Mapping out the connections of Oscar Winners

In this short piece, I use public Wikipedia data, Python programming, and network analysis to extract and draw up a network of Oscar-winning actors and actresses.
All images were created by the author.
1. Wikipedia as a data source
Wikipedia, as the largest free, crowdsourced online encyclopedia, serves as a tremendously rich data source on various public domains. Many of these domains, from film to politics, involve various layers of networks underneath, expressing different sorts of social phenomena such as collaboration. Due to the approaching Academy Awards Ceremony, here I show the example of Oscar-winning actors and actresses on how we can use simple Pythonic methods to turn Wiki sites into networks.
2. Collecting the list of winners
First, let's take a look at how, for instance, the Wiki list of all Oscar-winning actors is structured:

This subpage nicely shows all the people who have ever received an Oscar and have been granted a Wiki profile (most likely, no actors and actresses were missed by the fans). In this article, I focus on acting, which can be found in the following four subpages – including main and supporting actors and actresses:
Python">urls = { 'actor' :'https://en.wikipedia.org/wiki/Category:Best_Actor_Academy_Award_winners',
'actress' : 'https://en.wikipedia.org/wiki/Category:Best_Actress_Academy_Award_winners',
'supporting_actor' : 'https://en.wikipedia.org/wiki/Category:Best_Supporting_Actor_Academy_Award_winners',
'supporting_actress' : 'https://en.wikipedia.org/wiki/Category:Best_Supporting_Actress_Academy_Award_winners'}
Now let's write a simple block of code that checks each of these four listings, and using the packages urllib and beautifulsoup, extracts the name of all artists:
from urllib.request import urlopen
import bs4 as bs
import re
# Iterate across the four categories
people_data = []
for category, url in urls.items():
# Query the name listing page and parse it
sauce = urlopen(url).read()
soup = bs.BeautifulSoup(sauce,'lxml')
# Search for all URLs - trick: we will see that all lists start with 'Academy Award for...' and then the category name
# which will serve as a stop signal
its_a_person = False
for a in soup.find_all('a', {'href': re.compile('/wiki/')}):
if 'Categories' == a.text:
its_a_person = False
if its_a_person:
people_data.append({'name' : a.text,
'category' : category})
if 'Academy Award for Best ' + category.replace('_', ' ').title() == a.text:
its_a_person = True
The result of this data collection looks as follows, when turned into a Pandas DataFrame with the following snippet:
import pandas as pd
df = pd.DataFrame(people_data)
df

3. Download artist profiles
Once we have the names of each person, we can download their full profile – for instance, as the total text or the summary of their Wikipedia pages using the Wikipedia API.
Let's init the API:
import wikipediaapi
wiki_wiki = wikipediaapi.Wikipedia('Oscar Network Project', 'en')
wiki_wiki = wikipediaapi.Wikipedia( 'en')
Prepare folders to store the data, and get the names of artists into a list:
import os
folderout_1 = 'wiki_page_summary'
folderout_2 = 'wiki_page_content'
if not os.path.exists(folderout_1): os.makedirs(folderout_1)
if not os.path.exists(folderout_2): os.makedirs(folderout_2)
names = df.name.to_list()
Now, let's see how the API works, for instance, when querying for Jack Nicholson:
page = wiki_wiki.page('Jack Nicholson')
page.summary
The result of this block is the summary text of Nicholson's Wiki page:

Similarly, we can query the full text of each actor and actress's Wiki page as well. Now, using this, let's write a quick loop that iterates over all artist names and saves both their profile summary and the full texts too:
for idx, name in enumerate(names):
if idx%10==0:
print(idx)
if not os.path.exists(folderout_2 + '/' + name ):
page = wiki_wiki.page(name)
fout = open(folderout_1 + '/' + name , 'w')
fout.write(page.summary)
fout.close()
fout = open(folderout_2 + '/' + name , 'w')
fout.write(page.text)
fout.close()
To double-check, run this cell of code that should result in 314 profiles acquired:
print(len(os.listdir(folderout_1)))
print(len(os.listdir(folderout_1)))
4. Building the network
Quick recap: First, we collected the leading and supporting actors and actresses list. Then, I downloaded the full textual profile of each artist from Wikipedia, containing a detailed wordy description of their life and career. At the same time, these are by no means standardized lexicon articles; it's safe to assume that the collective wisdom and spirits of fans editing their favorite stars' Wikipedia pages results in a relatively homogenous pool of articles.
These articles, consequently, and supposedly, also include any major life events these stars have, including their awards and, even more so, their various encounters with other celebrities, including Oscar-winning actors and actresses, also present in Wikipedia.
And here we are: the logic behind my network is to count the number of times each artist's profile text mentions any of the other artists present in the Oscar-winning pool. Consequently, the nodes in this network are Oscar-winning artists, who are linked if their profiles reference each other, with a connection strength that is proportional to the number of these references.
Let's see this in Python! First, load the input data into a dictionary:
names_texts = {}
for name in [f for f in os.listdir(folderout_2) if '.DS' not in f]:
with open(folderout_2 + '/' + name) as myfile:
names_texts[name] = myfile.read().split('External links')[0]
len(names_texts)
Now count each name occurrence in each profile text, and in case there are more than 0 matches, add the name-pair of two artists into our network defined as a NetworkX graph object:
import networkx as nx
G = nx.Graph()
edges = {}
for name1, text1 in names_texts.items():
for name2, text2 in names_texts.items():
if name1 != name2:
weight = text1.count(name2) + text2.count(name1)
if weight > 0:
#print(name1, name2, weight)
G.add_edge(name1, name2, weight = weight)
print(G.number_of_nodes(), G.number_of_edges())
This code block outputs that in the Oscar network, we have 307 nodes and 2,368 edges, meaning that seven actors or actresses were not linked to anyone in the graph, while the rest of them bear altogether more than 2000 connections.
Let's visually observe the network, first using the most simple built-in drawer:
nx.draw(G)
Result:

It's not very visually appealing; now, can we really infer anything from the figure? Let's try to tweak the NetworkX visual using the underlying Matplotlib commands and see what we get:
import matplotlib.pyplot as plt
f, ax = plt.subplots(1,1,figsize=(13,13))
node_degrees = dict(G.degree())
node_sizes = [v * 20 for v in node_degrees.values()]
pos = nx.spring_layout(G, k = 1.4)
nx.draw(G, pos, with_labels=True, node_size=node_sizes, alpha = 0.7)

Here, we finally see some structure; a few nodes have much more connections than the rest (hubs), while there are many weakly connected artists on the periphery of the network.
Nevertheless, we are there – as the goal of this article was to show how we can build a network of actors and actresses based on Wikipedia data, which we just obtained! Finally, a closing figure that was separately created in Gephi and will be part of my next article, showing a lot more details and insights into the graph:
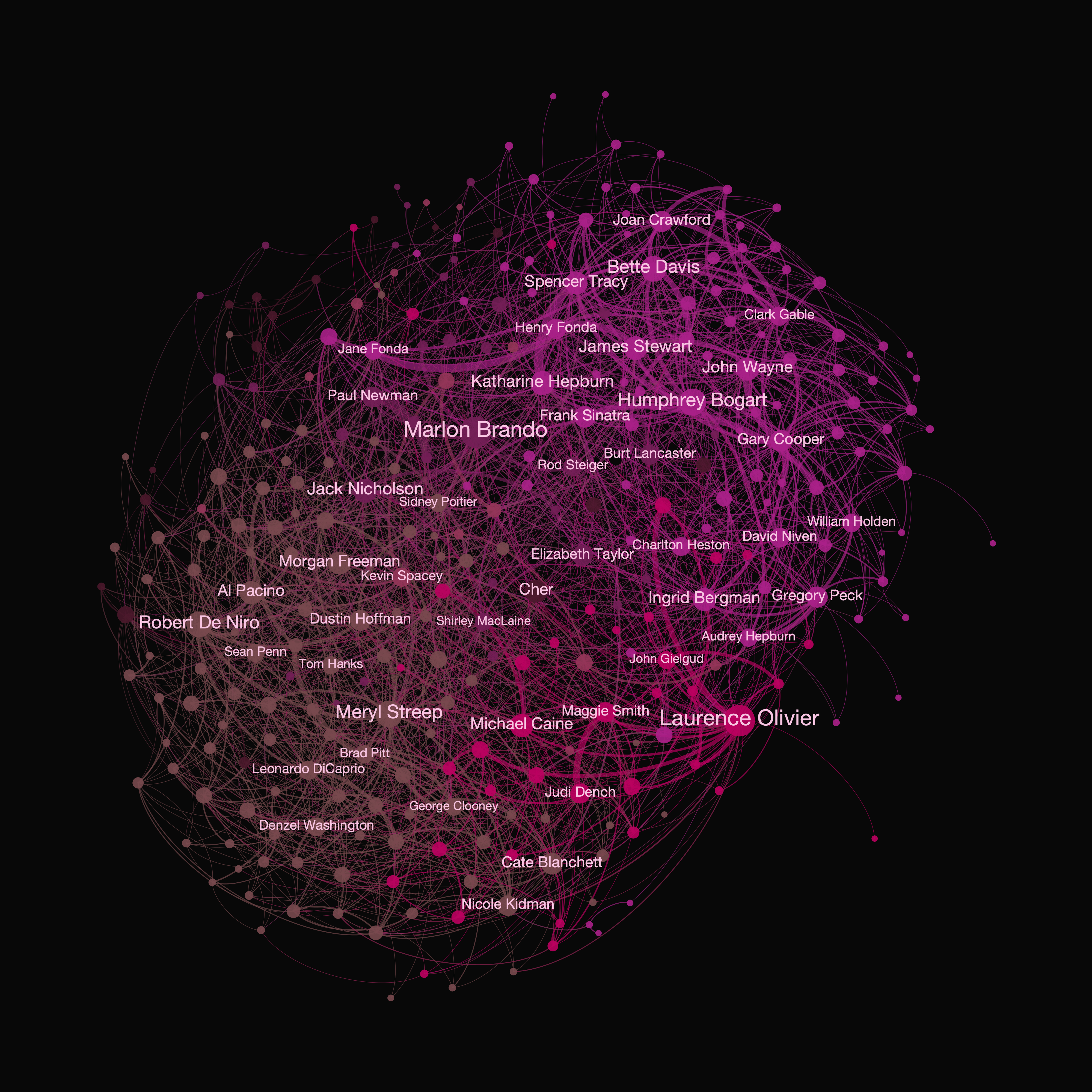