries like in this case, the popular requests
library.
Chat Completion
The latest djl-lmi
image of version 0.29.0
supports chat completion and the schema is compatible with OpenAI's format [6]. This allows us to instruct the model to assume different roles so that the response can be tuned specifically.
import json
from sagemaker import Predictor, serializers
name = "my-vllm-endpoint"
predictor = Predictor(
endpoint_name=name,
serializer=serializers.JSONSerializer(),
)
system_prompt = "you are an intelligent investor"
user_prompt = "what is the best way to make a million?"
# openai chat completion format
input_data = {
"messages": [
{"role": "system", "content": system_prompt},
{"role": "user", "content": user_prompt},
],
"max_tokens": 2400,
"temperature": 0.01
}
response = predictor.predict(input_data)
response = json.loads(response)
print(json.dumps(response, indent=4))
In the above example using SageMaker SDK, a system prompt is instructing the model to assume as an intelligent investor, and then a user prompt on how to make a million dollars is sent to it. (Note that this is just for demonstration purposes and does not constitute finance advice.)
Note that to use chat completion, the request payload must be within the messages
instead of the previously stated input
key in the SageMaker standard schema. The parameters are also not encapsulated within the parameters
key.

Endpoint Update & Deletion

from sagemaker import Predictor
from sagemaker.model import Model
predictor = Predictor(
endpoint_name=name,
)
# delete endpoint & endpoint configuration
predictor.delete_endpoint(
delete_endpoint_config=True
)
For each SageMaker endpoint that you launch, you will need to eventually destroy it, like those used for temporary model evaluation. To tear down your endpoint and endpoint configuration, you can use predictor.delete_endpoint()
.
# update endpoint configuration, e.g, instance type -------
predictor.update_endpoint(
instance_type="ml.g5.4xlarge",
wait=False
)
# update model object -------
# e.g., use a diff model
model_name = "my-new-model"
model_data = "s3-mymodel-bucket/model/model2.tar.gz"
model = Model(
name=model_name,
image_uri=container_uri,
role=infer_instance_role,
model_data=model_data,
env={
"TENSOR_PARALLEL_DEGREE": "max",
"OPTION_ROLLING_BATCH": "vllm",
"OPTION_MAX_ROLLING_BATCH_SIZE": "64",
}
)
predictor.update_endpoint(
model_name=model_name
wait=False
)
The first model you fine-tuned will rarely be the final version, and it is often necessary to update an existing endpoint; whether to update to a better model or change the configurations used in the development, staging, or production environments. This can be done with the predictor.update_endpoint()
. Note that any updates of endpoint configurations specifically from here will result in a new one being created.
Summary
This article shows how one can deploy their Llama model using vLLM via AWS's SageMaker endpoint. While it specifically targets the said LLM deployment, the code and workflow shown are generally applicable to all forms of model deployments in the SageMaker platform.
Nonetheless, I have only covered a small aspect of an Mlops engineer's scope of work, and SageMaker itself is a complex platform to master. In future articles, I will share more on how the entire machine-learning lifecycle can be automated on this platform. Meanwhile, you can check out a previous article I wrote in this domain.
References
- [1] Efficient Memory Management for Large Language Model Serving with PagedAttention (2023) [link]
- [2] AWS Deep Java Library: vLLM guide [link]
- [3] AWS Deep Java Library: HuggingFace transformers format [link]
- [4] GitHub: AWS's large model inference images list [link]
- [5] Sagemaker Python SDK: Predictor class [link]
- [6] AWS Deep Java Library: Chat completion support [link]